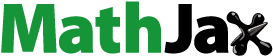
Abstract
This study investigates the effects of digital finance technology, i.e., mobile phones and internet usage on financial stability of the banking sector in developing market economies. We used data from 2000 to 2020 from 55 selected developing economies to examine the link between digital finance technology and financial stability. According to the empirical results from the quantile regression, mobile phone subscriptions as a proxy for digital finance technology has a negative impact on the financial stability of the chosen emerging economies. In contrast, the findings indicate that internet usage as a proxy for digital finance technology has a favorable impact on the financial stability (FS) of developing economies. Given that digital finance technology has a variety of implications on the financial stability of emerging countries, the study advises policymakers to assess current digital finance technology (internet usage and mobile phone subscription) and banking-related regulations and align them with various aspects of financial stability.
1. Introduction
The financial sector is regarded as a crucial element of the economic system. By the financial intermediation process, this industry can accelerate the capital mobilization for high-yielding projects and guarantee the effective distribution of financial resources between borrowers (deficit units) and lenders (surplus units). For instance, banks are important for long-term economic growth and are considered as a primary driving force in the current economic system (Babar et al., Citation2019). In addition, the banking sector provides operating and investment capital to all economic sectors, therefore any disturbance in this sector has an impact on all of the economic sectors in a nation. Financial intermediaries, particularly banks, have been claimed to be crucial to financial stability (Saha & Dutta, Citation2020). As a result, governments, regulators, and policymakers around the world have made maintaining the financial stability of the banking sector a priority because it is essential to the socioeconomic development of the global economy (Arun & Kamath, Citation2015). Without a doubt, during the past few decades, authorities have become more diligent and committed to fostering financial stability (Board, Citation2011; Čihák et al. Citation2016). Not surprisingly, given the impact of the 2007–2008 financial crisis, there is a call for financial stability. This is justified by the fact that a stable financial environment is better equipped to manage financial risks that are inherent in the financial environment and minimize negative price fluctuations of real or financial assets that could endanger monetary resilience (Ahmad, Citation2018). Hence, promoting and enhancing financial stability cannot be overemphasised, and in order to maintain it, it is necessary to examine the specific elements of the financial architecture that could result in development or destruction, particularly in terms of digital finance technology advancements and diffusion. There is so much when it comes to diffusion of digital finance technology across the world economies and the pace at which the populace are adopting them has been very astronomical. For instance, by the end of 2015, there were 3.2 billion internet users worldwide, up from 1 billion in 2005, and mobile phone penetration is already at 98% in developed countries and 80% in emerging economies. In addition, the number of people using mobile phones has steadily increased, from 1 to 10 people out of every 100 doing so between 2005 and 2015 (World Bank, Citation2018). With more people having access to the internet and mobile devices, financial services may become more accessible, which would improve financial inclusivity.
The internet and mobile technological facilities may make it easier for people to instantly access many different forms of financial services including payments, savings, credit, and even investments. That is, mobile phone penetration and internet usage can influence access to financial services, and largely, financial inclusion is increased (Lenka & Barik, Citation2018). This expansion of financial access could have an immediate impact on the stability of the financial system (Utari et al., Citation2012). For example, Pazarbasioglu and Mora (Citation2020) argue that as digital finance technology develops, more people are using formal banking, which lowers market risk and promotes the stability of the financial sector. The fact that digital finance technology supports the development of the financial industry, however, also opens the door for systematic risks. For instance, according to Ozili (Citation2018), digital finance technology does not only accelerate the pace of financial inclusion but also carries some risks. Vives (Citation2016) corroborated this by arguing that increased usage of digital finance technology increases the likelihood of digital risks like data theft and payment system disruption, and easy access to credit contributes to an increase in nonperforming loans (Vives, Citation2016). According to Berger and Mester (Citation2003), technology enables banks to gather and sort borrowers more effectively. Hence, more information sharing could reduce the likelihood of banks insolvency and reduce instability. On the other hand, Beck et al. (Citation2015) demonstrate a negative impact of financial innovation on the banking industry in terms of both performance and risk. This notwithstanding, the effect of digital finance technology diffusion on the banking industry is still an open question (Del Gaudio et al., Citation2021).
Moreover, it is a global problem to provide basic financial services to both disadvantaged populations and small businesses “at an affordable cost in a fair and transparent manner” (Irving Fisher Committee, Citation2015) without sacrificing financial stability (Dittus & Klein, Citation2011). This is an indication that increasing financial inclusion in an economy through digital finance technologies like internet and mobile phones has the tendency of causing banking instability or otherwise. Due to financial innovations, consumer demands and preferences are changing, which could jeopardize or have an impact on traditional financial services (FSB, Citation2017). In this instance, digital finance technology (DFT) is seen as a vital link between financial inclusion and stability of the banking system. However, a lot of empirical study have focused on the impact that digital finance technology (such as mobile phones subscription and internet usage) has on financial inclusion (FI). The question of whether these technological platforms influence financial stability has not received much attention. However, in order to take appropriate decisions as policymakers and regulators try to promote the use of digital finance technology, such as mobile phones and the internet, a deeper understanding of the relationship between these variables is necessary. This study therefore explores the relationship between financial stability and digital finance in developing economies.
The contributions of this study are summarised as follows. Firstly, in developing nations, this is among the few empirical studies that attempt to combine digital finance technologies with the financial stability. Uniquely, when examining individual variables’ roles in the financial stability, this study addressed digital finance technologies in two separate categories: mobile phone subscription and internet usage. In addition, focusing on developing nations is necessary because of the current phenomenal growth of digital finance technologies, particularly the penetration of mobile phones, as well as the underdeveloped physical financial infrastructure in these nations. In order to conduct a thorough and in-depth study in the quest to understand the impact of digital finance technology on financial stability, empirically examining the concept of financial stability in the context of developing countries using MMQREG is a great way to proceed. In addition, the findings of this study contributes to sparse and still-emerging body of research that looks at how digital finance technologies affect the stability of the banking system. The findings of the study can deepen our understanding of the relationship between digital finance technology and the stability of the financial system, as well as assist regulators in that understanding. Lastly, the study is distinct from Syed et al. (Citation2021) who measured financial stability with Bank Z-score ignoring its multidimensional nature in assessing the impact of digital finance on financial stability. However, unlike Ozili (Citation2018) who carried out a theoretical assessment of the connection between digital finance and financial stability, this study conduct empirical investigation to test the relationship. The rest of the paper is organized as follows. Section 2 summarizes the related literature. The methodology is presented in section 3 and 4 covers findings and discussions. The conclusions and recommendations as well as limitations and suggestions for further studies are presented in section 5 and 6 respectively.
2. Literature review
Banks have begun and intensified efforts to broaden the scope of their current offerings to new consumer segments or develop a wholly new financial products and services to cater for such clients in the advent of increased technological diffusion. This is one of the banks’ responses to the entry of non-traditional financial service providers who are mainly using digital finance platforms such as the internet and mobile phones in providing banking services. This in a way can push the traditional banks into areas that they do not have the expertise or possibly end up dealing with noncredit worthy customers and subsequently endangering the stability of the banks. The implementation of digital finance in the banking sector especially in developing economies raises the question of whether or not it can be a solution for achieving banking stability in light of both the positive and/or negative effects of financial inclusion on financial stability. Researchers have therefore empirically examined the impact that the diffusion of digital finance technology has on the banking industry (Del Gaudio et al., Citation2021), indicating that there is a connection between digital finance technology and the banking sector, particularly in terms of financial stability and accessibility. Mobile banking, which enables users to obtain credit and conduct transactions, is available to mobile device users. Del Gaudio et al. (Citation2021) analyze the relationship between digital finance technology (ICT diffusion) and banking sector features in terms of stability using data from the country level in the EU 28 banking industry from 1995 to 2015. The findings imply that ICT advancements enhance the banking industry’s performance with reference to the shift to digital payment services. According to them, adoption of digital finance technology (ICT adoption) and country-level bank stability are positively correlated. That is, digital finance infrastructure and diffusion in particular have a positive and significant effect on banking stability. According to DeYoung (Citation2005), an internet delivery channel may produce advantageous economies of scale that can compete with traditional distribution techniques. Using 60 Europeans banks, Delgado et al. (Citation2007) studied whether the European primarily internet banks show scale and experience efficiencies or not and the findings of the study was that scale efficiencies based on technology are present in European Internet banks, and that as Internet banks get larger, the profitability gap with traditional banks closes. Additionally, the impact of digital finance on financial stability is beneficial and aids banks in lending, extending the reach of financing, and assisting consumers in switching from cash to non-cash payments. The implementation of network-based technology infrastructure and a set of standards for network tele-communication (SWIFT) has a significant impact on banking performance in 6848 banks in 29 countries in Europe and the US, according to research by Scott et al. (Citation2017). This is an indication that in the midst of proper standards and appropriate infrastructure, the banking sector stands to gain and the findings of Demirgüç-Kunt et al. (Citation2008) and Djankov et al. (Citation2007) shows that improved information availability and open contract enforcement have been found to strengthen the financial system, particularly in low-income nations.
Another study by Banna and Alam (Citation2021) using 153 Islamic banks from 32 countries during the period 2011 to 2017 demonstrates that financial inclusion after the global financial crunch played a significant role in promoting banking efficiency. In this line, it can be said that in order for the banking industry to keep up with the competitive world and ensure banking stability as well as promote sustainable economic development, digital finance should be implemented. Additionally, it guarantees accurate recording and supervision of financial transactions (Hannig & Jansen, Citation2010; Morgan & Pontines, Citation2014; Pazarbasioglu & Mora, Citation2020; Pazarbasioglu et al., Citation2020). The growth of digital financial services raises the proportion of the population who use formal banking, which reduces market risk and encourages financial sector stability (Pazarbasioglu & Mora, Citation2020). Similarly, the advancement of fintech technologies benefits the banking industry and encourages financial deregulation in developing nations (Scott et al., Citation2017).
On the other hand, numerous studies, including the findings of , Buchak et al. (Citation2018), Tang (Citation2019), Nugroho et al. (Citation2020) and others, demonstrate negative effects of digital finance, particularly fintech and peer-to-peer lending, which disrupt banking performance and financial stability. Thus digital finance, while aiding in the expansion of the financial sector, also presents opportunities for systematic dangers. Diffusion of digital finance technologies may potentially have a detrimental impact on banking risk. In fact, the widespread use of telecommunications technologies and associated e-banking capabilities may alter a bank’s total risk. Particularly, after the deployment of e-banking activities, various risks associated with traditional banking, such as those related to strategy, operations, law, and reputation, have become more severe. Authorities now understand how crucial it is to manage digital technology diffusion and related risk. This necessitates a proactive strategy, emphasising the significance of integrating security with company operations to achieve successful results (Board, Citation2017; Lessambo, Citation2023). A number of factors, such as institutional connections, financial market connectivity, macroeconomic conditions, and the size of the financial sector, can result in systemic or commercial risks (Hodula & Pfeifer, Citation2018). The fact that various countries may have different macroeconomic and banking industry conditions, it is imperative to assess the impact on financial stability with special attention.
Ozili (Citation2018) concluded that, despite speeding up the path of financial inclusion, there are some risks associated with digital finance. For example, easy access to credit contributes to an increase in nonperforming loans, and excessive use of fintech technology raises the danger of digital risks including data theft and payment system disruption (Vives, Citation2016). Systematic risk can be worsened by the absence of prompt and strict regulatory procedures, particularly in poor nations (Ketterer, Citation2017). Additionally, García and José (Citation2016) pointed out that new financial outreach operations have a higher systematic risk because they are not well regulated. In a similar vein, Khan (Citation2011) emphasized that organizations using the business correspondent structure of financial inclusion are more vulnerable to compliance and strategic risks. It should be emphasized that an excessive use of digital platforms and payments can cause unethical activity to increase and the financial sector to become more unstable. Digital finance technology supports financial sector expansion, but this growth also brings with it the potential for systemic risks. This implies that in an environment where there is so much diffusion of mobile phone and internet for financial transactions without appropriate regulation may lead to financial instability.
A detailed examination of the empirical literature revealed that the connection between digital finance technologies and financial stability of the banking sector has been examined but scanty particularly in developing countries. Besides, the findings of existing literature are conflicting concerning the role that digital finance technologies play in the banking sector and how it might support financial stability. Hence, there is still much debate in the literature on how digital finance technologies including mobile phones and internet affect the banking sector and how it might help maintain financial stability (Del Gaudio et al., Citation2021). Premised on this, further research is needed to supplement the scant conversation on digital finance technologies and stability of the banking system particularly in the context of developing countries where there is a massive usage of mobile and internet financial services. Most significantly, we observed that no research has been conducted by employing a heterogeneous estimator such as MMQREG and employing a composite index for financial stability in investigating the link. By investigating the nexus between digital finance technologies and financial stability of the banking sector in developing countries, we aimed to formulate significant policy recommendations in this study in light of the aforementioned objectives. Based on the above review of literature, the study hypothesised that:
H1:
Mobile phone subscription has a significant positive effect on the financial stability of the banking system of developing countries.
H2:
Internet Usage has a significant positive effect on the financial stability of the banking system of developing countries.
3. Data, model, and methodology
3.1. Description and source of data
This study examined the connection between Digital Finance Technology and Financial Stability by using annual data from 2000 to 2020 from 55 developing countries. The developing countries were selected based on World Bank’s classification and the period is limited to 2000 to 2020 based on data availability for a country. Financial stability, which is the main variable of interest in the current study is considered as multi-dimensional in nature and it has been proposed that if a concept is complex and multi-dimensional in nature, then the most appropriate measure would be a composite construct or index (Engel & Schutt, Citation2012; Sarma, Citation2008, Citation2016). Based on that, this current study followed existing studies that operationalized FS as index (see Albulescu et al., Citation2013; Cheang & Choy, Citation2011; Ertugrul et al., Citation2019; Jadoon et al., Citation2021; Karanovic & Karanovic, Citation2015; Nasreen et al., Citation2017). Therefore, the current study developed an index for Financial Stability (FS) using principal component analysis (PCA). Again, the usage of mobile phone and internet services for online payments in developing economies encourages financial literacy, which can promote financial development and stability (Sapovadia, Citation2018). For instance, Evans (Citation2018) stated that there is excessive usage of internet and mobile services, thereby increasing the level of financial inclusion in the African region (Syed et al., Citation2021). Mobile cellular subscription per 100 people and internet usage, according to Ismael and Ali (Citation2021), can serve as proxies for digital infrastructure. Additionally, World Bank (Citation2017), argue that mobile phones can potentially open access to mobile money accounts while internet is seen as a means of digital payments or make an online purchase. Therefore, following the work of (Agyekum et al., Citation2016; Andrianaivo & Kpodar, Citation2012; Awan et al., Citation2022; Bayar et al., Citation2021; Del Gaudio et al., Citation2021; Haider, Citation2018; Ismael & Ali, Citation2021), digital finance technology is represented by mobile phone subscription and internet usage separately in this study. In terms of data source, data for the financial stability index (FS), bank concentration, and financial development are collected from the World Bank’s Global Financial Development Database and Financial Development Index Database of IMF, respectively. Last but not least, data for mobile phone subscription, internet usage, and trade openness GDPPC, and are gathered from the Global Development Indicators (WDI) of the World Bank. This current study used panel data from 55 selected developing economies and the definition, source, and other details about the data are displayed in Table .
Table 1. Variables, variable Description and data sources
3.2. Specifications of models
In order to achieve its goals, the study proposed the following structure for the framework of the relationship between financial stability and digital finance technology. The financial stability (FS) as a dependent variable, the digital finance technology variables (mobile phone subscription and internet usage) as independent variables, and the control variables (bank concentration, economic growth, financial development, and trade openness as control variables are the important elements involved in the model formulation. The following econometric model is suggested based on the aforementioned assumptions:
stands for MMQREG parameters of the τth distributional point, τ denotes distributional point of the independent variables as ατ represents fixed effects. The dependent variable is financial stability (FS), and the independent variables are mobile phone subscription (MOB) and internet use (INTUSE). Banking concentration, economic growth, financial development, and trade openness are the four control variables designated as CON, GDPPC, FD, and TO. To obtain comprehensive results from the quantile regression estimations, four different quantiles—the 25th, 50th, 75th, and 90th—are used.
4. Methodology
4.1. Crosssectional Dependence (CSD), Slope Homogeneity (SH) & unit root
The econometric methods used in this study are appropriate for the data’s characteristics. A poor technique will produce unreliable and erratic results. To avoid these effects, this study employs the CD, SH, and unit root tests from (Pesaran, Citation2004, Citation2015; Pesaran & Yamagata, Citation2008, Citation2008), and (Pesaran, Citation2007). The CD has gained popularity as a result of the macro- and microeconomic factors’ spillover effects. In addition to the CD and SH tests, data integration qualities are necessary. The unit root test selection is influenced by the presence of CD and SH. Second-generation testing are preferred around CD and SH. As a result, our inquiry made use of CIPS and CADF (Pesaran, Citation2007). Both tests adjust for CD and heterogeneity.
4.2. Cointegration
It is necessary to ascertain whether variables have a long-run agreement when they have the same integration order, such as I (1) when the variables are related. To assess the potential existence of a cointegrating relationship between these variables, we employ the Westerlund test. This test is designed to examine the null hypothesis of no cointegration, while the alternative hypothesis suggests that all panels demonstrate cointegration. This test is based on a model in which the autoregressive (AR) parameter remains consistent across all panels.
4.3. Coefficient estimation
The study used a heterogeneous panel data model to handle differences within the chosen economies. In order to assess the heterogeneous and distributional effects across quantiles among the variables utilized in the investigation, this study used the MMQREG model. The economic variable sequence does not have to follow a normal distribution when using panel quantile regression (Liu et al., Citation2021; Xu & Lin, Citation2020). Even though traditional panel quantile techniques (Canay, Citation2011; Koenker, Citation2004) are accurate and reliable estimates in the presence of outliers, heteroskedasticity (Zhu et al., Citation2018), and when the conditional means of two variables are weakly correlated, they are unable to detect the likely existence of unnoticed heterogeneity across the cross sections of the panel data. With the advantage of capturing any asymmetric effect between the variables, it illustrates the relationship between the variables through the quantiles. It can withstand unusual errors and outliers as well. The MMQREG technique necessarily examines the conditional heterogeneous covariance effect of financial stability determinants on the entire distribution by allowing the individual fixed effects. As a result, the models are estimated using the MMQREG command, which has the advantage of taking the panel’s fixed effect structure into consideration. Moreover, unlike (Canay, Citation2011; Koenker, Citation2004), MMQREG captures the covariance impact in the whole distribution rather than shifting the means. This approach also handles the potential presence of endogenous characteristics in the explanatory variables (Awan et al., Citation2022), which is a benefit.
The technique is also appropriate in instances where individual effects overrides the panel data model and it produces reliable estimates when it comes to non-linear model. Furthermore, MMQR permits asymmetries based on location and can provide enormous bits of information when it comes to creating non-crossing estimates in quantile regression (Chernozhukov et al., Citation2010; Rios-Avila, Citation2020). Based on this, the study used the Machado and Santos Silva quantiles-via-moments estimator (2019). The approach considers calculating the conditional quantiles of the subsequent location-scale ( model:
with . Z is a k-vector of known variables in X mechanisms, and (αi,δi) are the parameters that capture each fixed effect separately. In addition to being independent across t, i.i.d. for any fixed I, statistically independent of, and streamlined to suit the needs of the moment, the sequence is also independent. However, the model provided in EquationEquation 1
(1)
(1) has problems with incidental parameters and, in this case, has no advantages over alternative methods.
When the scalar coefficient is a quantile-τ to the fixed effect or the distribution effect to the individual i, the propagation effect differs from the ordinary fixed effect in that there is typically no change in position. Put differently, the influence of the distribution indicates the effects of each invariant time characteristic, which, like other variables, can have range of consequences depending on each region of the conditional distribution of Y.
might be interpreted as the mean result for separate i. Hence, the jackknife adjustment in EquationEquation 2
(2)
(2) essentially eliminates the bias that would otherwise result in a significant loss of accuracy (Machado & Santos Silva, Citation2019). For the purpose of dealing with endogeneity problems, the study employed this dynamic panel data estimator—panel quantile regression with fixed effects (MMQREG), which gives more accurate, efficient, and reliable results. This approach helps in handling endogeneity concerns as it incorporates fixed effects (Awan et al., Citation2022; Rao et al., Citation2023). That is, the estimator is known for its reliability and robustness in handling endogeneity problems (Ntarmah et al., Citation2020). Generally, it enables the correction of problems with endogeneity and heterogeneity, such as reverse causality, during the estimation process. That is, endogeneity problems are lessened because the conditions in EquationEquation 2
(2)
(2) above do not require strict exogeneity (Awan et al., Citation2022; Ntarmah et al., Citation2020). Moreover, control variables were also added to improve statistical precision, address endogeneity problems, and lessen model uncertainty.
5. Results and discussions
Table shows that 1155 observations were used in this study. Since each of the variables had 1155 observations, the data utilized in the study are balanced panel data (N = 55, T = 21). The mean values for FS were 7.66e-08, whereas those for MOB, INTUSE, CON, GDPPC, FD, and TO were 76.708, 30.607, 66.637, 2.405, 0.282, and 84.738, respectively. With respect to minimum values, FS had −1.713, MOB had 0.255 and INTUSE had 0.047; the corresponding maximum values were 6.539, 5.534, 212.639, and 100. MOB had the largest standard deviation of 49.212 among the key variables. For the control variables, GDPPC had the lowest value of −21.116 and TO had the highest value of 322.677 with a standard deviation of 45.223.
Table 2. Descriptive & correlation analysis
Table displays the correlation between the variables of the study. The findings indicate that, with the exception of GDPPC, all explanatory variables correlate with the outcome variable, suggesting a relationship between the dependent variable and all independent variables, such as internet usage and mobile phone subscription. Digital finance technology proxies: mobile phone and internet usage are inversely related to the outcome variable. The negative relationship with the outcome variable may be that the developing countries are not appropriately utilizing these platforms in a way that will provide support for the enhancement of financial stability of the banking sector. This provides support for research on how the use of mobile and internet technology in finance affects the stability of the financial system. However, there is criticism of the Pearson correlation evaluation (Appiah et al., Citation2023), hence the need to conduct additional econometric analysis using Method of Moment Quantile Regression (MMQREG) estimator, which is the main estimation method in this study.
Table 3. Correlation matrix
To ascertain whether there is multicollinearity among the explanatory variables, the study performed a multicollinearity test, and the findings are shown in Table . The findings indicate that none of the variables’ VIF values are greater than 10. The results also indicate that each explanatory variable’s tolerance value exceeds the minimum threshold of 0.2. According to the usual rule, a tolerance level of 0.2 and a VIF value of less than 10 indicate the absence of multicollinearity. The study can draw the conclusion that there is no problem with multicollinearity among the explanatory factors based on the results.
Table 4. Multicollinearity test
The empirical analysis began with cross-section dependence tests and according to Table , the CD test results show that “no cross-section dependence” is rejected for all factors and the study concludes that there is a cross sectional dependence in existence. This implies that a shock could spread to other countries from one of the sample countries. The findings regarding slope homogeneity rejects the invalid notion of homogeneous variables and accepts the alternative viewpoint. As a result, unit root-stationarity methods that operate under the assumption of cross-section dependency can be used to determine the stationarity level of the variables, in contrast to panel data estimating techniques that work under the postulation of cross-section independence (Hurlin, Citation2010; Pesaran, Citation2004). The tests of CIPS and CADF applications were utilized to overcome the problem of cross-sectional dependence and eliminate the problem of homogeneity (Appiah et al., Citation2021), resulting in the stationary results being more reliable.
Table 5. Pre-diagnostic tests
Taking into consideration cross-section dependence, we employed robust-based panel unit root tests (Pesaran, Citation2004) and the results is reported in Table . The outcomes show that the FS, INTUSE, and TO variables in the CIPS model have a non-stationary process at the level. However, all the variables at the first difference exhibit a stationary process. In the CADF test, the variables FS, FD and TO are non-stationary while the remaining variables are stable. All variables were stationary after applying the first difference estimation, proving that the variables under consideration have an I (0) and I (1) integration order. Hence, the long-run relationship between variables was tested using the panel long-term test established by (Pedroni, Citation2004; Westerlund, Citation2005).
Table 6. Panel unit root outcomes
Table displays the cointegration test results from Westerlund (Citation2005). The VR statistics provide evidence to reject the null hypothesis of no cointegration, indicating that all panels exhibit cointegration. Overall, when considering a 5% significance level, the statistical tests consistently reject the null hypothesis of no cointegration. Therefore, we have identified cointegration relationships between financial stability and the variables, namely mobile phone subscription, internet usage, bank concentration, financial development, economic growth, and trade openness. These analyzed variables demonstrate a synchronized movement in the long run, indicating a long-term relationship across all countries in the sample. The Pedroni cointegration test results as shown in Table affirms the cointegration or long-run relationship between the variables.
Table 7. Westerlund cointegration outcomes
Table 8. Pedroni cointegration outcomes
Table shows that, digital finance technology, as measured by mobile phone subscriptions, has a negative impact on the financial stability of developing nations. The results from the Pooled OLS indicates that a percentage increase in mobile phone subscription will result in a 0.007% decline in financial stability (FS). The MMQREG results show that for developing countries distributed along the low and moderate quantiles (25% and 50%) and high and very high quantiles (75% and 90%), a percentage increase in mobile phone subscription will result in a decrease in financial stability by 0.003%, 0.006%, 0.009%, and 0.014%, respectively. This shows that the goals of financial stability are not being met by strategies to increase the use of digital financial technology (mobile phones) in the banking sector and the economy as a whole in the developing world. Thus, efforts by the developing countries to digitalize their economies, particularly their banking sector, and to make transactions easier and more efficient on digital platforms like mobile phones end up posing a danger to the stability of the banking sector. This result is consistent with the findings of Ozili (Citation2018), Vives (Citation2019), who argue that using fintech technology excessively increases digital risk, and Ketterer (Citation2017), who said that the lack of prompt and strict regulatory processes exacerbates systematic risk, brought on by digital finance technology, particularly in developing countries. Moreover, the study corroborates Syed et al. (Citation2021) who draw the conclusion that among a chosen sample of Asian countries, an increase in digital finance raises the chance of systematic risks and increases financial instability.
Table 9. Results of the impact of digital finance technology on financial stability
On the other hand, the findings indicate that internet use as a proxy for digital finance technology has a positive impact on the financial stability (FS) of developing economies. For developing economies distributed along low and moderate quantiles (25th and 50th) and high and very high quantiles (75th and 90th), a 1% increase in internet usage will increase financial stability (FS) by 0.005%, respectively, according to the POLS results while the MMQREG results show that a 1% increase in internet usage will increase FS by 0.002%, 0.004%, 0.007%, and 0.011%.
The use of the internet, as opposed to mobile phone subscriptions, improves the financial stability of developing economies. This indicates that developing economies are more financially stable when internet usage or diffusion is higher. These findings support the arguments put forth by earlier authors who have stressed the advantages of financial technology development for the stability of the financial industry. For instance, research by Morgan and Pontines (Citation2014) and Hannig and Jansen (Citation2010) indicated that digital finance provides better risk management strategies that lower systemic risk and financial sector instability. However, the findings are contrary to that of Syed et al. (Citation2021) who concluded that increased use of digital finance among a sample of Asian countries increases the likelihood of systemic hazards and financial instability.
The results show that trade openness, financial development, and banking concentration are some of the key elements affecting financial stability in developing nations. The findings suggest that bank concentration in emerging market nations does not undermine financial stability but rather strengthens it as it shows a positive association with financial stability. The study’s findings corroborate empirical research by Beck et al. (Citation2006), which showed that increased banking industry concentration did not increase banking fragility. Once more, it confirms the findings of Allen and Gale (Citation2004) that financial stability is higher in a concentrated banking sector with a small number of large, easily observable institutions. The results, however, contradict that of Berger et al. (Citation2009), who show that increasing market dominance has a detrimental effect on the risk of bank portfolios.
The findings also show that the financial stability of developing nations was negatively and significantly impacted by financial development. The results of this study contradict those of Demirgüç-Kunt and Detragiache (Citation1999, Citation2005), who claim that the level of the credit-to-GDP ratio and the rate of credit growth have a strong beneficial influence on the occurrence of bank instability. It does, however, concur with Sahay et al. (Citation2015), who discovered that financial development has a negative influence on financial stability and stated that when the financial system is poorly governed and overseen, a higher rate of financial depth increases financial instability. Finally, according to the findings, trade openness had a positive and significant impact on the financial stability of developing nations. This study confirms that of Alamgir Hossain et al. (Citation2020), who looked at the relationship between trade openness and bank risk-taking behavior across Bangladeshi banks and found that there was a negative relationship between the two. On the other hand, other studies (Bourgain et al., Citation2012; Cubillas & González, Citation2014) that examined the micro-level impacts of financial openness on bank risk-taking behavior discovered a direct association between financial openness and bank risk-taking.
For the purposes of checking robustness of the results and to incorporate the long-run relation among the variables, the study employed FMOLS, a tool that is dependable and effective for panel cointegration research. Table gives the results of the FMOLS and it is evident from the results that they are comparable to the MMQREG results in Table . Comparatively, the magnitude and marginal impacts are similar, supporting the validity of the findings.
Table 10. Fully Modified least squares estimator as robustness
6. Conclusions and policy recommendation
This study examined the impact of digital finance technologies: mobile phone subscription and internet usage on financial stability of banking sector in developing counties among selected over the period 2000–2020. We used 55 selected developing countries in this study to examine the links between digital finance technology and financial stability by applying Methods of Moment Quantile Regression (MMQREG). According to the empirical results from the quantile regression, mobile phone subscriptions as a proxy for digital finance have a detrimental impact on the financial stability of the chosen emerging economies. Overall, we conclude that conditioning on other financial stability determinants, digital finance represented by mobile phone subscription has an adverse impact on financial stability among the selected developing countries. In other words, a rise in mobile phone subscriptions causes developing economies’ financial stability to decline. The implication is that, these countries’ use of digital financial technology by way of mobile phones subscription does not promote the stability of the banking industry, which creates instability. This supports the digital finance diffusion and instability nexus. In contrast, the findings indicate that internet usage as a proxy for digital finance has a positive impact on the financial stability (FS) of developing economies. We conclude that internet usage is favorable to the financial stability of the selected developing countries. The contradicting findings of how mobile phone subscription and internet usage individually affects financial stability implies that there are several different ways in which financial stability is impacted. In developing countries, a large number people who use mobile phones as a means of accessing financial services are generally poor and not credit worthy and that may explain the negative impact of mobile phones on stability of the banking sector in these countries.
The following policy recommendations are provided based on the aforementioned findings. First, we recommend that the developing countries must revisit their digital finance policies in order to properly align the use of mobile phones in line with financial services and ultimately, financial stability of their banking system. Given that digital finance technology has a variety of implications on the financial stability of emerging countries, the study advises policymakers to assess current digital finance platforms (internet usage and mobile phone subscription specifically) and banking-related regulations and put in place regulations that will check banks and customers in adopting digital finance technologies. Particularly, to determine how they will improve the soundness of the banking system, policies, and initiatives relating to banking and digital finance technology should be evaluated against a number of different aspects of financial stability. It is possible that more loans are being accessed through mobile phones without proper credit assessment and hence causing instability. Therefore, checking policies and the routes through which the unbanked people get into the financial web via digital finance technologies must be checked. The study also recommends that the selected countries maintain or improve upon whatever policies that is yielding positive impact of internet usage on financial stability.
7. Limitations and suggestion for future research
To our knowledge, this study is among the few that considers digital finance technologies: combining mobile phones subscription and internet usage in the context of financial stability of developing countries. The study however, does not include the entire developing countries due to unavailability of data. Moreover, the study period is limited by data availability especially in terms of data pertaining to financial stability indicators. Lastly, regional economies have worked to improve financial stability outcomes as well as the digitisation process hence the impact of digital finance technologies on financial stability in developing economies may differ between regional economies. However, this current study did not focus on the regional groupings. Therefore, future studies may consider more in-depth research, which will consider the regional groupings. In addition, individual countries can be studied to know the impact of digital finance technologies on banking stability of a specific country. Upon availability of data, future studies can extend the study period to allow for more long-term relationship analysis. Lastly, the Method of Moments Quantile Regression (MMQREG) was utilized in this study. Therefore, future studies may employ time series threshold model as well as the dynamic threshold model in determining the potential threshold of particular nations and regions.
About the corresponding author.docx
Download MS Word (13.5 KB)Disclosure statement
No potential conflict of interest was reported by the author(s).
Supplementary data
Supplemental data for this article can be accessed online at https://doi.org/10.1080/23311975.2023.2284738
Additional information
Funding
Notes on contributors
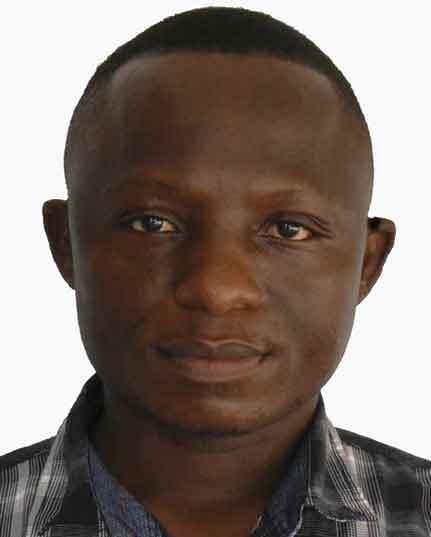
Frank Antwi
Frank Antwi has a bachelor’s degree in Banking and Finance from Pentecost University and MPhil in Business Administration (Risk Management and Insurance option) from the University of Ghana. Frank is currently pursuing PhD in Management Science (Finance option) at Jiangsu University in the People’s Republic of China and also a lecturer at the Department of Accounting and Finance, Faculty of Business Administration, Pentecost University, Accra-Ghana. Frank has been a full-time faculty member at Pentecost University since 2013. He has published a number of papers in peer-reviewed journals and his focus is on financial performance, related party transactions, financial inclusion, sustainable finance, stability, risk management and entrepreneurship. The current study has a link with other studies being conducted in the area of financial inclusion, digital finance technology and stability. The focus is on developing economies as they still have a chunk of their population being unbanked.
References
- Agyekum, F., Locke, S., & Hewa-Wellalage, N. (2016). Financial inclusion and digital financial services: empirical evidence from Ghana. Munich Personal RePec Archive. MPRA Paper No. 82885. https://mpra.ub.uni-muenchen.de/82885/
- Ahmad, D. (2018). Financial inclusion and financial stability: Survey of the Nigeria’s financial system. International Journal of Research in Finance and Management, 1(2), 47–17. https://doi.org/10.33545/26175754.2018.v1.i2a.15
- Alamgir Hossain, S., Moudud-Ul-Huq, S., Kader, M. B., & McMillan, D. (2020). Impact of trade openness on bank risk-taking behavior: Evidence from a developing country. Cogent Economics & Finance, 8(1), 1765468. https://doi.org/10.1080/23322039.2020.1765468
- Albulescu, C. T., Goyeau, D., & Pépin, D. (2013). Financial instability and ECB monetary policy. Economics Bulletin, 33(1), 388–400.
- Allen, F. & Gale, D. (2004). Competition and financial stability. Journal of Money, Credit, and Banking, 453–480.
- Andrianaivo, M., & Kpodar, K. (2012). Mobile phones, financial inclusion, and growth. Review of Economics and Institutions, 3(2), 1–29. https://doi.org/10.5202/rei.v3i2.75
- Appiah, M., Li, F., & Korankye, B. (2021). Modeling the linkages among CO 2 emission, energy consumption, and industrialization in Sub-Saharan African (SSA) countries. Environmental Science and Pollution Research, 28(29), 38506–38521. https://doi.org/10.1007/s11356-021-12412-z
- Appiah, M., Li, M., Naeem, M. A., & Karim, S. (2023). Greening the globe: Uncovering the impact of environmental policy, renewable energy, and innovation on ecological footprint. Technological Forecasting and Social Change, 192, 122561. https://doi.org/10.1016/j.techfore.2023.122561
- Arun, T., & Kamath, R. (2015). Financial inclusion: Policies and practices. IIMB Management Review, 27(4), 267–287. https://doi.org/10.1016/j.iimb.2015.09.004
- Awan, A., Abbasi, K. R., Rej, S., Bandyopadhyay, A., & Lv, K. (2022). The impact of renewable energy, internet use and foreign direct investment on carbon dioxide emissions: A method of moments quantile analysis. Renewable Energy, 189, 454–466. https://doi.org/10.1016/j.renene.2022.03.017
- Babar, S., Latief, R., Ashraf, S., & Nawaz, S. (2019). Financial stability index for the financial sector of Pakistan. Economies, 7(3), 81. https://doi.org/10.3390/economies7030081
- Banna, H., & Alam, M. R. (2021). Impact of digital financial inclusion on ASEAN banking stability: Implications for the post-covid-19 era. Studies in Economics and Finance, 38(2), 504–523. https://doi.org/10.1108/SEF-09-2020-0388
- Bayar, Y., Ozkaya, M. H., Herta, L., & Gavriletea, M. D. (2021). Financial development, financial inclusion and primary energy use: Evidence from the European Union transition economies. Energies, 14(12), 3638. https://doi.org/10.3390/en14123638
- Beck, T., Demirgüç-Kunt, A., & Levine, R. (2006). Bank concentration, competition, and crises: First results. Journal of Banking & Finance, 30(5), 1581–1603. https://doi.org/10.1016/j.jbankfin.2005.05.010
- Beck, T., Senbet, L., & Simbanegavi, W. (2015). Financial inclusion and innovation in Africa: An overview. Journal of African Economies, 24(suppl_1), i3–i11. https://doi.org/10.1093/jae/eju031
- Berger, A. N., Klapper, L. F., & Turk-Ariss, R. (2009). Bank competition and financial stability. Journal of Financial Services Research, 35(2), 99–118. https://doi.org/10.1007/s10693-008-0050-7
- Berger, A. N., & Mester, L. J. (2003). Explaining the dramatic changes in performance of US banks: Technological change, deregulation, and dynamic changes in competition. Journal of Financial Intermediation, 12(1), 57–95. https://doi.org/10.1016/S1042-9573(02)00006-2
- Board, F. S. (2011). International monetary fund, and Bank for International settlements. Macroprudential policy tools and frameworks. Progress Report to G, 20.
- Board, F. S. (2017). Financial stability board. Financial Stability Board.
- Bourgain, A., Pieretti, P., & Zanaj, S. (2012). Financial openness, disclosure and bank risk-taking in MENA countries. Emerging Markets Review, 13(3), 283–300. https://doi.org/10.1016/j.ememar.2012.01.002
- Buchak, G., Matvos, G., Piskorski, T., & Seru, A. (2018). Fintech, regulatory arbitrage, and the rise of shadow banks. Journal of Financial Economics, 130(3), 53–483. https://doi.org/10.1016/j.jfineco.2018.03.011
- Canay, I. A. (2011). A simple approach to quantile regression for panel data. The Econometrics Journal, 14, 368–386. https://doi.org/10.1111/j.1368-423X.2011.00349.x
- Cheang, N., & Choy, I. (2011). Aggregate financial stability index for an early warning system. Macao Monetary Research Bulletin, 21(1), 27–51.
- Chernozhukov, V., Fernandez-Val, I., & Galichon, A. (2010). Quantile and probability curves without crossing. Econometrica, 78(3), 1093–1125.
- Čihák, M., Mare, D. S., & Melecky, M. (2016). The nexus of financial inclusion and financial stability: A study of trade-offs and synergies. World Bank Policy Research Working Paper, 7722.
- Cubillas, E., & González, F. (2014). Financial liberalization and bank risk-taking: International evidence. Journal of Financial Stability, 11, 32–48. https://doi.org/10.1016/j.jfs.2013.11.001
- Delgado, J., Hernando, I., & Nieto, M. J. (2007). Do European primarily internet banks show scale and experience efficiencies? European Financial Management, 13(4), 643–671. https://doi.org/10.1111/j.1468-036X.2007.00377.x
- Del Gaudio, B. L., Porzio, C., Sampagnaro, G., & Verdoliva, V. (2021). How do mobile, internet and ICT diffusion affect the banking industry? An empirical analysis. European Management Journal, 39(3), 327–332. https://doi.org/10.1016/j.emj.2020.07.003
- Demirgüç-Kunt, A., & Detragiache, E. (1999). Monitoring banking sector fragility: A multivariate logit approach with an application to the 1996-97 banking crises. Available at SSRN 620670.
- Demirgüç-Kunt, A., & Detragiache, E. (2005). Cross-country empirical studies of systemic bank distress: A survey. National Institute Economic Review, 192, 68–83. https://doi.org/10.1177/002795010519200108
- Demirgüç-Kunt, A., Detragiache, E., & Tressel, T. (2008). Banking on the principles: Compliance with Basel Core principles and Bank Soundness. Journal of Financial Intermediation, 17(4), 511–542. https://doi.org/10.1016/j.jfi.2007.10.003
- DeYoung, R. (2005). The performance of internet‐based business models: Evidence from the banking industry. The Journal of Business, 78(3), 893–948. https://doi.org/10.1086/429648
- Dittus, P., & Klein, M. U. (2011). On harnessing the potential of financial inclusion BIS Working Papers No 347. Basel. https://doi.org/10.2139/ssrn.1859412.
- Djankov, S., McLiesh, C., & Shleifer, A. (2007). Private credit in 129 countries. Journal of Financial Economics, 84(2), 299–329. https://doi.org/10.1016/j.jfineco.2006.03.004
- Engel, R. J., & Schutt, R. K. (2012). The practice of research in Social work. Sage.
- Ertugrul, H. M., Ozun, A., & Kirikkaleli, D. (2019). How is financial stability impacted by political and economic stabilities in emerging markets? A dynamic panel analysis. Journal for Economic Forecasting, 22(4), 148–159.
- Evans, O. (2018). Connecting the poor: The internet, mobile phones and financial inclusion in Africa. Digital Policy, Regulation & Governance, 20(6), 568–581.
- Financial Stability Board. (2017). Financial stability implications from fintech: Supervisory and regulatory issues that merit authorities’ attention.
- García, M. J. R., & José, M. (2016). Can financial inclusion and financial stability go hand in hand. Economic Issues, 21(2), 81–103.
- Haider, H. (2018). Innovative financial technologies to support livelihoods and economic outcomes. K4D Helpdesk Report. Institute of Development Studies.
- Hannig, A., & Jansen, S. (2010). Financial inclusion and financial stability: Current policy issues. ADBI Working Paper, 259. Asian Development Bank Institute,
- Hodula, M., & Pfeifer, L. (2018). Fiscal-monetary-financial stability interactions in a data-rich environment. Review of Economic Perspectives, 18(3), 195–224. https://doi.org/10.2478/revecp-2018-0012
- Hurlin, C. (2010). What would Nelson and Plosser find had they used panel unit root tests? Applied Economics, 42(12), 1515–1531. https://doi.org/10.1080/00036840701721539
- Irving Fisher Committee. (2015). Proceedings of the workshop on “financial inclusion indicators”, cohosted by the Bank Negara Malaysia, Sasana Kijang, Kuala Lumpur, 5–6 November 2012. IFC Bulletins No. 38, Bank for International Settlements.
- Ismael, D. M., & Ali, S. S. (2021). Measuring digital and traditional financial inclusion in Egypt: A new index. International Journal of Applied Research in Management and Economics, 4(2), 13–34. https://doi.org/10.33422/ijarme.v4i2.629
- Jadoon, I. A., Mumtaz, R., Sheikh, J., Ayub, U., & Tahir, M. (2021). The impact of green growth on financial stability. Journal of Financial Regulation & Compliance, 29(5), 533–560. https://doi.org/10.1108/JFRC-01-2021-0006
- Karanovic, G., & Karanovic, B. (2015). Developing an aggregate index for measuring financial stability in the Balkans. Procedia Economics and Finance, 33, 3–17. https://doi.org/10.1016/S2212-5671(15)01690-1
- Ketterer, J. A. (2017). Digital finance: New times, New challenges, New opportunities. IDB-Inter American Development Bank.
- Khan, H. R. (2011). Financial inclusion and financial stability: Are they two sides of the same coin? Address given at BANCON. Retrieved November 4–6, 2011.
- Koenker, R. (2004). Quantile regression for longitudinal data. Journal of Multivariate Analysis, 91(1), 74–89. https://doi.org/10.1016/j.jmva.2004.05.006
- Lenka, S. K., & Barik, R. (2018). Has expansion of mobile phone and internet use spurred financial inclusion in the SAARC countries? Financial Innovation, 4(1). https://doi.org/10.1186/s40854-018-0089-x
- Lessambo, F. I. (2023). The European banking authority. In M., Philip (Ed.), Fintech regulation and supervision challenges within the banking industry: A comparative study within the G-20 (pp. 73–80). Springer Nature Switzerland.
- Liu, Y., Lin, B., & Xu, B. (2021). Modeling the impact of energy abundance on economic growth and CO2 emissions by quantile regression: Evidence from China. Energy, 227, 120416. https://doi.org/10.1016/j.energy.2021.120416
- Machado, J. A. F., & Santos Silva, J. M. C. (2019). Quantiles via moments. Journal of Econometrics, 213(1), 145–173.
- Morgan, P., & Pontines, V. (2014). Financial stability and financial inclusion. Asian Development Bank Institute Working Paper Series No. 488, Asian Development Bank.
- Nasreen, S., Anwar, S., & Ozturk, I. (2017). Financial stability, energy consumption and environmental quality: Evidence from South Asian economies. Renewable and Sustainable Energy Reviews, 67, 1105–1122. https://doi.org/10.1016/j.rser.2016.09.021
- Ntarmah, A. H., Kong, Y., Cobbinah, E., Gyan, M. K., & Manu, E. K. (2020). Analysis of the responsiveness of environmental sustainability to non-performing loans in Africa. Applied Economics Journal, 27(2), 77–109.
- Nugroho, L., Harnovinsah, P. Y., & Prinoti, P. (2020). Analysis of comparison of Islamic banks with financial technology (fintech) in disbursements of micro-financing based on requirements, services speed and margin. Journal of Islamic Economics & Social Science-JIESS, 1(1), 1–10. https://doi.org/10.22441/jiess.2020.v1i1.001
- Ozili, P. K. (2018). Impact of digital finance on financial inclusion and stability. Borsa Istanbul Review, 18(4), 329–340. https://doi.org/10.1016/j.bir.2017.12.003
- Pazarbasioglu, C., & Mora, A. G. (2020). Expanding digital financial services can help developing economies cope with crisis now and boost growth later. World BankVoices (Blog).
- Pazarbasioglu, C., Mora, A. G., Uttamchandani, M., Natarajan, H., Feyen, E., & Saal, M. (2020). Digital financial services. The World Bank, 54.
- Pedroni, P. (2004). Panel cointegration: Asymptotic and finite sample properties of pooled time series tests with an application to the PPP hypothesis. Econometric Theory, 20(3), 597–625. https://doi.org/10.1017/S0266466604203073
- Pesaran, M. H. (2004). General diagnostic tests for Cross Section dependence in panels; CESifo. Working Paper (No. 1229). Cambridge University,
- Pesaran, M. H. (2007). A simple panel unit root test in the presence of cross‐section dependence. Journal of Applied Econometrics, 22(2), 265–312. https://doi.org/10.1002/jae.951
- Pesaran, M. H. (2015). Testing weak cross-sectional dependence in large panels. Econometric Reviews, 34(6–10), 1089–1117. https://doi.org/10.1080/07474938.2014.956623
- Pesaran, M. H., & Yamagata, T. (2008). Testing slope homogeneity in large panels. Journal of Econometrics, 142(1), 50–93. https://doi.org/10.1016/j.jeconom.2007.05.010
- Rao, A., Dagar, V., Sohag, K., Dagher, L., & Tanin, T. I. (2023). Good for the planet, good for the Wallet: The ESG impact on financial performance in India. Finance Research Letters, 56, 104093. https://doi.org/10.1016/j.frl.2023.104093
- Rios-Avila, F. (2020). Recentered Influence Functions (RIFs) in Stata: RIF regression and RIF decomposition. The Stata Journal, 20(1), 51–94. https://doi.org/10.1177/1536867X20909690
- Saha, M., & Dutta, K. D. (2020). Nexus of financial inclusion, competition, concentration and financial stability: Cross-country empirical evidence. Competitiveness Review: An International Business Journal, 31(4), 669–692. https://doi.org/10.1108/CR-12-2019-0136
- Sahay, M. R., Cihak, M., N’Diaye, M. P., Barajas, M. A., Mitra, M. S., Kyobe, M. A., & Yousefi, M. R. (2015). Financial inclusion: Can it meet multiple macroeconomic goals? International Monetary Fund.
- Sapovadia, V. (2018). Financial inclusion, digital currency, and mobile technology. In Handbook of Blockchain, Digital Finance, and Inclusion (Vol. 2, pp. 361–385). Academic Press.
- Sarma, M. (2008). Index of financial inclusion, Indian Council for Research on International Economic Relations (ICRIER). Working Paper, 2(152), 008.
- Sarma, M. (2016). Measuring financial inclusion for Asian economies, financial inclusion in Asia. Palgrave Macmillan UK.
- Scott, S. V., Van Reenen, J., & Zachariadis, M. (2017). The long-term effect of digital innovation on bank performance: An empirical study of SWIFT adoption in financial services. Research Policy, 46(5), 984–1004. https://doi.org/10.1016/j.respol.2017.03.010
- Syed, A. A., Ahmed, F., Kamal, M. A., & Trinidad Segovia, J. E. (2021). Assessing the role of digital finance on shadow economy and financial instability: An empirical analysis of selected South Asian countries. Mathematics, 9(23), 3018. https://doi.org/10.3390/math9233018)
- Tang, H. (2019). Peer-to-peer lenders versus banks: Substitutes or complements? The Review of Financial Studies, 32(5), 1900–1938. https://doi.org/10.1093/rfs/hhy137
- Utari, G. D., Arimurti, T., & Kurnia, I. N. (2012). Optimal credit growth. Buletin Ekonomi Moneter dan Perbankan, 15(2), 3–34. https://doi.org/10.21098/bemp.v15i2.419
- Vives, X. (2016). Competition and stability in banking. In H., Jill, & P., Hannah (Eds.), Competition and stability in banking (p. 106). Princeton University Press.
- Vives, X. (2019). Digital disruption in banking. Annual Review of Financial Economics, 11, 243–272.
- Westerlund, J. (2005). New simple tests for panel cointegration. Econometric Reviews, 24(3), 297–316. https://doi.org/10.1080/07474930500243019
- World Bank. (2018). Information and communications for development 2018: Data-driven development. The World Bank.
- Xu, B., & Lin, B. (2020). Investigating drivers of CO2 emission in China’s heavy industry: A quantile regression analysis. Energy, 206, 118159. https://doi.org/10.1016/j.energy.2020.118159
- Zhu, X., Li, L., Zhou, K., Zhang, X., & Yang, S. (2018). A meta-analysis on the price elasticity and income elasticity of residential electricity demand. Journal of Cleaner Production, 201, 169–177.