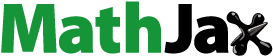
Abstract
Technology, digital advances, and social media are increasingly used to facilitate and influence online consumer purchasing behavior. Growth at the intersection of e-commerce, social networking usage, and functionality has given rise to the social commerce phenomenon. Social commerce sites allow consumers and sellers to engage in two concurrent types of interaction during the buying process. Consumers engage in marketing content from sellers and user-generated content from other consumers. This study investigates how social commerce sites’ combined e-commerce and social networking functionality may impact consumer purchasing behavior. It focuses on seller-generated and user-generated social cues communicated through profile pictures, logos, and other social networking practices (e.g. “follows,” “likes”) and their correlation to retail shop performance indicators on a social commerce site. Using a sample of social and commercial performance indicators from retail shops on “Etsy.com” and a negative binomial regression analysis, we evaluate the effect of social signals on consumer purchasing behavior with a classical Stimulus-Response framework. Findings suggest consumers are more likely to purchase from shops with seller-generated social cues and show greater engagement in the volume of reviews and follows. More importantly, these features also appear to lower the negative impact of the age of an online shop. This finding may be advantageous to new entrants to social commerce or online retail outlets.
1. Introduction
The rise of Web 2.0 transformed e-commerce with its inherent advantages of higher participation and collective intelligence. The growing adoption of social media further accentuates the prospects of making marketplaces more customer-centric with abundant user-generated content and participatory culture (Dabbous et al., Citation2020; Huang & Benyoucef, Citation2013; Vithayathil et al., Citation2020). Social commerce thereby optimizes the social networking and interactive capabilities of social media to facilitate purchase transactions (Yadav et al., Citation2013). The interoperability of the social web functions coupled with existing e-commerce functionality of social commerce is now harnessed by marketers to reach their target consumers efficiently. The collaborative structure of social commerce enables higher customer engagement, paving the way for long-term customer relationships. With reference to Insider Intelligence Forecast, the growing user engagement with social media, estimated as a daily average of 65.44 minutes in 2020 (Phaneuf, Citation2021), is driving brands to utilize social commerce as part of their online retail strategy.
In 2019, more than one-third of the internet users in the US reported the importance of social commerce (Vithayathil et al., Citation2020); as such, marketers are gearing up to explore various formats of social commerce to reach their target (Cutshall et al., Citation2021). While social media is increasingly used for managing customer relationships, advertising, and generation of word-of-mouth (Alalwan, Citation2018), marketers are resorting to social commerce sites such as Instagram, WeChat, Amazon Live, Facebook, and others to directly sell to their consumers with ample opportunities for concurrent engagement and interactions (Han et al., Citation2018). While consumer-to-consumer social commerce has been picking up (Yeon et al., Citation2019; Zhao et al., Citation2019), more organized business models based on structured social commerce platforms that apply social media applications such as community-based networking to create collaborative online marketplaces are also on the rise (Akram et al., Citation2021; Huang & Benyoucef, Citation2013; Nadeem et al., Citation2020).
Although direct and indirect influences of social media on consumer behavior across online and offline marketplaces have been investigated by researchers (Alalwan, Citation2018; Vithayathil et al., Citation2020), the amplified effects of consumer-to-consumer communications, communities, and influences (Kaplan & Haenlein, Citation2010) in social commerce is attracting the attention of researchers and practitioners in the context of the evolving online retail landscape. Here, the application of traditional theories, such as social identity theory, as a basis for examining the role of in-group behavior, social groups, and communities of social networking-based retail platforms is gaining ground in consumer behavior research (Brown, Citation2000; Hogg & Abrams, Citation2007; Kleine et al., Citation2009). The need to understand the specific impact of atypical social environment cues like profile pages, pictures, social exchanges, and reviews (Gardiner, Citation2019; Shanmugam et al., Citation2016; Yeon et al., Citation2019) is considered essential for marketers who want to make the best use of this evolving online retail opportunity.
In the wake of the rising importance of social commerce and the clear paucity of academic studies in the domain (Han et al., Citation2018), this study seeks to fill a gap by investigating how the combined e-commerce and social networking functionality of social commerce sites may impact consumer purchasing behavior. More specifically, it focuses on those social cues explicitly communicated through profile pictures, logos, and other social networking practices (e.g., “follows,” “likes”) and their correlation to retail outlet performance indicators on a social commerce site. Following a discussion of social commerce phenomena and literature, a sample of social and commerce performance indicators from US-based shops on “Etsy.com” are used to evaluate the influence of explicit and implicit social signals on consumer purchasing behavior.
2. Theoretical foundation
2.1. Social identity theory in the social commerce context
Derived from social psychology, the Social Identity Theory (SIT) developed in the mid to late 1970s (Hogg et al., Citation1995) identified social identity as a person’s sense of self based on group memberships. Unlike personal identity, the seminal work in SIT suggests that an individual’s interpersonal behavior within an identified group or community gives individuals a sense of belonging that ultimately defines their social identity (Hogg & Turner, Citation1985; Tajfel et al., Citation1979). SIT becomes pertinent in consumer research as it is accepted that the components of social identity influence an individual’s attitude and behavior within and even outside their membership groups. As a consequence of social identity- in-group bias, out-group discrimination, intragroup homogeneity, and changing intergroup attitudes through contact (Brown, Citation2000) are highlighted by several studies.
In consumer research, it is observed that social networks and interactions serve as socialization agents that lead to social learning and, in the process, influence consumer choices (Kleine et al., Citation2009; Singh & Basu, Citation2023). As reviewed by Singh and Basu (Citation2023), research articles on online shopping have repeatedly claimed that across platforms, such social influences bring in a change in attitude and other internal dispositions of a consumer that shape their beliefs and intentions to adapt to the changing marketplaces. Social interactions create public and private identities for the consumer that are often shaped by the normative beliefs of their membership groups (Brown, Citation2000; Hogg & Abrams, Citation2007). Conforming in-group behavior for explicitly categorized groups of consumers, which is the starting point of the cognitive process of forming social identity, has been recognized by research (Hogg & Abrams, Citation2007).
The present study considers SIT as the underlying premise for understanding the behavior of individual consumers in social commerce platforms. Unlike traditional e-commerce, social commerce, similar to social networking, has more scope for creating human-to-human business models. Membership-based social commerce platforms embedded with features of person-to-person and even consumer-to-consumer communications create communities with distinct social identities. As interpersonal communication is the best-established idea for the transmission of marketplace information (Feick & Price, Citation1987), social media networks serve as an important interpersonal source to connect not only with friends and family but also strangers who may share a common goal as consumers (Kaplan & Haenlein, Citation2010). Therefore, the community-driven interactions and exchanges in social commerce give a sense of belonging to its members that, in turn, guides their consumer preferences and choices (Abed, Citation2020; Yusuf & Busalim, Citation2018). The credibility of interpersonal sources of information scoring over non-personal sources (Feick & Price, Citation1987; Yusuf & Busalim, Citation2018) of marketing communication accentuated by social identity-based interactions significantly influences purchase decisions (Dellarocas, Citation2006).
In the context of social commerce, individuals may use such platforms to express their group identity and seek validation from their social groups. For example, individuals may share their purchases on social media, seek opinions from their online friends and followers, and participate in online communities or groups that align with their interests or identities (Jacobsen & Barnes, Citation2017; Reeder et al., Citation2022). These online interactions and behaviors can be seen as an extension of individuals’ group identity, as they seek social affirmation and belonging through online shopping (Basu & Sondhi, Citation2021; Singh & Basu, Citation2023; Sondhi & Basu, Citation2023).
Moreover, the in-group dynamic characteristic of Social Identity Theory may lead consumers to prefer products or brands associated with their social groups (Reeder et al., Citation2022; Tajfel et al., Citation1979). Within social commerce, individuals may be more likely to make purchases from online sellers or brands that are affiliated with their social groups, as it reinforces their group identity and strengthens their sense of belonging (Basu & Sondhi, Citation2021; Jacobsen & Barnes, Citation2017; Sondhi & Basu, Citation2023). Additionally, individuals may be more influenced by the opinions and recommendations of their social groups as they seek to conform to group norms and values (Basu & Sondhi, Citation2021; Li, Citation2022). This can impact their purchasing decisions on social commerce platforms as they may rely heavily on social cues from their social groups to guide their choices.
2.2. Classical S-R model in the social commerce context
The S (Stimulus)-O (Organism)-R (Response) framework developed by Mehrabian and Russel (Citation1974) was an extension of the classical Stimulus-Response (S-R) Theory (Zhang & Benyoucef, Citation2016). The relevance of the classical S-R model in explaining an individual’s learned responses to external stimuli was extended to capture the significant role of consumer’s internal disposition as a determinant of behavioral responses actually motivated researchers to apply the S-O-R model in the context of the online retail environment (Eroglu et al., Citation2003; Fiore et al., Citation2005; Ha & Lennon, Citation2010). However, a number of studies exploring the role of virtual retail atmospheric stimuli in the form of visual elements or website design to the overall web environment, etc. in determining the resultant response of consumers in e-commerce platforms have established the applicability of the classical S-R as well as the S-O-R framework in non-physical contexts (Basu et al., Citation2022; Eroglu et al., Citation2001; Kühn & Petzer, Citation2018; Peng & Kim, Citation2014; Wang et al., Citation2011).
Subsequently, as a natural extension of the classical S-R model-based consumer research in online retail, similar relevance has been noted in social commerce. The role of social commerce-specific environmental stimuli- like content characteristics, information availability, information valence, or even social interactions have been studied for their effect on the consumer’s attitude and behavioral responses (Alalwan, Citation2018; Wang & Chang, Citation2013; Zhang & Benyoucef, Citation2016, Park et al., Citation2014; Zhang et al., Citation2014). Therefore, the present study logically highlights the relevance of the classical S-R framework as a model to explain the determinants of consumer’s behavioral responses in a social commerce platform. As we understand that social commerce environmental stimuli are a blend of stimuli pertaining to e-commerce and social networking, the S-R framework lends support in categorizing a range of stimuli under the single umbrella of a stimulus leading to the consumers’ attitude and resultant behavior in the process.
2.3. An integrated research model
Considering the relevance of SIT and the classical S-R framework in understanding consumer behavior in social commerce, the present study proposes an integrative model (see Figure ). The proposed research model from the broader dimension of the S-R framework highlights that the environmental cues of the social commerce platforms can be broadly classified under marketer-generated cues and social interaction cues, respectively. While the marketer generated cues would typically cover all relevant information shared by the marketer to draw the attention of the consumer, the social interaction cues are identified as the user or consumer-generated content such as group affiliation, information sharing and shopping experience feedback, characteristic of the online consumer purchase journey that becomes relevant in the interactive social commerce environment. Hence, an integrated model that brings together the composite influence of traditional cues of a marketer in the parlance of a classical S-R framework with that of the consumer-generated social cues as a hallmark of SIT helps in developing a comprehensive understanding of external stimuli applicable in the context of the evolving social commerce environment. Therefore, the overall stimulus characterized by environmental cues as a composite of classical marketer driven stimulus and supplemental social identity driven stimulus is considered as an antecedent of consumer’s response in a social commerce environment. The novel use of SIT helps identify and categorize the environmental community-based social cues specific to social commerce in addition to the traditional environmental cues of an e-commerce platform. The use of both frameworks, when operationalized, allows for an integrated model where consumers’ social belonging driven perceived stimulus supported by marketer’s action driven stimulus works together to influencing response in the form of purchase behaviors. The integrative model also helps in accounting for not only event-based marketer’s action driven stimulus but also attempts to capture the long term perception driven stimulus that is most likely to be at play in the community driven interoperability of a social commerce platform.
3. Hypothesis development
The hypotheses for the study are developed with reference to the proposed research model that integrated the SIT in a classical S-R framework. Considering that social commerce blends the functionalities of traditional e-commerce with that of social networking, the study argues that along with the classical marketing stimuli of the social commerce platforms, the collaborative social interactions of the community acting as a social identity driven influence on a social commerce consumer, impact the consumers’ purchase intention.
3.1. The effect of seller-generated social cues on consumer purchase
Various web cues that affect online shopping have been identified by researchers. Findings suggest that the quality of website functions and design considerations positively affect a consumer’s intention to continue using the social commerce site (Liang et al., Citation2011) with evidence of substantial economic value or sales performance for the retail platform (Stephen & Toubia, Citation2010). A range of web cues varying in their degrees of human characteristics, typically referred to as social cues are increasingly used by online retailers (Wang et al., Citation2007). In congruence with the importance of visual cues in the physical retail environment (Helmefalk & Hultén, Citation2017), its importance in “webmospherics” has been established by researchers (Basu et al., Citation2022; Ha & Lennon, Citation2010; Liu et al., Citation2013; Peng & Kim, Citation2014; Wang et al., Citation2019). The importance of pictures over words in invoking users’ feelings across online platforms has been established (Pittman & Reich, Citation2016), where pictures or even simple interactive texts with greater human characteristics may seem to have greater effects on online purchase intentions (Wang et al., Citation2007). Specifically, in social networking, the quality or type of profile pictures in creating a positive identity is well accepted (Hum et al., Citation2011; Zheng et al., Citation2016). Therefore, task-relevant visual cues using a mix of images, pictures, colors, shapes, and fonts with appropriate visual rhetoric used by the seller as environmental stimuli are seen to influence a shopper’s pleasure or arousal, which in turn positively related to purchase intention (Ha & Lennon, Citation2010; Liu et al., Citation2013; Roy et al., Citation2020; Wang et al., Citation2007; Wang et al., Citation2019). However, the quality of information can be an important determinant of the real effect of such seller-generated cues in the social commerce context (Huang & Benyoucef, Citation2013). So, our first broad hypothesis that forms the basis of two sub-hypothesis is
H1:
Consumers are more likely to purchase (# of transactions) from social commerce platforms with seller-generated social cues (shop and owner images) present on the platform.
H1A:
Consumers are more likely to purchase (# of transactions) from social commerce platforms with seller-generated shop-level social cues (human likeness shop images) present on the platform.
H1B:
Consumers are more likely to purchase (# of transactions) from social commerce platforms with seller-generated shop owner-level social cues (human likeness owner images) present on the platform.
3.2. The effect of user interactions (social cues) on consumer purchase
Consumers are influenced in their consumption choices via their interaction with active opinion leaders and influencers (posters) in social media platforms and online social networks. This development has led to numerous online word-of-mouth communities and forums. Information exchanges, particularly those in online settings, often happen among consumers who have no prior relationship with one another as the participatory tools of Web 2.0 enable individuals worldwide to share their personal experiences, thoughts, and opinions to the global community in an easily accessible manner (Andrade et al., Citation2019; Roy et al., Citation2017, Citation2020). Research shows that the user-turned-opinion leaders who create and share task-relevant content impact consumer decision-making in social media settings (Dellarocas, Citation2006; Huang & Benyoucef, Citation2013). In online shopping environments, consumers can post (openly communicate) their shopping experience with a particular product or vendor or not communicate their experience. Regardless of their choice, online consumers are influenced by the content of existing reviews and ratings other consumers leave (Roy et al., Citation2020). Consumers who actively participate in online communities creating content are known as posters. In contrast, those who belong to online communities primarily as content consumers are called lurkers. Both types of members evaluate the commentary and activity of other members but may evaluate them differently (Schlosser, Citation2005). The creation and consumption of this user-generated content, often composed of word-of-mouth, feedback, or other means of experiential product or service quality reviews, is characteristic of social commerce (Shanmugam et al., Citation2016). Typically, consumers or online community members consume this user-generated content on a digital, social media, or social commerce platform (Chen & Shen, Citation2015; Roy et al., Citation2020). Hence, our second broad hypothesis, which leads us to the three sub-hypotheses, is:
H2
Consumers are more likely to purchase from social commerce platforms with higher user-generated social networking cues (#of admirers, # of followers, and #of reviews) present on the platform.
H2A
Consumers are more likely to purchase from social commerce platforms with a higher number of admirers present on the platform.
H2B
Consumers are more likely to purchase from social commerce platforms with a higher number of followers present on the platform.
H2C
Consumers are more likely to purchase from social commerce platforms with a higher number of reviews present on the platform.
4. Methodology
The purpose of this study is to provide empirical evidence to support the effect of socially relevant cues in the social commerce context. As the nomenclature suggests, social commerce includes qualitative social considerations and quantitative purchase transaction components. By examining qualitative and quantitative characteristics, the study seeks to evaluate whether and which social networking factors may influence consumer purchasing decisions in social commerce environments. This section provides a detailed discussion of the research platform, data collection method, chosen variables, qualitative and quantitative data analyses, and research questions used to further substantiate the social commerce context.
4.1. Research platform
“Etsy.com” (hereafter, Etsy) is an online retail collection of small businesses selling handmade goods across various product categories. The site’s self-description states it “is a marketplace where people around the world connect, both online and offline, to make, sell, and buy unique goods.” In terms of social networking site categorization, Etsy is a social selling or social commerce site. Over 1.6 million active sellers are on the site, offering over 35 million unique items for sale to more than 24 million active consumers. The Etsy platform allows each retail shop to maintain a shop profile (see Figure ) and a shop owner profile page (see Figure ).
Figure 2. Example of an Etsy retail shop profile page (with human likeness seller cues). [print in color].
![Figure 2. Example of an Etsy retail shop profile page (with human likeness seller cues). [print in color].](/cms/asset/d0a1b308-513b-4730-acc6-e568ea897745/oabm_a_2285037_f0002_oc.jpg)
Figure 3. Example of Etsy shop owner profile page (with no human likeness social cues). [print in color].
![Figure 3. Example of Etsy shop owner profile page (with no human likeness social cues). [print in color].](/cms/asset/4efdf58e-f2fb-4ed6-bee5-fc0b3fc9f4bb/oabm_a_2285037_f0003_oc.jpg)
These paired profile pages combine to provide qualitative data on social cues like signaling images and quantitative data for commerce measurement of consumer purchase behavior. The pages also act as a platform where consumers may consume information about a respective shop’s product offerings, prices, and social markers of the shop and the owner. Data elements from both profile pages for each respective shop in the data set are used in this study.
4.2. Data
This study uses data collected from a sample of the associated shop and shop owner retail pages of registered Etsy retail outlets. Since social commerce platforms leverage social media characteristics, we identified data based on commonly measured social media metrics. These metrics include valance and volume of content, and size of network structure, as measured through relevant count and rating data (Peters et al., Citation2013). On Etsy, retail outlets or shops, and individual items can be marked favorites by registered users (members) of the site, making them an admirer of the shop. Consumers may also rate shops (1–5 stars) for their experience with an actual purchase transaction with the shop. Additional social signals may be communicated via photos or images at the shop or shop owner level. Individual shop data includes a historical number of sales, the number of times a shop has received unique favorite designations from registered users, the geographic location of the shop, and an overall rating from customers. These discrete data points offer many possibilities for testing consumer behavior regarding purchases, “favoriting,” or product associations. In summary, traditional and image-based data elements available from the paired Etsy Shop page and the Etsy Shop Owner pages are used in this study. Relevant data elements available to be mined from the visual rendering of Etsy Shop and Owner pages, and their respective descriptions are described in detail in Table .
Table 1. Detailed descriptions of variables
4.3. Data collection & coding techniques
For this study, data collection required manual retrieval of both the traditional data elements and social signals requiring shop and shop owner-related image coding. There is a specific need in this study to analyze both quantitative and qualitative data. Images are a central focus of the data. These images allow for the assessment of the impact of one of the important types of social cues that may be influencing purchasing decisions as established by prior research, especially in the context of social networking platforms (Hum et al., Citation2011; Pittman & Reich, Citation2016; Zheng et al., Citation2016). Other social cues can include considerations of gender, age, race/ethnicity, or income level. Given the nature of the image data, the focus is on a more generalized social cue of whether or not an image is displaying a human likeness. In this respect, many images are straightforward, depicting an identifiable person or human image, or lack thereof. The qualitative nature of the images and human likeness signals allow for an analysis of the social aspects of social commerce. In contrast, the commerce component is best analyzed via quantitative data. This dual requirement of qualitative and quantitative analysis is a key characteristic of a study best suited for a mixed-methods approach (Tashakkori & Teddlie, Citation2008). The qualitative data consists of human likeness signals communicated through a series of images and the quantitative data centers around counts of commerce-related performance indicators. The data exist in a single location on the respective web pages of the Etsy platform, and both types of data are collected simultaneously. This type of dually located data suggests using a concurrent nested mixed-methods approach. However, should future research emphasize a consumer advocacy angle in the study, a concurrent transformative mixed-methods approach may be better suited (Hanson et al., Citation2005). See Table for a detailed description of the coding framework utilized.
Table 2. Coding framework
4.4. Sample
A sample of 200 Etsy retail outlets was gathered for data mining to create a usable dataset. This study considered those shops actively engaging in commercial activities and generating consumer sales. The site’s filtering capabilities in search functionality were utilized to increase the likelihood that the sample of Etsy retail outlets includes those with observable activity. First, a broad filter was applied within the generic “All Shops” search by selecting the flag “Most Recent.” This flag indicates those shops that are active registered participants on Etsy and have made at least a minimum level of content update at the time of data collection. The resulting listing of registered retail outlets was captured and reviewed via the method previously described.
4.5. Operationalizing the variables
Referring to the examples of Etsy registered shop and shop owner associated pages, dummy coding of the social cue images based on their human likeness factor were used as follows:
0 - Human likeness not present
1 - Human likeness present
An image was considered to have a human likeness present if it contained a picture of a person(s) face or full body. If an image did not include one or more faces or full-body images, it was coded as not having a human likeness present. Images in this category may have had no picture, a logo, or a picture of merchandise. (See “Image Data Elements” in Table )
As discussed in the social commerce literature, there are discrete social and commerce-related characteristics in social commerce sites like Etsy.com”. Therefore, the Etsy retail shop performance indicators were captured in two separate groups – social (# of Admirers and # of Followers) and commerce (# of Transactions).
Given the nature of the research questions, seeking to determine if seller-generated and user-generated social cues (social) are influencing consumer purchasing behavior (commerce), the number of transactions (# of transactions) was considered as the dependent variable. If a consumer has chosen to complete a purchase and engage in a commerce transaction with a given registered Etsy retail shop, it is accounted for in this variable. Note that the number of sales counts unique transactions over the business’s life, but not product counts. If a consumer purchases two items from a given retail shop, it counts as a single commerce transaction.
The independent variables in the analysis represent the social cues available to registered Etsy consumers while shopping on the site. These variables represent the “social” characteristics of social commerce that make it distinctly different from traditional e-commerce. The coded Owner Image and Shop Image observations comprise the social cue variables. Likewise, three social networking variables representing the existence of user-generated content (# of Reviews), the popularity of a retail shop (# of Admirers), and the sphere of influence or connectivity of a retail shop (# of Followers) make up the last three independent variables.
Several covariates are included in the model. These covariates represent additional characteristics, neither social nor commerce considerations, that also influence a given Etsy shop’s commerce performance or likelihood of consumer purchase. The Age of Shop represents the time since a shop was registered as a retail outlet on the site and the date of data collection (rounded to the nearest year). Finally, the total number of items available for purchase by consumers at the data collection date is accounted for in the variable number (#) of Items.
4.6. Data analysis methodology
The purpose of this applied analysis of social commerce is to test the premise that the social cues and social networking functionality of a social commerce site like Etsy influence consumer purchase behavior on such sites. To do so, the study uses a hierarchical approach to build a fully specified linear regression analysis. This technique allows the social and social networking cues to be added in blocks to see how the effects may progress. For the initial model (Model 1), the commerce-related dependent variable (# of Transactions) is regressed on the operational independent variables (# of Items and Age of Shop). The second model (Model 2) introduces the seller-generated social cue as independent variables (Owner Image and Shop Image). Finally, the third model (Model 3) adds the user-generated social networking cue as independent variables (# of Admirers, # of Followers, and # of Reviews). This method is hierarchical in that the independent variables are added in two blocks of “social cue” variables and not one by one. Each of the specified models is explained in detail as follows:
Model 1
Model 2
Model 3
Where:
Y = # of Transactions,
X1 = # of Items,
X2 = Age of Shop,
X3 = Shop Owner Image,
X4 = Shop Image,
X5 = # of Reviews,
X6= # of Admirers,
X7 = # of Follower
The dependent variable, the number (#) of (unique) transactions, is a count variable. As such, the model uses a negative binomial regression in lieu of a linear multiple regression model. While the data does include eight observations with a zero value for the dependent variable, this is not excessive, and a negative binomial regression model may be used. It is important to note that, in some instances, it is common practice to use an offset variable to standardize the observation time length in this model. However, for this study, the effect of the age of a shop is considered part of the operational characteristics of a shop. It is included as an independent variable in the model, not standardized and controlled for across the sample.
5. Results
Results from a descriptive analysis of the collected data provide context for the Etsy shops observed in this study. The sample represents a collection of shops of varying sizes and activity levels. The data show the average shop in the analysis is just over six years old, with roughly 360 items for sale and approximately 4,800 unique transactions. The average consumer-generated social cues were 2,564 admirers, 3244 followers, and 1,134 reviews. Just under half (49%) of all shop owners exhibited the social cue of a human likeness in their profile image, while only 10% chose to do so in the shop image thumbnail. For additional details regarding the sample’s descriptive statistics, please refer to Table .
Table 3. Descriptive statistics for select variables
In Table , selected Etsy variables other than the binary image variables are detailed in a correlation table. The table shows that only the number of reviews and the number of transactions are highly correlated or greater than a .80 correlation coefficient. This relationship is unsurprising, given that a consumer may only leave a review if they have engaged in a transaction with a shop.
Table 4. Correlation table for select variables
5.1. Baseline “simple” model with operational controls
Model 1, as shown in Table , represents the “simple” model, including only the traditional operational independent variables of the number of items for sale and the age of the shop. The results of this model show that both variables are positive and significant. Results for the age of a shop indicate that it has greater significance and a larger impact on overall shop performance than the number of items available for sale. This supports the conventional understanding that start-up retail outlets often falter, and those who stay in business longer will outperform their younger counterparts.
Table 5. Negative binomial regression results for models 1 through 3
5.2. Seller-generated social cues (shop-level & owner-level)
In Model 2, with the simple addition of seller-generated social cues, the number of items available for sale and the age of the shop continues to be significant and positively associated with shop performance. However, full results do not support our hypothesis (H1A or H1B) for seller-generated social cues. The owner-level social cue is not significant, while the shop-level social cue is significant but negatively associated with shop performance.
5.3. User-generated social networking cues (admirers, followers, reviews)
The final, fully loaded Model 3 introduces the user-generated social networking variables along with the earlier model. Two of the three user-generated social networking cues are significant in the model, indicating partial support for our second hypothesis, H2. The number of admirers is not significant and does not support H2A. The number of followers and the number of reviews are both significant and positively associated with shop performance, supporting H2B and H2C in our second hypothesis.
5.4. Seller-generated social cues vs. user-generated social networking cues
Model 3 also presents the opportunity to evaluate a comparison of seller-generated social cues and user-generated social networking cues. In addition to the partial support of H2, there is evidence of partial support for H1 in this model. Adding the user-generated social networking cues leads to the observation that the seller-generated shop social cue ceases to be significant. In contrast, the seller-generated shop owner social cue becomes significant. Therefore, we see that H1 is now partially supported by this result, at H1B, with the shop owner image having a significant and positive effect in the model. All preceding results are presented in condensed summary form in Table .
Table 6. Summary results
6. Discussion
The results of the statistical analyses provide a fascinating insight into consumer behavior on social commerce platforms. Starting with descriptive statistics, certain behaviors and possible preferences are evident. Sellers are more likely to communicate social cues via human images at the owner level than at the retail outlet level. A shop-level image or thumbnail often displays the shop logo, a picture of merchandise, or even a featured product instead of a human image. Conversely, sellers show a human image at the owner level, often their likeness, about as often as they choose to show some other image. When opting not to provide a human likeness for their profile picture, sellers are duplicating the image from the shop they own. Considering the user-generated social cues, social commerce platform consumers choose to connect to and receive updates at the shop level more than at the owner level. Consumers choose to communicate information about their consumption experience with a given shop for almost one in four transactions. Social cues generated by consumers encompass elements of SIT, including group membership, the exchange of information, and providing feedback on shopping experiences. These aspects are integral to the online consumer buying process. The credibility of these cues is reinforced by the validation provided by the classical stimulus of social cues generated by marketers, prominent in social commerce platforms. When both of these frameworks are put into practice, they create an integrated model in which consumers’ practices of belonging serve as the stimuli that shape their purchasing behaviors.
6.1. Theoretical contributions
This study offers two theoretical contributions. Social Identity Theory (SIT) introduced the observation that individuals derive their personal identity in part through the influences of the groups they belong to. In consumer contexts, SIT helps explain how group membership, in-group behaviors, and norms shape individual consumption. These group communications are even more important and influential in information-rich social networking environments. The Stimulus-Response (S-R) framework also focuses on external or environmental effects. Though traditionally studied in physical environments, our work demonstrates and emphasizes the relevance of both of these directionally different frameworks in the context of atypical virtual environment of social commerce characterized by collaboration and participation of key stakeholders other than the marketer. While marketer or seller generated cues captures event specific dimension, the social identity driven user generated cues help with a long term perspective on the buying process. The integrative model provides a comprehensive view of the overall stimuli of social commerce and its effect on consumer response to cover short term as well as long term perspectives of buying process in socially connected environments. With its combination of social networking and e-commerce characteristics, Social Commerce presents a unique opportunity to test our theoretical model integrating SIT into the traditional S-R framework. We find support that authenticity in the identity of owners and consumers, established through social cues in social commerce retail outlets, acts as stimuli leading to a positive response in purchase behavior.
6.2. Implications of practice
In today’s online commerce landscape, firms (and small businesses) have many models and options. Our findings emphasize the importance of leveraging social networking functionality for single-platform social commerce sites. Leveraging such functionality is especially crucial for newer entities to overcome their “newness” as retail outlets on social commerce platforms. For both small businesses and larger firms, it is important for any outlet to send a personable signal to potential consumers. Social commerce profiles, adopted from traditional social media platforms, provide an ideal vehicle for these signals. At a minimum, the social commerce owner’s profile page should offer an authentic human likeness or a personal picture. Social commerce sites are often associated with an identity of handmade or artisan products provided by individuals and small businesses. This differs from large-scale corporate social commerce platforms, like Amazon, which are more commonly associated with products sold by businesses. Compared to prior studies, we believe our most impactful findings are those that propose the multidimensional identity aspect, as our results show consumers place a stronger emphasis on identity at the owner level and not the item or merchandise level (see H1A & H1B). The identity aspect is multidimensional, as our results show consumers strongly emphasize identity at the owner level and not the item or merchandise level (see H1A & H1B). This suggests consumers’ desire to have a personal/authentic presence in ownership but not in branding-like spaces such as the thumbnail for the retail outlet itself. Furthermore, we find that the sphere of influence and not popularity is most impactful (see H2A, H2B, & H2C) from consumer cues with regard to purchase intention. This again emphasizes the importance of an authentic or personal connection at the owner level with consumers and not merely the popularity or attractiveness of the online storefront or product inventory therein. User-generated content in the form of reviews continues to be an important and integral part of informational and experiential consumer communication in the online retail marketplace, adding to the authenticity of the purchase transaction. Focusing on an authentic, personal, owner-level connection with solid user-generated content and engagement mitigates the critical risk of new entrant underperformance on social commerce sites. This strategy may benefit small businesses, sole proprietors, and large commercial outlets as they begin to adopt a retail presence on existing and new social commerce platforms.
6.3. Limitations and future studies
The preceding study and data set focused solely on shops in the United States. A more extensive study of internationally based shops in specific countries or regions may provide insight into how these social cues and social networking variables influence consumer purchasing when the seller is not domestic and the user base is cross-cultural.
There may be great value in an analysis that delves further into the authenticity implications found in this study and how they may provide more insight into the organism component of our SIT/S-R integrated framework. The role of human images and personalization of the shopping experience appears to strongly influence consumption, replacing the age of a shop as a significant predictor. Further study may allow observation into the mechanism behind this relationship. Is authenticity truly at play? Does human likeness represent credibility? Moreover, understanding interactions between the social signals and other variables, like age of shop or social networking variables, could be investigated in future studies.
Disclosure statement
No potential conflict of interest was reported by the authors.
References
- Abed, S. S. (2020). Social commerce adoption using TOE framework: An empirical investigation of Saudi Arabian SMEs. International Journal of Information Management, 53, 102118. https://doi.org/10.1016/j.ijinfomgt.2020.102118
- Akram, U., Junaid, M., Zafar, A. U., Li, Z., & Fan, M. (2021). Online purchase intention in Chinese social commerce platforms: Being emotional or rational? Journal of Retailing and Consumer Services, 63, 102669. https://doi.org/10.1016/j.jretconser.2021.102669
- Alalwan, A. A. (2018). Investigating the impact of social media advertising features on customer purchase intention. International Journal of Information Management, 42, 65–18. https://doi.org/10.1016/j.ijinfomgt.2018.06.001
- Andrade, N. A., Rainatto, G. C., Paschoal, D. G. E., Da Silva, F. R., & Renovato, G. (2019). Computational vision and business intelligence in the beauty segment-an analysis through instagram. Journal of Marketing Management, 7(2), 11–17.
- Basu, R., Paul, J., & Singh, K. (2022). Visual merchandising and store atmospherics: An integrated review and future research directions. Journal of Business Research, 151, 397–408. https://doi.org/10.1016/j.jbusres.2022.07.019
- Basu, R., & Sondhi, N. (2021). Online versus offline: Preferred retail choice for premium brand purchase. International Journal of Retail & Distribution Management, 49(10), 1447–1463. https://doi.org/10.1108/IJRDM-05-2020-0181
- Brown, R. (2000). Social identity theory: Past achievements, current problems and future challenges. European Journal of Social Psychology, 30(6), 745–778. https://doi.org/10.1002/1099-0992(200011/12)30:6<745:AID-EJSP24>3.0.CO;2-O
- Chen, J., & Shen, X. L. (2015). Consumers’ decisions in social commerce context: An empirical investigation. Decision Support Systems, 79, 55–64. https://doi.org/10.1016/j.dss.2015.07.012
- Cutshall, R., Changchit, C., Pham, H., & Pham, D. (2021). Determinants of social commerce adoption: An empirical study of Vietnamese consumers. Journal of Internet Commerce, 21(2), 1–27. https://doi.org/10.1080/15332861.2021.1907274
- Dabbous, A., Aoun Barakat, K., & Merhej Sayegh, M. (2020). Social commerce success: Antecedents of purchase intention and the mediating role of trust. Journal of Internet Commerce, 19(3), 262–297. https://doi.org/10.1080/15332861.2020.1756190
- Dellarocas, C. (2006). Strategic manipulation of internet opinion forums: Implications for consumers and firms. Management Science, 52(10), 1577–1593. https://doi.org/10.1287/mnsc.1060.0567
- Eroglu, S. A., Machleit, K. A., & Davis, L. M. (2001). Atmospheric qualities of online retailing: A conceptual model and implications. Journal of Business Research, 54(2), 177–184. https://doi.org/10.1016/S0148-2963(99)00087-9
- Eroglu, S. A., Machleit, K. A., & Davis, L. M. (2003). Empirical testing of a model of online store atmospherics and shopper responses. Psychology & Marketing, 20(2), 139–150. https://doi.org/10.1002/mar.10064
- Feick, L. F., & Price, L. L. (1987). The market maven: A diffuser of marketplace information. Journal of Marketing, 51(1), 83–97. https://doi.org/10.1177/002224298705100107
- Fiore, A. M., Kim, J., & Lee, H. H. (2005). Effect of image interactivity technology on consumer responses toward the online retailer. Journal of Interactive Marketing, 19(3), 38–53. https://doi.org/10.1002/dir.20042
- Gardiner, S. P. (2019). Do luxury car brands market premium status in a world of likes, Followers, and user-generated content? Journal of Marketing Management, 7(2), 18–27.
- Ha, Y., & Lennon, S. J. (2010). Online visual merchandising (VMD) cues and consumer pleasure and arousal: Purchasing versus browsing situation. Psychology & Marketing, 27(2), 141–165. https://doi.org/10.1002/mar.20324
- Hanson, W. E., Creswell, J. W., Clark, V. L. P., Petska, K. S., & Creswell, J. D. (2005). Mixed methods research designs in counseling psychology. Journal of Counseling Psychology, 52(2), 224. https://doi.org/10.1037/0022-0167.52.2.224
- Han, H., Xu, H., & Chen, H. (2018). Social commerce: A systematic review and data synthesis. Electronic Commerce Research and Applications, 30, 38–50. https://doi.org/10.1016/j.elerap.2018.05.005
- Helmefalk, M., & Hultén, B. (2017). Multi-sensory congruent cues in designing retail store atmosphere: Effects on shoppers’ emotions and purchase behavior. Journal of Retailing and Consumer Services, 38, 1–11. https://doi.org/10.1016/j.jretconser.2017.04.007
- Hogg, M. A., & Abrams, D. (2007). Intergroup behavior and social identity. In Hogg, M. A., & Cooper, J. (Eds.), The Sage Handbook of Social Psychology: Concise Student Edition (pp. 335–360). SAGE. https://doi.org/10.4135/9781848608221
- Hogg, M. A., Terry, D. J., & White, K. M. (1995). A tale of two theories: A critical comparison of identity theory with social identity theory. Social Psychology Quarterly, 58(4), 255–269. https://doi.org/10.2307/2787127
- Hogg, M. A., & Turner, J. C. (1985). Interpersonal attraction, social identification and psychological group formation. European Journal of Social Psychology, 15(1), 51–66. https://doi.org/10.1002/ejsp.2420150105
- Huang, Z., & Benyoucef, M. (2013). From e-commerce to social commerce: A close look at design features. Electronic Commerce Research and Applications, 12(4), 246–259. https://doi.org/10.1016/j.elerap.2012.12.003
- Hum, N. J., Chamberlin, P. E., Hambright, B. L., Portwood, A. C., Schat, A. C., & Bevan, J. L. (2011). A picture is worth a thousand words: A content analysis of Facebook profile photographs. Computers in Human Behavior, 27(5), 1828–1833. https://doi.org/10.1016/j.chb.2011.04.003
- Jacobsen, S., & Barnes, N. G. (2017). On being social: How social identity impacts social commerce for the millennial shopper. International Journal of Management Science and Business Administration, 3(4), 38–45. https://doi.org/10.18775/ijmsba.1849-5664-5419.2014.34.1005
- Kaplan, A. M., & Haenlein, M. (2010). Users of the world, unite! The challenges and opportunities of social media. Business Horizons, 53(1), 59–68. https://doi.org/10.1016/j.bushor.2009.09.003
- Kleine, R. E., III, Kleine, S. S., & Brunswick, G. J. (2009). Transformational consumption choices: Building an understanding by integrating social identity and multi‐attribute attitude theories. Journal of Consumer Behaviour: An International Research Review, 8(1), 54–70. https://doi.org/10.1002/cb.273
- Kühn, S. W., & Petzer, D. J. (2018). Fostering purchase intentions toward online retailer websites in an emerging market: An SOR perspective. Journal of Internet Commerce, 17(3), 255–282. https://doi.org/10.1080/15332861.2018.1463799
- Li, Y. (2022). Identity construction in social media: A study on blogging continuance. Behaviour & Information Technology, 41(8), 1671–1688. https://doi.org/10.1080/0144929X.2021.1895319
- Liang, T. P., Ho, Y. T., Li, Y. W., & Turban, E. (2011). What drives social commerce: The role of social support and relationship quality. International Journal of Electronic Commerce, 16(2), 69–90. https://doi.org/10.2753/JEC1086-4415160204
- Liu, Y., Li, H., & Hu, F. (2013). Website attributes in urging online impulse purchase: An empirical investigation on consumer perceptions. Decision Support Systems, 55(3), 829–837. https://doi.org/10.1016/j.dss.2013.04.001
- Mehrabian, A., & Russell, J. A. (1974). An approach to environmental psychology. The MIT Press.
- Nadeem, W., Khani, A. H., Schultz, C. D., Adam, N. A., Attar, R. W., & Hajli, N. (2020). How social presence drives commitment and loyalty with online brand communities? The role of social commerce trust. Journal of Retailing and Consumer Services, 55, 102136. https://doi.org/10.1016/j.jretconser.2020.102136
- Park, M. S., Shin, J. K., & Ju, Y. (2014). The effect of online social network characteristics on consumer purchasing intention of social deals. Global Economic Review, 43(1), 25–41. https://doi.org/10.1080/1226508X.2014.884047
- Peng, C., & Kim, Y. G. (2014). Application of the stimuli-organism-response (SOR) framework to online shopping behavior. Journal of Internet Commerce, 13(3–4), 159–176. https://doi.org/10.1080/15332861.2014.944437
- Peters, K., Chen, Y., Kaplan, A. M., Ognibeni, B., & Pauwels, K. (2013). Social media metrics—A framework and guidelines for managing social media. Journal of Interactive Marketing, 27(4), 281–298. https://doi.org/10.1016/j.intmar.2013.09.007
- Phaneuf, A., 2021. Social commerce 2021: Social media and ecommerce convergence trends brings growth opportunity for brands. Business Insider. [Retrieved August 11, 2021]. Available at: https://www.businessinsider.com/social-commerce-brand-trends-marketing-strategies?IR=T
- Pittman, M., & Reich, B. (2016). Social media and loneliness: Why an Instagram picture may be worth more than a thousand Twitter words. Computers in Human Behavior, 62, 155–167. https://doi.org/10.1016/j.chb.2016.03.084
- Reeder, M. B., Burkhalter, J. N., & Wood, N. T. (2022). ‘Scandal,’ wine and shopping: The social customer journey for the Camille 23-oz. wine glass. Journal of Digital & Social Media Marketing, 10(2), 159–172.
- Roy, G., Datta, B., & Basu, R. (2017). Trends and future directions in online marketing research. Journal of Internet Commerce, 16(1), 1–31. https://doi.org/10.1080/15332861.2016.1258929
- Roy, G., Datta, B., Mukherjee, S., & Basu, R. (2020). Effect of eWOM stimuli and eWOM response on perceived service quality and online recommendation. Tourism Recreation Research, 46(4), 1–16. https://doi.org/10.1080/02508281.2020.1809822
- Schlosser, A. E. (2005). Posting versus lurking: Communicating in a multiple audience context. Journal of Consumer Research, 32(2), 260–265. https://doi.org/10.1086/432235
- Shanmugam, M., Sun, S., Amidi, A., Khani, F., & Khani, F. (2016). The applications of social commerce constructs. International Journal of Information Management, 36(3), 425–432. https://doi.org/10.1016/j.ijinfomgt.2016.01.007
- Singh, K., & Basu, R. (2023). Online consumer shopping Behaviour: A review and research agenda. International Journal of Consumer Studies, 47(3), 815–851. https://doi.org/10.1111/ijcs.12899
- Sondhi, N., & Basu, R. (2023). Profiling the online premium brand consumers based on their fashion orientation. Asia Pacific Journal of Marketing & Logistics, 35(2), 380–397. https://doi.org/10.1108/APJML-07-2021-0492
- Stephen, A. T., & Toubia, O. (2010). Deriving value from social commerce networks. Journal of Marketing Research, 47(2), 215–228. https://doi.org/10.1509/jmkr.47.2.215
- Tajfel, H., Turner, J. C., Austin, W. G., & Worchel, S. (1979). An integrative theory of intergroup conflict. Organizational Identity: A Reader, 56(65), 9780203505984–16.
- Tashakkori, A., & Teddlie, C. (2008). Introduction to mixed method and mixed model studies in the social and behavioral science. In Plano-Clark, V. L., & Creswell, J. W. (Eds.), The Mixed Methods Reader (pp. 7–26). SAGE.
- Vithayathil, J., Dadgar, M., & Osiri, J. K. (2020). Social media use and consumer shopping preferences. International Journal of Information Management, 54, 102–117. https://doi.org/10.1016/j.ijinfomgt.2020.102117
- Wang, L. C., Baker, J., Wagner, J. A., & Wakefield, K. (2007). Can a retail web site be social? Journal of Marketing, 71(3), 143–157. https://doi.org/10.1509/jmkg.71.3.143
- Wang, J. C., & Chang, C. H. (2013). How online social ties and product-related risks influence purchase intentions: A Facebook experiment. Electronic Commerce Research and Applications, 12(5), 337–346. https://doi.org/10.1016/j.elerap.2013.03.003
- Wang, W., Chen, R. R., Ou, C. X., & Ren, S. J. (2019). Media or message, which is the king in social commerce? An empirical study of participants’ intention to repost marketing messages on social media. Computers in Human Behavior, 93, 176–191. https://doi.org/10.1016/j.chb.2018.12.007
- Wang, Y. J., Minor, M. S., & Wei, J. (2011). Aesthetics and the online shopping environment: Understanding consumer responses. Journal of Retailing, 87(1), 46–58. https://doi.org/10.1016/j.jretai.2010.09.002
- Yadav, M. S., De Valck, K., Hennig-Thurau, T., Hoffman, D. L., & Spann, M. (2013). Social commerce: A contingency framework for assessing marketing potential. Journal of Interactive Marketing, 27(4), 311–323. https://doi.org/10.1016/j.intmar.2013.09.001
- Yeon, J., Park, I., & Lee, D. (2019). What creates trust and who gets loyalty in social commerce? Journal of Retailing and Consumer Services, 50, 138–144. https://doi.org/10.1016/j.jretconser.2019.05.009
- Yusuf, A. S., & Busalim, A. H. (2018). Influence of e-WOM engagement on consumer purchase intention in social commerce. Journal of Services Marketing, 32(4), 493–504. https://doi.org/10.1108/JSM-01-2017-0031
- Zhang, K. Z., & Benyoucef, M. (2016). Consumer behavior in social commerce: A literature review. Decision Support Systems, 86, 95–108. https://doi.org/10.1016/j.dss.2016.04.001
- Zhang, H., Lu, Y., Gupta, S., & Zhao, L. (2014). What motivates customers to participate in social commerce? The impact of technological environments and virtual customer experiences. Information & Management, 51(8), 1017–1030. https://doi.org/10.1016/j.im.2014.07.005
- Zhao, J. D., Huang, J. S., & Su, S. (2019). The effects of trust on consumers’ continuous purchase intentions in C2C social commerce: A trust transfer perspective. Journal of Retailing and Consumer Services, 50, 42–49. https://doi.org/10.1016/j.jretconser.2019.04.014
- Zheng, W., Yuan, C. H., Chang, W. H., & Wu, Y. C. J. (2016). Profile pictures on social media: Gender and regional differences. Computers in Human Behavior, 63, 891–898. https://doi.org/10.1016/j.chb.2016.06.041