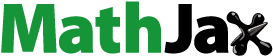
Abstract
This paper delves into the intricate interplay among Customer Lifetime Value (CLV), strategic marketing practices, and their impact on organizational financial performance. Aimed at informing strategic decision-making for marketers and business leaders, the study employs a mixed-methods research approach, combining quantitative and qualitative methods. Of the 375 participants invited to partake, 332 questionnaires were deemed valid for analysis. Quantitative data from customer transaction records and qualitative insights from a customized questionnaire reveal positive customer perceptions regarding CLV factors. Respondents show moderate to high agreement with statements related to product alignment, long-term outlook, repeat purchases, loyalty rewards, and customer-oriented services. The results underscore the pivotal role of CLV in influencing financial metrics, notably market valuation (P/E ratio). Recommendations include enhancing customer service, refining marketing strategies, and maintaining financial stability to bolster customer confidence.
1. Introduction
In today’s highly competitive business landscape, organizations are constantly seeking effective strategies to maximize their marketing efforts and drive sustainable growth. Amidst this pursuit, understanding and leveraging the concept of Customer Lifetime Value (CLV) has emerged as a critical component for strategic marketing success (Jasek et al., Citation2019). CLV represents the total value a customer generates over their entire relationship with a company, encompassing not only immediate transactions but also the potential for long-term loyalty and profitability. (Kumar & Reinartz, Citation2016).
It is impossible to exaggerate the significance of Customer Lifetime Value (CLV) and strategic marketing techniques in modern business settings. A crucial research issue still remains, notwithstanding the wealth of studies conducted in this field: a thorough comprehension of the complex interactions between CLV, strategic marketing techniques, and their effects on the financial performance of organizations. By examining the complex dynamics of CLV and strategic marketing, this study seeks to close this knowledge gap and offer crucial insights to practitioners in the field as well as scholars. Fragmented knowledge and theoretical inconsistencies impede academic understanding in this field, underscoring the need for a comprehensive analysis of CLV’s influence on marketing strategies and financial outcomes.
Furthermore, practically speaking, companies are realizing how crucial CLV is to decision-making regarding resource allocation, CRM tactics, and overall business performance. Therefore, this study not only adds to academic scholarship but also provides actionable insights that can enhance business competitiveness in today’s dynamic market landscape and inform strategic decision-making by clarifying the complex relationship between CLV, strategic marketing practices, and financial performance.
This research paper delves into the multifaceted realm of CLV and its pivotal role in shaping marketing strategies. By unlocking the treasure trove of CLV insights, organizations can gain a deeper understanding of their customers, tailor marketing initiatives, and make informed decisions that fuel long-term customer value creation. Through an exploration of various dimensions within the CLV-Marketing nexus.
The foundation of this research lies in establishing a conceptual framework for CLV. This research begins by defining CLV and examining its theoretical underpinnings. By exploring the core components of CLV, including customer acquisition cost, retention rate, and average revenue per customer, this research lay the groundwork for understanding its intricate calculations and implications.
Within this context, a critical aspect of this research is an in-depth analysis of the different methodologies and approaches used to measure CLV. Traditional methods, such as historic CLV and customer segmentation-based CLV, will be explored alongside more advanced techniques, including predictive modeling and machine learning algorithms (Kubacka, Citation2020; Sunder et al., Citation2016). Through a comparative evaluation of these methods, this research aims to shed light on their respective strengths, limitations, and practical applications. Moreover, this research endeavors to examine the diverse factors that shape CLV, such as customer behavior, satisfaction, loyalty, and engagement. By exploring the dynamic interplay between marketing strategies and the creation of customer value over the customer lifecycle. Moreover, the financial implications of CLV on an organization’s bottom line cannot be overlooked. By harnessing the potential for enhanced profitability, return on investment (ROI), and long-term sustainable growth, organizations can capitalize on the power of CLV to drive superior financial performance.
However, while the benefits of CLV are evident, its practical implementation comes with challenges. This research addresses these challenges, including data availability, accuracy, and integration across organizational functions. Furthermore, as marketing landscapes evolve, exploring CLV in the context of digital marketing, social media, and Omni-channel strategies presents an exciting avenue for future research.
The structure of this paper is as follows. Section 1 the introduction. Section 2 the relevant literature. Section 3 Methodology. Section 4 presents the results. Finally, section 5 presents the conclusions.
2. Literature review
2.1. Conceptual framework
Customer Lifetime Value (CLV) serves as a vital metric for understanding the long-term value that customers bring to a business. It represents the total economic value generated by a customer over their entire relationship with a company, encompassing both their initial purchase and subsequent transactions. CLV provides insights into customer profitability, guiding marketing strategies and resource allocation decisions (Chen et al., Citation2018; Gupta et al., Citation2021).
CLV is composed of several key components that collectively contribute to its calculation and interpretation. One such component is the Customer Acquisition Cost (CAC), which represents the expenses incurred in acquiring a customer. It encompasses marketing and sales costs associated with lead generation, advertising, promotional activities, and sales efforts (Reinartz & Kumar, Citation2022). The CAC is an essential factor in determining the profitability of acquiring new customers and should be weighed against their CLV.
The concept of Customer Lifetime Value (CLV) has its roots in marketing theory, where it serves as a fundamental metric for assessing the long-term profitability of customer relationships (Rust et al., Citation2004). CLV is grounded in the economic theory of customer valuation, which posits that customers contribute varying levels of value to a firm over their lifetime based on their purchase behavior, loyalty, and profitability (Berger & Nasr, Citation1998). From a strategic marketing perspective, CLV is integral to the formulation of effective customer acquisition, retention, and development strategies (Blattberg et al., Citation2001).
Strategic marketing theories provide valuable insights into the drivers of CLV and the development of marketing strategies aimed at maximizing customer lifetime value. The Resource-Based View (RBV) of the firm emphasizes the importance of leveraging firm-specific resources and capabilities to create and sustain competitive advantage (Barney, Citation1991). In the context of CLV, RBV suggests that firms can enhance customer value by investing in resources that improve customer satisfaction, loyalty, and retention (Homburg et al., Citation2017). Additionally, Relationship Marketing theory emphasizes the significance of building long-term, mutually beneficial relationships with customers through personalized interactions, trust-building, and value co-creation (Dandis et al., Citation2022; Grönroos, Citation1994),
Furthermore, financial theories provide insights into the financial implications of CLV for organizational performance. The Market-Based View (MBV) of the firm focuses on the impact of market conditions and investor perceptions on firm value (Castéran et al., Citation2021; Wernerfelt, Citation1984). In the context of CLV, MBV suggests that firms with higher CLV may command higher market valuations due to their ability to generate sustainable cash flows and achieve superior financial performance (Rust et al., Citation2004).
Drawing on these theoretical foundations, our conceptual framework integrates key constructs such as customer value, loyalty, satisfaction, and retention to elucidate the drivers and outcomes of CLV. By synthesizing insights from marketing, finance, and strategic management theories, our study aims to provide a comprehensive understanding of the complex relationship between CLV, strategic marketing practices, and organizational financial performance. This theoretical framework guides the development of our research hypotheses and informs our empirical analysis, thereby contributing to both theoretical advancement and practical implications in the field of CLV and strategic marketing. Damm & Rodríguez Monroy, Citation2011).
Retention rate is another crucial component of CLV. It measures the likelihood that a customer will continue their relationship with the company over a specified period. Higher retention rates indicate stronger customer loyalty, longer-lasting relationships, and increased CLV (Lemon & Verhoef, Citation2021). By focusing on improving customer retention, organizations can cultivate profitable, long-term customer relationships and enhance CLV.
Average Revenue per Customer is an additional component of CLV. It represents the average amount of revenue generated by a customer during their relationship with the company. This metric captures both the frequency of purchases and the value of each transaction. By increasing the average revenue per customer, businesses can boost their overall CLV and drive sustainable growth (Lew, Citation2017; Winer, Citation2021).
Based on the conceptual framework illustrate the study model.
The theoretical underpinnings of CLV are rooted in the concept of customer-centricity and relationship marketing. CLV recognizes that customers are not uniform entities; they have different characteristics, preferences, and lifetime behaviors. By incorporating these aspects into CLV calculations, organizations can tailor their marketing efforts to specific customer segments, providing customized experiences and targeted value propositions (Venkatesan & Kumar, Citation2021).
The relevance of CLV for marketing decision-making cannot be overstated. It guides strategic marketing planning, budget allocation, and customer acquisition strategies. By identifying high-value customers and focusing on cultivating long-term relationships with them, businesses can optimize their marketing investments and maximize customer lifetime profitability (Berger & Nasr, Citation2021).
In summary, CLV serves as a crucial metric for understanding customer value and profitability over the course of the customer relationship. Its components, including Customer Acquisition Cost, Retention Rate, and Average Revenue per Customer, contribute to a comprehensive assessment of CLV. The theoretical foundations of customer-centricity and relationship marketing underscore the importance of CLV in guiding marketing decisions and optimizing long-term customer value.
2.2. CLV Measurement methods
Calculating Customer Lifetime Value (CLV) involves employing various approaches and methodologies that consider different aspects of customer behavior, purchase patterns, and future revenue potential (Wang et al., Citation2019). Traditional methods such as historic CLV and customer segmentation-based CLV provide a foundation, while more advanced techniques like predictive modeling and machine learning algorithms offer enhanced accuracy and predictive capabilities (Chamberlain et al., Citation2017; Jasek et al., Citation2019; Su et al., Citation2023).
Historic CLV is a widely used method that estimates CLV based on past customer transaction data. It considers the historical purchase behavior, average transaction value, and frequency of purchases to predict future revenue potential (Reinartz & Kumar, Citation2022). This method is relatively straightforward to implement and does not require complex data analysis. However, it assumes that past behavior is a reliable indicator of future behavior, which may not always hold true.
Customer segmentation-based CLV takes a more granular approach by dividing customers into distinct segments based on shared characteristics, preferences, or behaviors. Each segment is assigned a specific CLV based on its unique purchase patterns and revenue potential (Gupta et al., Citation2021). This method allows for personalized CLV calculations and enables targeted marketing strategies. However, it requires robust data collection and segmentation capabilities, which may pose challenges for organizations lacking sufficient data infrastructure (Sunder et al., Citation2016).
Predictive modeling techniques employ statistical models to forecast future customer behavior and estimate CLV. These models consider a range of variables, such as demographics, purchase history, customer interactions, and external market factors, to predict future revenue potential (Fader & Hardie, Citation2021). Predictive modeling allows for more accurate and dynamic CLV calculations, capturing the evolving nature of customer behavior. Nevertheless, it requires sophisticated data analysis techniques, substantial data sets, and expert modeling capabilities (Gadgil et al., Citation2023).
Machine learning algorithms offer further advancements in CLV estimation by leveraging complex algorithms to analyze large volumes of customer data and identify patterns and trends. These algorithms can identify non-linear relationships, uncover hidden insights, and make accurate predictions (Kumar & Reinartz, Citation2022). Machine learning-based CLV models can consider a wide range of factors and deliver highly accurate forecasts. However, their implementation requires substantial computational resources, extensive data preprocessing, and expertise in machine learning techniques.
Each CLV measurement method has its strengths and limitations. Historic CLV is straightforward to calculate and provides a baseline estimation. Customer segmentation-based CLV allows for personalized insights and targeted marketing efforts. Predictive modeling techniques provide enhanced accuracy and dynamic forecasting capabilities. Machine learning algorithms offer sophisticated analysis and uncover complex relationships within the data (Gadgil et al., Citation2023)
The practical application of CLV measurement methods depends on various factors, including the availability and quality of data, organizational resources, and the desired level of precision (Safari et al., Citation2016). For organizations with limited data infrastructure and resources, traditional methods may be more feasible and provide valuable insights. On the other hand, organizations with robust data capabilities and analytical expertise can explore advanced techniques to gain a competitive edge and refine their marketing strategies (Dandis et al., Citation2022)
In summary, CLV measurement methods encompass a range of approaches, from traditional methods like historic CLV and customer segmentation-based CLV to advanced techniques such as predictive modeling and machine learning algorithms. Each method offers distinct advantages and considerations in terms of accuracy, complexity, and practical implementation. Organizations should select the appropriate method based on their data capabilities, resources, and the desired level of precision for CLV estimation.
2.3. Factors Influencing CLV
Customer Lifetime Value (CLV) is influenced by a multitude of factors that shape customer behavior, satisfaction, loyalty, and engagement. Understanding these factors and their interplay with marketing strategies is crucial for organizations seeking to optimize CLV and drive long-term customer value creation (Chamberlain et al., Citation2017).
Customer behavior plays a significant role in CLV. Factors such as purchase frequency, average transaction value, and product/service preferences impact the revenue potential of individual customers. By analyzing historical transaction data and identifying patterns in customer behavior, organizations can tailor marketing strategies to encourage desirable behaviors and increase CLV (Reinartz & Kumar, Citation2022).
Customer satisfaction is another critical factor that influences CLV. Satisfied customers are more likely to make repeat purchases, exhibit higher levels of loyalty, and engage in positive word-of-mouth recommendations. By delivering exceptional products, services, and customer experiences, organizations can enhance customer satisfaction and cultivate long-term relationships, ultimately driving higher CLV (Lemon & Verhoef, Citation2021).
Customer loyalty is closely linked to CLV. Loyal customers not only contribute to recurring revenue but also tend to have higher CLV due to their longer tenure and increased purchase frequency. Effective loyalty programs, personalized communications, and superior customer service can foster loyalty and enhance CLV by incentivizing repeat purchases and strengthening the emotional connection between customers and the brand (Rane et al., Citation2023).
Engagement is a key driver of CLV, encompassing customer interactions, brand affinity, and active participation. Engaged customers are more likely to explore additional offerings, provide feedback, and advocate for the brand. By implementing targeted engagement strategies, such as personalized marketing communications, social media interactions, and community-building initiatives, organizations can foster stronger customer relationships and boost CLV (Kumar & Reinartz, Citation2022).
Marketing strategies and initiatives play a vital role in influencing these factors and ultimately affecting CLV. By aligning marketing efforts with customer needs and preferences, organizations can enhance customer satisfaction, encourage desired behaviors, and foster loyalty. Targeted marketing campaigns, personalized recommendations, and relationship-building activities can strengthen customer engagement and increase CLV throughout the customer lifecycle (Venkatesan & Kumar, Citation2021).
Moreover, the interplay between marketing efforts and customer value creation is critical for maximizing CLV. Effective marketing strategies not only drive initial customer acquisition but also focus on nurturing customer relationships over time. By continuously delivering value, addressing evolving customer needs, and adapting marketing tactics to different stages of the customer lifecycle, organizations can enhance CLV by increasing customer retention and expanding revenue opportunities (Berger & Nasr, Citation2021; Yassin, Citation2017).
In summary, multiple factors influence CLV, including customer behavior, satisfaction, loyalty, and engagement. Marketing strategies and initiatives play a significant role in shaping these factors and ultimately affecting CLV. By understanding customer needs, delivering exceptional experiences, fostering loyalty, and promoting active engagement, organizations can optimize CLV and drive long-term customer value creation.
2.4. Marketing strategies for CLV optimization
Maximizing Customer Lifetime Value (CLV) requires the implementation of effective marketing strategies and tactics that foster long-term customer relationships and drive revenue growth (Jasek et al., Citation2019). By leveraging personalized marketing approaches, customer relationship management (CRM), customer retention programs, and upselling/cross-selling techniques, organizations can enhance CLV and create sustained value for customers (Chamberlain et al., Citation2017; Yaseen et al., Citation2023). Personalized marketing approaches play a pivotal role in CLV optimization. By tailoring marketing communications and offers to individual customer preferences, organizations can deliver targeted messages that resonate with customers on a personal level. This approach enhances customer engagement, satisfaction, and loyalty, leading to increased CLV (Venkatesan & Kumar, Citation2021). For example, Amazon utilizes personalized recommendations based on customer browsing and purchase history, effectively driving cross-selling and increasing CLV.
Customer relationship management (CRM) systems provide a framework for managing customer interactions and fostering long-term relationships. Through CRM, organizations can capture and analyze customer data, gain insights into individual preferences and behaviors, and develop targeted marketing strategies (Venkatesan & Kumar, Citation2021). CRM systems enable personalized communication, effective segmentation, and proactive customer service, all of which contribute to improved customer satisfaction, loyalty, and ultimately, increased CLV. Salesforce is a prime example of a CRM platform that helps organizations optimize CLV by centralizing customer data, automating processes, and facilitating personalized interactions.
Customer retention programs are instrumental in driving CLV by reducing customer churn and extending customer lifetimes. Loyalty programs, VIP memberships, and exclusive benefits incentivize customers to stay engaged with the brand and continue making repeat purchases. By rewarding customer loyalty, organizations cultivate stronger relationships and increase the likelihood of future revenue streams (Kumar & Reinartz, Citation2022; Shaban et al., Citation2023). The Starbucks Rewards program is a notable example, where customers accumulate points with each purchase, leading to enhanced customer retention and increased CLV.
Upselling and cross-selling techniques also contribute to CLV optimization. Upselling involves encouraging customers to upgrade or purchase higher-priced products or services, thereby increasing the average transaction value and CLV. Cross-selling, on the other hand, entails suggesting complementary products or services to customers, expanding their purchase portfolio and driving incremental revenue (Reinartz & Kumar, Citation2022). For instance, online retailers like Amazon excel in cross-selling by recommending related products based on customers’ purchase history, leading to increased CLV through additional purchases.
To align these marketing strategies with CLV goals, organizations must have a deep understanding of customer needs and preferences. By leveraging customer data, behavior analytics, and segmentation techniques, organizations can identify customer segments with high CLV potential and tailor marketing strategies accordingly (Berger & Nasr, Citation2021). It is crucial to ensure that marketing initiatives focus not only on short-term transactions but also on cultivating long-term relationships and customer loyalty.
Successful implementation of these strategies requires a customer-centric mindset and continuous evaluation of their effectiveness. Regularly monitoring customer satisfaction, retention rates, and CLV metrics allows organizations to refine their approaches, identify opportunities for improvement, and adapt marketing strategies to evolving customer needs and preferences (Huang et al., Citation2023; Lemon & Verhoef, Citation2021).
In summary, marketing strategies for CLV optimization encompass personalized marketing approaches, CRM systems, customer retention programs, and upselling/cross-selling techniques. By aligning these strategies with CLV goals and leveraging customer data, organizations can foster long-term customer relationships, drive revenue growth, and maximize CLV.
2.5. Financial implications of CLV
The financial impact of Customer Lifetime Value (CLV) on an organization’s bottom line is significant. CLV insights provide valuable information that can inform resource allocation, budgeting, and marketing investment decisions, ultimately leading to enhanced profitability, return on investment (ROI), and long-term sustainable growth (Wang et al., Citation2019).
Resource allocation is a critical aspect of CLV management. By understanding the value that each customer segment brings to the organization, companies can allocate resources more effectively. CLV insights enable organizations to prioritize high-value customer segments and allocate resources accordingly, focusing on activities that have the greatest potential to drive long-term profitability (Kumar & Reinartz, Citation2022). This targeted resource allocation approach ensures that limited resources are utilized optimally, resulting in improved overall financial performance (Bauer & Jannach, Citation2021; Bolton, Citation2004).
Budgeting decisions can be significantly influenced by CLV insights. CLV provides organizations with a forward-looking perspective, enabling them to forecast revenue potential and allocate budgets accordingly. By considering the expected future value of customers, organizations can make informed decisions about marketing budgets, customer acquisition costs, and customer retention programs. CLV-driven budgeting helps align expenditures with expected returns, enhancing cost-effectiveness and maximizing financial outcomes (Ascarza et al., Citation2018).
Marketing investment decisions are also heavily impacted by CLV insights. CLV allows organizations to evaluate the profitability of different marketing initiatives and channels (Bolton, Citation2004). By assessing the CLV generated from different marketing campaigns, organizations can identify the most effective strategies and channels that yield the highest returns. CLV-driven marketing investment decisions ensure that resources are allocated to activities that generate the greatest long-term customer value and deliver a positive impact on the organization’s financial performance (Bauer & Jannach, Citation2021).
The effective management of CLV has the potential to enhance profitability and ROI. By focusing on high-value customer segments and implementing targeted marketing strategies, organizations can increase customer retention, drive repeat purchases, and stimulate cross-selling and upselling opportunities. This leads to a higher customer lifetime value and improved profitability as the revenue generated from loyal and high-value customers outweighs the costs associated with acquiring and serving them (Kanchanapoom & Chongwatpol, Citation2023; Venkatesan & Kumar, Citation2021).
Moreover, effective CLV management supports long-term sustainable growth. By nurturing strong customer relationships, organizations can foster customer loyalty, reduce churn rates, and generate consistent revenue streams over an extended period. CLV-driven strategies focus on building customer lifetime value rather than short-term gains, enabling organizations to establish a foundation for sustainable growth and maintain a competitive advantage in the market (Berger & Nasr, Citation2021).
In summary, CLV has significant financial implications for organizations. CLV insights inform resource allocation, budgeting, and marketing investment decisions, enabling organizations to optimize their financial performance. By focusing resources on high-value customer segments, aligning budgets with revenue potential, and investing in strategies that maximize CLV, organizations can enhance profitability, ROI, and long-term sustainable growth.
2.6. Challenges and future directions
While Customer Lifetime Value (CLV) provides valuable insights for organizations, its implementation and measurement present several challenges. Addressing these challenges is crucial for organizations seeking to harness the full potential of CLV and adapt to evolving marketing landscapes.
One key challenge is data availability and accuracy. CLV calculations require comprehensive and accurate data on customer transactions, interactions, and preferences (Yılmaz Benk et al., Citation2022). Organizations often face obstacles in obtaining complete and clean data, as data may be fragmented across various systems and departments. Data quality issues, such as missing or inconsistent information, can affect the accuracy of CLV calculations (Reinartz & Kumar, Citation2022). Overcoming these challenges requires robust data collection processes, data integration efforts, and data governance practices to ensure reliable and comprehensive data availability for CLV measurement.
Integration of CLV across organizational functions is another challenge. CLV should be integrated into marketing, sales, customer service, and other relevant departments to drive coordinated efforts and optimize customer value. However, organizational silos and a lack of cross-functional collaboration can hinder the effective integration of CLV insights into decision-making processes (Yılmaz Benk et al., Citation2022). Overcoming this challenge requires a cultural shift towards a customer-centric mindset and the establishment of collaborative frameworks that facilitate the sharing of CLV insights across departments.
Emerging trends and advancements in CLV research offer opportunities for addressing these challenges and unlocking the full potential of CLV. One significant trend is the increasing importance of digital marketing and omnichannel strategies. As customer interactions occur across multiple touchpoints and channels, incorporating digital data sources into CLV calculations becomes essential. Advanced analytics techniques, such as machine learning and artificial intelligence, can help organizations leverage large volumes of digital data to gain deeper insights into customer behavior and enhance CLV predictions (Gupta et al., Citation2021).
Another future direction is the integration of CLV with customer experience management (CEM). By combining CLV insights with customer feedback and sentiment analysis, organizations can gain a holistic understanding of customer value and the factors influencing it. This integration allows organizations to identify and prioritize investments in areas that enhance the overall customer experience and drive CLV growth (Lemon & Verhoef, Citation2021).
Furthermore, exploring CLV in the context of emerging technologies, such as blockchain and Internet of Things (IoT), presents new avenues for research. These technologies offer opportunities to enhance data security, traceability, and customer engagement, potentially leading to more accurate CLV calculations and personalized marketing approaches (Amendah et al., Citation2023; Venkatesan & Kumar, Citation2021).
To overcome the challenges associated with CLV implementation and measurement, organizations should invest in advanced analytics capabilities, data management infrastructure, and organizational alignment. Collaboration between data scientists, marketers, and business leaders is crucial for developing robust CLV models, ensuring data accuracy, and aligning CLV insights with business goals.
In summary, challenges related to data availability, accuracy, and organizational integration pose hurdles in CLV implementation. However, emerging trends and advancements in CLV research, including the incorporation of digital marketing, omnichannel strategies, and integration with customer experience management, provide opportunities for overcoming these challenges. Exploring CLV in the context of emerging technologies further expands the potential for improving CLV measurement and application in marketing strategies.
Based on the review of the literature, the study can generate the main hypothesis:
H01: CLV has no significant impact on an organization’s financial performance (ROA, ROE and P/E Ratio)
3. Method
This study used a mixed-methods research approach. This approach combines quantitative and qualitative methods to gather data, analyze it, and draw meaningful conclusions. A mixed-methods research design is suitable for this study as it allows for a comprehensive analysis of CLV and marketing. By combining quantitative and qualitative approaches, this design provides a more holistic understanding of the topic, capturing both numerical data and rich insights from customer perspectives. While the qualitative analysis explores the complex experiences, attitudes, and perceptions of customers, the quantitative analysis allows for the statistical examination of relationships and patterns. The integration of both methods allows for a deeper exploration and validation of the findings, enhancing the overall rigor and validity of the study (Komulainen & Nätti, Citation2023; Leone et al., Citation2018).
Qualitative data was gathered through a customized questionnaire exclusively designed for this study. Respondents were requested to rate their agreement or disagreement with each statement on a 5-point Likert scale, with options ranging from 1 (Strongly Disagree) to 5 (Strongly Agree). The questionnaire examined customers’ perceptions, preferences, and experiences with Customer Lifetime Value (CLV) and marketing strategies.
The optimal number of respondents for this research depends on the scale and diversity of the chosen companies; however, a sample size that allows for meaningful representation across industries and ensures statistical reliability could range from 300 to 400 respondents. In this research a total of 375 participants were invited to take part, and 332 questionnaires were deemed valid for analysis. The sample size of 332 is often considered sufficient for statistical analysis, particularly if it meets the minimum requirements for the chosen analytical techniques. Statistical power analysis can be conducted to determine if the sample size is adequate to detect meaningful effects with a certain level of confidence. We can ensure that the sample is representative of the population under study, thereby increasing the generalizability of our findings. Techniques such as random sampling or stratified sampling may have been employed to ensure a diverse and representative sample. The response rate, i.e. the percentage of individuals who completed the questionnaire out of those who were invited to participate, can be considered. A high response rate indicates a higher likelihood that the sample is representative and that the findings are reliable.
Quantitative data were collected through customer transaction records to gather quantitative data on CLV. Quantitative data were analyzed utilizing techniques, such as standard deviation, the mean scores, percentages and descriptive statistics.
The optimal number of purchase or transaction records that allows for meaningful representation across industries and ensures statistical reliability were 600 purchase observations for the loyal customers of the company’s sample. The CLV formula employed in this study is adapted from Berger and Nasr (Citation1998, Donkers et al. Citation2007), and is defined as follows:
Where:
CLVi: Customer Lifetime Value for customer i.
r: Time periods into the future
Profit i,t+r: Profit generated by customer i at time
d: Discount rate.
This data are obtained through online surveys, customer databases, and financial reports (ROA, ROE, and E/P artio). Quantitative data were analyzed utilizing various statistical techniques (the regression and correlation analysis).
3.1. Ethical approval statement
This study received ethical approval from the Faculty of Business Ethics Committee (Approval Reference: 10/04/2023-2024). The approval was obtained following review of the research proposal and questionnaire items by the committee.
3.2. Human participants a statement
Prior to participation in this study, all human participants provided informed consent. The nature and purpose of the study, as well as the rights and responsibilities of participants, were explained in detail. Informed consent was obtained in written form from each participant before any data collection procedures were initiated. Participants were assured of confidentiality and anonymity, and they were informed that they had the right to withdraw from the study at any time without consequence.
4. Results
4.1. Reliability test
In this study, the reliability and trustworthiness of the research instrument were evaluated using the Cronbach Alpha test. The questionnaire consisted of five study fields: Customer Lifetime Value (CLV), Marketing Strategies, Factors Influencing CLV, Financial Performance, and Marketing Decision-Making, each comprising five Likert-scale questions. The obtained reliability coefficients for each study field were 0.782 for CLV, 0.724 for Marketing Strategies, 0.801 for Factors Influencing CLV, 0.756 for Financial Performance, and 0.714 for Marketing Decision-Making. Overall, the questionnaire demonstrated satisfactory internal consistency, with most of the study fields surpassing the commonly accepted threshold of 0.7 for reliability (Sekaran & Bougie, Citation2016). The calculated overall average of all factors was approximately 0.7554, indicating a generally consistent measurement of constructs throughout the questionnaire. However, researchers should consider other validity indicators alongside Cronbach Alpha to ensure the overall rigor and quality of the research instrument. illustrate the results of the reliability and trustworthiness of the study.
Table 1. Values for reliability coefficients.
4.2. Demographic variables
The demographic characteristics of the study community are illustrated in . The demographic features of the respondents were as follows:
Table 2. Demographic characteristics.
The demographic characteristics of the study were assessed among a total of 332 respondents. The participants consisted of 68% males and 32% females. Regarding age distribution, the majority of respondents were between 35 to 44 years old (36%), followed by those aged 25 to 34 years (23%), and 45 to 54 years (16%). The remaining age groups constituted smaller proportions, with 8% between 18 to 24 years, 10% between 55 to 64 years, and 7% over 65 years. In terms of education level, the study encompassed participants with diverse educational backgrounds. The largest group had a Bachelor’s degree (62%), followed by Master’s degree holders (21%), and those with a Ph.D. degree (8%). Respondents with a High School education or below accounted for 9%. Concerning monthly income, the majority of respondents had incomes ranging between 501 to 1000 JD (60%), while 22% reported incomes below 500 JD. Smaller proportions fell into higher income brackets, with 11% earning between 1001 to 1500 JD, 4% earning between 1501 to 2000 JD, and 3% having incomes over 2000 JD. These demographic variables provide a comprehensive overview of the participants’ characteristics, facilitating a better understanding of their diverse perspectives and experiences in the study on Customer Lifetime Value (CLV) and marketing.
4.3. Questionnaires outcomes
, present the results of the questionnaires’ perspectives Customer Lifetime Value (CLV), Marketing Strategies, Factors Influencing CLV, Financial Performance, and Marketing Decision-Making.
Table 3. Customer lifetime value (CLV).
Table 4. Marketing strategies.
Table 5. Factors influencing CLV.
Table 6. Financial performance.
Table 7. Marketing decision-making.
The results of illustrated the mean scores, standard deviations, percentages, and order of importance for each item in the Customer Lifetime Value (CLV) study. The items were rated on a 5-point Likert scale.
First question received a mean score of 3.50, indicating that, on average, respondents moderately agreed that the company meets their needs and preferences. The standard deviation of 0.57 suggests that the responses were relatively consistent around the mean. Approximately 70.28% of respondents agreed with this statement, ranking it as the fifth most important item in the study. The second question showed a slightly higher agreement with this statement, with a mean score of 3.52. The standard deviation of 0.77 suggests some variability in responses. About 70.30% of respondents expressed agreement, ranking it as the fourth most important item. The third question received a mean score of 3.55, indicating a slightly higher level of agreement compared to the previous two items. The standard deviation of 0.57 suggests consistent responses. Around 70.85% of respondents agreed with this statement, ranking it as the third most important item. The fourth question indicated a relatively high level of agreement. The standard deviation of 0.68 suggests moderate variability in responses. Approximately 72.56% of respondents agreed with this statement, ranking it as the second most important item. The last question of this group received the highest mean score of 3.68, indicating that, on average, respondents agreed that the company’s customer service is effective and customer-oriented. The standard deviation of 0.64 suggests relatively consistent responses. Around 73.72% of respondents agreed with this statement, ranking it as the most important item in the study.
Overall, the results suggest that respondents generally held positive perceptions related to Customer Lifetime Value (CLV) factors. The top-rated item was the perception of the company’s customer service, which appears to be highly valued by the respondents. These findings provide valuable insights into the customers’ perspectives and experiences concerning the studied CLV components and can guide marketing decision-making and customer-focused strategies.
In , which presents the results of the Marketing Strategies section, respondents rated their agreement with five statements related to the effectiveness of the company’s marketing efforts. Here’s the main key finding:
In the first question the respondents moderately agreed with its statement, giving it a mean score of 3.42. Approximately 68.58% of respondents agreed with this statement, ranking it as the fourth most important item. In the second question the respondents also moderately agreed with this statement, resulting in a mean score of 3.42. About 68.56% of respondents expressed agreement, making it the fifth most important item in the study. In the third question the respondents showed a higher level of agreement with this statement, with a mean score of 3.64. Around 72.56% of respondents agreed with this statement, ranking it as the third most important item. The fourth question received the highest mean score of 3.68, indicating that respondents highly agreed that they regularly encounter the company’s marketing efforts. Approximately 73.70% of respondents agreed with this statement, making it the most important item in the study. In the last question of this group the respondents indicated a relatively high level of agreement with this statement, giving it a mean score of 3.67. About 73.15% of respondents agreed with this statement, ranking it as the second most important item.
In summary, the results suggest that respondents generally have positive perceptions of the company’s marketing strategies. They perceive the company’s marketing campaigns as engaging, influential in their purchasing decisions, and effectively communicating the brand message. Additionally, the use of multiple marketing channels appears to be positively recognized by respondents. These insights provide valuable guidance for marketing decision-making and can help refine marketing strategies to further engage the target audience and boost overall brand performance.
presents the results of the Factors Influencing CLV section, where respondents rated their agreement with five statements related to factors that influence their Customer Lifetime Value (CLV). Here’s the main key finding:
In the first question the respondents moderately agreed that the company’s loyalty program incentivizes them to make repeat purchases, with a mean score of 3.52. Approximately 70.28% of respondents agreed with this statement, ranking it as the fifth most important item. In the second question the respondents displayed a higher level of agreement with this statement, resulting in a mean score of 3.62. About 72.56% of respondents expressed agreement, making it the fourth most important item in the study. The third question received a higher mean score of 3.73, indicating that respondents agreed that positive customer experiences contribute to their loyalty to the company. Approximately 74.85% of respondents agreed with this statement, ranking it as the third most important item. In the fourth question the respondents indicated a relatively higher level of agreement with this statement, giving it a mean score of 3.78. About 75.44% of respondents agreed with this statement, making it the second most important item in the study. The fifth question of this group received the highest mean score of 3.91, indicating that respondents highly agreed that they actively refer friends and family to the company based on positive experiences. Approximately 78.29% of respondents agreed with this statement, making it the most important item in the study.
In summary, the results suggest that respondents’ Customer Lifetime Value (CLV) is influenced by various factors. The most influential factors include positive customer experiences, personalized offers, and a strong emotional connection with the brand. Additionally, the presence of a loyalty program and the likelihood of customers referring friends and family based on positive experiences play significant roles in enhancing CLV. These insights provide valuable information for businesses to strengthen customer loyalty, enhance customer experiences, and optimize their marketing strategies to maximize CLV.
presents the results of the Financial Performance section, where respondents rated their agreement with five statements related to the company’s financial performance and its impact on their perceptions and behaviors. Here’s the main key finding:
The first question received the highest mean score of 3.68, indicating that respondents highly agreed that the company’s financial stability affects their confidence in its long-term success. Approximately 73.72% of respondents agreed with this statement, making it the most important item in the study. In the second question the respondents moderately agreed with this statement, giving it a mean score of 3.55. Around 70.87% of respondents expressed agreement, ranking it as the third most important item. The third question received a mean score of 3.58, indicating that respondents agreed that the company’s financial performance is linked to its customer value proposition. Approximately 71.42% of respondents agreed with this statement, making it the second most important item in the study. In the fourth question the respondents showed a relatively lower level of agreement with this statement, resulting in a mean score of 3.32. About 66.28% of respondents agreed with this statement, ranking it as the fifth most important item. The fifth question received a mean score of 3.41, indicating that respondents agreed that they perceive the company as financially secure and trustworthy. Approximately 68.00% of respondents agreed with this statement, making it the fourth most important item in the study.
In summary, the results suggest that respondents’ perceptions of the company’s financial performance influence their confidence in its long-term success and their commitment to a long-term relationship with the brand. Positive financial performance is associated with their perception of the company’s customer value proposition, which contributes to the perception of the brand as financially secure and trustworthy. These insights highlight the significance of financial performance in shaping customer perceptions and their relationship with the company, indicating that financial stability is a key driver of customer loyalty and trust. Businesses can leverage these findings to strengthen their financial performance and communicate their financial stability to enhance customer trust and loyalty.
presents the results of the Marketing Decision-Making section, where respondents rated their agreement with five statements related to the company’s marketing decision-making process. Here’s the main key finding:
The first question of this group received the highest mean score of 3.68, indicating that respondents highly agreed that the company’s marketing decisions are aligned with customer needs and preferences. Approximately 73.73% of respondents agreed with this statement, making it tied for the first most important item in the study. In the second question the respondents moderately agreed with this statement, giving it a mean score of 3.47. Around 69.13% of respondents expressed agreement, ranking it as the fifth most important item. The third question received a mean score of 3.55, indicating that respondents agreed that the company’s marketing investments positively impact customer satisfaction and loyalty. Approximately 70.87% of respondents agreed with this statement, ranking it as the fourth most important item. In the fourth question the respondents highly agreed with this statement, resulting in a mean score of 3.78. About 75.44% of respondents agreed with this statement, making it tied for the first most important item in the study. The fifth question received a mean score of 3.68, indicating that respondents highly agreed that the company’s marketing strategies are well thought out and data-driven. Approximately 73.72% of respondents agreed with this statement, making it the third most important item in the study.
In summary, the results suggest that respondents highly value a customer-centric approach in the company’s marketing decision-making process. They believe that the company’s marketing decisions are aligned with customer needs and preferences and are well thought out and data-driven. Trust in the marketing team’s ability to make effective decisions and the positive impact of marketing investments on customer satisfaction and loyalty are also significant factors. These insights highlight the importance of customer-centricity and data-driven strategies in shaping customers’ perceptions of marketing decision-making and can guide businesses to optimize their marketing strategies to better meet customer needs and preferences.
4.4. Hypothesis outcomes
The regression analysis performed to understand the relationship between CLV and financial performance metrics is illustrated as follows:
Where:
Financial Metric i is the financial performance metric for observation i.
CLVi is the calculated CLV for observation i.
β0 is the intercept term.
β1 is the coefficient representing the impact of CLV on financial performance.
ϵi is the error term.
The regression results suggest a relationship between Customer Lifetime Value (CLV) and the financial performance metrics (Return on Assets - ROA, Return on Equity - ROE, and the Price-to-Earnings - P/E ratio). illustrate the key aspects of the results:
Table 8. Regression analysis.
The R-squared value is 0.0299, indicating that the model explains a small but measurable proportion of the variance in the dependent variable (financial performance metrics). The adjusted R-squared is slightly lower, suggesting that the inclusion of CLV as a predictor may be contributing to the model’s fit.
The intercept represents the estimated value of the financial performance metrics when all predictors are zero. The highly significant p-value (< 0.001) indicates that the model is not meaningful when all predictors are zero. The coefficients for ROA, ROE, and P/E are -15.0890, 2.2969, and 3.6075, respectively. ROA has a p-value of 0.5511, suggesting that it is not significantly associated with CLV in this model. ROE has a p-value of 0.6185, indicating that it is also not significantly associated with CLV. P/E has a highly significant p-value (< 0.001), suggesting a significant association with CLV.
The ANOVA table indicates that the overall model is statistically significant, with a p-value of 0.0004 associated with the F-statistic. This suggests that the model as a whole is providing a significant improvement in explaining the variance in financial performance metrics.
In summary, the results suggest that, based on the current dataset, Customer Lifetime Value (CLV) is significantly associated with the Price-to-Earnings (P/E) ratio. However, there is no significant association between CLV and Return on Assets (ROA) or Return on Equity (ROE) in this model. The overall model is statistically significant, indicating that, collectively, the predictors contribute to explaining the variance in financial performance metrics. Careful consideration of practical significance, model assumptions, and the context of the study is crucial for a comprehensive interpretation of these findings.
4.5. Correlation analysis
The correlation matrix provides insights into the relationships between Customer Lifetime Value (CLV), Return on Assets (ROA), Return on Equity (ROE), and Price-to-Earnings ratio (P/E). Here are some observations. illustrate the correlation analysis.
Table 9. Correlation analysis.
It is noticed that there is a weak negative correlation between CLV and ROA (-0.0896), suggesting a slight tendency for higher CLV to be associated with lower ROA. However, the correlation is close to zero, indicating a limited linear relationship. Similarly, there is a weak negative correlation between CLV and ROE (-0.0811). This implies a marginal tendency for higher CLV to be associated with lower ROE. As with CLV and ROA, the correlation is relatively small.
The correlation between CLV and P/E is positive (0.1711), indicating a slight tendency for higher CLV to be associated with higher P/E ratios. Again, the correlation is modest, suggesting a limited linear relationship.
There is a strong positive correlation between ROA, ROE and P/E (0.8848), (-0.4548) respectively. This indicates a robust linear relationship, suggesting that companies with higher ROA tend to have higher ROE.
5. Conclusions
5.1. Conclusions
The findings of this study hold significant theoretical and managerial implications for both academia and industry practitioners.
Theoretical Implications:
Advancing CLV Theory: By elucidating the complex relationship between CLV, strategic marketing practices, and organizational financial performance, this study contributes to the theoretical understanding of CLV dynamics. The integration of insights from marketing, finance, and strategic management theories provides a holistic framework for understanding the drivers and outcomes of CLV.
Filling Knowledge Gaps: This study addresses important knowledge gaps in the literature by providing empirical evidence on the influence of CLV factors on customer perceptions and financial performance metrics. Theoretical inconsistencies and fragmented knowledge are addressed, paving the way for future research in this area.
Enhancing Methodological Approaches: The mixed-methods research approach utilized in this study showcases the benefits of combining quantitative and qualitative methods for a comprehensive analysis of CLV and strategic marketing. This methodological approach can serve as a model for future research in similar domains.
Managerial Implications:
Strategic Decision-Making: The insights generated from this study can inform strategic decision-making processes for marketers and business leaders. By understanding the drivers of CLV and their impact on financial performance, organizations can develop more effective marketing strategies and resource allocation decisions.
Customer Relationship Management: Practitioners can leverage the findings of this study to enhance customer relationship management practices. Strategies aimed at improving customer satisfaction, loyalty, and perceived value can contribute to higher CLV and overall business success.
Financial Performance Enhancement: Organizations can use the insights from this study to enhance their financial performance by focusing on CLV-driven strategies. By optimizing customer acquisition, retention, and development efforts, firms can improve key financial metrics such as market valuation and profitability.
Key findings:
The qualitative results provide valuable insights into customer perceptions and preferences across key dimensions, including Customer Lifetime Value (CLV), Marketing Strategies, Factors Influencing CLV, Financial Performance, and Marketing Decision-Making.
Respondents generally hold positive perceptions of CLV factors, with the highest-rated item being the effectiveness of the company’s customer service, including insights that highlight the significance of customer service in influencing customer loyalty and lifetime value.
Positive perceptions of the company’s marketing strategies are observed, emphasizing the effectiveness of marketing campaigns, relevance, engagement, and the use of diverse channels. Moreover, continued emphasis on effective communication and diverse marketing channels is recommended to maintain positive customer perceptions.
Respondents associate the company’s financial stability with confidence in its long-term success. Moreover, positive financial performance is linked to perceptions of customer value proposition, emphasizing the importance of financial stability in building customer trust and loyalty.
Trust in the effectiveness of marketing decisions, alignment with customer needs, and data-driven strategies are crucial for positive customer perceptions.
The regression analysis provides insights into the relationship between CLV and financial performance metrics, including Return on Assets (ROA), Return on Equity (ROE), and Price-to-Earnings (P/E) ratio. Moreover, the model, collectively, is statistically significant in explaining the variance in financial performance metrics.
CLV is significantly associated with the Price-to-Earnings (P/E) ratio, as indicated by a highly significant p-value, but no significant associations are found between CLV and Return on Assets (ROA) or Return on Equity (ROE) in this model. In other words, the results suggest that, within the current dataset, the P/E ratio is a meaningful predictor of financial performance, whereas ROA and ROE do not significantly contribute to the model.
The correlation analysis shows that the relationships between CLV, ROA, ROE, and P/E are not strongly linear based on the correlation coefficients provided. Other factors and nonlinear relationships might influence the dynamics between these financial and customer-related metrics. Further analysis and consideration of additional variables could provide a more comprehensive understanding of these relationships.
5.2. Recommendations
Enhancing customer service excellence by strengthening efforts to consistently offer effective and customer-oriented services, as it emerged as the top-rated CLV factor, and by adopting a regular mechanism to assess and improve customer service strategies to maintain high levels of customer satisfaction.
Optimizing marketing communication throughout continuous and effective communication of brand messages through marketing campaigns, and investing in strategies that enhance relevance, engagement, and multichannel presence, ensuring sustained positive perceptions of marketing efforts.
Leveraging influential CLV factors throughout capitalizing on factors influencing CLV, such as positive customer experiences, personalized offers, and emotional connections. Moreover, implementing and enhancing loyalty programs, personalized marketing, and strategies to foster emotional connections.
Emphasizing financial stability throughout communicating and highlighting the company’s financial stability to build customer confidence. Moreover, aligning financial performance with the perceived customer value proposition, emphasizing transparency and trust.
Investing in employee training programs for employees, especially those involved in customer service and marketing. In addition to equipping employees with the skills and knowledge necessary to deliver exceptional customer experiences and effectively communicate the brand message.
Adopting a regular update customer feedback mechanism, and incorporating customer opinions and preferences to adapt strategies and offerings to evolving customer expectations.
As a contribution to future research, exploring additional variables and conducting longitudinal studies could provide a more comprehensive understanding of the dynamic interplay between CLV, marketing strategies, and financial performance. The findings of this study offer a robust foundation for marketers and business leaders to strategically align their efforts with customer expectations, ultimately fostering enduring customer relationships and organizational prosperity.
Author contributions
Conceptualization, O.S. and N.A.; methodology, O.S.; software, O.S.; validation, O.S., N.A.; formal analysis, O.S.; investigation, N.A.; resources, O.S. and N.A.; data curation, N.A.; writing—original draft preparation, O.S writing—review and editing, O.S. and N.A.; visualization, O.S.; supervision, O.S and N.A.; project administration, O.S. and N.A.
All authors agree to be accountable for all aspects of the work.
Contribution of the study
The study enhances theoretical understanding of CLV by integrating insights from marketing, finance, and strategic management. It elucidates the complex interplay between CLV and marketing strategies, highlights its financial implications, and offers a comprehensive framework for future research. Overall, it expands theoretical boundaries and advances our understanding of customer value dynamics.
Informed consent statement
Not applicable.
Disclosure statement
The authors declare no conflict of interest.
Data availability statement
Data is applicable upon request.
Additional information
Notes on contributors
Nafez Ali
Nafez Ali is an Associate professor in the Marketing Department of Al-Zaytoonah university of Jordan. He received his Ph.D in Marketing from Rajasthan university/India. He has 15 years of experience, and more than 25 research papers in peers reviewed international scientific journals. Ex-head of Marketing department. He also supervised more than 10 graduated students through his academic career.
Osama Samih Shabn
Osama Samih Shabn is a professor in the accounting department of Al-Zaytoonah university of Jordan. He received his Ph.D in accounting from JNV university/India. He has 28 years of experience, and more than 30 research papers in peers reviewed international scientific journals. Member of the International Society for Development & Sustainability (ISDS). Ex-head of accounting department, and Ex-head of evening studies. Participated in more than 15 international conferences. He also supervised more than 30 graduated students through his academic career.
References
- Amendah, E. R., Kohli, A., Kumthekar, N., & Singh, G. (2023). Impact of financial and nonfinancial constructs on customer lifetime value (CLV): US retailer’s perspective. Journal of Relationship Marketing, 22(3), 1–21. https://doi.org/10.1080/15332667.2023.2197769
- Ascarza, E., Neslin, S. A., Netzer, O., Anderson, Z., Fader, P. S., Gupta, S., Hardie, B. G. S., Lemmens, A., Libai, B., Neal, D., Provost, F., & Schrift, R. (2018). In pursuit of enhanced customer retention management: Review, key issues, and future directions. Customer Needs and Solutions, 5(1-2), 65–81. https://doi.org/10.1007/s40547-017-0080-0
- Barney, J. (1991). Firm resources and sustained competitive advantage. Journal of Management, 17(1), 99–120. https://doi.org/10.1177/014920639101700108
- Bauer, J., & Jannach, D. (2021). Improved customer lifetime value prediction with sequence-to-sequence learning and feature-based models. ACM Transactions on Knowledge Discovery from Data, 15(5), 1–37. https://doi.org/10.1145/3441444
- Berger, P. D., & Nasr, N. I. (1998). Customer lifetime value: Marketing models and applications. Journal of Interactive Marketing, 12(1), 17–30. https://doi.org/10.1002/(SICI)1520-6653(199824)12:1<17::AID-DIR3>3.0.CO;2-K
- Berger, P. D., & Nasr, N. I. (2021). Customer lifetime value and firm valuation. Journal of Marketing Research, 58(4), 707–725.
- Blattberg, R. C., Getz, G., & Thomas, J. S. (2001). Customer equity: Building and managing relationships as valuable assets. Harvard Business Press.
- Bolton, R. N. (2004). Linking marketing to financial performance and firm value. Journal of Marketing, 68(4), 73–75. https://doi.org/10.1509/jmkg.68.4.73.42727
- Castéran, H., Meyer-Waarden, L., & Reinartz, W. (2021). Modeling customer lifetime value, retention, and churn. In Handbook of market research (pp. 1001–1033). Springer International Publishing.
- Chamberlain, B. P., Cardoso, A., Liu, C. B., Pagliari, R., & Deisenroth, M. P. (2017, August). Customer lifetime value prediction using embeddings. In Proceedings of the 23rd ACM SIGKDD International Conference on Knowledge Discovery and Data Mining (pp. 1753–1762). https://doi.org/10.1145/3097983.3098123
- Chen, P. P., Guitart, A., del Río, A. F., & Periánez, A. (2018, December). Customer lifetime value in video games using deep learning and parametric models. In 2018 IEEE International Conference on Big Data (Big Data) (pp. 2134–2140). IEEE. https://doi.org/10.1109/BigData.2018.8622151
- Damm, R., & Rodríguez Monroy, C. (2011). A review of the customer lifetime value as a customer profitability measure in the context of customer relationship management. Intangible Capital, 7(2), 261–279. https://doi.org/10.3926/ic.2011.v7n2.p261-279
- Dandis, A. O., Al Haj Eid, M. B., Robin, R., & Wierdak, N. (2022). An empirical investigation of the factors affecting customer lifetime value. International Journal of Quality & Reliability Management, 39(4), 910–935. https://doi.org/10.1108/IJQRM-12-2020-0412
- Donkers, B., Verhoef, P. C., & de Jong, M. G. (2007). Modeling CLV: A test of competing models in the insurance industry. Quantitative Marketing and Economics, 5(2), 163–190. https://doi.org/10.1007/s11129-006-9016-y
- Fader, P., & Hardie, B. (2021). How to project customer retention. Harvard Business Review, 99(1), 130–136.
- Gadgil, K., Gill, S. S., & Abdelmoniem, A. M. (2023). A meta-learning based stacked regression approach for customer lifetime value prediction. Journal of Economy and Technology, 1, 197–207. https://doi.org/10.1016/j.ject.2023.09.001
- Grönroos, C. (1994). From marketing mix to relationship marketing: Towards a paradigm shift in marketing. Management Decision, 32(2), 4–20. https://doi.org/10.1108/00251749410054774
- Gupta, S., Lehmann, D. R., & Stuart, J. A. (2021). Valuing customers. Journal of Marketing, 85(2), 1–21.
- Homburg, C., Klarmann, M., & Schmitt, J. (2017). Brand-specific leadership: Turning employees into brand champions. Journal of Marketing, 81(3), 59–83.
- Huang, L., Liu, M. T., Song, X., & Yen, J. (2023). A method of customer valuation score and implementation for marketing strategy. Asia Pacific Journal of Marketing and Logistics, 35(2), 344–363. https://doi.org/10.1108/APJML-05-2021-0299
- Jasek, P., Vrana, L., Sperkova, L., Smutny, Z., & Kobulsky, M. (2019). Predictive performance of customer lifetime value models in e-commerce and the use of non-financial data. Prague Economic Papers, 28(6), 648–669. https://doi.org/10.18267/j.pep.714
- Kanchanapoom, K., & Chongwatpol, J. (2023). Integrated customer lifetime value (CLV) and customer migration model to improve customer segmentation. Journal of Marketing Analytics, 11(2), 172–185. https://doi.org/10.1057/s41270-022-00158-7
- Komulainen, R., & Nätti, S. (2023). Barriers to blockchain adoption: Empirical observations from securities services value network. Journal of Business Research, 159(February), 113714. https://doi.org/10.1016/j.jbusres.2023.113714
- Kubacka, M. (2020). Review and analysis of selected customer value measurement methods. Studia i Materiały, (1(32), 34–46.
- Kumar, V., & Reinartz, W. (2016). Creating enduring customer value. Journal of Marketing, 80(6), 36–68. https://doi.org/10.1509/jm.15.0414
- Kumar, V., & Reinartz, W. (2022). Increasing the ROI of customer management: Strategies to drive profitable customer behavior. Journal of Marketing, 86(3), 70–94.
- Lemon, K. N., & Verhoef, P. C. (2021). Understanding customer experience throughout the customer journey. Journal of Marketing, 85(3), 1–20.
- Leone, C., Lim, J. S. L., Stern, A., Charles, J., Black, S., & Baecker, R. (2018). Communication technology adoption among older adult veterans: The interplay of social and cognitive factors. Aging & Mental Health, 22(12), 1666–1677. https://doi.org/10.1080/13607863.2017.1381946
- Lew, G. (2017). The importance of customer lifetime value in determining their profitability. The Business & Management Review, 8(4), 24.
- Rane, N. L., Achari, A., & Choudhary, S. P. (2023). Enhancing customer loyalty through quality of service: Effective strategies to improve customer satisfaction, experience, relationship, and engagement. International Research Journal of Modernization in Engineering, Technology and Science, 5(5), 427–452.
- Reinartz, W., & Kumar, V. (2022). Customer lifetime value: Progress and challenges. Journal of Marketing, 86(1), 1–15.
- Rust, R. T., Lemon, K. N., & Zeithaml, V. A. (2004). Return on marketing: Using customer equity to focus marketing strategy. Journal of Marketing, 68(1), 109–127. https://doi.org/10.1509/jmkg.68.1.109.24030
- Safari, F., Safari, N., & Montazer, G. A. (2016). Customer lifetime value determination based on RFM model. Marketing Intelligence & Planning, 34(4), 446–461. https://doi.org/10.1108/MIP-03-2015-0060
- Sekaran, U., & Bougie, R. (2016). Research methods for business: A skill building approach. John Wiley & Sons.
- Shaban, O. S., Yassin, M. M., Al-Hawatmah, Z., Amoush, A. H., & Hamad, M. J. (2023 Maximizing financial performance and sustainability through innovative dividend policies: Evidence from emerging market [Paper presentation]. In Conference on sustainability and cutting-edge business technologies, Cham, May. (pp. 127–137) Springer Nature Switzerland.
- Su, H., Du, Z., Li, J., Zhu, L., & Lu, K. (2023). Cross-domain adaptative learning for online advertisement customer lifetime value prediction. In Proceedings of the AAAI Conference on Artificial Intelligence, 37(4), 4605–4613. June)(https://doi.org/10.1609/aaai.v37i4.25583
- Sunder, S., Kumar, V., & Zhao, Y. (2016). Measuring the lifetime value of a customer in the consumer packaged goods industry. Journal of Marketing Research, 53(6), 901–921. https://doi.org/10.1509/jmr.14.0641
- Venkatesan, R., & Kumar, V. (2021). A strategic framework for customer relationship management. Journal of Marketing, 85(6), 16–36.
- Wang, X., Liu, T., & Miao, J. (2019). A deep probabilistic model for customer lifetime value prediction. arXiv preprint arXiv:1912.07753.
- Wernerfelt, B. (1984). A resource-based view of the firm. Strategic Management Journal, 5(2), 171–180. https://doi.org/10.1002/smj.4250050207
- Winer, R. S. (2021). Marketing management: A customer-centric approach (3rd ed.). Pearson.
- Yaseen, S. G., Dajani, D., & Odeh, M. (2023, May) Intellectual capital and sustainable competitive advantage: The mediating role of marketing knowledge management. In Conference on sustainability and cutting-edge business technologies (pp. 237–251). Springer Nature Switzerland.
- Yassin, M. M. (2017). The determinants of internet financial reporting in Jordan: Financial versus corporate governance. International Journal of Business Information Systems, 25(4), 526–556. https://doi.org/10.1504/IJBIS.2017.085176
- Yılmaz Benk, G., Badur, B., & Mardikyan, S. (2022). A new 360° framework to predict customer lifetime value for multi-category e-commerce companies using a multi-output deep neural network and explainable artificial intelligence. Information, 13(8), 373. https://doi.org/10.3390/info13080373
Appendix.
Questionnaire
Dear Participant,
Thank you for participating in this research study. Your valuable insights will contribute to a better understanding of Customer Lifetime Value (CLV) and its relationship with marketing strategies. The purpose of this questionnaire is to gather your perceptions and experiences related to the topics discussed.
Please be assured that all your responses will be treated with the utmost confidentiality and used only for research purposes. The questionnaire will take approximately [estimated time] to complete. Your participation is voluntary, and you may choose to withdraw at any time without any consequences.
Part 1: Demographic information
Age:
Gender:
Education Level:
Employment Status:
Monthly Income:
Part 2: Customer lifetime value (CLV)
Please rate your level of agreement with the following statements on a scale of 1 to 5, where 1 represents ‘Strongly Disagree’ and 5 represents ‘Strongly Agree’.
Part 3: Marketing strategies
Part 4: Factors Influencing CLV
Part 5: Financial performance
Part 6: Marketing decision-making
Thank you for your participation! Your input is invaluable in advancing our understanding of Customer Lifetime Value (CLV) and marketing strategies. If you have any additional comments or insights you would like to share, please feel free to do so in the space provided below.