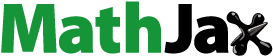
Abstract
Malaria potentially affects everyone in the tropics and sub-tropics, however, the poor and vulnerable are worse affected mainly due to the socio-economic constraints that confront them. In Ghana, the Upper West Region, which is the poorest, is one of the worse affected in terms of malaria burden. Given social and economic factors directly relate to malaria morbidity, global malaria control strategy unfortunately has not particularly targeted the effects of socio-economic deprivation on the disease morbidity and control. This study investigates the linkages between poverty and malaria morbidity using count data models, with Jirapa District in the Upper West Region of Ghana as the study area. Empirical results confirm the presence of poverty in the study area as more than half of households depend on heads whose incomes are below the poverty line of US$1 per day and that significant relationships exist between poverty and education on the one hand and malaria morbidity on the other, since gender and level of education of household head, and household poverty situation are significant determinants of malaria morbidity. The study thus recommends that policies aimed at reducing and/or eradicating malaria should include measures to increase income earning capacity of households in the study area.
Public Interest Statement
Malaria is viewed as a disease of poverty. Globally, malaria incidence is concentrated in the world’s poorest countries, with 90% of malaria deaths occurring in sub-Saharan Africa. Malaria affects individuals’ health and lowers economic growth. Malaria has a high inequality distribution than any other disease of public health importance and continues to present a public health challenge for those in the tropics and sub-tropics. Though the disease potentially affects everyone, the poor and vulnerable are worse affected mainly due to the socio-economic constraints that confront them. This study investigates the link between poverty and malaria morbidity in the Jirapa District, Ghana. Results confirm that poverty is endemic in the study area and that malaria morbidity is significantly influenced by poverty and education. Policies aimed at eradicating malaria should include measures to increase income earning capacity of households if the Sustainable Development Goal of ending malaria by 2030 is to be achieved.
1. Introduction
The phenomenon of economic and social inequality is not new to humanity, except that its persistence between different segments of a population can entrench such negative attitudes as discrimination and cultural biases which result in social exclusion. Unfortunately, the distribution of income, for example, between households continues to be more unequal now than it was two decades ago—rising by 9.0% in developed countries and by 11.0% in developing countries. Such inequality in income often leads to inequalities in social outcomes such as education, health and nutrition. Indeed, up to 87.0% of the variation in the ratio of child mortality rates between the richest and lowest quintiles can be attributed to variations in wealth inequality (United Nations Development Programme, Citation2013).
Health inequality dates back to the days of Hippocrates, and since then health inequities and the role of social and environmental factors in the determination of marked differences in health status have been well recognized (Casas-Zamora & Ibrahim, Citation2004). Malaria currently has a higher inequality in distribution than any other disease of public health importance, as up to 58.0% of malaria deaths occur among the poorest 20.0% of the world’s population (Yadav, Dhiman, Rabha, Saikia, & Veer, Citation2014) and continues to present a public health burden in the tropical and sub-tropical regions of the world (Dako-Gyeke & Kofie, Citation2006; World Health Organisation [WHO], Citation2011). In Ghana, for example, outpatient department attendance due to malaria alone increased from 37.1% in 1985 to 44.7% in 2003 and the disease accounts for 24.6% of total hospital admission, 19.5% total bed days and 17.1% of total deaths in facilities (Adams, Darko, & Accorsi, Citation2004).
At the regional level in Africa, efforts targeted at reducing the disease burden include the Abuja Declaration in 2000 on Roll Back Malaria (RBM) and in response, Ghana set up the National Malaria Control Programme (NMCP) in order to increase access to malaria treatment (Dako-Gyeke & Kofie, Citation2006). Regrettably, these efforts are yet to yield the desired results as malaria continues to present a significant health and economic burden to countries in the tropics (Chuks & Aboh, Citation2006; United Nations Children Fund, Citation2004).
Whiles the disease potentially affects everyone, the poor and vulnerable (or the socially excluded) are worse affected. In Ghana, the Upper West Region, which is the poorest, is one of the worse affected in terms of malaria burden (Müller, Cham, Jaffar, & Greenwood, Citation1997; Tarimo, Lwihula, Minjas, & Bygbjerg, Citation2000). The poor might be affected most because of the socio-economic constraints they are faced with, which together with culture and traditions affect the way people perceive the disease regarding its cause and possible treatment (Okrah, Traore, Pale, Sommerfeld, & Müller, Citation2002). In some places in the world, cerebral malaria, for example, is commonly classified as a distinct condition attributable to supernatural forces (Winch et al., Citation1996) and such perceptions of malaria are strikingly similar across malaria-endemic areas of sub-Sahara Africa (Ahorlu, Dunyo, Afari, Koram, & Nkrumah, Citation1997; Makemba et al., Citation1996; Minja, Schellenberg, & Mukasa, Citation2001; Tarimo et al., Citation2000).
It therefore implies that malaria control requires a multidimensional approach, especially due to the fact that it is a very expensive venture for the poor to bear. As at the year 2011, the global resource requirements for malaria control were estimated to exceed US$5 billion a year between 2010 and 2015 and US$4.75 billion between 2020 and 2025 (WHO, Citation2011). The obvious implications of such staggering estimates probably explain why Africa continues to top the chart of malaria incidence as the resource constraints make it difficult for the region to achieve much in that regard. In fact, while the cost of malaria care is just 1.0% of the income of the rich, it is as high as 34.0% of the income of poor households (see, for example, Akazili, Citation2003). As such, the burden of illness from malaria rests disproportionately on the economically disadvantaged (Kuate, Citation1997).
Given social and economic factors directly relate to malaria morbidity, efforts towards reducing the disease burden need to envisage such linkages and assign specific targets at tackling poverty as part of such efforts. Unfortunately, the global malaria control strategy has largely emphasized malaria control by insecticide treated bed nets (ITNs), indoor residual spraying (IRS), increased case detection and subsequent treatment, as well as other vector control interventions while neglecting the socio-economic deprivation from which people have difficulty in adequately handling malaria (Yadav et al., Citation2014). This study thus seeks to add to the literature on the subject matter by investigating the linkages between poverty and malaria morbidity in the Jirapa District of the Upper West Region of Ghana.
2. Review of related literature
It is increasingly becoming common knowledge that poor people are at risk of not only becoming infected with malaria but also becoming infected more frequently largely due to the fact that poor families cannot afford dwellings that offer some protection against mosquitoes and are less likely to be able to afford insecticide treated nets, pay either for effective treatment or for transportation to facilities capable of treating the disease. Indeed, the disease is largely understood to be one of poverty and a cause of poverty, a situation which makes the disease such a public health problem across the world, especially as an estimated 40.0% of the world’s population lives in areas prone to malaria (Adams et al., Citation2004; WHO, Citation2013).
Malaria causes poverty through its effects on diverse features of human existence including mobility, investment choices, fertility decisions and indeed every fabric of human existence. The effect of the disease is quite large and burdensome on the “poorest of the poor”; the costs of prevention, treatment and loss of productivity resulting from malaria related morbidity and mortality usually represent a significant proportion of the annual income of poor agricultural households. Indeed, the disease significantly affects long-term economic growth and development such that promoting a cycle of health and wealth in an economy, resulting in improved standard of living may only be possible through the reduction in malaria burden. The link between malaria and poverty is one that is intimate the world over; the per capita GDP, adjusted for differences in purchasing power, in highly malarious countries is on average one-fifth that of non-endemic countries (Malaney, Spielman, & Sachs, Citation2004).
Malaria causes poor health and subsequently impacts negatively on the growth of affected countries. The disease is one of the major causes of food insecurity and thus chronic malnourishment in sub-Saharan Africa. It costs the continent more than $12 billion annually and may have slowed down economic growth by up to 1.3% per year, constitutes 10% of overall disease burden in sub-Saharan Africa, it is the leading cause of mortality in children under five years, and accounts for about 40.0% of public health expenditure (Asenso-Okyere, Asante, Tarekegn, & Andam, Citation2009).
In Ghana, for example, the disease is said to be hyper-endemic, resulting in between 30 and 40% of outpatient attendance and constituting one of the leading causes of deaths. Private expenditure on health as a percentage of total expenditure on health stood at 65.8% for Ghana, 52.9% for Africa and 42.4% for the world (Asenso-Okyere et al., Citation2009; Ghana Health Service, Citation2011).
Given malaria is responsible for a substantial amount of public health expenditure, an equally substantial reduction in malaria incidence should free up available health resources, facilities and workers’ time to tackle other health problems. Sociocultural factors such as individual education level, people’s perception, age, income and gender roles also influence the level of commitment to malaria control and hence malaria morbidity (Boadu & Trovato, Citation2006; Donovan, Siadat, & Frimpong, Citation2012).
Empirical studies have shown, for example, that pregnant women, who would normally be between the ages 15 and 49 years, have been identified as the most adult group at risk of malaria (Boadu & Trovato, Citation2006; RBM, Citation2001), and the proportion of female household heads with malaria is found to be higher compared to the proportion for male household heads in both the rural and urban areas (Boadu & Trovato, Citation2006).
Level of education has also been found to affect malaria control efforts and morbidity. The Kenya National Bureau of Statistics (Citation2011), for example, reported that women with secondary or higher education were twice (34.0%) as likely as those with no education (17.0%) to receive Intermittent Preventive Treatment in pregnancy (IPTp).
Household size is not only an important correlate of poverty but also has a direct bearing on the incidence of malaria. For example, it is suggested that as average household sizes continue to decline around the world, malaria would also gradually disappear (Hulden, McKitrick, & Hulden, Citation2012).
In a review of empirical studies, Worrall, Basu, and Hanson (Citation2003) found that in Nigeria, malaria burden is heavier on the poor than on the rich as individuals with mean incomes below US$1/day were less likely to perceive malaria as a preventable disease, more likely to report having fever presently and suffered significant bouts of malaria per month compared to individuals earning greater than US$1/day. Some empirical studies (see, for example, Usman & Adebayo, Citation2011) support this fact as up to 40.8, 27.7, 17.5 and 11.0% of households headed by individuals aged between 41 to 50 years, 51 to 60 years, less than 30 years and 61 to 70 years, respectively, were found to be infected with malaria over the last 12 months. Usman and Adebayo (Citation2011) further found that about 74.2% of households headed by married people were infected with malaria over the last 12 months and that about 8.5% of households headed by males were infected compared to 12.4% of households headed by females.
Empirical studies in Ghana (including Afudego, Citation2011; Nyarko & Cobblah, Citation2014) point to the fact that the group affected most by malaria are children under-five (accounting for 20% of the population) and pregnant women (accounting for 4% of the population). The crude parasite rates are found to range from 10 to 70%, with Plasmodium falciparum accounting for 90–98%, Plasmodium malariae 2–9% and Plasmodium ovale 1% of all infections, respectively (Afudego, Citation2011). In their study, Nyarko and Cobblah (Citation2014) found that the highest proportion (22.7%) of malaria under-five was reported among children whose mothers had primary school education, while mothers with higher education reported the lowest (17.4%) malaria cases among such children. The study further found the highest proportion of cases was reported among children whose mothers were from poor households, pointing to the fact that solving the malaria problem even among children might better be done through social and economic interventions rather than scientific-based interventions alone.
3. Methodology
3.1. Study location, design and sampling
The Jirapa District is one of the 10 districts in the Upper West Region of Ghana. It is located in the tropical continental climate regime with mean annual temperature ranging between 28 and 31°C. The vegetation is generally the guinea savannah woodland with light undergrowth scattered medium size trees. The district is not well drained as no major rivers are found except the intermittent tributaries of the Black Volta River. These are Kaabaa around Ullo, Bakpong near Baazu, Dazugri in Jirapa and Telenbe at Tizza. In the long dry season, these tributaries dry up leaving the district with no surface water catchment for domestic and agricultural purposes. The valleys of these tributaries are suitable for the development of small-scale irrigation dams and dug-outs for dry season gardening, fishing and watering of animals, especially cattle. There are small-scale dams and dug-outs scattered throughout the district. Konzokala, Tizza, Jirapa and Ullo are some of the places where there are dams and dug-outs. Topographically, the landscape of the district is generally flat and low-lying with average height of 300 m above the sea level. There are few plateau surfaces ranging between 1,000 and 1,150 ft. These are found in Yagha and Jirapa (Ghana Statistical Service, [GSS], Citation2014).
The population of Jirapa District, according to the 2010 Population and Housing Census, is 88,402 representing 12.6% of the region’s total population. Males constitute 47.0% and females 53.0%, giving a sex ratio of 88.7. About 85.6% of the population live in rural localities. The population of the district is youthful (under 15 years) (42.8%) depicting a broad-based population pyramid which tapers off with a small number of elderly persons 60 years and above (9.3%). The total age dependency ratio for the District is 99.0, the dependency ratio in the rural localities is higher (105.8) than that of the dependency ratio in the urban areas (66.44) (GSS, Citation2014).
The study used the survey design and employed a multi-stage sampling procedure to select 200 households. Using the proportion of 85.6% for households in rural localities in the district (GSS, Citation2014), Z-value of 1.96 for the 95% confidence level, 0.05 confidence interval and the formula for sample size determination by Godden (Citation2004)Footnote1, a sample size of 189.41 (approximated to 200) was arrived at.
The Jirapa District has seven sub-districts for the purpose of health care delivery. These are Han, Ulio, Saboli, Jirapa Urban, Duori, Tugo and Yaga. The Jirapa urban sub-district was selected for data collection using the simple random procedure. Groups, albeit informal, were identified in communities within the sub-district and where possible group list was used to randomly sample 20 heads of households in each of 10 localities as respondents. Data were then obtained through questionnaire administration and interviews and malaria morbidity were reported cases at the household level, rather than from a health institution because of the difficulty of tracing these households from the hospitals.
3.2. Context and definition of variables
The focus of this study is the household rightly so because of the critical role the household plays in making critical health decisions for its members. In particular, the social orientation and economic position of the household head is critical in deciding what constitutes sickness, the cause(s) of such a sickness, the type of treatment to seek and where to seek treatment. The combined effects of such chain of actions could influence the general morbidity or otherwise of the household. In all, 200 households were selected out of the 1,785 households (GSS, Citation2012).
Given most households in the study area rely on farming as their principal means of livelihood, household heads do not only have total control over household resources, but they also decide how these resources are distributed in securing various household needs. In this regard, it is important to investigate how the socio-economic status of the household head affects household’s decisions regarding malaria control and how such decisions influence the morbidity of household members due to malaria.
The level of education of the household head is critical because people’s orientation changes with education and this, in turn, affects their healthcare-seeking behaviour. Specifically, as people’s level of education increases in terms of the number of years spent in school, their awareness of the disease also increases (Dufie, Citation2010), thereby placing the household in a better position to take steps to control the disease leading to low morbidity. In this study, the educational level of the head of household is measured by the last grade completed.
Similarly, the poverty situation of the household is estimated using the income level of household head. Household income is sometimes used as a measure of household welfare (Worrall et al., Citation2003). In this study, income level of household head is preferred because as a result of limited economic opportunities in the area, farming remains the major source of income and the head of household holds output from farming in trust for the household. Further, because the head is ultimately responsible even when the household runs out of these farm resources the general welfare of the household still depends on the head’s ability to raise additional income, where possible. Thus, the income of the head is the most representative of the income of the household. Also, income influences the ability of a household to afford anti-malarial drugs and preventive tools such as ITNs and insecticide spray to help treat or fight malaria. Indeed, it affects the extent to which the household is able to provide clothes for children to wear during the day and at night, and how much the bodies of such children are exposed to mosquito bites.
Gender is perceived to be an important factor in explaining morbidity, especially at the household level. The fact that a household head is a male may likely affect the number of malaria cases that household suffers since men own more of the economic resources (Boadu & Trovato, Citation2006), and depending on how these resources are applied to fighting malaria by the head of household, malaria infections may go up or decrease. Gender is also sometimes used as a proxy for socio-economic status (Worrall et al., Citation2003), reflecting the social and material disadvantage experienced by women in many parts of the world.
Household size is measured in terms of number of people living in the household. A household is defined as a person or a group of persons, who live together in the same house or compound and share the same housekeeping arrangements. Thus, it may consist of a man, his wife, children and some other relatives or a house help who may be living with them (GSS, Citation2012). It is expected that when average household size drops, the number of malaria infections in a household will also decline (Hulden et al., Citation2012). In the typical farming community where household members depend primarily on one source of livelihood, the ability of the household to deploy resources to purchasing inputs such as mosquito nets, decent accommodation and other preventive methods is impeded by other equally important livelihood issues such as proper feeding, paying children’s school bills and clothing.
3.3. Empirical model
The study employs both descriptive and inferential statistics. Descriptive statistics allows for the exploration of the data and explanation of the socio-economic attributes of respondents. Additionally, it serves as good basis for the subsequent inferential statistics since interrelationships among variables can only be successfully explained when put in their proper socio-economic contexts.
The outcome variable, malaria morbidity, refers to the number of people falling ill due to malaria in a typical household. It is thus a discrete nonnegative integer-valued count variable which requires the use of count data regression. Given the counts of malaria morbidity occur randomly, the appropriate probability distribution is the Poisson distribution. Malaria morbidity at any given period, , subject to household socio-economic attributes xi, can thus be modelled using the basic Poisson model as (Cameron & Trivedi, Citation2010; Greene, Citation2008; Maddala, Citation1983; Winkelmann, Citation2008):
(1)
(1)
From Equation (1), the parameter , and the mean is usually defined as
, where
is a vector of characteristics specific to household
, and
is a vector of unknown parameters to be estimated. The marginal (or partial) effects in the Poisson model are given by:
(2)
(2)
This marginal effect, as in other count data models, is interpreted as the unit change in the morbidity variable resulting from a change in the explanatory variable (Cameron & Trivedi, Citation1998).
However, the basic Poisson model although attractive for use in empirical studies suffers a major shortcoming of assuming equality between the variance of the count-dependent variable and its conditional mean, called the equi-dispersion assumption (Cameron & Trivedi, Citation1998; Winkelmann, Citation2008). In most empirical studies, however, the count-dependent variable has been observed to exhibit over-dispersion, a typical feature of most health count data resulting from the presence of many zero observations, which implies the variance of the count-dependent variable is greater than the conditional mean (Congdon, Citation2013). To be able to handle over- or under-dispersion, the generalized Poisson model is employed in this study. The generalized Poisson model is a flexible form allowing for both over- and under-dispersion with equi-dispersion (as in the Poisson model in Equation (1)) as a special case. The flexibility in the model emanates from the addition of an extra parameter, μi, so that the resultant probability distribution function for the model is given by (Cameron & Trivedi, Citation1998; Consul, Citation1989; Winkelmann, Citation2008):
(3)
(3)
where ,
and
is the largest positive integer for which the inequality
is satisfied when
is negative. The mean and variance for the distribution are, respectively,
and
so that the distribution exhibits underdispersion for
, overdispersion for
and nests the basic Poisson distribution as a special case for which
.
Letting and
, the probability density for generalized Poisson model can be parameterized as (Greene, Citation2007):
(4)
(4)
The mean and variance are then given by and
. The marginal effects in this model are thus identical to that of the basic Poisson model (in Equation (2)). The constant
serves as the dispersion parameter with θ < 0 and θ > 0 denoting under- and overdispersion, respectively, but θ = 0 reduces the model to the basic Poisson model.
Finally, the empirical model estimated in this study is of the form:
(5)
(5)
where = number of people (count) falling ill due to malaria in household
(i.e. malaria morbidity);
= personal characteristics;
= educational characteristics; and
= poverty situation variables.
4. Results
4.1. Socio-economic characteristics of household heads
The study employs the household as the unit of analysis because the study area is largely a farming community where the head of household wields a lot of social, cultural and economic power which ultimately affects the daily welfare of the household. This makes it imperative to explore the characteristics of the household heads and how such characteristics subsequently affect households’ malaria morbidity.
From Table , the educational level of household heads is low, as up to 29.0% of them have never had any formal education, while over 42.0% attained only primary education. All together only a little over 28.0% attained at least secondary education with only 12.5% completing tertiary education. This apparent low level of education poses a difficulty regarding the households’ ability to adequately put in place malaria control measures, given that formal education is expected to influence peoples’ perception positively about the causes of malaria and available treatment (Worrall et al., Citation2003).
Table 1. Characteristics of household heads
The age distribution of household heads reveals that most households are headed by relatively younger persons, most of whom are men. From the results, a little below 20.0% of household heads are aged 50 years and above while the rest are younger than 50 years.
Similarly, up to 59.0% of households are headed by men while the rest of the 41.0% are headed by women. According to Boadu and Trovato (Citation2006), men and women do not have exactly the same aspirations and the fact of a household head being a male or female has an influence on the commitment level to malaria control. In their opinion, the proportion of female-headed households with malaria is higher compared to that of male-headed households in both the rural and urban areas, reflecting the differences in commitment level to malaria control and prevention for the two sexes.
Another variable of interest is income. This variable is chosen instead of consumption because the data on consumption had over 65% missing values. This is mostly due to the fact these households consume from their farm produce rather than from the market and so find it difficult to quantify and/or put value to their consumption. The income level of the household reveals that many of these household heads fall below the poverty line. Indeed, up to 52.0% of household heads had incomes falling below the poverty line since they earned incomes below US$1.00 per day or GHS 3.94.Footnote2 Put together, up to 52.0% of household heads had incomes below $1.00 (or GHS 3.94) a day or US$31.00 (or GHS 122.14) a month. Those who earn between $31.00 (or GHS 122.14) a month and US$155.28 (or GHS 611.80) also referred to as being in the lower middle income, are a little above 24.0% of the sample, while 23.5% are classified as high middle income.
This result clearly depicts the presence of poverty in the study area as more than half of households depend on people whose incomes are below the poverty line of US$1 per day. Income levels, and for that matter the poverty levels, of households have implications for malaria morbidity. For example, Dako-Gyeke and Kofie (Citation2006) are of the view that income level of the household head inversely relates to malaria morbidity as high income earners are able to implement malaria prevention and control measures.
4.2. Malaria morbidity and control measures
Malaria morbidity in the study area is between 1 person per household to 6 persons per household in a year, even though 35 households (or 17.5% of households) did not experience any morbidity due to malaria. Overall, 14.5% of households experienced a single episode of morbidity due to malaria, 18.5 and 17.5% of households, respectively, experienced 2 and 3 episodes each, 13.5% of households experienced 4 episodes, 10.0% of households experienced 5 episodes, while 8.5% of households experienced the highest episodes of 6 (see Table ).
Table 2. Malaria morbidity and modes of control
The most effective way to control malaria is to avoid mosquito bites. Sleeping under nets, using mosquito coil, mosquito repellent and insecticide spray are some of the ways one can avoid mosquito bites. From Table , a higher proportion of households (i.e. 63%) chose to sleep under the mosquito net as a way of controlling malaria. This is possibly due to Ghana’s national policy to supply free insecticide treated nets (ITN) to all households in the country, a policy which started in 2012. Following such a policy, one would have expected that every household uses a net, but some households find it uncomfortable to use, especially in the warm season and hence resort to other alternative methods such as the coil, spray and repellent even though the use of these methods are not mutually exclusive; some households employ more than one method.
4.3. Determinants of households’ malaria morbidity
The results in Table reveal that gender of household head and level of education are positive and significant determinants of malaria morbidity, while household income is a negative and significant determinant of malaria morbidity. Given poverty is measured by income of household head, it implies households whose head earn less income are likely to experience high morbidity cases. In other words, household poverty affects malaria morbidity positively. The results can also be said to be reasonably uniform across the Poisson and the generalized Poisson models.
Table 3. Results of count data models
However, a number of formal tests of dispersion revealed the existence of underdispersion. The underdispersion in the data-set emanates from the fact that only about 17.5% of the surveyed households had no case of morbidity, implying a few zeros recorded for the count-dependent variable. Results in Table indicate two of the statistics developed by Cameron and Trivedi (Citation1990), and discussed by Greene (Citation2007), for testing for dispersion in the Poisson count data model, and
, are −8.694 and −11.027, respectively. The two statistics have limiting chi-squared distributions with one degree of freedom under the null hypothesis of equidispersion, thereby giving a critical value of 6.635 at the 0.01 level. The absolute values of both statistics exceed the critical value. The equidispersion assumption is therefore rejected, and the fact that both values are negative indicates underdispersion. The results of the generalized Poisson model, as shown in Table , also confirm there is underdispersion in the data since the dispersion parameter is negative and statistically significant. The rest of the discussion of the results is thus based on the chosen generalized Poisson model.
Given coefficient estimates do not provide much meaningful interpretation by themselves, the estimated parameters are transformed into marginal effects. The computed marginal effects give the magnitude of the change in the morbidity level of a particular household when the explanatory variable changes by 1 unit.
The results show that male-headed households (since gender = 1 for male and zero otherwise) have 0.5542 more episodes of morbidity compared to female-headed households. Women are responsible for child bearing and upbringing, which responsibility also makes them more vulnerable (especially during pregnancy) to malaria than men and consequently more responsible in taking steps to control malaria. Another plausible explanation has to do with how resources are allocated at the household level, especially to fighting malaria (Boadu & Trovato, Citation2006). From this results, one can infer that male household heads in the study area do not allocate enough resources to fighting malaria given majority (59.0%) of household heads of the study sample are men. If enough resources were allocated to fighting malaria by male household heads, one would expect, all things being equal, that morbidity would drastically decrease.
Even though the level of education of the household head significantly relates to malaria morbidity in the study area, the positive sign contradicts findings from some previous studies (see, for example, Dufie, Citation2010; Worrall et al., Citation2003). This observation is likely due to the probable low income effect education may have on households in the study area. From the summary statistics (Table ), only 12.5% of household heads attained education beyond secondary level. Indeed, level of education is only relevant if it translates to increased income which enhances the capacity of the household to fight malaria or if it influences the set of attitudes and behaviour of people in ways that are favourable to fighting malaria. The apparent mismatch between the two seems to account for the unexpected sign.
Household poverty also significantly affects morbidity. Compared to households below the poverty line (less than US$1.00 per day), those in the lower middle income and upper middle income are less likely to witness increases in malaria morbidity. This confirms the assertion that poverty tends to impede households’ ability to invest in malaria control. The interaction variable of income and education is not significant.
5. Concluding remarks
The linkage between social and economic conditions and malaria morbidity is so apparent. Yet, not much has been done in terms of research and the global efforts at controlling malaria regarding the recognition of such linkages and the possible implications in terms of fighting malaria. In this study, the linkage between poverty and malaria morbidity is investigated in the Jirapa District of the Upper West Region of Ghana, using data from a total of 200 households.
The empirical results confirm that there is poverty in the study area as more than half of the surveyed households depend on people whose incomes are below the poverty line of US$1 per day and that significant relationships exist between poverty and education on the one hand and malaria morbidity on the other, since gender of household head and level of education and household poverty situation are significant determinants of malaria morbidity.
The study thus recommends that the income earning capacity of the people in the study area, and indeed at the national level, be improved by institutions and stakeholders whose agenda is the reduction and/or eradication of malaria. In addition, women, especially at the household level should be empowered in roles and strategies of malaria control as female-headed households tend to record lower episodes of morbidity compared to male-headed households.
Funding
The authors received no direct funding for this research.
Acknowledgements
The authors wish to thank Philip Tenglee, Amidu Wahabu, Benjamin Twumasi, Richard Wulo and Winter Yacham for their assistance with data collection.
Additional information
Notes on contributors
Paul Kwame Nkegbe
Paul Kwame Nkegbe is a senior lecturer at the Department of Economics and Entrepreneurship Development, University for Development Studies, Ghana, where he teaches courses in economics and applied statistics at both the undergraduate and graduate levels. His research focuses on applied microeconomic analysis. He currently serves as an editorial secretary to the Ghana Journal of Development Studies, and he is also a member of the Royal Economic Society.
Naasegnibe Kuunibe
Naasegnibe Kuunibe holds MPhil in Economics and currently a PhD candidate in Health Economics at the University of Heidelberg, Germany. His main research activities are in the area of applied microeconomics. He has researched and published on various themes relating to health economics, natural resource economics and generally development economics.
Samuel Sekyi
Samuel Sekyi holds MPhil in Economics from the University of Cape Coast, Ghana. He currently lectures at University for Development Studies, Ghana. He has published in both local and international peer-reviewed journals. His speciality includes econometrics, international economics and health economics.
Notes
1. For infinite population (i.e. 50,000+), the formula is given as , where z, p and c denote z-value, percentage of households in rural areas in the district and confidence interval expressed as a decimal (in this case 0.05 or ± 0.05), respectively.
2. The rate as quoted by www.oanda.com as at 15 September 2016.
References
- Adams, I., Darko, D., & Accorsi, S. (2004). The burden of malaria: Patterns and trends. Bulletin of Health Information. Retrieved from http://www.ghanahealthservice.org
- Afudego, C. E. (2011). Strengthening institutions to improve public expenditure accountability: Cost efficiency analysis of Insecticide Treated Mosquito Nets (ITNs) and Indoor Residual Spraying (IRS)—Malaria Interventions in Ghana (Children under Five). Retrieved from http://www.isodec.org.gh/publications/Final%20-%20HEALTH%20CEA%20Report%20June
- Ahorlu, C. K., Dunyo, S. K., Afari, E. A., Koram, K. A., & Nkrumah, F. K. (1997). Malaria-related beliefs and behaviour in southern Ghana: Implications for treatment, prevention and control. Tropical Medicine and International Health, 2, 488–499.10.1111/tmi.1997.2.issue-5
- Akazili, J. (2003). Costs to households of seeking malaria care in the Kassena-Nankana District of Northern Ghana. Presented at the Third Multilateral Initiative on Malaria (MIM) Pan-African Conference on Malaria, Arusha.
- Asenso-Okyere, K., Asante, F. A., Tarekegn, J., & Andam, K. S. (2009). The linkages between agriculture and malaria: Issues for policy, research and capacity strengthening (Discussion Paper 00861). Washington, DC: International Food Policy Research Institute.
- Boadu, K., & Trovato, F. (2006). Association of social class with malaria incidence among household heads in Ghana. Canadian Studies in Population, 33, 271–299.
- Cameron, A. C., & Trivedi, P. K. (1990). Regression-based tests for overdispersion in the Poisson model. Journal of Econometrics, 46, 347–364.10.1016/0304-4076(90)90014-K
- Cameron, A. C., & Trivedi, P. K. (1998). Regression analysis of count data. Cambridge: Cambridge University Press.10.1017/CBO9780511814365
- Cameron, A. C., & Trivedi, P. K. (2010). Microeconometrics using stata. College Station, TX: StataCorp LP.
- Casas-Zamora, J. A., & Ibrahim, S. A. (2004). Confronting health inequity: The global dimension. American Journal of Public Health, 94, 2055–2058.10.2105/AJPH.94.12.2055
- Chuks, J. M., & Aboh, I. K. (2006). Incidence and management of malaria in Ghana: A case study in Volta Region. African Population Studies, 22, 137–165.
- Congdon, P. (2013). Spatially interpolated disease prevalence estimation using collateral indicators of morbidity and ecological risk. International Journal of Environmental Research and Public Health, 10, 5011–5025. doi:10.3390/ijerph10105011
- Consul, P. C. (1989). Generalized Poisson distributions: Properties and applications (Vol. 99 of statistics: Textbooks and monographs). New York, NY: Marcel Dekker.
- Dako-Gyeke, M., & Kofie, H. (2006). Examining factors that influence malaria prevention and control practices among pregnant women residing in slum area in Southern Ghana. Accra: Department of Social Work, University of Ghana.
- Donovan, C., Siadat, B., & Frimpong, J. (2012). Seasonal and socio-economic variations in clinical and self-reported malaria in Accra, Ghana: Evidence from facility data and a community survey. Ghana Medical Journal, 46, 85–94.
- Dufie, A. G. (2010). Factors influencing the uptake of intermittent preventive treatment of malaria in pregnancy in the Bosomtwe District of Ghana. Kumasi: Department of Community Health, School of Medical Sciences, KNUST.
- Ghana Health Service. (2011). The health sector in Ghana: Facts and figures 2010. Retrieved from http://www.moh-ghana.org
- Ghana Statistical Service. (2012). 2010 population and housing census: Summary reports and final results. Accra: Author.
- Ghana Statistical Service. (2014). 2010 Population and housing census. District analytical report: Jirapa District. Accra: Author.
- Godden, W. (2004). Formula for sample size determination. Retrieved from www.williamgodden.com/samplesizeformula.pdf
- Greene, W. H. (2007). Econometric modelling guide. New York, NY: Econometric Software Inc.
- Greene, W. (2008). Econometric analysis (6th ed.). New York, NY: Pearson.
- Hulden, L., McKitrick, R., & Hulden, L. (2012). Average household size and the eradication of malaria. Retrieved from http://www.uoguelph.ca/economics/research/discussion-papers
- Kenya National Bureau of Statistics. (2011). 2010 Kenya malaria indicator survey. Nairobi: Division of Malaria Control, Ministry of Public Health and Sanitation.
- Kuate, D. B. (1997). Effects of socioeconomic advantage and women’s status on women’s health in Cameroon. Social Science and Medicine, 44, 1023–1042.
- Maddala, G. S. (1983). Limited-dependent and qualitative variables in econometrics. New York, NY: Cambridge University Press.10.1017/CBO9780511810176
- Makemba, A. M., Winch, P. J., Makame, V. M., Mehl, G. L., Premji, Z., Minja, J. N., & Shiff, C. J. (1996). Treatment practices for degedege, a locally recognized febrile illness, and implications for strategies to decrease mortality from severe malaria in Bagamoyo District, Tanzania. Tropical Medicine and International Health, 1, 305–313.
- Malaney, P., Spielman, A., & Sachs, J. (2004). The malaria gap. American Journal of Tropical Medicine and Hygiene, 71, 141–146.
- Minja, H., Schellenberg, J. A., & Mukasa, O. (2001). Introducing insecticide-treated nets in the Kilombero Valley, Tanzania: The relevance of local knowledge and practice for an Information, Education and Communication (IEC) campaign. Tropical Medicine and International Health, 6, 614–623.10.1046/j.1365-3156.2001.00755.x
- Müller, O., Cham, K., Jaffar, S., & Greenwood, B. (1997). The Gambian national impregnated bednet programme: Evaluation of the 1994 cost recovery trial. Social Science and Medicine, 44, 1903–1909.10.1016/S0277-9536(96)00299-7
- Nyarko, S. H., & Cobblah, A. (2014). Sociodemographic determinants of malaria among under-five children in Ghana. Malaria Research and Treatment, 2014, 6 p. doi:10.1155/2014/30436
- Okrah, J., Traore, C., Pale, A., Sommerfeld, J., & Müller, O. (2002). Community factors associated with malaria prevention by mosquito nets: An exploratory study in rural Burkina Faso. Tropical Medicine and International Health, 7, 240–248.10.1046/j.1365-3156.2002.00856.x
- Roll Back Malaria. (2001). Report on the Zambia Roll back malaria baseline study undertaken in 10 Sentinel Districts. Lusaka: RBM National Secretariat.
- Tarimo, D. S., Lwihula, G. K., Minjas, J. N., & Bygbjerg, I. C. (2000). Mothers’ perceptions and knowledge on childhood malaria in the holendemic Kibaha district, Tanzania: Implications for malaria control and the IMCI strategy. Tropical Medicine & International Health, 5, 179–184.10.1046/j.1365-3156.2000.00537.x
- United Nations Children Fund. (2004). Malaria, a major cause of child death and poverty in Africa. Retrieved from http://www.unicef.org/publications/files/malaria_rev_5296_Eng.pdf
- United Nations Development Programme. (2013). Humanity divided: Confronting inequality in developing countries. New York, NY: United Nations Development Programme Bureau for Development Policy.
- Usman, A., & Adebayo, M. O. (2011). Socio-economic impact of malaria epidemics on households in Nigeria: Micro evidence from Kwara State. International Journal of Asian Social Science, 1, 188–196.
- Winch, P. J., Makemba, A. M., Kamazima, S. R., Lurie, M., Lwihula, G. K., Premji, Z., ... Shiff, C. J. (1996). Local terminology for febrile illnesses in Bagamoyo District, Tanzania and its impact on the design of a community-based malaria control programme. Social Science and Medicine, 42, 1057–1067.10.1016/0277-9536(95)00293-6
- Winkelmann, R. (2008). Econometric analysis of count data. Berlin Heidelberg: Springer-verlag.
- World Health Organisation. (2011). World malaria report 2011. Geneva: Author. Retrieved from http://www.who.int/malaria/world_malaria_report_2010/worldmalariareport2011
- World Health Organisation. (2013). World malaria report. Retrieved from http://www.who.int
- Worrall, E., Basu, S., & Hanson, K. (2003). The relationship between socio-economic status and malaria: A Review of the literature. A background paper for “ensuring that malaria control interventions reach the poor”, London.
- Yadav, K., Dhiman, S., Rabha, B., Saikia, P. K., & Veer, V. (2014). Socio-economic determinants for malaria transmission risk in an endemic primary health centre in Assam, India. Infectious Diseases of Poverty, 3, 19. doi:10.1186/2049-9957-3-19