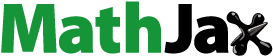
Abstract
In this paper, we empirically investigate the causal nexus between economic growth (GDP), CO2 emissions (environmental degradation), financial development, and trade openness using the ordinary least squares technique for a yearly panel data of 40 European economies, during the period of study from 1985 to 2014. To examine this causal link, we utilize the Cobb–Douglas production function. The empirical findings point to a bidirectional Granger causal linkage among GDP and pollution, GDP and financial sector development, GDP and trade openness, financial sector development and trade openness, and trade openness and pollution in the case of European economies. From the causal link between GDP and environmental pollutants, we validate the existence/confirm the validity of the environmental Kuznets curve hypothesis. Also, we confirm/bear out the feedback suggestion of the bidirectional causality among trade openness and financial sector development. Besides, we find the neutrality hypothesis linking carbon emissions and financial sector development inflows. We find the presence of the bidirectional nexus between GDP and financial sector development and among GDP and trade openness in the European economies. Finally, panel causality verifies that bidirectional causal connection is found between economic growth, environmental degradation (CO2), financial development, and trade openness.
Public Interest Statement
This paper investigates empirically the causal nexus between economic growth, pollution, financial development, and trade openness in the European economies. The empirical findings point to a bidirectional Granger causal linkage among the four indicators GDP, pollution, financial sector development, and trade openness. We validate the existence of the environmental Kuznets curve hypothesis between GDP and environmental pollutants. We confirm out the feedback suggestion of the bidirectional causality among trade openness and financial sector development. We find the neutrality hypothesis linking pollution and financial development inflows. We find the presence of the bidirectional nexus between GDP and financial development and among GDP and trade openness.
1. Introduction
Over the past two decades, the topic of fundamental link between energy consumption and macroeconomics variables has been examined by many researchers (Fodha & Zaghdoud, Citation2010; Jamel & Derbali, Citation2016; Jaunky, Citation2010; Kahia, Ben Aïssa, & Charfeddine, Citation2016, Citation2017; Ozturk & Acaravci, Citation2010; Saboori, Sulaiman, & Mohd, Citation2012). Numerous studies have analyzed the causal relationship between energy consumption and several independent variables such as economic growth, financial development, employment, and population (Jamel & Derbali, Citation2016; Kahia et al., Citation2016, Citation2017). Then, energy is considered to be the life line of an economy, the most vital mechanism of socioeconomic development and renowned as one of the most important strategic commodities.
In this alignment, the link between GDP and energy consumption has been investigated empirically by different significant academic researches during the last few decades. Various researchers have been interested in yearly data for several countries by utilizing a diversity of econometric techniques and numerous proxy indicators which have been employed for the causal relationship between CO2, energy consumption, and GDP (Apergis & Payne, Citation2009; Baranzini, Weber, Bareit, & Mathys, Citation2013; Ghosh, Citation2010; Stern, Citation1993; Wolde-Rufael, Citation2005; Yuan, Zhao, Yu, & Hu, Citation2007). However, their main empirical conclusions are diverse and have not indicated unique results (Chen, Kuo, & Chen, Citation2007; Omri, Citation2014). Farhani, Shahbaz, Sbia, and Chaibi (Citation2014) use the procedure of Granger causality test to empirically examine the link between energy consumption and GDP.
This problem has led some of which recent Granger causality-based studies investigating the causal relations among energy consumption and economic growth to incorporate capital and labor in the multivariate models. Recently, some other studies have incorporated trade openness in the production function in order to investigate the link between economic growth, capital, labor, and trade.
More recently, a few readings have studied the causal association between CO2 emissions, energy consumption, and GDP. However, these empirical findings have demonstrated mixed results, which make an encouragement for supplementary study to clarify this causal linkage. Numerous recent studies confirm the presence of the relationship among GDP, CO2 emissions, energy consumption, financial sector development, and trade openness by utilizing numerous econometric method as (1) the structural break unit root test; (2) the co-integration test for long-run linkage among the variables; (3) the ordinary least squares (OLS) technique and the error–correction model for long-run and short-run impacts; (4) the vector error–correction model (VECM) with Granger causality method for causal link; and (5) innovative accounting approach to study the robustness of causality analysis.
In this context, the main idea of this study is to examine empirically the causal relationship between GDP, CO2 emissions, financial sector development, and trade openness. For the econometric method, we utilize the OLS technique to investigate this nexus for a yearly panel data of 40 European economies during the period of study from 1985 to 2014. The empirical findings indicate the existing of bidirectional causality among GDP and financial sector development, between GDP and environmental pollutants, between GDP and trade openness, between financial sector development and trade openness, and among trade openness and environmental pollutants. However, we remark the inexistence of the causal relation between the financial sector development and environmental pollutants. Finally, panel causality verifies that bidirectional causal connection is found between economic growth, environmental degradation (CO2), financial development, and trade openness. These results are in conformity with the previous studies.
The rest of this study is organized as follow: in Section 2, we show a review of previous literature on the nexus between GDP, financial sector development, CO2 emissions, and trade openness. In Section 3, we explore the econometric method utilized in our paper. In Section 4, we present the empirical results by studying the data description of all variables, the stationarity of variables, and providing the empirical findings analysis. Section 5 finds concluding remarks. The policy implications are showed in Section 6. Finally, Section 7 provides the Suggestions for future research.
2. Literature review
A host of researchers are concerned with the empirical examination of the presence of bidirectional causal nexus among GDP, energy consumption, environmental degradation, financial sector development, and trade openness.
The relationship between GDP and CO2 emissions is investigated in various empirical studies. This link is focused on the EKC hypothesis. This hypothesis supposes that the causal nexus between GDP and environmental pollutants is significant with an extremely positive sign. Grossman and Krueger (Citation1991) and Selden and Song (Citation1994) confirm that the connection between GDP and pollution is positively significant with different threshold level. Their empirical conclusions prove that an increase in economic growth augment the environmental degradation as measured by the CO2 emissions.
Additionally, Azomahou, Laisney, and Van (Citation2006) corroborate the existence of a linear causal connection between GDP and CO2 emissions. Lean and Smyth (Citation2010) and Saboori et al. (Citation2012) illustrate the existence of an inverted U-shaped liaison linking GDP and pollution.
The study developed by Friedl and Getzner (Citation2003) indicates an N-shaped nexus between GDP per capita and CO2 emissions. But, Richmond and Kaufmann (Citation2006) show a non-causal link among GDP and CO2 emissions.
The relationship connecting GDP and environmental pollutants can be examined by reference to the EKC hypothesis. The EKC can explain the causal linkage between GDP and pollution since the 1990s. Moreover, Grossman and Krueger (Citation1991) and Selden and Song (Citation1994) demonstrate that economic development as measured by the GDP per capita leads to a gradual pollution. This influence is acquired in the initial stages and after a certain threshold of economic growth. The EKC hypothesis maintains that the link between economic growth and pollution is nonlinear and takes the form of inverted-U shape. This empirical finding indicates that economic growth is correlated with an augment in environmental degradation initially and reduces it.
The EKC hypothesis is examined empirically by several researches which demonstrate contradictory empirical findings (Ekins, Citation1997; Fodha & Zaghdoud, Citation2010; Heil & Selden, Citation1999; Jaunky, Citation2010; Managi & Jena, Citation2008; Ozturk & Acaravci, Citation2010; Saboori et al., Citation2012; Stern, Common, & Barbier, Citation1996).
For specific country studies, we present the studies elaborated by Ang (Citation2008), Soytas and Sari (Citation2009) and Fodha and Zaghdoud (Citation2010) for the case of Malaysia, Turkey, and Tunisia, respectively, which find the existence of bidirectional causality linking GDP and environmental pollutants. However, Ang (Citation2007), Jalil and Mahmud (Citation2009), Nasir and Rehman (Citation2011), and Saboori et al. (Citation2012) for France, China, Pakistan, and Malaysia, respectively, demonstrate the presence of an inverted U-shaped curve amid GDP and environmental pollutants.
For multi-country study, Tsai (Citation1994) for a panel of 62 economies, Apergis and Payne (Citation2009) for a panel of 6 Central American economies, Pao and Tsai (Citation2010) for a panel of 19 countries and Omri (Citation2013) for a panel of 12 MENA economies show in their empirical outcomes an inverted U-shaped curve with GDP and environmental degradation. Besides, the econometric method utilized in all cited studies is based on Granger causality tests.
In addition, the liaison among GDP and pollution is not concluded by Richmond and Kaufmann (Citation2006) for the data of 36 countries, by Halicioglu (Citation2009) and Ozturk and Acaravci (Citation2010) for a data-set relative to Turkey, by Jaunky (Citation2010) for a panel of 36 high-income economies, and by Menyah and Wolde-Rufael (Citation2010) for South Africa.
The significance of CO2 emissions in the environmental degradation and their implication in all economic and financial sectors motivated some researchers to incorporate some potential indicators to examine empirically the EKC hypothesis. Then, CO2 emissions are associated with trade openness as developed by Halicioglu (Citation2009), Nasir and Rehman (Citation2011), Shahbaz, Hye, Tiwari, and Leitão (Citation2013), Omri, Nguyen, and Rault (Citation2014), and Omri, Daly, Rault, and Chaibi (Citation2015), to urbanization rate as edited by Zhang and Cheng (Citation2009), Hossain (Citation2011), Sharma (Citation2011), Omri et al. (Citation2014, Citation2015), to financial sector development as studied by Tamazian, Piñeiro, and Vadlamannati (Citation2009), Tamazian and Bhaskara Rao (Citation2010), Yuxiang and Chen (Citation2010), Ozturk and Acaravci (Citation2013), Omri et al. (Citation2014, Citation2015).
To observe the causal link among pollution and trade liberalization, Halicioglu (Citation2009) studies how trade openness can influence the relation among GDP, environmental degradation and energy consumption in Turkey. His empirical results illustrate that trade liberalization is one of the most significant determinants of GDP, while income can amplify the threshold of pollution. For a panel of Chinese provinces, Chen (Citation2009) concludes that the industrial sector growth is accompanied with an increase of the environmental pollutants due to energy consumption.
Also, by employing augmented Dickey–Fuller unit root test and cointegration test, Nasir and Rehman (Citation2011) examine empirically the EKC hypothesis in Pakistan and conclude a positive impact of trade openness on environmental degradation. However, Shahbaz, Lean, and Shabbir (Citation2012) prove that the increase in trade openness can reduce CO2 emissions. Furthermore, Tiwari, Shahbaz, and Hye (Citation2013) demonstrate that an augment in trade openness can increase environmental pollutants in India.
For the link between the environmental degradation and the economic and financial indicators, Tamazian et al. (Citation2009) examine the influence of supplementary potential determinants on environmental pollutants such as economic, financial, and institutional indicators. Tamazian et al. (Citation2009) investigate the influence of the financial sector development on environmental degradation in Brazil, Russia, India, China, the United States, and Japan. Also, Tamazian and Bhaskara Rao (Citation2010) examine the effect of institutions indicators on environmental pollutants. Their findings indicate that GDP, trade liberalization, financial sector development, and institutions have a fundamental role in controlling gas emissions, while supporting the existence of the EKC hypothesis.
Yuxiang and Chen (Citation2010) conclude that the financial sector in China facilitate the firms to employ advanced technology which reduce CO2 emissions and increase GDP.
Moreover, in the case of the United States, Soytas, Sari, and Ewing (Citation2007) study the dynamic causal connection between environmental pollutants, national income, and energy consumption. Their findings prove that income and energy consumption contribute to pollution. A similar previous study conducted by Ang (Citation2007, Citation2008) in France and Malaysia concludes that GDP per capita affects energy consumption and environmental pollutants in both countries. Also, a unidirectional causality is concluded running from GDP to energy consumption.
For data-set of Tunisia, Chebbi (Citation2010) examines the causal linkage between energy consumption, national income, and environmental pollutants. Chebbi (Citation2010) finds that the volatility of energy consumption affects GDP and CO2 emissions.
By utilizing Indian data, Ghosh (Citation2009) studies the causal liaison among GDP and environmental pollutants by incorporating the investment and employment factors as additional indicators of carbon emissions, even though his results show the nonexistence of causal nexus among income and pollution.
Additionally, Chang (Citation2010) utilizes a multivariate Granger causality test for the case of China to study empirically the causal connection between GDP, energy consumption, and pollution. His results show that GDP affects energy consumption that leads to pollution.
Jamel and Derbali (Citation2016) investigate empirically the impact of energy consumption and economic growth on the environmental degradation as measured by CO2 emissions. They utilize the cointegration test, the fully modified OLS (FMOLS), and the panel causality to study the causality between environmental pollution and economic aggregates from a panel data of eight Asian countries during the period of study from 1991 to 2013. They find that the cointegration tests provide long-run relationship between environmental degradation and energy consumption and economic growth along with financial development, trade openness, capital stocks, and urbanization as control variables. In addition, FMOLS results provide that economic growth and energy consumption have a positive and significant impact on environmental degradation. Besides, panel causality through VECM confirms that bidirectional causal connection is found between energy consumption and economic growth and environmental degradation.
Charfeddine and Ben Khediri (Citation2016) extend the recent study of Shahbaz, Khraief, Uddin, and Ozturk (Citation2014) by implementing recent unit root tests with multiple structural breaks and regime-switching cointegration techniques considering for one and two unknown regime shifts to examine the relationship among CO2, electricity consumption, economic growth, financial development, trade openness, and urbanization for the UAE during the period of study from 1975 to 2011. Their empirical results confirm the presence of EKC. In addition, we show an inverted U-shaped relationship among financial development and CO2 emissions. They also conclude that electricity consumption, urbanization, and trade openness contribute to improve environmental quality.
Kahia et al. (Citation2016) explore the economic growth–energy consumption nexus for two samples of MENA Net Oil Exporting Countries (NOECs) over the period of study from 1980 to 2012. Using panel cointegration approach, they find strong evidence for the presence of a long-run linkage between real GDP, renewable and non-renewable energy consumption, real gross-fixed capital formation, and the labor force. In addition, the empirical findings from the estimation of the panel error correction model provide an evidence of a unidirectional causality running from economic growth to renewable energy consumption in the short run. In the long run, the empirical results confirm evidence of bidirectional causality for the entire group of MENA NOECs. For the five selected MENA NOECs sample, Kahia et al. (Citation2016) find strong evidence for bidirectional causality among renewable energy and economic growth. Moreover, their findings confirm bidirectional causality among renewable and non-renewable energy consumption with negative and significant coefficient in the short run indicating substitutability between these two types of energy.
Magazzino (Citation2016) examines the relationship between real GDP, CO2 emissions, and energy use in the six Gulf Cooperation Council (GCC) countries. Using annual data for the years 1960–2013, stationarity, structural breaks, and cointegration tests have been conducted. The empirical results strongly support the existence of unit roots. Cointegration tests reveal the presence of a clear long-run linkage only for Oman. Granger causality analysis shows that for three GCC countries (Kuwait, Oman, and Qatar) the predominance of the “growth hypothesis” emerges, since energy use drives the real GDP. In addition, only for Saudi Arabia a clear long-run relation has not been discovered. Finally, the empirical findings of the variance decompositions and impulse response functions broadly confirm his previous empirical findings. His empirical results significantly reject the assumption that energy is neutral for growth. Notwithstanding, since the causality findings are different for the 6 GCC countries, unified energy policies would not be the good recipe for the whole area.
Kahia et al. (Citation2017) study the energy use–economic growth nexus by disaggregating energy use into two types of energy, renewable and non-renewable energy use. Their sample is composed of 11 MENA Net Oil Importing Countries (NOICs) over the period of study from 1980 to 2012. A multivariate panel framework was utilized to estimate the long-run link and the panel Granger causality tests was used to investigate the causality direction between variables. Their empirical findings provide evidence for long-term equilibrium linkage among real GDP, renewable and non-renewable energy use, real gross-fixed capital formation, and labor force. The empirical results show evidence also for positive and statistically significant elasticities. Besides, the results from panel error–correction model provide the presence of bidirectional causality among renewable energy use and economic growth, and between non-renewable energy use and economic growth, results that support the feedback hypothesis. In addition, their empirical results confirm evidence for two-way (bidirectional) causal relationship in both the short and long run between renewable and non-renewable energy use which proves the substitutability and interdependence between these two types of energy sources.
In Table , we present a review of existing empirical studies which examine the causal relation among GDP and environmental pollutants.
Table 1. Summary of previous empirical studies
Based on the studies cited above, the empirical evidence of our paper is developed to examine the causal nexus between GDP, the financial sector development, trade openness, and pollution. Also, we utilize some indicators, such as Foreign Direct Investment, energy consumption, inflation, urbanization, and capital stocks, which prove highly significant in studying this causal connection. The empirical investigation is elaborated for an annually panel data of 40 European economies during the period of study from 1985 to 2014. The econometric method draws on/is founded on the utilization of the Cobb–Douglas production function which is estimated by the OLS technique.
3. Econometric methodology
To empirically examine the four-way causal link among GDP, CO2 emissions, the financial sector development, and trade openness in the European region, we utilize the Cobb–Douglas production function.
The Cobb–Douglas production function is widely used in economics to present the relationship between output and inputs (Kahia et al., Citation2016, Citation2017). The general production function is parameterized as follows:(1)
(1)
where Y is the total production; A is the total factor productivity; K is the capital input; L is the labor input; and α and β are the output elasticities of capital and labor, respectively. These values are constants determined by available technology.
Based on this function, we can explain the GDP by economic indicators as capital and labor force. Besides, the economic growth depends on the financial sector development, trade liberalization, foreign direct investment, environmental pollutants, urbanization, energy consumption, and capital stocks. In our study, we empirically estimate four equations, as follows:(2)
(2)
(3)
(3)
(4)
(4)
(5)
(5)
where ln GDP measures the gross domestic product per capita for each country i, ln FD denotes the financial sector development, ln T measures the trade liberalization, ln CO2 measures the CO2 emissions per capita, ln EC denotes the energy consumptions, ln FDI measures the foreign direct investment, ln INF measures the inflation rate, ln K measures the capital stock, and ln U measures the urbanization rate. α0 indicates the constant. ɛit measures the residual term. αji measures the estimated coefficients of all explicative variables (where, j = 1, …, 8). The subscript i = 1, …, 40 measures the country. The subscript t = 1, …, 30 is the time period. In Table , we define all variables utilized in our paper.
Table 2. Variables definition and source
In Equation (Equation2(2)
(2) ), we edit the influence of financial sector development, trade liberalization, environmental pollutants, energy uses, foreign direct investment, inflation rate, capital stock, and urbanization rate on GDP in the European economies (Ang, Citation2008; Anwar & Sun, Citation2011; Menyah & Wolde-Rufael, Citation2010; Omri et al., (Citation2014, Citation2015).
In the third equation, we study the impact of GDP, trade liberalization, CO2 emissions, energy consumptions, foreign direct investment, inflation rate, capital stock, and urbanization rate on financial sector development (Ahlin & Pang, Citation2008; Omri et al., Citation2014, Citation2015; Ozturk & Acaravci, Citation2013).
In Equation (4), we examine the impact of trade liberalization on the financial sector development, GDP, CO2 emissions, energy consumptions, foreign direct investment, inflation, capital stock, and urbanization (Belloumi, Citation2014; Omri et al., Citation2014; Ozturk & Acaravci, Citation2013).
Finally, and with reference to Lotfalipour, Falahi, and Ashena (Citation2010), Hossain (Citation2011), Sharma (Citation2011), Saboori et al. (Citation2012), Lee (Citation2013), Omri et al. (Citation2014, Citation2015), we utilize Equation (5) to examine the consequence of trade liberalization, financial sector development, GDP, energy consumptions, foreign direct investment, inflation, capital stock, and urbanization on pollution.
To estimate the above equations, we choose the OLS as a preferred technique for an annually panel data-set in the European region.
4. Empirical results
Following the standard panel data analysis procedure, we start to examine the data description of all variables used in our paper. Then, we study the stationarity of variables by employing a battery of panel unit root tests. Also, we employ OLS to investigate the causal link connecting four economic, environmental, and financial aggregates (economic growth (GDP), financial sector development, trade openness (liberalization), and CO2 emissions) in the European economies during the period of study through 1985–2014. Finally, we test the Granger causality.
4.1. Data description
This study aims at empirically examining the causal link connecting four economic, environmental, and financial aggregates (economic growth (GDP), financial sector development, trade openness (liberalization), and CO2 emissions) in the European economies during the period of study through 1985–2014. We utilize an annually panel data for a sample of 40 European countries (Albania, Armenia, Iceland, Ireland, Italy, Latvia, Lithuania, Austria, Belarus, Belgium, Bosnia and Herzegovina, Bulgaria, Moldova, the Netherlands, Norway, Croatia, Cyprus, the Czech Republic, Denmark, Estonia, Finland, France, Georgia, Germany, Greece, Hungary, Luxembourg, the Republic of Macedonia, Poland, Portugal, Romania, Russia, Slovakia, Slovenia, Spain, Sweden, Switzerland, Turkey, Ukraine, and the United Kingdom). Thus, we present in Table the listing of all European countries utilized in our paper.
Table 3. List of European countries used in this paper
Table summarizes the descriptive statistics of all variables utilized in our paper. From the empirical results in this table, we can observe that on average, the highest levels of CO2 is equal to 27.42196, EC is equal to 18774.97, FDI is equal to 430.6407, CO2 is equal to 86127.24, INF is equal to 4734.914, K is equal to 372.3756, T is equal to 371.4397, U is equal to 5.657103, and FD is equal to 311.0630. Then, we can remark that the highest level of risk (standard deviation) is for GDP (18263.42), followed by EC (2180.304) and INF (532.3235), respectively.
Table 4. Descriptive statistics
Moreover, Table conducts the estimation coefficients of the Pearson correlation matrix between all indicators used in this paper. The empirical results shown in this table find that all estimating coefficients are inferior to the tolerance limit of Pearson (0.7), which doesn’t cause problems in estimating the four equations.
Table 5. Matrix of Pearson correlation
4.2. Panel unit root test
We apply the unit root test for panel data in Table . Then, we utilize two econometric tests as Levin–Lin–Chu (LLC) and Im–Pesaran–Shin (IPS). The null hypothesis (H0) relative to these tests supposes that all variables are non-stationary and the alternative hypothesis (H1) assumes all variables are stationary.
Table 6. Unit root tests
To accept or refuse the null hypothesis, we can refer to the p-value of unit root test which is compared to 10%. If p-value is inferior to 10%, then we refuse the null hypothesis and if it is superior to 10%, while we admit the null hypothesis. In Table , we summarize the results of the unit root tests of all indicators. Based on this table, we can assume that all indicators are statistically stationary in the level and in the first difference based on the two tests of LLC and IPS. Consequently, we can reveal that all indicators are non-integrated. Accordingly, we can find that all indicators utilized in this paper are stationary.
4.3. Results discussion
In this section, we identify the nature of estimation for all used equations, which is a regression on panel data. Then, the choice of the panel regression is determined by the presence of the double dimensions in the data employed, the first dimension is time using a period of 30 years and the second is specific by the sample utilized which is composed of 40 European countries.
We can observe that the panel structure is homogeneous. Then, we can apply the method of OLS which allows a better fit by reducing the summation of squared error terms. But, the problem that arises when estimating the model is the selection of the estimation technique, as to the estimation with fixed or random effects models. So, to resolve this problem, the Hausman test is utilized to choose either the estimation with fixed effects or the estimation with random effects.
We start our empirical analysis by the presentation of the estimation findings and coefficients of Equation (1). In this equation, we study the influence of financial sector development, trade liberalization, environment pollutants, energy consumptions, foreign direct investment, inflation rate, capital stock, and urbanization on economic growth. The estimation findings of Equation (1) are reported in Table . Moreover, the Hausman test support evidence for the fixed effects models, p-value = 0.000. Next, we employ supplementary statistic tests to validate the estimated models and to defend the significance of the estimations.
Table 7. Estimation results of OLS
We test the correlation among the explicative variables and the residual terms. This type of test is based on the value (Prob > chi2). If the p-value is inferior to 5%, then we refuse the null hypothesis for the presence of the correlation between explicative variables and residual terms. And, if it is superior to 10%, we admit the null hypothesis. In Equation (1), the probability (Prob > chi2) is inferior to 5%. Consequently, we can assume the nonexistence of problems of dependence among the explicative variables and residual terms.
To test the implication of the estimated equation, we base our analysis on the probability of Fisher. Thus, we remark that the probability (Prob > F) is inferior to 5% in Equation (1). Therefore, we can remark that the estimated model is globally significant. In consequence, we find that the coefficient of R2 is larger than 0.91, then the Equation (1) is characterized by a good linear fit.
From the estimated coefficients in Table , we can observe that pollution (ln CO2) has a positive effect on ln GDP. Then, a 5% increase in pollution augments the economic growth with 0.12%.
Additionally, the financial sector development has a positive and significant impact on ln GDP. So, the augment of the financial sector development by 1% can increase the ln GDP with 0.41%.
Also, we can stipulate that energy use has a positive consequence on the ln GDP. So, we can assume that an augment of 1% in the energy use increases the economic growth with 0.74%.
The coefficient of inflation is negative and significant in threshold of 1%. Thus, an augment of 1% in inflation can reduce the GDP by 0.11%.
The capital stocks have a positive and significant impact on ln GDP in threshold of 1%. This result shows that an increase of 1% in capital stocks augments the economic growth with a level of 0.13%.
Then, the trade liberalization influences negatively the ln GDP. This finding implies that an augment of 5% in the trade liberalization reduces the ln GDP with 0.14%.
Finally, we observe that urbanization has a positive and significant consequence on ln GDP with threshold of 5%. So, this finding implies that a supplement of 5% in the urbanization involves an augment of 0.04% in GDP. However, foreign direct investment doesn’t have an impact on GDP in the European region.
In the Equation (2), we examine the impact of the GDP, trade liberalization, CO2 emissions, energy use, FDI, inflation, capital stock, and urbanization on the financial sector development. The estimation findings of the Equation (2) are presented in Table . We choose the fixed effects model as a preferred estimation method because the p-value of the Hausman test is less than 1% (0.0000).
Table 8. Estimation results of OLS
Additionally, we test the correlation among the explicative variables and the residual terms. As the probability (Prob > chi2) is less than 5%, then there are no problems of connection among the explicative variables and residual terms.
Next, we apply the test of significance of the model based on the value of the probability of Fisher. We observe that the p-value (Prob > F) is inferior to 5%. Therefore, we can assume that the model is globally significant. Also, we mentioned that the coefficient of R2 is equal to 0.67 in Equation (2); consequently, the model has a good linear fit.
The main empirical conclusions of Table indicate that ln GDP has a positive and crucial effect on the financial sector development. Then, 1% increases in GDP raises the financial sector development with 0.42%. In addition, the energy use has a negative influence on financial sector development in the European economies. Thus, we can find that an increase of 1% in energy use reduces the financial sector development with 0.19%.
The coefficient of ln FDI is negative and significant in a threshold of 1%. This result verifies that an augment of 1% in ln FDI reduces the financial development sector by 0.08%. Inflation, in turn, has a 1% negative influence on the financial sector development. Thus, a raise of 1% in inflation rate decreases the financial sector development with (−0.09%). The capital stocks positively influence the level of financial sector development in the European region. This finding implies that a 1% raise in capital stocks augments financial development by 0.05%.
Trade liberalization has a positive consequence on the financial sector development. Then, the 1% increase in trade openness can elevate the financial sector development by 0.21%.
Finally, we observe that urbanization has a positive impact on the financial sector development. This empirical finding implies that the raise of a threshold level of 5% in urbanization involves an increase of 0.04% in the financial sector development.
However, CO2 emissions don’t have an influence on financial sector development in Europe.
In Equation (3), we estimate the influence of the financial sector development, ln GDP, pollution (ln CO2), energy use (ln EC), ln FDI, inflation, capital stocks, and urbanization on trade liberalization (ln TO). The estimation results of Equation (3) are shown in Table . From the main findings of this table, we choose the fixed effects model as a suitable estimation method because the p-value of Hausman test is less than 10% (0.0000).
Table 9. Estimation results of OLS
We find that the probability (Prob > chi2) is less than 5%. Then, there are no problems of correspondence among explicative variables and error term.
Afterward, we prove that the probability (Prob > F) is inferior to 5% in Equation (3). Therefore, the estimated model is globally significant. Consequently, the coefficient of determination R2 is equal to 0.67, and then the model (Equation 3) is characterized by a good linear fit.
The results of Table prove that the financial sector development has a positive impact on trade liberalization. Therefore, 1% increase in the financial development sector can increase trade liberalization with 0.06%.
ln GDP has a negative effect on trade liberalization. So, 5% increase in the GDP diminishes trade liberalization by 0.04%. So, the high level of the economic growth can reduce the dependence to the trade openness.
Furthermore, gas emissions have a positive impact on trade liberalization. Thus, an increase of 1% in pollution can reduce the trade liberalization by 0.08%.
In addition, energy use has a positive influence on trade liberalization in Europe. So, an augment of 1% in energy use can diminish trade liberalization by 0.23%. Besides, the coefficient of ln FDI is of a positive effect on trade liberalization. This result means that a raise of 1% in FDI has a decrease in trade liberalization with 0.12%.
Inflation has a negative effect on trade liberalization. Consequently, an augment of 10% in the inflation reduces the trade liberalization by 0.01%. The capital stocks negatively influence trade liberalization in Europe. This implies that an increase of 1% in the capital stocks can decrease the trade liberalization with 0.07%.
Finally, we illustrate that urbanization has a positive effect on trade liberalization. This indicates that the supplement of 1% in urbanization implies an augment of 0.05% in trade liberalization.
In Equation (4), we summarize the effect of trade liberalization, the financial sector development, the GDP, energy use, the FDI, inflation, the capital stock, and urbanization on the environment degradation. The estimation findings of Equation (4) are concluded in Table . We choose the random effects model as a preferred estimation method because the probability of the Hausman test is equal to 0.30 (more than 10%).
Table 10. Estimation results of OLS
We test the correlation between the explicative variables and the residual terms. In Equation (4), the p-value (Prob > chi2) is less than 5%. Thus, there are no problems of dependence between the explicative variables and error terms.
The probability of Fisher is used to examine the significance of the model. We notice that the p-value (Prob > F) is less than 5% in Equation (4). Consequently, the model is globally significant. Thus, we observe that R2 is bigger than 0.68, therefore, the estimated equation has a good linear fit.
The main results of Table prove that trade liberalization has a positive consequence on the environmental pollutants with a significant threshold level of 5%. So, an increase of 5% in trade liberalization augments the environmental pollutants (CO2 emissions) with 0.08%.
ln GDP has a positive effect on environmental pollutants. Then, a raise of 1% in the GDP diminishes the environmental pollutants within 0.05%.
Moreover, energy use (ln EC) has an effect (positive) on the gas emissions (ln CO2) in the European region. Thus, an augment of 1% in energy use (consumption) decreases the environmental pollutants by 0.74%.
In addition, the coefficient of ln FDI is negative. This result implies that a raise of 5% in FDI can result in a 0.02% decline in CO2 emissions.
The capital stocks have a positive consequence on the CO2 emissions. Thus, an increase of 1% in capital stocks can decrease gas emissions by 0.02%.
Finally, we notice that urbanization has a negative impact on CO2 emissions with a threshold level of 1%. This result denotes that the increase of 1% in urbanization implies a decrease of 0.05% in trade openness.
However, the financial sector development and consumer price index (inflation rate) does not affect CO2 in Europe.
4.4. Causality test
In order to study the causal link between the economic variables of the model, we used a causality test initiated by Granger in 1969, which over time has become a reflective framework as interesting as that relating to the identification of econometric links.
In general, from this test, we can show whether it is a close link to environmental degradation and energy consumption, environmental degradation and economic growth, environmental degradation and financial development, CO2 emissions and trade openness, environmental degradation and capital stock, and environmental degradation and urbanization rate.
The results reported in Table indicates short run dynamics that there exists bidirectional causal relationship between environmental degradation and energy consumption, environmental degradation and economic growth, environmental degradation and financial development, CO2 emissions and trade openness, environmental degradation and capital stock, and environmental degradation and urbanization rate. Additionally, the error–correction term results show adjustment speed and it is significant at the level of 1% that also confirms long-run relationship between GDP, CO2 emissions, the financial sector development, and trade liberalization.
Table 11. Panel causality
5. Conclusion
In this study, we have empirically investigated the causal connection among GDP, CO2 emissions, the financial sector development, and trade liberalization. For that goal, we have utilized the OLS as an econometric methodology for yearly panel data composed of 40 European economies through the period from 1985 to 2014. The main objective of this manuscript is to empirically inspect the four-way connection among GDP, financial sector development, trade liberalization, and CO2 emissions by employing four models to examine the consequence of (1) CO2, financial sector development, trade liberalization, and other explicative variables on GDP; (2) GDP, CO2, trade liberalization (openness), and other control variables on the financial sector development; (3) financial sector development, GDP, CO2, and other explicative variables on trade openness; and (4) trade liberalization, the financial sector development, GDP, and other explicative variables on environmental pollutants.
The main empirical conclusions of our manuscript prove an evidence of bidirectional rapport among GDP and gas emissions, GDP and financial sector development, GDP and trade openness, the financial sector development and trade liberalization, and trade openness and carbon emissions. But, we indicate a nonexistence of the significant link among the financial sector development and gas emissions in Europe. Figure recapitulates the existing link between the four fundamental economic indicators in Europe.
Figure 1. The causal relationship between CO2 emissions, GDP, the financial sector development, and trade openness.
The main empirical findings of our paper find that GDP and the environmental degradations are positively and extremely associated. The GDP per capita predicts a positive linkage with gas (CO2) emissions. Furthermore, GDP per capita promotes the financial sector development. However, GDP can prevent trade openness. The increase in financial development can increase trade openness. We, also, find a positive connection between CO2 and trade liberalization. Finally, we can watch the nonexistence of causal nexus between financial development and CO2 emissions.
Our manuscript is on conformity with the previous literature on the relationship between financial development and trade openness, financial development and GDP, GDP and trade openness, GDP and CO2, and trade openness and CO2. However, we find a different result for the nexus between financial development and CO2.
Finally, panel causality verifies that bidirectional causal connection is found between economic growth, environmental degradation (CO2), financial development, and trade openness.
6. Policy implications
In our case, the economy is called energy using policies may be implemented with adverse influences on real economic growth. Therefore, if energy consumption affects economic growth, then energy preservation policies aiming at protecting the environment are expected to deteriorate the current phase of economic growth. Also, energy production and preservation policies, financial development, trade openness, and economic growth would be the fine method for the whole area. Even though there may be political will to construct the common objects and purposes, different strategy, and plan design for subgroups of member states ought to probably be considered.
7. Suggestions for future research
For the possible future works, we can decompose our sample into developing and emerging countries. Also, we can use the analysis by the cross-section methodologies.
Acknowledgment
The authors would like to thank the editor and anonymous reviewers for their supportive comments and suggestions.
Additional information
Funding
Notes on contributors
Lamia Jamel
Lamia Jamel is a PhD in Economics at the Faculty of Economic Sciences and Management of Sousse in the University of Sousse, Tunisia. Her research interests include economic analysis, financial economics, quantitative finance, financial development, and energy commodities. She is one of the Editorial Board members in the in Cogent Economics and Finance. She has published articles, among others, in Cogent Economic & Finance, Journal of the Knowledge Economy, International Journal of Critical Accounting, and African Journal of Accounting, Auditing and Finance.
Samir Maktouf
Samir Maktouf is a professor in Economics at the Faculty of Economic Sciences and Management of El Manar in the University of El Manar, Tunisia. His research interests include economic analysis, financial economics, quantitative finance, financial development, and financial liberalization. He has published articles, among others, in International Review of Applied Economics, International Journal of Emerging Markets, International Business Research, and International Journal of Development Issues.
References
- Ahlin, C., & Pang, J. (2008). Are financial development and corruption control substitutes in promoting growth? Journal of Development Economics, 86, 414–433.10.1016/j.jdeveco.2007.07.002
- Ang, J. B. (2007). CO2 emissions, energy consumption, and output in France. Energy Policy, 35, 4772–4778.10.1016/j.enpol.2007.03.032
- Ang, J. B. (2008). Economic development, pollutant emissions and energy consumption in Malaysia. Journal of Policy Modeling, 30, 271–278.10.1016/j.jpolmod.2007.04.010
- Anwar, S., & Sun, S. (2011). Financial development, foreign investment and economic growth in Malaysia. Journal of Asian Economics, 22, 335–342.10.1016/j.asieco.2011.04.001
- Apergis, N., & Payne, J. E. (2009). CO2 emissions, energy usage, and output in Central America. Energy Policy, 37, 3282–3286.10.1016/j.enpol.2009.03.048
- Azomahou, T., Laisney, F., & Van, P. N. (2006). Economic development and CO2 emissions: A nonparametric panel approach. Journal of Public Economics, 90, 1347–1363.10.1016/j.jpubeco.2005.09.005
- Baranzini, A., Weber, S., Bareit, M., & Mathys, N. A. (2013). The causal relationship between energy use and economic growth in Switzerland. Energy Economics, 36, 446–470.
- Belloumi, M. (2014). The relationship between trade, FDI and economic growth in Tunisia: An application of the autoregressive distributed lag model. Economic Systems, 38, 269–287.10.1016/j.ecosys.2013.09.002
- Chang, C. C. (2010). A multivariate causality test of carbon dioxide emissions, energy consumption and economic growth in China. Applied Energy, 87, 3533–3537.10.1016/j.apenergy.2010.05.004
- Charfeddine, L., & Ben Khediri, K. (2016). Financial development and environmental quality in UAE: Cointegration with structural breaks. Renewable and Sustainable Energy Reviews, 55, 1322–1335.10.1016/j.rser.2015.07.059
- Chebbi, H. E. (2010). Long and short-run linkages between economic growth, energy consumption and CO2 emissions in Tunisia. Middle East Development Journal, 2, 139–158.10.1142/S1793812010000186
- Chen, S. (2009). Energy consumption, CO2 emission and sustainable development in Chinese industry. Economic Research Journal, 4(1), 1–5.
- Chen, S. T., Kuo, H. I., & Chen, C. C. (2007). The relationship between GDP and electricity consumption in 10 Asian countries. Energy Policy, 35, 2611–2621.10.1016/j.enpol.2006.10.001
- Ekins, P. (1997). The Kuznets curve for the environment and economic growth: Examining the evidence. Environment and Planning A, 29, 805–830.10.1068/a290805
- Farhani, S., Shahbaz, M., Sbia, R., & Chaibi, A. (2014). What does MENA region initially need: Grow output or mitigate CO2 emissions? Economic Modelling, 38, 270–281.10.1016/j.econmod.2014.01.001
- Fodha, M., & Zaghdoud, O. (2010). Economic growth and environmental degradation in Tunisia: An empirical analysis of the environmental Kuznets curve. Energy Policy, 38, 1150–1156.10.1016/j.enpol.2009.11.002
- Friedl, B., & Getzner, M. (2003). Determinants of CO2 emissions in a small open economy. Ecological Economics, 45, 133–148.10.1016/S0921-8009(03)00008-9
- Ghosh, S. (2009). Electricity supply, employment and real GDP in India: Evidence from cointegration and Granger-causality tests. Energy Policy, 37, 2926–2929.
- Ghosh, S. (2010). Examining carbon emissions-economic growth nexus for India: A multivariate cointegration approach. Energy Policy, 38, 2613–3130.
- Grossman, G., & Krueger, A. (1991) Environmental impacts of a North American free trade agreement (National Bureau of Economics Research Working Paper No. 3194). Cambridge: NBER.10.3386/w3914
- Halicioglu, F. (2009). An econometric study of CO2 emissions, energy consumption, income and foreign trade in Turkey. Energy Policy, 37, 1156–1164.10.1016/j.enpol.2008.11.012
- Heil, M. T., & Selden, T. M. (1999). Panel stationarity with structural breaks: Carbon emissions and GDP. Applied Economics Letters, 6, 223–225.10.1080/135048599353384
- Hossain, M. S. (2011). Panel estimation for CO2 emissions, energy consumption, economic growth, trade openness and urbanization of newly industrialized countries. Energy Policy, 39, 6991–6999.10.1016/j.enpol.2011.07.042
- Jalil, A., & Mahmud, S. F. (2009). Environment Kuznets curve for CO2 emissions: A cointegration analysis for China. Energy Policy, 37, 5167–5172.10.1016/j.enpol.2009.07.044
- Jamel, L., & Derbali, A. (2016). Do energy consumption and economic growth lead to environmental degradation? Evidence from Asian economies. Cogent Economics & Finance, 4, 1170653. doi:10.1080/23322039.2016.1170653
- Jaunky, V. C. (2010). The CO2 emissions–income nexus: Evidence from rich countries. Energy Policy, 39, 1228–1240.
- Kahia, M., Ben Aïssa, M. S., & Charfeddine, L. (2016). Impact of renewable and non-renewable energy consumption on economic growth: New evidence from the MENA Net Oil Exporting Countries (NOECs). Energy, 116, 102–115.10.1016/j.energy.2016.07.126
- Kahia, M., Ben Aïssa, M. S., & Lanouar, C. (2017). Renewable and non-renewable energy use - economic growth nexus: The case of MENA Net Oil Importing Countries. Renewable and Sustainable Energy Reviews, 71, 127–140.10.1016/j.rser.2017.01.010
- Lean, H. H., & Smyth, R. (2010). CO2 emissions, electricity consumption and output in ASEAN. Applied Energy, 87, 1858–1864.10.1016/j.apenergy.2010.02.003
- Lee, J. W. (2013). The contribution of foreign direct investment to clean energy use, carbon emissions and economic growth. Energy Policy, 55, 483–489.
- Lotfalipour, M. R., Falahi, M. A., & Ashena, M. (2010). Economic growth, CO2 emissions, and fossil fuels consumption in Iran. Energy, 35, 5115–5120.10.1016/j.energy.2010.08.004
- Magazzino, C. (2016). The relationship between real GDP, CO2 emissions, and energy use in the GCC countries: A time series approach. Cogent Economics & Finance, 4, 1152729. doi:10.1080/23322039.2016.1152729
- Managi, S., & Jena, P. R. (2008). Environmental productivity and Kuznets curve in India. Ecological Economics, 65, 432–440.10.1016/j.ecolecon.2007.07.011
- Menyah, K., & Wolde-Rufael, Y. (2010). CO2 emissions, nuclear energy, renewable energy and economic growth in the US. Energy Policy, 38, 2911–2915.10.1016/j.enpol.2010.01.024
- Nasir, M., & Rehman, F. (2011). Environmental Kuznets curve for carbon emissions in Pakistan: An empirical investigation. Energy Policy, 39, 1857–1864.10.1016/j.enpol.2011.01.025
- Omri, A. (2013). CO2 emissions, energy consumption and economic growth nexus in MENA countries: Evidence from simultaneous equations models. Energy Economics, 40, 657–664.10.1016/j.eneco.2013.09.003
- Omri, A. (2014). An international literature survey on energy-economic growth nexus: Evidence from country-specific studies. Renewable and Sustainable Energy Reviews, 38, 951–959.10.1016/j.rser.2014.07.084
- Omri, A., Daly, S., Rault, C., & Chaibi, A. (2015). Financial development, environmental quality, trade and economic growth: What causes what in MENA countries. Energy Economics, 48, 242–252.10.1016/j.eneco.2015.01.008
- Omri, A., Nguyen, D. K., & Rault, C. (2014). Causal interactions between CO2 emissions, foreign direct investment, and economic growth: Evidence from dynamic simultaneous equation models. Economic Modelling, 42, 382–389.10.1016/j.econmod.2014.07.026
- Ozturk, I., & Acaravci, A. (2010). CO2 emissions, energy consumption and economic growth in Turkey. Renewable and Sustainable Energy Reviews, 14, 3220–3225.10.1016/j.rser.2010.07.005
- Ozturk, I., & Acaravci, A. (2013). The long-run and causal analysis of energy, growth, openness and financial development on carbon emissions in Turkey. Energy Economics, 36, 262–267.10.1016/j.eneco.2012.08.025
- Pao, H., & Tsai, C. (2010). CO2 emissions, energy consumption and economic growth in BRIC countries. Energy Policy, 38, 850–860.
- Richmond, A. K., & Kaufmann, R. K. (2006). Is there a turning point in the relationship between income and energy use and/or carbon emissions? Ecological Economics, 56, 176–189.10.1016/j.ecolecon.2005.01.011
- Saboori, B., Sulaiman, J., & Mohd, S. (2012). Economic growth and CO2 emissions in Malaysia: A cointegration analysis of the environmental Kuznets curve. Energy Policy, 51, 184–191.10.1016/j.enpol.2012.08.065
- Selden, T. M., & Song, D. (1994). Environmental quality and development: Is there a Kuznets curve for air pollution emissions? Journal of Environmental Economics and Management, 27, 147–162.10.1006/jeem.1994.1031
- Shahbaz, M., Hye, Q. M. A., Tiwari, A. K., & Leitão, N. C. (2013). Economic growth, energy consumption, financial development, international trade and CO2 emissions in Indonesia. Renewable and Sustainable Energy Reviews, 25, 109–121.10.1016/j.rser.2013.04.009
- Shahbaz, M., Khraief, N., Uddin, G. S., & Ozturk, I. (2014). Environmental Kuznets curve in an open economy: A bounds testing and causality analysis for Tunisia. Renewable Sustainable Energy Review, 34, 325–336.
- Shahbaz, M., Lean, H. H., & Shabbir, M. S. (2012). Environmental Kuznets curve hypothesis in Pakistan: Cointegration and Granger causality. Renewable and Sustainable Energy Reviews, 16, 2947–2953.10.1016/j.rser.2012.02.015
- Sharma, S. S. (2011). Determinants of carbon dioxide emissions: Empirical evidence from 69 countries. Applied Energy, 88, 376–382.10.1016/j.apenergy.2010.07.022
- Soytas, U., & Sari, R. (2009). Energy consumption, economic growth, and carbon emissions: Challenges faced by an EU candidate member. Ecological Economics, 68, 2706–2712.
- Soytas, U., Sari, R., & Ewing, B. T. (2007). Energy consumption, income, and carbon emissions in the United States. Ecological Economics, 62, 482–489.10.1016/j.ecolecon.2006.07.009
- Stern, D. I. (1993). Energy and economic growth in the USA. Energy Economics, 15, 137–150.10.1016/0140-9883(93)90033-N
- Stern, D. I., Common, M. S., & Barbier, E. B. (1996). Economic growth and environmental degradation: The environmental Kuznets curve and sustainable development. World Development, 24, 1151–1160.10.1016/0305-750X(96)00032-0
- Tamazian, A., & Bhaskara Rao, B. (2010). Do economic, financial and institutional developments matter for environmental degradation? Evidence from transitional economies. Energy Economics, 32, 137–145.10.1016/j.eneco.2009.04.004
- Tamazian, A., Piñeiro, J., & Vadlamannati, K. C. (2009). Does higher economic and financial development lead to environmental degradation: Evidence from BRIC countries Energy Policy, 37, 246–253.10.1016/j.enpol.2008.08.025
- Tiwari, A. K., Shahbaz, M., & Hye, Q. M. A. (2013). The environmental Kuznets curve and the role of coal consumption in India: Co-integration and causality analysis in an open economy. Renewable and Sustainable Energy Reviews, 18, 519–527.10.1016/j.rser.2012.10.031
- Toda, H. Y., & Yamamoto, T. (1995). Statistical inference in vector autoregressions with possibly integrated processes. Journal of Econometrics, 66, 225–250.10.1016/0304-4076(94)01616-8
- Tsai, P. (1994). Determinants of foreign direct investment and its impact on economic growth. Journal of Economic Development, 19, 137–163.
- Wolde-Rufael, Y. (2005). Energy demand and economic growth: The African experience. Journal of Policy Modeling, 27, 891–903.10.1016/j.jpolmod.2005.06.003
- Yuan, J., Zhao, C., Yu, S., & Hu, Z. (2007). Electricity consumption and economic growth in China: Cointegration and co-feature analysis. Energy Economics, 29, 1179–1191.10.1016/j.eneco.2006.09.005
- Yuxiang, K., & Chen, Z. (2010). Financial development and environmental performance: Evidence from China. Environment and Development Economics, 16(1), 1–19.
- Zhang, X. P., & Cheng, X. M. (2009). Energy consumption, carbon emissions, and economic growth in China. Ecological Economics, 68, 2706–2712.10.1016/j.ecolecon.2009.05.011