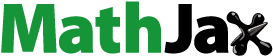
Abstract
In three different ways of lead–lag causal relationship, covariance/correlation and coherence, we apply the wavelets analysis via the Continuous Morlet Wavelet Transform to delineate the significant frequency–time domain lead–lag relationships for the West African Monetary Zone member countries for real US dollar exchange rates and their absolute log returns from January 2001 to April 2015. The results indicate that lead–lag associations at different periodicities vary across the countries. No one country comes off as leading conveniently for both real and absolute returns of the exchange rates. Our results corroborate other evidences of non-convergence of exchange rates in the monetary zone, which hinders the eventual implementation of the single currency in the ECOWAS region.
Public Interest Statement
This research is important for governments and policy-makers in the West African Monetary Zone (WAMZ) and ECOWAS alike; there is the need to adopt the single currency for the sub-region to reap the perceived benefits of economic and trade integration. Since member countries have failed to achieve a sort of purchasing power parity hitherto, by the wavelets methodology, we are able to show at what point in time of the year the dollar exchange rates across the countries differed, in which months, in what direction, which country caused the change, etc. using monthly exchange rate data for four WAMZ (The Gambia, Ghana, Liberia and Nigeria) member countries from January 2001 to April 2015. This will help policy-makers make informed decisions in working towards the single currency. We find that different countries have both leading and lagging roles in the dynamics of the exchange rates.
1. Introduction
Given the high dependence of the African continent on foreign exchange, a full-blown monetary integration is imperative but has been dragged in the glare of exchange rate risk. One of the main prerequisites for the West African Monetary Zone (WAMZ) to take off is to achieve convergence in macroeconomic variables amongst member countries.Footnote1 According to Seck (Citation2014), the ability of the WAMZ countries to meet the convergence measures seems elusive. Considering the failure of the WAMZ to achieve convergence in exchange rates and other macroeconomic fundamentals since its inception, makes the study of comovements a very relevant undertaking for policy-making in the sub-region (see Adam, Agyapong, & Gyamfi, Citation2012; Agyapong & Adam, Citation2012; Seck, Citation2014; Tweneboah, Agyapong, & Frimpong, Citation2016).
The literature is replete with study of co-movements via correlation analysis. For asset returns, Corsetti, Pericoli, and Sbracia (Citation2005) assert if correlation across assets is abnormally high, diversification of international portfolios may fail to deliver just when its benefits are most needed. By the same token, excessive co-movements of exchange rates may spread country-specific shocks to other economies, even when these countries possess better fundamentals. The recent developments in the financial markets of advanced economies have raised both theoretical and practical concerns regarding regionalisation and the adoption of common currency. The caption of the theoretical and policy debate on this issue is “contagion” (Corsetti et al., Citation2005).
According to Scott (Citation2016), “contagion”, was the most potentially destructive feature of the 2008 financial crisis and not “connectedness”. However, since the monetary union incorporates the adoption of a common currency, the interconnectedness of the economies as well as convergence of real exchange rates is an admirable attribute. Why should we not be wary of exchange rate instability that often besets some member countries of the WAMZ such as Ghana and Nigeria both in the past and in the present? Unfortunately, the divergence in the exchange rates of the WAMZ economies remains one of the main reasons behind the delay of the launch of the common currency “eco”, with the introduction dates moved from 2000 to 2005 to 2009 then to 2015 and now not anymore. Unfortunately, the previous studies have focused on the behaviour of real exchange rates either by using stationarity tests or convergence of prices and exchange rates in individual countries. None of the studies have considered the convergence of exchange rates as proposed by the current study.
The need, therefore, to study in detail co-movements of exchange rates in the sub-region has never been greater. In setting up monetary unions, exchange rate regime has always been a major challenge that merits detailed discussion (Dupasquier, Osakwe, & Thangavelu, Citation2006). Since liberalisation in the 1980s, most African countries have recorded high volatility in exchange rates and prices (Kargbo, Citation2000). The majority of econometric analyses of the nexus between exchange rate and inflation in the continent indicate that currency depreciation is associated with a reduction in output and increase in inflation (see Alagidede, Tweneboah, & Adam, Citation2008). The call for monetary integration has become very imperative in addressing the problems of multiplicity of currencies and exchange rate variability that often impede trade flows amongst African countries (Yehoue, Citation2005). A deeper understanding of the extent of co-movement in exchange rates in the WAMZ will not only inform policy regarding the direction of the zone but also in the West African sub-region. Policy decisions in this regard are as crucial as the implications of exchange rates on the economic well-being of the economies in Africa—not least at the backdrop of the journey towards the adoption of a single currency at the sub-regional level.
The behaviour of real exchange rates in member countries and the implications for their accession to the monetary union could not be more relevant to study as has been done for European, Asian and Middle Eastern regions (see Abdalla, Citation2012; Baig, Citation2001; Baxter & Stockman, Citation1989; Ghosh, Gulde-Wolf, & Wolf, Citation2002; Hsing, Citation2007; Johnson & Soenen, Citation2002; Lane & Milesi-Ferretti, Citation2006; Lin, Citation2012; Orlov, Citation2009; Reboredo & Rivera-Castro, Citation2013). The study is a significant contribution to the literature on the application of wavelets and policy-making in the sub-region considering the limited studies in Africa, and particularly on the WAMZ, as well as the long-run sustainability of the exchange rate requirements, and ultimately the accomplishment of the ECOWAS single currency by the year 2020.
Studies into co-movements of exchange rates have merited different methodologies. Examining the yen/dollar exchange rate to determine whether news in the New York market can predict volatility in the Tokyo market several hours later in 1988, Engle, Ito and Lin used GARCH model to argue that exchange rates react not only to macroeconomic fundamentals in the domestic market but also to spillover effects from other markets (Engle, Ito, & Lin, Citation1988). This set the table for many papers to explore the interdependence of exchange rate returns based on the GARCH framework and other variants thereof (see Pérez-Rodríguez, Citation2006; Tamakoshi & Hamori, Citation2014). Other concerns are the investigation into cause-and-effect relationships amongst different currencies through Markov switching, VAR models and Granger causality tests (for example, Antonakakis, Citation2012; Beirne & Gieck, Citation2014; Inagaki, Citation2007; Nikkinen, Pynnönen, Ranta, & Vähämaa, Citation2011) and non-linear dependence based on the copula functions (see for example, Dias & Embrechts, Citation2010; Patton, Citation2006).
Aloui and Hkiri (Citation2014) find it unfortunate that these techniques have generally yielded conflicting findings and failed to cover the frequency domain of co-movements associated with financial markets. As a result, most recent attempts intended to discern the co-movements of currencies have applied the wavelet methodology introduced by Grossman and Morlet (Citation1984). The wavelets methodology has gathered pace in recent years due to its valuable means of exploring the complex dynamics of financial time series through multi-scale decomposition (Bekiros & Marcellino, Citation2013). We apply the wavelets methodology to extract the relevant time-scale components in exchange rates in the WAMZ. The analysis is based on monthly data of real exchange rates for four (The Gambia, Ghana, Liberia and Nigeria) member countries out of six (Guinea and Sierra Leone excluded) of the WAMZ from January 2001 to April 2015. The sample period and the four countries are so chosen for the availability of consistent data. Despite that fact that Liberia only joined the WAMZ in 2010, it is included in this study because it has consistent real exchange rates from the formation of the WAMZ in 2001. In their work, Vlachos, Yu, and Castelli (Citation2005) motivate the need for more flexible structural similarity measures between time-series sequences, which are based on the extraction of important periodic features. Specifically, they present non-parametric methods for accurate periodicity detection. In this paper, we use Wavelets analysis of the exchange rate time series to better extract important features of co-movement than, for instance, correlation analysis. We introduce new periodic distance measures for time-series sequences for the purposes of aiding in the detection, monitoring and visualisation of structural periodic changes.
The wavelet-based methodology allows us to assess simultaneously the co-movement at the frequency level and over time in the exchange rate dynamics of the WAMZ; thus analysing transitory dynamic associations between two series. One of the widely used methodologies to assess co-movement is the Vector Error Correction Model (VECM). The advantage of the wavelets technique over the VECM is the inability of the latter to accommodate frequency and time-scale domain of time series (Masih & Majid, Citation2013). In this way, it is possible to capture the time and frequency varying features of co-movement within a unified framework which constitutes a refinement to previous approaches (Boako, Alagidede, & et al., Citation2016; Rua, Citation2010). As foreshadow of the results, we report that lead–lag associations at different periodicities vary across the countries. No one country comes off as leading conveniently for both real and absolute returns of real exchange rates.
The remainder of this paper is as follows: Section 2 presents a background to the wavelets methodology via the continuous Morlet wavelet transform followed by data and descriptive analysis in Section 3. Section 4 presents the results and discussions whilst we devote Section 5 to conclusions and recommendations.
2. Wavelet-based measure of co-movement
We apply the continuous wavelet transform propounded by Morlet, Arens, Fourgeau, and Glard (Citation1982), hereafter referred to as the Continuous Morlet Wavelet Transform (CMWT) which has preferable properties. The CMWT allows for good identification and isolation of periodic signals, by providing a balance between localisation of time and frequency Grinsted, Moore, and Jevrejeva (Citation2004), and also appears to provide a better trade-off between detecting oscillations and peaks or discontinuities. Principal to this paper are the estimators of wavelet variance, wavelet correlation and wavelet cross-correlation, and wavelet coherence which allows co-movement analysis in the state space.
The use of a generalised Fourier integral to convey simultaneous time and frequency information in a signal goes back at least to D. Gabor in Citation1946 (Heil & Walnut, Citation1989). In In and Kim (Citation2013), the continuous wavelet transform is defined as the integral over all time of the signal multiplied by scaled, shifted versions of the wavelet function ψ (scale, position and time):(1)
(1)
The results of the CWT are many wavelet coefficients C, which are a function of scale and position. The scale and position can take on any values compatible with the region of the time series xt. If the signal is a function of a continuous variable and a transform that is a function of two continuous variables is desired, the continuous wavelet transform can be defined by Burrus, Gopinath, and Guo (Citation1998) as:(2)
(2)
with inverse(3)
(3)
Gabor (Citation1946) defines a windowed Fourier transform operator, using a Gaussian window. Now often called the wavelet transforms, Grossman and Morlet (Citation1984) defined an affine coherent state integral operator. The Morlet wavelet, a plane wave modulated by Gaussian can be expressed in the simplest form as:(4)
(4)
where ω is a non-dimensional “time” parameter. The “angular frequency” (or rotation rate in radians per unit time) is set to 6 to generate the admissibility of the Morlet function. The period or inverse frequency measured in time units is equal to 2π/2, since one revolution equals 2π (radians). is complex, non-orthogonal, and normalised to have unit energy. Whilst the cross-wavelet power acts as correlation, we use the cross-wavelet coherence concept, like the coefficient of determination which provides proper examination of the time-varying relationship for bivariate time series.
A better definition of the wavelet coherence can be attained by considering the cross-wavelet transform and wavelet power spectrum (WPS) and phase difference (Boako et al., Citation2016). Torrence and Compo (Citation1998) define the bivariate cross-wavelet transform (XWT) of xt and yt as:(5)
(5)
where Wx and Wy are the respective wavelet transforms which decompose the Fourier co- and quadrature-spectra in the frequency–time (time-scale) domain (Roesch & Schmidbauer, Citation2014). In line with Veleda, Montagne, and Araujo (Citation2012), the Wavelet Comp implements the rectified version of (5) as:(6)
(6)
where s and τ are frequency and time, respectively. The modulus of (6) can be interpreted as cross-wavelet power (7); it lends itself, with certain limitations, to an assessment of the similarity of the two series’ wavelet power in the time–frequency (or time-scale) domain:(7)
(7)
In a geometric sense, the cross-wavelet transform (6) is the analogue of the covariance, and like the latter (7), it depends on the unit of measurement of the series and may not be ready for interpretation with regard to the degree of association of the two series; wavelet coherency may remedy this (Roesch & Schmidbauer, Citation2014).
The concept of Fourier coherency measures the cross-correlation between two time series as a function of frequency; an analogous concept in wavelet theory is the notion of wavelet coherency, which, however, requires smoothing of both the cross-wavelet spectrum and the normalising individual wavelet power spectra (without smoothing, its absolute value would be identical (Roesch & Schmidbauer, Citation2014). In the spirit of Torrence and Webster (Citation1999), the wavelet coherence of two time series x and y can be defined as:(8)
(8)
where S is a smoothing operator. Mimicking the traditional correlation coefficient, it is useful to think of the wavelet coherence as a localised correlation coefficient in the frequency–time space (Ftiti, Tiwari, Belanès, & Guesmi, Citation2015; Madaleno & Pinho, Citation2010a, b; Uddin, Tiwari, Arouri, & Teulon, Citation2013). Wavelet coherence close to one shows a higher similarity between the time series, whilst coherence near zero depicts no relationship (Boako et al., Citation2016).
According to Madaleno and Pinho (Citation2012) and Torrence and Compo (Citation1998), the phase for wavelet depicts any lead/lag linkages between two time series, and can be defined as:(9)
(9)
An absolute value of θxy less (larger) than π/2 indicates that the two series move in phase (anti-phase, respectively) referring to the instantaneous time as time origin and at the frequency under consideration, while the sign of the phase shows which series is the leading one in the relationship. In the graphical plots, the phase vectors are shown by arrows (Boako & Alagidede, Citation2017; Boako et al., Citation2016; Ranta, Citation2010; Roesch & Schmidbauer, Citation2014).
Wavelet analysis is able to derive all information about structural changes in the data through a phase difference technique (Conraria & Soares, Citation2011). Further, unlike wavelets, the correlation analysis is unable to provide information about when correlations occur and lead–lag relationships—having different data series showing similar periodicities do not necessarily connote lead–lag relationship (Pinho & Madaleno, Citation2011).
3. Data and descriptive analysis
3.1. Description of data
The analysis is based on monthly data of real exchange rates for four member countries of the WAMZ from January 2001 to April 2015 due to the availability of consistent data for these countries. This applies to Liberia given that it joined the WAMZ almost a decade after its formation. Thus, the choice of period and countries was based on availability of consistent data rather than the formation of the monetary zone. By real exchange rate, we are referring to the price of a unit US dollar in the respective country’s local currency; USD/GHS for Ghana, USD/NGN for Nigeria, USD/LRD for Liberia and USD/GMD for The Gambia. The use of common currency returns in related studies is been justified to be most appropriate in alleviating exchange rate noise (Pukthuanthong & Roll, Citation2009). The real exchange rate is defined as the nominal rate adjusted for differences in price levels in the two countries. Mathematically, the real exchange rate is equal to the respective nominal exchange rate multiplied by the domestic consumer price index divided by the foreign consumer price index. All data have been collected from the International Financial Statistics database hosted by the International Monetary Fund (IMF). We analyse the returns (based on absolute returns computed as the log difference between monthly exchange rates) of the series. The log return series was calculated as:(10)
(10)
where rt is the continuously compounded return (natural logarithm of the simple gross return of an asset).
3.2. Graphical representation
The graphical representation of the real exchange rate and absolute log returns series are exhibited in Panels , respectively, portray non-normality by visual inspection. Formal tests of normality are provided in Table together with other descriptive statistics. The up and down movements in the series suggest inherent volatilities across the board over sample period.
Table 1. Summary statistics
3.3. Descriptive/summary statistics
In Table , we present a descriptive statistics of real exchange rates across the four WAMZ countries. Liberia recorded the highest mean of 5.14 as against the least of 0.49 for Ghana, albeit positive means across the board. On the other side, Nigeria and The Gambia shared the highest and lowest unconditional volatility of 0.25 and 0.15, respectively; as measured by the standard deviation. Except for The Gambia, all the other series have positive skewness with Liberia witnessing the most severe right skewness of 0.92 followed by Ghana (0.65), Nigeria (0.27) and The Gambia (−0.19). Likewise, all the series are platykurtic except Liberia which is leptokurtic with Nigeria (−1.15) possessing the lowest peak. By the kurtosis and skewness, the series could not be more non-normal with thinner tails.
The descriptive statistics of the absolute log-returns of the exchange rates are also presented. Ghana recorded the highest mean of 0.028 as against the least of 0.003 for Nigeria, albeit positive means across the board. Similarly, Ghana and Nigeria shared the highest and lowest unconditional volatility of 0.029 and 0.004, respectively; as measured by the standard deviation. Nigeria witnessed the most severe right skewness of 4.448 followed by Liberia (3.411), The Gambia (2.263) and lastly Ghana (1.663). Likewise, all the series are leptokurtic with the Nigeria (26.961) possessing the highest peakness as against the lowest by Ghana (3.797); the rest follow in the order of the positive skewness.
Except for real exchange rate for The Gambia, the Jarque–Bera test statistic rejects normality at the 5% level of statistical significance.
3.4. Correlation analysis
As a prelude to the wavelet analysis values of Pearson product–moment correlations are presented Table for all the series to ascertain the degree of association of exchange rates in the WAMZ. The Pearson correlation coefficients indicate an inverse weak association exist between The Gambia and Liberia (−0.18) and between The Gambia and Nigeria (−0.23) on the other hand. The rest show positive associations with each other, with the highest (lowest) of which (0.90) is between Liberia and Nigeria (The Gambia and Liberia). We, thus, see significant levels of associations in the monetary zone which can be further explored for co-movements with the superior wavelet analysis.
Table 2. Correlation of real exchange rate in the WAMZ
For the returns series, an inverse weak association exists between The Gambia and Ghana (−0.01) on the one hand and Ghana and Liberia (−0.15) on the other hand. The rest show positive associations with each other—the highest (lowest) of which (0.08) is between The Gambia and Nigeria (Ghana and Nigeria). We thus see significant levels of co-movement in the monetary zone which can be further explored with the superior wavelet analysis.
4. Results and discussions
4.1. Univariate analysis of real exchange rates
The wavelet power is relative to unit-variance white noise and directly comparable to results of other time series (Roesch & Schmidbauer, Citation2014). Hence, we employ the WPS as a measure of local variance of the underlying series in the univariate case. The WPS is presented in plots with contours in time (years) and frequency (monthly) axes indicated on the horizontal and vertical axes, respectively. The frequency is expressed in powers of two, ranging from 2 months (lower or bottom of plot) to 64 months (upper or top of plot). The thick white contours indicate regions of energy denoting significance at the 5% (95% confidence) level. Following a white noise process, the WPS is estimated from Monte Carlo simulations.
To the right of the WPS is a colour bar depicting the steep power gradient of the significant contours ranging from blue (lower power) to red (higher power) distributed according to the intervals in the frequency. The n-shaped cone indicates the region of influence affected by edge effects. Periods outside the cone do not represent statistical confidence and are not considered for analysis (Panel ).
In Panel , we present WPS of the levels of real exchange rates for four countries in the WAMZ. The significant variances for The Gambia and Ghana occur at higher frequencies of 34–50-month band between 2003 through to the end of 2005 and 20–32-month band, respectively; however, most of the variance for the latter falls outside the cone of influence and hence discarded. On the other hand, we record variances at lower frequencies for Liberia and Nigeria both in 2004 in the 2–3-month band. These variances are very similar to the return series of the corresponding series in Panel . To see clearly, the periods whose average power were found to be significant over the entire time interval, we use the wavelet transform to reproduce the original exchange rates and the absolute return series in Panels and , respectively.
The WPS of the absolute exchange rate returns is presented in Panel . Across the board, few variances occur at low frequencies through the 2–4-month frequency band. We observe medium variance towards middle of 2014 for Gambia, a high variance concentration for a few months in 2003 for Ghana and towards the end of 2013 for Liberia. There is no account for Nigeria (and a low variance concentration at the end of 2015 is outside the cone of influence). Lastly, to see clearly the periods whose average power were found to be significant over the entire time interval, we use the wavelet transform to reproduce the original real exchange rate return series. The reconstructed versions of the original series are presented in Panel .
4.2. Co-movement of exchange rates in the WAMZ
In this sub-section, we employ the wavelet coherency and phase difference to examine the co-movement of real exchange rates and returns in the WAMZ. We show these plots to examine bivariate co-movements in the frequency–time domains with the wavelet coherency as a measure of local correlation amongst our variables; and phase differences to depict any lag or lead (causality) relationships between countries. In the wavelet coherence plot, we note that the area of significance at 5% confidence is where the arrows are plotted only within white contour lines (with respect to two white noise processes simulated by Monte Carlo method) with Bartlett default smoothing window type.
Arrows pointing to the right suggest that the series are in phase. To the right and up means the first series is lagging. Arrows to the right and down means the first series is leading. Arrows pointing to the left mean that the two series are out of phase. To the left and up shows the first series is leading. When the move goes to the left and down, then the first series is said to be lagging (Roesch & Schmidbauer, Citation2014). A red colour inside the white contour at the bottom (top) of the plots represents strong co-movement at low (high) frequencies, whilst a red colour in the white contours at the left-hand (right-hand) side symbolises strong co-movement at the beginning (end) of the sample period. The phase difference between two series is indicated by arrows. The order of the series is same as the order in which the names appear on top of the plots.
4.3. Co-movement of levels of exchange rates
Panel presents the cross-wavelet coherency of monthly real exchange rates. At the composite level, all six pairs of countries exhibit high significant levels of co-movements within the region of influence. Moreover, phase-difference (for lead–lag relationship) depicted by the direction of arrows differs across pairs of countries, frequencies and time period. Hence, we can reject the null hypothesis that there is no (joint) periodicity in the pairs of series via p-values obtained from simulation (a few of these provided in Appendix I) At the high frequencies of 45–50, the Gambia–Ghana pair is in-phase with Ghana leading for 2008–2009, so is the case at 26–28-month band for 2012–2013, and at low frequency of 3–4 band for late 2006 and 2013. We also observe no lead–lag relationship between 3–6 band in late 2007, and lastly in-phase in 2003 and late 2011–2013 in 12–14 and 10–12-month band, respectively, with The Gambia leading. Unlike the level series, the return pair has high co-movement in-phase at the highest frequency; but with The Gambia leading. Thus, comparatively The Gambia leads in the co-movement of exchange rates.
At the high frequency of 60, there is no lead–lag relation between The Gambia and Liberia for 2008–2011, similar to the return series of the same pair. In-phase and Liberia leading, we see co-movements in the 16–20 and 3–5-month bands in 2003 and 2008–2009, respectively. There are many isolated significant regions of periodicity in the 3–15-month band at the 5% level akin to the return pair; lead–lag strengths are shared as well.
The Gambia and Nigeria exhibit about five evident contours of co-movement; the rest are scattered and sparse. The pair is in-phase with the Gambia leading in the 20–32-month band for 2006–2010 and 18–20-month band for 2009–2011. On the other hand, the pair is out-of-phase at between 3–9 month-band for 2011–2012 with The Gambia leading; in similar a fashion as the absolute log return pair of the same series. The pair is in-phase with Ghana leading in 2004 at the 20-month frequency, from 2007 to late 2008 within the 20–30-month band, and in 2009 at the 60-month frequency although we spot no lead–lag relation for 2007–2007 within 16–20-month band. Yet, we see out-of-phase co-movement within 10–14 bands for 2003–2004 with Nigeria leading and Ghana leading in 2003 within the 5–7 bands. There are many isolated significant regions of periodicity in the 3–15-month band at the 5% level from 2004 to late 2013. There are obvious parallels with the return pair of the same series.
Interestingly, there is no lead–lag relation between Ghana and Liberia from 2003 through to 2007 within the 10–14-month band. Also, out-of-phase 2007–2009 and 2011 co-movement for which Liberia leads within the 10–14 band. There is the isolated high 26 monthly frequency in-phase co-movement in which Ghana leads in late 2009. Finally, there is sparsity as well as differing phases in the cone of influence albeit weak co-movements from 2002–2014 with the 2–10-month band.
Also, between Liberia and Nigeria seems to exhibit the strongest co-movement. From top–down; at the 50-month frequency from 2005 to late 2007, within the 20–32 band for 2008–2011, within the 32–36 band for 2011–2013, within 10–12 band for 2003–2005 and within the 3–5 band for late 2011 the pair is in-phase and Nigeria leads. For 2007–2009 at 14 and 20 frequencies there is no discernible lead–lag relationship between the pair. Amidst differing sparsely distributed lead–lag and in-phase/out-phase co-movements, in 2003 and 2004 the pair is out-of-phase with the 4–6- and 3–5-month bands for which Nigeria leads in the former and Liberia leads in the latter.
4.4. Co-movement of returns of exchange rates
Similar to the levels of real exchange rates, all six pairs of countries exhibit high significant levels of co-movements within the region of influence at the composite level, although there are some red contours at varying frequencies over the sample period. Moreover, phase-difference (for lead–lag relationship) depicted by the direction of arrows differs across, pairs of countries, frequencies and time period. In essence, we can reject the null hypothesis that there is no (joint) periodicity in the pairs of series via p-values obtained from simulation.
The Gambia and Ghana exhibit strong co-movement at very high 33–64 monthly frequency band between late 2003 to end 2010 with The Gambia leading and Ghana lagging, although the series are in-phase. Next, in between 6–20 monthly frequency band different co-movements can be observed for which the more pronounced are out-of-phase with The Gambia leading for 2002–2004 and 2007–2008 but the series tends to be in-phase with Ghana leading for 2010–2011. Lastly, for late 2001 and early 2006 at low frequencies of 3–4, the series are out-of-phase with Ghana leading and The Gambia is lagging.
For The Gambia and Liberia there is no lead–lag relationship for 2008–2011, early 2004, and early 2011 at the 62–63, 14–15 and 15 frequency bands respectively. From 2002–2005, the pair is in-phase with Liberia leading in the 17–18-month band, however, the same is out-of-phase with The Gambia leading in the 15–17-month band for 2007–2009. Again, the pair is in-phase in the middle of 2009 at lower frequencies of 3–4 whilst Liberia leads. There are many isolated significant regions of periodicity in the 3–15-month band at the 5% level.
Between The Gambia and Nigeria, 5% significant contours portray sparsely weak co-movements all over the cone of influence with differing phases; at the 63-month band for 2008–2011, 3–4-month band in 2012 and 2013 there are no lead–lag associations. Nonetheless, between 8–10- and 30–40-month bands the pair is out-of-phase and The Gambia lags for 2005 and 2007–2009, respectively.
The co-movements between Ghana and Liberia are very similar in many respects to those between The Gambia and Nigeria. There are sparse as well as differing phases in the cone of influence albeit weak co-movements.. At the 63-month band for 2009–2010, 15-month band in 2008 and between 3–4-month bands in 2012 and 3013 there are no lead–lag associations. Nevertheless, between 8–10-and 33–50-month bands the pair is out-of-phase and The Gambia lags for late 2005 and early 2009–2011, respectively.
So far the strongest co-movement observed between Ghana and Nigeria with a few isolated significant regions of periodicity in the 3–63-month band at the 5% level. Between the 20–50-month band for 2002–2004 and 2007–2011, the pair is in-phase and Ghana leads. There are secluded no lead–lag (the most pronounced is in 2003 in the 6–7-month band) and differing phase relationships in the pair with the time interval. But, we observe that in the 15-month band between late 2004 and late 2006 and in the 5–15-month band in 2007, the pair is out-of-phase with Nigeria leading and in-phase with Nigeria leading, respectively. Conversely, Ghana leads the pair out-of-phase in late 2007 and for 2013–2014 at the 2–3- and 5–10-month band, correspondingly.
The last of the pair is between Liberia and Nigeria; characterised by weak and sparse contours of co-movement of varying lead and phase difference relationships. The best of co-movement is observed from 2003–2007 in the 20–32-month band when the pair is in-phase and Nigeria leads. Next Liberia leads in-phase in 2009 between 18–20 monthly frequency band (Panel ).
5. Conclusions and recommendations
The quest of convergence and integration of economies in the WAMZ has remained an integral constituent of the attempts by the proponents of the formation of regional economic integration and adoption of a single currency for the ECOWAS sub-region. The WAMZ project is an important step geared towards the amalgamation of Africa and to purposefully alleviate the multiplicity of currencies in Africa. There is a growing concern and interest in the nature of real exchange rate changes in the member countries and their convergence for the WAMZ integration. Such changes are an important influence on cross-border trade and investment. In view of attempts to promote regional trade and investment, there is also undaunted interest in currency blocs and the co-movement of exchange rates in a region. In addition, after the late 1980s’ and early 1990s’ move towards economic integration in the region, there seems to be some evidence of cointegration among the currencies.
We employ the wavelet coherency and phase difference within the Continuous Morlet Wavelet Transform to examine the co-movement of real exchange rates and returns in the WAMZ. This paper is the first to examine the stochastic nature and co-movements of real exchange rates in the WAMZ over the recent period for evidence of synchronisation of exchange rates markets using this technique. The analysis is based on monthly data of real exchange rates for four member countries of the WAMZ from January 2001 to April 2015. This advanced technique enables us to delineate the significant frequency–time domain lead–lag relationships between the bilateral real exchange rates for the WAMZ member countries and their absolute log returns. Three different ways of lead–lag causal relationship, covariance/correlation and coherence are assessed.
The results indicate changing dynamics of the relationships between real exchange rates at different periodicities/timescales. No one country comes off as leading conveniently for both real and absolute returns of the exchange rates. These results indicate weakened prospects for economic integration amongst the ECOWAS countries over time. The findings corroborate other evidences of non-convergence of exchange rates in the monetary zone, which encumbers the eventual implementation of the single currency in the ECOWAS region. Real exchange rates in the WAMZ behave in dissimilar dimensions since it is difficult to use the returns of real exchange rates in other markets to explain the returns obtained in any of the foreign exchange markets. The discrepancies between real exchange rates are high, suggesting a more disparate dynamics in the exchange rates markets over the long run.
In a bid to consolidate and speed up the integration process in the sub-region, member countries must fully subscribe to the pursuance of credible macroeconomic policies aimed at attaining the convergence benchmarks. Member countries must relentlessly strive to implement appropriate monetary and fiscal policies as well as rigorous structural and institutional policies in accordance with the ECOWAS Multilateral Surveillance Mechanism. Issues of profligate spending and fragile fiscal positions, weakened governance institutions, unwarranted political pressures, amongst others must be purged. This implores the need for more determined and committed political leaders and governments.
The evidence of dissimilar dynamics of foreign exchange markets underscores the need to ensure the stability of real exchange rates in the economies by changing the structures of the economies as well as ensuring stability of macroeconomic fundamentals. Such policies must seek to alleviate the production and export of primary commodities, dependence on foreign goods and services, use of foreign currencies for domestic transactions, and other factors that weaken their currencies. The proper coordination and harmonisation of fiscal, monetary and exchange rate policies is also imperative to facilitate trade and investment within and amongst member countries.
Funding
The authors received no direct funding for this research.
Additional information
Notes on contributors
Peterson Owusu Junior
Our main areas of research coalesce into financial econometrics. Coming from similar backgrounds of finance and financial engineering, we delight in research encompassing these related fields. Some of our previous topics are in financial distributions, volatility models, interest convergence, long memory of interest rates, integration of African stock markets, generalised lambda distributions, etc. This study is in line with the broad studies on the convergence on exchange rates in the West African Monetary Zone (WAMZ).
Peterson Owusu Junior has MSc Financial Engineering from Malardalen University in Sweden. He currently facilitates the Financial Engineering & Risk Management course at the Institute for Distance Learning, Kwame Nkrumah University of Science & Technology and Financial Modelling and Statistics for Business Research courses at the College of Distance Education, University of Cape Coast, both in Ghana.
Notes
1. There are two categories of convergence benchmarks: primary and secondary. They include stable exchange rates, single digit inflation rate, budget deficit as a percentage of Gross Domestic Product (GDP), central bank financing of government debt, gross reserves per import cover, ratio of tax revenue to GDP target, wage bill, public investment finance from tax revenue, interest rate, stock of domestic arrears, amongst others.
References
- Abdalla, S. Z. S. (2012). Modelling exchange rate volatility using GARCH models: Empirical evidence from Arab countries. International Journal of Economics and Finance, 4, 216.
- Adam, A. M., Agyapong, D. A., & Gyamfi, E. N. (2012). Dynamic macroeconomic convergence in the West Africa Monetary Zone (WAMZ). Retrieved from https://papers.ssrn.com/sol3/papers.cfm?abstract_id=2007994
- Agyapong, D., & Adam, A. M. (2012). Exchange rate behaviour: Implication for West African Monetary Zone. International Journal of Academic Research in Accounting, Finance and Management Sciences, 2, 216–229.
- Alagidede, P., Tweneboah, G., & Adam, A. M. (2008). Nominal exchange rates and price convergence in the West African Monetary Zone. International Journal of Business and Economics, 7, 181.
- Aloui, C., & Hkiri, B. (2014). Co-movements of GCC emerging stock markets: New evidence from wavelet coherence analysis. Economic Modelling, 36, 421–431.10.1016/j.econmod.2013.09.043
- Antonakakis, N. (2012). Exchange return co-movements and volatility spillovers before and after the introduction of euro. Journal of International Financial Markets, Institutions and Money, 22, 1091–1109.10.1016/j.intfin.2012.05.009
- Baig, M. T. (2001). Characterizing exchange rate regimes in post-crisis East Asia. International Monetary Fund. Retrieved from https://books.google.com/books?hl=en&lr=&id=Iohyl0oabrsC&oi=fnd&pg=PP2&dq=exchange+rate+comovement+in+middle+east&ots=hDYMYF5DyY&sig=iMGqiQPWhaV07PZMCwW9jm7Wfw4
- Baxter, M., & Stockman, A. C. (1989). Business cycles and the exchange-rate regime: Some international evidence. Journal of Monetary Economics, 23, 377–400.10.1016/0304-3932(89)90039-1
- Beirne, J., & Gieck, J. (2014). Interdependence and contagion in global asset markets. Review of International Economics, 22, 639–659.10.1111/roie.2014.22.issue-4
- Bekiros, S., & Marcellino, M. (2013). The multiscale causal dynamics of foreign exchange markets. Journal of International Money and Finance, 33, 282–305.10.1016/j.jimonfin.2012.11.016
- Boako, G., & Alagidede, P. (2017). Co-movement of Africa’s equity markets: Regional and global analysis in the frequency–time domains. Physica A: Statistical Mechanics and Its Applications, 468, 359–380.10.1016/j.physa.2016.10.088
- Boako, G., & Alagidede, P., & et al. (2016). Regionalization versus internationalization of African stock markets: A frequency-time domain analysis. Retrieved from https://www.researchgate.net/profile/Gideon_Boako/publication/309160305_Regionalization_versus_internationalization_of_African_stock_markets_A_frequency-time/links/5802068908ae6c2449f7c5fa.pdf
- Burrus, C. S., Gopinath, R. A., & Guo, H. (1998). Wavelets and wavelet transforms (Houston ed., p. 98). Houston: Rice University.
- Conraria, L. A., & Soares, M. J. (2011). The continuous wavelet transform: A primer. NIPE Working Paper, 16, 1–43.
- Corsetti, G., Pericoli, M., & Sbracia, M. (2005). “Some contagion, some interdependence”: More pitfalls in tests of financial contagion. Journal of International Money and Finance, 24, 1177–1199.10.1016/j.jimonfin.2005.08.012
- Dias, A., & Embrechts, P. (2010). Modeling exchange rate dependence dynamics at different time horizons. Journal of International Money and Finance, 29, 1687–1705.10.1016/j.jimonfin.2010.06.004
- Dupasquier, C., Osakwe, P. N., & Thangavelu, S. M. (2006). Singapore Centre for Applied and Policy Economics. Retrieved from https://citeseerx.ist.psu.edu/viewdoc/download?doi=10.1.1.527.6533&rep=rep1&type=pdf
- Engle, R. F., Ito, T., & Lin, W.-L. (1988). Meteor showers or heat waves? Heteroskedastic intra-daily volatility in the foreign exchange market (Working Paper No. 2609). National Bureau of Economic Research. Retrieved from https://www.nber.org/papers/w260910.3386/w2609
- Ftiti, Z., Tiwari, A., Belanès, A., & Guesmi, K. (2015). Tests of financial market contagion: Evolutionary cospectral analysis versus wavelet analysis. Computational Economics, 46, 575–611.10.1007/s10614-014-9461-8
- Gabor, D. (1946). Theory of communication. Part 1: The analysis of information. Journal of the Institution of Electrical Engineers-Part III: Radio and Communication Engineering, 93, 429–441.
- Ghosh, A. R., Gulde-Wolf, A.-M., & Wolf, H. C. (2002). Exchange rate regimes: Choices and consequences (vol. 1). MIT press. Retrieved from https://books.google.com/books?hl=en&lr=&id=lnDFKt0S48UC&oi=fnd&pg=PA1&dq=exchange+rate+movement+in+europe&ots=B9PmCIAec4&sig=Dv_tIODqPZ1ese1O3E7dc7vKRLM
- Grinsted, A., Moore, J. C., & Jevrejeva, S. (2004). Application of the cross wavelet transform and wavelet coherence to geophysical time series. Nonlinear Processes in Geophysics, 11, 561–566.10.5194/npg-11-561-2004
- Grossman, A., & Morlet, J. (1984). SIAM (Society for Industrial and Applied Mathematics). Journal of Mathematical Analysis, 15, 273.
- Heil, C. E., & Walnut, D. F. (1989). Continuous and discrete wavelet transforms. SIAM Review, 31, 628–666. doi:10.1137/1031129
- Hsing, Y. (2007). The roles of the exchange rate and the foreign interest rate in Estonia’s money demand function and policy implications. Applied Financial Economics Letters, 3, 221–224.10.1080/17446540600706916
- In, F., & Kim, S. (2013). An introduction to wavelet theory in finance: A wavelet multiscale approach. World Scientific. Retrieved from https://books.google.com/books?hl=en&lr=&id=D3O6CgAAQBAJ&oi=fnd&pg=PR5&dq=in+and+kim+book++Wavelet&ots=Hw6MzzNMtx&sig=1U8Q1WSo1CwPEBnclbGvVzCJRtU
- Inagaki, K. (2007). Testing for volatility spillover between the British pound and the euro. Research in International Business and Finance, 21, 161–174.10.1016/j.ribaf.2006.03.006
- Johnson, R., & Soenen, L. (2002). Asian economic integration and stock market comovement. Journal of Financial Research, 25, 141–157.10.1111/jfir.2002.25.issue-1
- Kargbo, J. M. (2000). Impacts of monetary and macroeconomic factors on food prices in eastern and southern Africa. Applied Economics, 32, 1373–1389.10.1080/00036840050151467
- Lane, P. R., & Milesi-Ferretti, G. M. (2006). Exchange rates and external adjustment: Does financial globalization matter? Retrieved from https://papers.ssrn.com/sol3/papers.cfm?abstract_id=923770
- Lin, C.-H. (2012). The comovement between exchange rates and stock prices in the Asian emerging markets. International Review of Economics & Finance, 22, 161–172.10.1016/j.iref.2011.09.006
- Madaleno, M., & Pinho, C. (2010a). Hedging performance and multiscale relationships in the German electricity spot and futures markets. Journal of Risk and Financial Management, 3, 26–62.10.3390/jrfm3010026
- Madaleno, M., & Pinho, C. (2010b). Relationship of the multiscale variability on world indices. Revista De Economia Financiera, 20, 69–92.
- Madaleno, M., & Pinho, C. (2012). International stock market indices comovements: A new look. International Journal of Finance & Economics, 17, 89–102.10.1002/ijfe.v17.1
- Masih, M., & Majid, H. A. (2013). Comovement of selected international stock market indices: A continuous wavelet transformation and cross wavelet transformation analysis. Retrieved from https://mpra.ub.uni-muenchen.de/id/eprint/58313
- Morlet, J., Arens, G., Fourgeau, E., & Glard, D. (1982). Wave propagation and sampling theory—Part I: Complex signal and scattering in multilayered media. Geophysics, 47, 203–221. doi:10.1190/1.1441328
- Nikkinen, J., Pynnönen, S., Ranta, M., & Vähämaa, S. (2011). Cross-dynamics of exchange rate expectations: A wavelet analysis. International Journal of Finance & Economics, 16, 205–217.10.1002/ijfe.v16.3
- Orlov, A. G. (2009). A cospectral analysis of exchange rate comovements during Asian financial crisis. Journal of International Financial Markets, Institutions and Money, 19, 742–758.10.1016/j.intfin.2008.12.004
- Patton, A. J. (2006). Modelling asymmetric exchange rate dependence. International Economic Review, 47, 527–556.10.1111/iere.2006.47.issue-2
- Pérez-Rodríguez, J. V. (2006). The euro and other major currencies floating against the US dollar. Atlantic Economic Journal, 34, 367–384.10.1007/s11293-006-9042-x
- Pinho, C., & Madaleno, M. (2011). Multiscale analysis of European electricity markets. Departemento de Economia Gestão E Engenharia Industrial, Universidade de Aveiro. Retrieved from https://pdfs.semanticscholar.org/e7e3/ee5356efec3e43675426bb05a371b81ed5ac.pdf
- Pukthuanthong, K., & Roll, R. (2009). Global market integration: An alternative measure and its application. Journal of Financial Economics, 94, 214–232.10.1016/j.jfineco.2008.12.004
- Ranta, M. (2010). Wavelet multiresolution analysis of financial time series. Universitas Wasaensis. Retrieved from https://www.uva.fi/materiaali/pdf/isbn_978-952-476-303-5.pdf
- Reboredo, J. C., & Rivera-Castro, M. A. (2013). A wavelet decomposition approach to crude oil price and exchange rate dependence. Economic Modelling, 32, 42–57.10.1016/j.econmod.2012.12.028
- Roesch, A., & Schmidbauer, H. (2014). Package “WaveletComp.” Retrieved from https://pbil.univ-lyon1.fr/CRAN/web/packages/WaveletComp/WaveletComp.pdf
- Rua, A. (2010). Measuring comovement in the time–frequency space. Journal of Macroeconomics, 32, 685–691.10.1016/j.jmacro.2009.12.005
- Scott, H. S. (2016). Connectedness and contagion: Protecting the financial system from panics. Cambridge: MIT Press.10.7551/mitpress/9780262034371.001.0001
- Seck, D. (2014). Regional economic integration in West Africa|Diery Seck|Springer. Springer International Publishing. Retrieved from https://www.springer.com/gp/book/978331901281010.1007/978-3-319-01282-7
- Tamakoshi, G., & Hamori, S. (2014). Co-movements among major European exchange rates: A multivariate time-varying asymmetric approach. International Review of Economics & Finance, 31, 105–113.10.1016/j.iref.2014.01.016
- Torrence, C., & Compo, G. P. (1998). A practical guide to wavelet analysis. Bulletin of the American Meteorological Society, 79, 61–78.10.1175/1520-0477(1998)079<0061:APGTWA>2.0.CO;2
- Torrence, C., & Webster, P. J. (1999). Interdecadal changes in the ENSO–monsoon system. Journal of Climate, 12, 2679–2690.10.1175/1520-0442(1999)012<2679:ICITEM>2.0.CO;2
- Tweneboah, G., Agyapong, D., & Frimpong, S. (2016). Economic integration and exchange rate dynamics in the West African monetary zone. African Finance Journal, 18, 53–76.
- Uddin, G. S., Tiwari, A. K., Arouri, M., & Teulon, F. (2013). On the relationship between oil price and exchange rates: A wavelet analysis. Economic Modelling, 35, 502–507.10.1016/j.econmod.2013.07.035
- Veleda, D., Montagne, R., & Araujo, M. (2012). Cross-wavelet bias corrected by normalizing scales. Journal of Atmospheric and Oceanic Technology, 29, 1401–1408.10.1175/JTECH-D-11-00140.1
- Vlachos, M., Yu, P., & Castelli, V. (2005). On periodicity detection and structural periodic similarity. In Proceedings of the 2005 SIAM international conference on data mining (pp. 449–460). SIAM. Retrieved from https://epubs.siam.org/doi/abs/10.1137/1.9781611972757.40
- Yehoue, E. B. (2005). International risk sharing and currency unions: The CFA zones. Retrieved from https://papers.ssrn.com/sol3/papers.cfm?abstract_id=888141