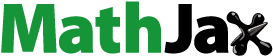
Abstract
This study investigates the causal relationship between poverty reduction and foreign direct investment (FDI) inflows in South Africa using time-series data from 1980 to 2014. The main objective of this study is to establish the direction of causality between FDI and poverty reduction, which is important to policy-makers as it identifies which variable to target first. Gross domestic product is included as an intermittent variable giving a trivariate framework. Employing the autoregressive distributed lag (ARDL) bounds testing approach to cointegration and ECM-based causality tests, the results from this study reveal a distinct unidirectional causality from poverty reduction to FDI in both the short run and the long run when poverty reduction is measured by life expectancy and infant mortality rate. However, the study failed to find any causality, irrespective of the time considered, when poverty reduction is measured by household consumption expenditure. It can be concluded therefore, that the causal relationship between FDI and poverty reduction is sensitive to the proxy used to measure the level of poverty reduction.
Public Interest Statement
This study investigates the causality between foreign direct investment inflows and poverty reduction in South Africa using a trivariate framework. The intermittent variable added to the Granger-causality equation is real gross domestic product. To capture the multidimensional aspects of poverty, three poverty reduction measures are used in this study namely, household consumption expenditure (Pov1), infant mortality rate (Pov2) and life expectancy (Pov3). The findings from this study reveal that the causality between FDI and poverty reduction is sensitive to the poverty reduction used and the time considered—long run or short run. Based on the results from this study, it can be concluded that the measure of poverty reduction is important if policy-makers are to use foreign direct investment-based policies to reduce poverty.
1. Introduction
The causal relationship between poverty reduction and foreign direct investment (FDI) has received little coverage in the literature with only a few studies having analysed the causal relationship between the two variables. The majority of the studies on poverty reduction and FDI have focused on the impact of FDI on poverty reduction. Yet, it is equally important to establish the causal relationship between FDI and poverty reduction for policies that effectively assists in poverty reduction. Thus, the direction of causality indicates which of these two variables can be influenced first in order to achieve a change in the other variable as desired. Moreover, of the few studies that have attempted to establish the casual relationship between FDI and poverty, most have employed a bivariate causality framework, which is now known to have some limitations (see Odhiambo, Citation2008; Solarin & Shahbaz, Citation2013). The inclusion of a third intermittent variable can alter the direction of causality or the magnitude of variables (Loizides & Vamvoukas, Citation2005; Odhiambo, Citation2009b). In this study, gross domestic product is included as an intermittent variable. The link between poverty reduction, GDP and FDI is influenced by the growth theories. In the endogenous, exogenous and Schumpeterian growth theories, economic growth is achieved through capital accumulation—including FDI. Growth realised from capital accumulation results in improved living standards that lead to poverty reduction. Thus making GDP an important variable on the causality between poverty reduction and FDI.
Moreover, among the few studies that have analysed causality between FDI and poverty reduction the results are inconclusive. Some studies have found unidirectional causality between the two variables (see Gohou & Soumaré, Citation2012). A bidirectional causal relationship has also been found (see, e.g. Soumare, Citation2015). Other studies have found no causal relationship between FDI and poverty (see Ogunniyi & Igberi, Citation2014). The results of these studies have varied depending on the poverty measure used, the sample period, and the methodology employed. This makes generalisation of the findings across all countries inappropriate.
The main objective of this study, therefore, is to establish the causal relationship between FDI and poverty reduction in South Africa between 1980 and 2014 using the Granger-causality test. The study differs fundamentally from previous studies in a number of ways. First, the study analyses the causal relationship between FDI and poverty reduction within a trivariate framework. This overcomes the limitations of a bivariate framework that has been employed in other studies with the omission of variable bias (see Solarin & Shahbaz, Citation2013). Second, the study investigates the causal relationship between FDI and poverty reduction using three poverty reduction proxies, which are household consumption expenditure (Pov1), infant mortality rate (Pov2) and life expectancy (Pov3). Unlike previous studies that have relied on one poverty reduction proxy, the inclusion of three poverty reduction proxies gives another angle on causality in the study country. Third, the ARDL bounds testing approach to cointegration employed in this study has a number of advantages. For instance, the ARDL bounds approach to cointegration is robust in small samples (see also Odhiambo, Citation2008; Solarin & Shahbaz, Citation2013).
South Africa has been selected in this study mainly because it is one of the largest economies as measured by GDP in Africa, while the country also receives fairly high FDI inflows (World Bank, Citation2016). An investigation of South Africa will shed more light on the causal relationship between FDI inflows and poverty reduction. This will provide more information to policy-makers regarding policy directions in relation to poverty reduction and FDI.
The rest of the paper is set out as follows: Section 2 provides a brief review of the literature; Section 3 outlines estimation techniques covering variable definition, specification of the models, and data sources; Section 4 discusses the results of the study; and Section 5 concludes the study.
2. Literature review
2.1. Foreign direct investment and poverty reduction dynamics in South Africa
South Africa was among the nations that signed the United Nations’ Millennium Development Goals (MDGs) declaration in 2000, indicating the government’s effort to reduce poverty (United Nations, Citation2000). South Africa is also a signatory to the Sustainable Development Goals 2030, which was signed in 2015 after the expiry of the MDGs (United Nations, Citation2017). The country is actively involved in programmes that involve the eradication of poverty. Beside international collaboration, the country’s development plans also support poverty reduction, starting with the Reconstruction and Development White paper to the National Development Plan 2030 (Government Gazette, Citation1994; National Planning Commission, Citation2011). Apart from poverty reduction initiatives, the government has rolled out policies that have opened the South African economy to the global economy (Government Gazette, Citation1994; National Planning Commission, Citation2011). The policies that the government adopted aimed, among other objectives, to increase foreign direct investment (FDI). For instance, government implemented polices that focused on increasing FDI inflows to augment domestic capital and spur economic growth, which is associated with an increase in standards of living. The policies implemented by the government include sound industrial policies, bilateral and multilateral investment agreements aimed at increasing market access for South African goods, regional integration initiatives, trade liberalisation, regulatory reforms and capital account liberalisation, among other policies. In response to policies advanced by the government on poverty reduction and attracting FDI, South Africa has realised a gradual increase in FDI inflows and also a reduction in poverty (Statistics South Africa, Citation2015; World Bank, Citation2016).
The various policy reforms have resulted in a gradual increase in FDI flows into South Africa. Although FDI inflows as a percentage of GDP were depressed between 1980 and 1994, inflows took an upward trend from 1994 (World Bank, Citation2016). The average share of FDI to GDP between 1994 and 2014 was 0.9% (World Bank, Citation2016). On the poverty front, the incidence of poverty, as measured by the poverty headcount at $1.90/day, declined from 31.9% in 1993 to 16.6% in 2011 (World Bank, Citation2016). There has been a general decrease in poverty in South Africa as evidenced by other poverty measures like the human development index and poverty gap (World Bank, Citation2016). It is interesting to note, though, that there is a wide difference in poverty levels across provinces, according to sex, age and settlement type in South Africa (Statistics South Africa, Citation2014). What remains uncertain is if FDI has had a role to play in the reduction in poverty experienced.
2.2. Empirical literature review
Empirical literature on the causal relationship between FDI and poverty reduction is still at the nascent stage. Of the few studies that have attempted to analyse causality between FDI and poverty reduction, the results are mixed. Some studies have found unidirectional causality between FDI and poverty reduction, while others have found bidirectional causality between these variables. A further set of studies have found no causal relationship between FDI and poverty reduction.
Fauzel, Seetanah, and Sannassee (Citation2015) investigated the impact of foreign direct investment inflows on poverty reduction in selected Sub-Saharan countries from 1990 to 2010. Using poverty headcount as a poverty reduction measure, they found FDI to Granger-cause poverty reduction. Gohou and Soumaré (Citation2012), investigated the causality between FDI and poverty in five regional economic communities and five customs and monetary unions in Africa. Using Human Development Index (HDI) as a measure of welfare, a uni-directional causality was found running from FDI to HDI. In a separate study, Soumare (Citation2015) studied causal relationship between FDI and poverty in North Africa between 1990 and 2011. Using Granger-causality test a uni-directional causality was found running from FDI to HDI in Egypt, Morocco, Tunisia and Mauritania.
While some studies have found unidirectional causality between FDI and poverty, others have found bidirectional causality between these variables. Gohou and Soumaré (Citation2012) examined the causal relationship between FDI and poverty in five regional economic communities and five customs and monetary unions in Africa from 1990 to 2007. In this study, GDP per capita was used as a poverty proxy and the Granger-causality test was employed. They found a bidirectional relationship between GDP per capita and FDI in the whole region. In a study on North African countries from 1990 to 2011, Soumare (Citation2015) employed the Granger-causality test and found bidirectional causality between FDI and HDI in Algeria. In the same study, when real per capita GDP was used as poverty proxy, a bidirectional causal relationship was found in all the countries, except for Libya.
A further set of studies have found no causality between FDI and poverty. For example, Ogunniyi and Igberi (Citation2014) investigated the causal relationship between FDI and standard of living in Nigeria between 1980 and 2012. Using per capita income as a standard of living proxy and employing the Granger-causality test, they found no causality between FDI and poverty. Thus, the empirical findings with respect to the causal relationship between FDI and poverty are inconclusive.
3. Estimation techniques
This study is based on the ARDL-bounds test and the ECM-based causality test. The ARDL test has been selected because of a number of advantages. The ARDL approach to cointegration is robust in a small sample (see also Odhiambo, Citation2009a; Solarin & Shahbaz, Citation2013). While other conventional approaches to cointegration have a restrictive assumption concerning the order of integration of variables, the ARDL bounds test can be used even when series have a different order of integration (Pesaran, Shin, & Smith, Citation2001: 290; Solarin & Shahbaz, Citation2013). Another advantage of using the ARDL approach to cointegration is that it provides unbiased estimates of the long-run model, even in cases where some variables are endogenous (Odhiambo, Citation2009a). The ARDL approach also uses a reduced form single equation, while other conventional cointegration methods employ a system of equations (Pesaran & Shin, Citation1999). Given these advantages, the study used the ARDL bounds testing approach to cointegration. To determine cointegration, the null hypothesis of no cointegration is tested against the alternative hypothesis of cointegration. The calculated F-statistic is compared to the critical values provided by Pesaran et al. (Citation2001). If the calculated F-statistic falls above the critical value, we reject the null hypothesis of no cointegration. Alternatively, if the F-statistic falls below the lower bound, we conclude there is no cointegration. However, if the F-statistic falls between the upper and the lower bound, the results are inconclusive.
After confirming the existence of a long-run relation, the next step is establishing the direction of causality. The presence of cointegration only indicates the presence of a long-run relationship and the existence of causality in at least one direction (Narayan & Smyth, Citation2004). The causal relationship between poverty reduction and FDI is investigated using the ECM-based approach within a trivariate framework. The gross domestic product is added as an intermittent variable to give a trivariate causality framework consisting of poverty reduction—Pov1, Pov2, Pov3, FDI and GDP. This is in response to a weakness of a bivariate framework that the results may suffer from omission of variable bias (among others, see Odhiambo, Citation2008). The use of a trivariate framework can improve the magnitude of the results (see also Odhiambo, Citation2009a).
In the literature, a number of poverty reduction proxies have been used, including GDP per capita, infant mortality rate, household consumption expenditure, and certain poverty indices, among other poverty proxies. Due to limited time-series data on other proxies and the need to capture income and non-income poverty, household consumption expenditure (Pov1), infant mortality rate (Pov2) and life expectancy (Pov3) were used. Three models—Models 1–3—were specified to capture the three poverty reduction proxies. In Model 1, poverty reduction is proxied by household consumption expenditure, and the model specification is Pov1|FDI, GDP. Infant mortality rate (Pov2) is used as a poverty reduction proxy in Model 2, and the model specification is Pov2|FDI, GDP. In Model 3, life expectancy (Pov3) is used as a poverty reduction proxy, and the model is specified as Pov3|FDI, GDP. The definition of variables included in the study is given in Table .
Table 1. Variable definition
Following Odhiambo (Citation2008) and Narayan and Smyth (Citation2008), the ARDL-bounds specification for Models 1–3 are given Equations (1)–(9).
ARDL specification for Model 1 (Pov1, FDI and GDP)(1)
(1)
(2)
(2)
(3)
(3)
ARDL specification for Model 2 (Pov2, FDI and GDP)
(4)
(4)
(5)
(5)
(6)
(6)
ARDL specification for Model 3 (Pov3, FDI and GDP)(7)
(7)
(8)
(8)
(9)
(9)
where α0 is a constant, α1 − α3 and are regression coefficients, and
is an error term.
3.1. A Granger-causality model specification
The ECM-based Granger-causality models are specified for Model 1, Model 2, and Model 3. The introduction of the lagged error correction term reintroduces the long-run relationship that could have been lost with differencing (see Odhiambo, Citation2009a) The ECM-based causality test also enables analysis of causality in both the short run and the long run. The F-statistics obtained from the variable deletion test or the Wald test gives the short-run causality, while the long-run relationship is given by the t-statistic on the lagged error correction term. The ECM-based Granger-causality model used in this study can be expressed as follows:
ECM-based Granger-causality for Model 1 (Pov1, FDI, GDP)
The ARDL Granger-causality model specification for Model 1 is given in Equations (10)–(12).
(10)
(10)
(11)
(11)
(12)
(12)
where α0 is a constant, α1 − α3 and are regression coefficients, and
are the error terms.
ECM-based Granger-causality for Model 2 (Pov2, FDI, GDP)
The ARDL Granger-causality model specification for Model 2 is given in Equations (13)–(15).
(13)
(13)
(14)
(14)
(15)
(15)
where is a constant,
-
and
are regression coefficients and
are the error terms.
ECM-based Granger-causality for Model 3 (Pov3, FDI, GDP)
The ARDL Granger-causality model specification for Model 3 is given in Equations (16)–(18).
(16)
(16)
(17)
(17)
(18)
(18)
where α0 is a constant, α1 − α3 and are regression coefficients and
are the error terms
3.2. Data sources
The study used time-series data from 1980 to 2014 to investigate the dynamic causal relationship between poverty reduction and FDI. The data employed in this study was obtained from the World Bank development indicators. Microfit 5.0 was used to analyse the data in this study.
4. Empirical Results
4.1. Unit Root Test
The ARDL approach to cointegration does not require pretesting of variables for stationarity. In this study, unit root tests is done on Pov1, Pov2, Pov3, FDI and GDP to confirm if the variables are integrated of at most order 1 [I (1)]. The ARDL approach is only applicable if variables are integrated of order 0 [I (0)], order 1 [I (1)], or fractionally integrated (Pesaran et al., Citation2001). The results of Dickey-Fuller Generalised Least Square (DF-GLS), Phillip-Perron (PP root) and Perron unit root test (PPU root test) are presented in Table .
Table 2. Unit root test result
Although the results of the unit root tests varied from one test to the other and from one poverty reduction proxy to the other, overall the variables are stationary in first difference. Only FDI is consistently stationary in levels across all three tests of unit root. The results confirm the suitability of the ARDL approach to cointegration and causality analysis.
4.2. ARDL Bounds Testing Approach to Cointegration
The cointegration results are presented in Table .
Table 3. ARDL bounds test for cointegration results—Model 1–3
The results in Table confirm cointegration between poverty reduction, FDI and GDP. The F-statistics confirm the existence of cointegration between Pov1, Pov2, Pov3, FDI and GDP. Cointegration is confirmed in the following functions: Model 1, F (Pov1|FDI, GDP) and F (FDI|Pov1, GDP); Model 2, F (FDI|Pov2, GDP); and Model 3 F (Pov3|FDI, GDP) and F (FDI|Pov3, GDP). The presence of integration in these functions indicates the presence of causality in at least one direction (see Granger, Citation1988; Narayan & Smyth, Citation2008). The direction of causality is obtained by running an ECM-based causality test. A further investigation is done to determine the direction of causality using the ECM-based causality test.
4.3. ECM-based causality testing
The results of the ECM-based causality test are reported in Table .
Table 4. ECM-based causality results
The empirical results reported in Table , Panel A, for Model 1, where Pov1, FDI, and gross domestic product (GDP) are included, reveal that in South Africa, no short-run or long-run causality exists between FDI and poverty reduction (Pov1). The results suggest that there is no Granger-causality between FDI and poverty reduction in South Africa, irrespective of the time considered, when household consumption expenditure is used as a poverty reduction measure. The findings from this study, although not expected, compare favourably with some other studies (see Ogunniyi & Igberi, Citation2014). Other results presented in Table , Panel A, confirm that in South Africa there is (i) bidirectional causality between GDP and poverty reduction (Pov1) in the short run; (ii) unidirectional causality from GDP to poverty reduction (Pov1) in the long run; and (iii) unidirectional causality from GDP to FDI in the short run and in the long run.
Empirical results reported in Table , Panel B, for Model 2, where poverty reduction is captured by infant mortality rate (Pov2), show that in South Africa, there is unidirectional causality from poverty reduction (Pov2) to FDI. These results apply irrespective of whether the estimation is done in the short run or in the long run. Short-run causality is confirmed by an F-statistic for ΔPov2 in the ΔFDI function that is statistically significant at the 5% significance level. Long-run causality is shown by the error correction term (ECM) in the ΔFDI function that is negative and statistically significant at the 1% significance level. Thus, in South Africa, it is poverty reduction that Granger-causes FDI, both in the long run and the short run, when poverty reduction is measured by infant mortality rate. Other results reported in Table , Panel B, reveal that there is (i) bidirectional causality between poverty reduction and GDP in the short run and (ii) unidirectional causality from FDI to GDP in the short run.
The empirical results in Table , Panel C, for Model 3 show that there is a distinct unidirectional causality from Pov3 to FDI in the short run and in the long run in South Africa. The significant short-run causality is supported by a significant F-statistic for ΔPov3 in the ΔFDI function, while long run causality is supported by a negative error correction term that is statistically significant at the 1% level of significance. The results suggest that poverty reduction Granger-causes FDI in South Africa, irrespective of the time considered. The results are consistent with findings from other studies where absorption capacity was found to be important in increasing the capacity to benefit from FDI (see, e.g. Klein, Aaron, & Hadjimichael, Citation2001:5). Other results reported in Table , Panel C, show that in South Africa, there is (i) bidirectional causality between poverty reduction and GDP in the short run and unidirectional causality from GDP to poverty reduction in the long run; and (ii) bidirectional causality between FDI and GDP in the short run and unidirectional causality from GDP to FDI in the long run. A summary of the Granger-causality results is given in Table .
Table 5. Summary of granger causality results
5. Conclusion
In this study, the causal relationship between poverty reduction and FDI is investigated using time-series data for South Africa from 1980 to 2014. Gross domestic product is included as a third variable in the analysis to form a trivariate Granger-causality test. The intermittent variable was used to overcome the limitations of a bivariate causality test, where the results can be improved or altered due to the inclusion of the intermittent variable. The study employed the ECM-based causality test to investigate the Granger-causality relationship between FDI and poverty reduction. Three poverty reduction measures are used in this study to minimise reliance on one variable. To this end, three models are used: Model 1 with household consumption expenditure as a measure of poverty reduction, Model 2 with infant mortality rate as the second poverty reduction measure and Model 3 with life expectancy as a proxy for poverty reduction. The findings from this study reveal that the causal relationship between FDI and poverty reduction is sensitive to the poverty reduction proxy used and the time considered. No causality is confirmed when household consumption expenditure is used as a poverty reduction measure. However, when infant mortality rate and life expectancy are used as poverty reduction proxies, unidirectional causality is recorded from poverty reduction to FDI. The results, therefore, point to two policy implications which are: (i) the importance of the poverty reduction proxy selected by policy-makers; and (ii) a need for a perfect timing of FDI-based policies. These two aspects are crucial if FDI-based poverty reduction policies are used.
Additional information
Funding
Notes on contributors
M.T. Magombeyi
The researcher’s interests are in macroeconomics, econometrics and development economics.
This paper investigates the causal relationship between poverty reduction and foreign direct investment inflows in South Africa between 1980 and 2014. The research is motivated by the growing need to eradicate poverty championed by the United Nations, which resulted in the Millennium Development Goals and Sustainable Development Goals. To this end, the main objective of this paper is to establish if South Africa can use foreign direct investment inflows as a policy instrument to reduce poverty. The causality between the two variables assists policy-makers by establishing the variable that can be influenced first to get a positive impact on the other.
References
- Fauzel, S., Seetanah, B., & Sannassee, R. V. (2015). Foreign direct investment and welfare nexus in sub Saharan Africa. The Journal of Developing Areas, 49, 271–283.10.1353/jda.2015.0133
- Gohou, G., & Soumaré, I. (2012). Does foreign direct investment reduce poverty in Africa and are there regional differences? World Development, 40, 75–95.10.1016/j.worlddev.2011.05.014
- Government Gazette. (1994). White paper on reconstruction and development no 16085. Retrieved September 23, 2016, from https://www.info.gov.za
- Granger, C. W. (1988). Some recent development in a concept of causality. Journal of Econometrics, 39, 199–211.10.1016/0304-4076(88)90045-0
- Klein, M., Aaron, C., & Hadjimichael, B. (2001). Foreign direct investment and poverty reduction (Policy Research Working Paper 2613). Washington, DC: World Bank.10.1596/prwp
- Loizides, J., & Vamvoukas, G. (2005). Government expenditure and economic growth: Evidence from trivariate causality testing. Journal of Applied Economics, 8, 125–152.
- Narayan, P. K., & Smyth, R. (2004). Temporal causality and the dynamics of exports, human capital and real income in China. International Journal of Applied Economics, 1, 24–45.
- Narayan, P. K., & Smyth, R. (2008). Energy consumption and real GDP in G7 countries: New evidence from panel cointegration with structural breaks. Energy Economics, 30, 2331–2341.10.1016/j.eneco.2007.10.006
- National Planning Commission. (2011). National development plan: Vision for 2030. Retrieved June, 2016, from https://www.gov.za/sites/
- Odhiambo, N. M. (2008). Financial depth, savings and economic growth in Kenya: A dynamic causal linkage. Economic Modelling, 25, 704–713.10.1016/j.econmod.2007.10.009
- Odhiambo, N. M. (2009a). Energy consumption and economic growth nexus in Tanzania: An ARDL bounds testing approach. Energy Policy, 37, 617–622.10.1016/j.enpol.2008.09.077
- Odhiambo, N. M. (2009b). Electricity consumption and economic growth in South Africa: A trivariate causality test. Energy Economics, 31, 635–640.10.1016/j.eneco.2009.01.005
- Ogunniyi, M. B., & Igberi, C. O. (2014). The impact of foreign direct investment on poverty reduction in Nigeria. Journal of Economics and Sustainable Development, 5, 73–89.
- Pesaran, M. H., & Shin, Y. (1999). An autoregressive distributed lag modelling approach to cointegration analysis. In S. Storm (Ed.), Econometrics and Economic Theory in the 20th Century, The Ragnar Frisch Centennial Symposium (pp. 1–31). Cambridge: Cambridge University Press.
- Pesaran, M. H., Shin, Y., & Smith, R. (2001). Bounds testing approaches to the analysis of level relationship. Journal of Applied Econometrics, 16, 174–189.
- Solarin, S. A., & Shahbaz, M. (2013). Trivariate causality between economic growth, urbanisation and electricity consumption in Angola: Cointegration and causality analysis. Energy Policy, 60, 876–884.10.1016/j.enpol.2013.05.058
- Soumare, I. (2015). Does foreign direct investment improve welfare in North Africa? Abidjan: Africa Development Bank.
- Statistics South Africa. (2014). Poverty trends in South Africa: An examination of absolute poverty between 2006 and 2011. Retrieved June 13, 2016, from http://www.beta2.statssa.gov.za/publication/report
- Statistics South Africa. (2015). General household survey 2014. Retrieved June 8, 2016, from https://www.statssa.gov.za
- United Nations. (2000). United Nations Millennium Declaration. Ares/55/2. New York, NY: UN General Assembly.
- United Nations. (2017). Sustainable development goals. Retrieved April 10, 2017, from www.un.org/sustainabledevelopment/development-agent
- World Bank. (2016). Development indicators. Retrieved September 26, 2017, from http://www.data.worldbank.org