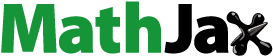
Abstract
It is very important to identify deviation mechanism of price volatility of an industry asset and the affecting factors, and it is important to give the reasonable explanation and measurement to the abnormality of price volatility of the industry asset. This paper adopts heterogeneous panel and exploratory factor analysis methods, measuring industry risk by industry risk premium index, and constructs an industry MVS three dimensions vector factor model to analyze the factors consistent and affecting extent to industry risk. Furthermore, this paper analyzes simultaneously the linkage effect and working mechanism of multi-industries risks and gives an empirical research to determinants mechanism affecting industry risk.
Public Interest Statement
It is very important to identify deviation mechanism of price volatility of an industry asset and the affecting factors, and it is important to give the reasonable explanation and measurement to the abnormality of price volatility of the industry asset. This paper constructs an industry MVS three dimensions vector factor model to analyze above questions and gives an empirical research to determinants mechanism affecting industry risk. Investors can use industry MVS model to give empirical data analysis for industry risk. Investors can identify separately the affecting extent to every industry’s risk for macroeconomic dimension, industry value dimension and noise investor irrational sentiment dimension, and give comparison and analysis among multi industries for further investment decision. An investor can select industries with suitable risk levels and risk affecting factors according to his/her own preferred investment principle.
1. Introduction
The reasonable allocation among the resources of different industries, and the realization of cooperative developments and structure optimization among different industries, are useful to deepen and promote the industrial structure and to make it more effective. After a period of operation of an industry asset, its value maybe occur some changes. In normal condition, price volatility of the industry asset should keep the same pace with this value volatility of industry asset. But the real fact is that price volatility of the industry asset often greatly deviates from this value volatility, and this will lead to many abnormalities in the operation of financial market, such as January effect and over reaction (Banz, Citation1981; Basu, Citation1977; De Bondt & Thaler, Citation1985; Froot & Dabora, Citation1999; Mehra & Prescott, Citation1985). It is very important to identify this deviation mechanism of price volatility of an industry asset and the affecting factors causing this deviation. And it is also very important to give the reasonable explanation and measurement to the abnormality of price volatility of the industry asset.
Industry risk expresses price volatility of an industry asset, that is, the asset price is sometime up or sometime down. The range and frequency of up or down usually change in different time. The reason leading to this up or down range and frequency of industry asset price includes many factors. The abnormal price volatility of the industry asset can be accurately measured and explained only if the consistent system and affecting extent of these factors have been disclosed.
Many factors can affect the levels and volatility ranges of industry risk, among which the market environments the enterprises surviving in and macroeconomic conditions affect significantly. Alves (Citation2005) and Castrén and Kavonìus (Citation2009) analyze the relationship and dynamic character of industry risk and emphasize the cross-sectional dynamics among industries. They think macroeconomic variables significantly affect industry risk. Saldías (Citation2013a) finds that macroeconomic variables are common factors to induce strong cross-section dependence. The macroeconomic variables affecting industry risk include many sides, some of which can promote or restrain growth to all industries, whereas others can only promote or restrain growth to an industry or some industries. For example, Francis, Hasan, and Hunter (Citation2008) find that currency risk variable greatly affects the expected returns of all 36 US industries.
Industry value (also called industry fair value) can also affect levels and volatility ranges of industry risk, which is mainly measured by industry finance indexes. Industry profit gaining indexes reflect the ability of acquiring value growth in an industry. Industry debt repaying indexes reflect the ability of repaying debt with current asset. Industry operating indexes reflect the efficiency of managing and operating current asset in an industry. Hand and Green (Citation2011) find that annual accruals, annual change in earnings, and annual asset growth variables have effects on general long-run return. Fodor, Krieger, Mauck, and Stevenson (Citation2013) find that size and age variables are negatively related to the probability of extreme price movements.
The character of this paper is analyzing the factors consistent and affecting extent for many different industries risks from the industry angle. Besides above observed variables, there are other variables unobserved directly which also affect levels and variations of industry risk. Saldías (Citation2013b) finds that unobserved factors have effects on industry risk.
About unobserved variables affecting industry risk, this paper indicates by means of data analysis that noise investor irrational sentiment variables are the unobserved variables, which are significantly correlative with industry risk and are the decisive factors affecting industry risk. Ignoring the important influence of noise investor irrational sentiment variables will lead to incomplete industry risk analysis. Chou, Ho, and Ko (Citation2012) find that rational finance theory and behavioral finance theory must be considered together to explain industry returns.
Noise investor irrational sentiment is that because investors’ personal knowledge, asset investment experience, risk preference, psychology change, and others are different, they have cognition deviations and heterogeneous beliefs to asset pricing. These irrational investors who have cognition deviations are called noise traders (investors), and Black (Citation1986) first proposes concept of noise. These noise traders invest with rational traders together in capital market (Baker & Wurgler, Citation2006; De Long, Shleifer, Summers, & Waldmann, Citation1990; Shefrin & Statman, Citation1994). There are massive noise traders in the market, and their irrational investment behaviors make stock prices deviate rational intervals and create unusual market phenomenon that stock prices volatilize drastically and deviate from normal condition in capital market (Das, Markowitz, Scheid, & Statman, Citation2010; Dumas, Kurshev, & Uppal, Citation2009; Escobar, Ferrando, & Rubtsov, Citation2015; Illeditsch, Citation2011). Unlike macroeconomic variables and industry value variables which can be directly observed, noise investor irrational sentiment variables belong to psychology variables which are difficult to be observed directly and can be measured only by indirect ways. The number of opening an account found by Shiller (Citation2005) and trading volume found by Baker and Stein (Citation2004) can be chosen as indirect representation variables of noise investor irrational sentiment.
This paper adopts heterogeneous panel and exploratory factor analysis methods, measuring industry risk by industry risk premium index, and constructs an industry MVS three dimensions vector factor model to analyze the factors consistent and affecting extent to industry risk. Furthermore, this paper analyzes simultaneously the linkage effect and working mechanism of multi-industries risks and gives an empirical research to determinants mechanism affecting industry risk to test MVS three dimensions vector factor model.
2. MVS three dimensions vector factor model
2.1. Definition of industry risk premium
Assume that there are M industries in market and No. i industry includes kinds of enterprise stocks.
is time variable. The stock price at
period is
for No. J enterprise stock in No. i industry, and the stock price at last period is
. Its weighted coefficient in the industry is
. Industry risk premium is defined as industry stocks weighted return rate minus risk-free rate of return, and its mathematical equation is:
(1)
(1)
Industry risk premium in year (or other time period which is satisfied with the following assumptions) is defined as follows:
(2)
(2)
According to capital asset pricing model (CAPM), the return rate of an asset can be divided into risk-free rate of return (RF) and return rate produced by risk factors. This paper adopts industry risk premium (IRP) rejecting risk-free rate of return to analyze industry risk. The advantage is only considering return rate produced by risk factors, and in contrast to return rate computed by stock price, it can measure levels and volatility ranges of industry risk more accurately.
2.2. MVS three dimensions vector model
The factors affecting industry risk not only include observed macroeconomic variables and industry value variables, but also include unobserved noise investor irrational sentiment variables, that is, including macroeconomic dimension, industry value dimension, and noise investor irrational sentiment dimension simultaneously.
Order that X vector represents macroeconomic dimension variables, Y vector represents industry value dimension variables,
Z vector represents noise investor irrational sentiment dimension variables,
α represents constant vector; Β, Ψ, Θ represent coefficient vector; ε represents random error vector, ε~i.i.dN(0,
). Measure industry risk by volatility of IRP and construct MVS three dimensions vector model (M represents macroeconomic dimension; V represents industry value dimension; S represents noise investor irrational sentiment dimension):
(3)
(3)
That is(4)
(4)
M industries’ risks can be represented as follows:(5)
(5)
2.3. Implicit factor analysis and MVS three dimensions vector factor model
Because MVS model includes vectors of three dimensions and every dimension vector includes some variables, MVS model will estimate massive variable parameters, which will make the model lose massive degrees of free. The total estimation number of variable parameters equals to product of individual number and variable number in the model. Accompanying with increasing of individual number or variable number, the estimation number of parameters will increase in geometric level, which will make parameters estimation of the model be difficult even unable to solve.
In the case that many individuals and variables lead to lose massive degrees of free and be difficult to solve the model, the variables need to be reduced in dimensions. Because previous variables information of MVS three dimensions should be separately kept as far as possible to give a further explanation, this paper adopts exploratory factor analysis method to extract common implicit factors from MVS three dimensions, which will solve the question of variable parameters estimation of MVS model. The idea of exploratory factor analysis is first proposed by Spearman (Citation1904). Common factors are extracted separately from MVS three dimension, and υ represents mean vector while f represents factor vector. ɛ is the random error vector, ε~i.i.dN(0, δ2). Ϛ is the coefficient vector. I is the unit matrix.(6)
(6)
Equation (6) extracts factor vector separately from MVS three dimensions. Equation (7) is mathematical expression for factor vectors.(7)
(7)
Assume that:(8)
(8)
(9)
(9)
(10)
(10)
(11)
(11)
(6) is substituted into (4), then(12)
(12)
Order that:(13)
(13)
(14)
(14)
(15)
(15)
MVS model converts to MVS three dimensions vector factor model:(16)
(16)
Only after above mathematics testimony, it is feasible to give empirical analysis for industry risk.
3. Data and variables choosing
To test MVS three dimensions vector factor model affecting industry risk, this paper gives an empirical research and selects A shares data of China’s capital market from 2001 to 2015 to analyze. Because starting time of China’s capital market is late, data analysis indicates that noise investor irrational sentiment variables are significantly correlative to industry risk and obviously express the decisive factors affecting industry risk. This paper adopts Wind second industries classification standard totally including 23 industries, whose character is comprehensive reference to authoritative international industries classification standard GICS (Global Industries Classification Standard). The data include 360 panel data, among which there are 24 individuals, including industry average and 15 years data. Besides dependent variable ΔIRP, there are 16 independent variables. The data involve with 2,904 listed companies and use 24,600 original observations, and the source is from Wind information database.
The variables affecting ΔIRP include macroeconomic dimension, industry value dimension, and noise investor irrational sentiment dimension variables. In each dimension, a few representative variables are chosen to measure ΔIRP. Macroeconomic dimension includes five variables. Industry value dimension includes eight variables. Noise investor irrational sentiment dimension includes three variables. Data analysis results show that these variables are representative and can well explain and measure ΔIRP.
After choosing variables, MVS three dimensions vector model converts to the following form:(17)
(17)
ΔPCDIGR is per capita disposable income growth rate. ΔWMCI is weighted macroeconomic climate index. ΔCPI is consumer price index. ΔM2GR is growth rate of M2 supply of money and quasi money. ΔCGPI is weighted corporate goods price index. ΔROE is return of equity. ΔNPM is net profit margin. ΔTEGR is total expenses, including operating expenses, administrative expenses, and financial expenses divided by gross revenue. ΔTATR is total assets turnover ratio. ΔRLA is ratio of liabilities to assets. ΔTAGR is total assets growth rate. ΔORGR is operating revenue growth rate. ΔTPGR is total profit growth rate. ΔTR is turnover rate. ΔIAGR is investor account growth rate. ΔTVGR is trading volume growth rate.
In Equation (17), macroeconomic dimension includes front five independent variables, and noise investor irrational sentiment dimension includes last three independent variables, and industry value dimension includes middle eight independent variables.
3.1. Volatility of industry risk premium
Industry average ΔIRP mean is −0.978%, and the minimum is −192.02%, whereas the maximum is 166.4%. Absolute value of variation coefficient of ΔIRP is 80.1341. RF is weighted three months deposit rate. The biggest volatility of industry risk is Retailing industry, and its ΔIRP absolute value of variation coefficient is 1,989.8453. The smallest volatility of industry risk is Energy industry, and its ΔIRP absolute value of variation coefficient is 19.2367. Ten industries’ risk volatilities are higher than industry average level whereas other thirteen industries’ risk volatilities are lower than industry average level. Five industries’ ΔIRP means are positive, which are Media, Banks, Consumer Durables & Apparel, Technology Hardware & Equipment, Household & Personal Products, and other industries’ ΔIRP means are negative. Affected by financial crisis in 2008, all industries’ ΔIRP appear significantly decreasing, all becoming negative from positive last year. The biggest decreasing industry of ΔIRP is Diversified Financials, and the decreasing number is −275.85%. The smallest decreasing industry of ΔIRP is Software & Services, and the decreasing number is −148.23% (see Table ).
Table 1. Volatility of industry risk premium
3.2. M dimension variables choosing
Just as the content mentioned in Introduction, macroeconomic variables generally include money supply, price index, income, etc. This paper chooses volatilities of the following variables in M dimension: Per capita disposable income growth rate (ΔPCDIGR), Weighted macroeconomic climate index (ΔWMCI), Consumer price index (ΔCPI), Growth rate of M2 supply of money and quasi money (ΔM2GR), Weighted corporate goods price index (ΔCGPI). ΔPCDIGR mean is −0.0733%, and absolute value of variation coefficient is 39.8864. ΔWMCI mean is −0.2253, and absolute value of variation coefficient is 12.2658. ΔCPI mean is 0.0667, and absolute value of variation coefficient is 40.6255. ΔM2GR mean is 0.072%, and absolute value of variation coefficient is 64.1453. ΔCGPI mean is −0.3467, and absolute value of variation coefficient is 16.9183. Affected by financial crisis in 2008, ΔPCDIGR and ΔWMCI become negative from positive last year (see Table ).
Table 2. M dimension variables volatility
3.3. V dimension variables choosing
Volatilities of the following variables are chosen in V dimension: Return of equity (ΔROE), Net profit margin (ΔNPM), Total expenses including operating expenses, administrative expenses and financial expenses divided by gross revenue (ΔTEGR), Total assets turnover ratio (ΔTATR), Ratio of liabilities to assets (ΔRLA), Total assets growth rate (ΔTAGR), Operating revenue growth rate (ΔORGR), Total profit growth rate (ΔTPGR).
Industry average ΔROE mean is 0.2367%, and the minimum is −3.66%, whereas the maximum is 5.92%. Absolute value of variation coefficient of ΔROE is 10.0706. The biggest ΔROE industry is Technology Hardware & Equipment whereas the smallest ΔROE industry is Household & Personal Products. Seventeen industries’ ΔROE are higher than industry average level, whereas other six industries’ ΔROE are lower than industry average level. Industry average ΔNPM mean is 0.0527%, and the minimum is −7.13%, whereas the maximum is 3.55%. Absolute value of variation coefficient of ΔNPM is 56.0747. The biggest ΔNPM industry is Commercial and Special Services, whereas the smallest ΔNPM industry is Health Care Equipment & Services. Five industries’ ΔNPM are higher than industry average level whereas other eighteen industries’ ΔROE are lower than industry average level. Affected by financial crisis in 2008, except that the ability of profit gaining increases comparing with last year for Telecommunication Services and Health Care Equipment & Services industry, the ability of profit gaining decreases comparing with last year for other industries, among which the biggest decreasing industry is Semiconductors & Semiconductor Equipment whose ΔROE decreases −30.11% and ΔNPM decreases −23.63%.
Industry average ΔTEGR mean is −0.0993%, and the minimum is −7.98%, whereas the maximum is 7.42%. Absolute value of variation coefficient of ΔTEGR is 32.9686. The biggest ΔTEGR industry is Commercial and Special Services, whereas the smallest ΔTEGR industry is Consumer Services. Two industries’ ΔTEGR are higher than industry average level, whereas other twenty-one industries’ ΔTEGR are lower than industry average level. Industry average ΔTATR mean is 0.0073 times, and the minimum is −0.05 times, whereas the maximum is 0.07 times. Absolute value of variation coefficient of ΔTATR is 6.1949. The biggest ΔTATR industry is Real Estate, whereas the smallest ΔTATR industry is Media. Eighteen industries’ ΔTATR are higher than industry average level, whereas other five industries’ ΔTATR are lower than industry average level.
Industry average ΔRLA mean is 0.2513%, and the minimum is −5.69%, whereas the maximum is 9.15%. Absolute value of variation coefficient of ΔRLA is 13.7941. The biggest ΔRLA industry is Capital Goods, whereas the smallest ΔRLA industry is Real Estate. Nine industries’ ΔRLA are higher than industry average level, whereas other fourteen industries’ ΔRLA are lower than industry average level. Industry average ΔTAGR mean is −4.4073%, and the minimum is −512.88%, whereas the maximum is 511.01%. Absolute value of variation coefficient of ΔTAGR is 44.6629. The biggest ΔTAGR industry is Telecommunication Services whereas the smallest ΔTAGR industry is Energy. Six industries’ ΔTAGR are higher than industry average level, whereas other seventeen industries’ ΔTAGR are lower than industry average level.
Industry average ΔORGR mean is −7.8667%, and the minimum is −603.77%, whereas the maximum is 614.25%. Absolute value of variation coefficient of ΔORGR is 30.1287. The biggest ΔORGR industry is Telecommunication Services, whereas the smallest ΔORGR industry is Food & Staples Retailing. Seven industries’ ΔORGR are higher than industry average level, whereas other sixteen industries’ ΔORGR are lower than industry average level. Industry average ΔTPGR mean is −1.042%, and the minimum is −529.79%, whereas the maximum is 593.4%. Absolute value of variation coefficient of ΔTPGR is 248.8826. The biggest ΔTPGR industry is Utilities, whereas the smallest ΔTPGR industry is Health Care Equipment & Services. Four industries’ ΔTPGR are higher than industry average level, whereas other nineteen industries’ ΔTPGR are lower than industry average level (see Table ).
Table 3. V Dimension Variables Volatility (ΔROE&ΔNPM)
3.4. S dimension variables choosing
Volatilities of the following variables are chosen in S dimension: Turnover rate (ΔTR), Investor account growth rate (ΔIAGR), Trading volume growth rate (ΔTVGR). Industry average ΔTR mean is 0.0613%, and the minimum is −1.16%, whereas the maximum is 1.38%. Absolute value of variation coefficient of ΔTR is 13.644. The biggest ΔTR industry is Utilities whose absolute value of variation coefficient of ΔTR is 1893.4192. The smallest ΔTR industry is Software & Services whose absolute value of variation coefficient of ΔTR is 4.8201. Fourteen industries’ ΔTR are higher than industry average level, whereas other nine industries’ ΔTR are lower than industry average level. Except that ΔTR means are negative for Telecommunication Services, Energy, Transportation and Utilities industry, ΔTR means of other nineteen industries are positive, which indicate that most industries totally exist common heterogeneous beliefs.
Industry average ΔIAGR mean is 0.924%, and the minimum is −114.5%, whereas the maximum is 92.92%. Absolute value of variation coefficient of ΔIAGR is 51.9466. The biggest ΔIAGR industry is Telecommunication Services whose absolute value of variation coefficient of ΔIAGR is 282.3683. The smallest ΔIAGR industry is Semiconductors & Semiconductor Equipment whose absolute value of variation coefficient of ΔIAGR is 5.0568. Five industries’ ΔIAGR are higher than industry average level, whereas other eighteen industries’ ΔIAGR are lower than industry average level. All industries’ ΔIAGR are positive in 2007, and because of influence of financial crisis in 2008, investors are generally pessimistic to future market expectation. Except that ΔIAGR is still positive for Health Care Equipment & Services industry, ΔIAGR of all the other industries greatly decrease becoming negative, among which the biggest decreasing industry is Energy, whose ΔIAGR becomes −225.02% from 234.92% last year, whereas the smallest decreasing industry is Diversified Financials whose ΔIAGR becomes −2.31% from 90.98% last year.
Industry average ΔTVGR mean is 4.812%, and the minimum is −149.15%, whereas the maximum is 142.3%. Absolute value of variation coefficient of ΔTVGR is 19.2198. The biggest ΔTVGR industry is Health Care Equipment & Services whose absolute value of variation coefficient of ΔTVGR is 383.7074. The smallest ΔTVGR industry is Software & Services whose absolute value of variation coefficient of ΔTVGR is 11.9629. Fifteen industries’ ΔTVGR are higher than industry average level, whereas other eight industries’ ΔTVGR are lower than industry average level (see Table ).
Table 4. S Dimension Variables Volatility (ΔTR&ΔIAGR)
4. MVS three dimensions implicit factor analysis
In this paper, MVS model includes 24 individuals and 16 independent variables and is unable to directly estimate variable parameters. According to selected variables of MVS three dimensions, common factors are extracted separately from MVS three dimensions.
4.1. M Dimension Common Factors Extracted from Variables
M dimension includes five variables: Per capita disposable income growth rate (ΔPCDIGR), Weighted macro-economic climate index (ΔWMCI), Consumer price index (ΔCPI), Growth rate of M2 supply of money and quasi money (ΔM2GR), Weighted corporate goods price index (ΔCGPI). Bartlett’s Test of Sphericity indicates that five variables are strongly correlative and Table is correlation matrix.
Table 5. Correlation matrix of M dimension variables
One common factor whose eigenvalue is greater than one is extracted from correlation matrix, which explains 73.6% of variance information. Conclusion can be made from loads matrix that this common factor and variables are strongly correlative, and this common factor can be explained as macroeconomic climate factor (see Table ).
Table 6. Component matrix of M dimension variables
4.2. V dimension common factors extracted from variables
V dimension includes eight variables: Return of equity (ΔROE), Net profit margin (ΔNPM), Total expenses including operating expenses, administrative expenses, and financial expenses divided by gross revenue (ΔTEGR), Total assets turnover ratio (ΔTATR), Ratio of liabilities to assets (ΔRLA), Total assets growth rate (ΔTAGR), Operating revenue growth rate (ΔORGR), Total profit growth rate (ΔTPGR).
Bartlett’s Test of Sphericity indicates that eight variables are strongly correlative. Three common factors whose eigenvalues are greater than one are extracted from correlation matrix, which explain 74.45% of variance information. Table is loads matrix between three common factors and variables. The first common factor can be explained as industry scale increasing factor . The second common factor can be explained as industry cost debt factor
. The third common factor can be explained as industry profit gaining and fluidity factor
.
Table 7. Component matrix of V dimension variables
4.3. S Dimension Common Factors Extracted from Variables
S dimension is noise investor irrational sentiment dimension, including three variables: Turnover rate (ΔTR), Investor account growth rate (ΔIAGR), and Trading volume growth rate (ΔTVGR).
Bartlett’s Test of Sphericity indicates that three variables are strongly correlative. Two common factors which can’t be observed directly and whose eigenvalues are greater than one are extracted from correlation matrix, which explain 96.55% of variance information of noise investor irrational sentiment. Table is loads matrix between two common factors and variables. The first common factor can be explained as expectation confidence factor . The second common factor can be explained as heterogeneous beliefs factor
.
Table 8. Component matrix of S dimension variables
All the absolute values of Spearman correlation coefficients for MVS three dimensions factors are no more than 0.36.
5. Heterogeneous panel data analysis of MVS three dimensions vector factor model
According to MVS three dimensions vector factor model after extracting common factors, estimation is made to variable parameters of this heterogeneous panel. The advantages of using heterogeneous panel analysis are considering simultaneously linkage of cross-sectional data and time data and solving the question of omitted variables. Because different industries’ ΔIRP are different, this kind of difference can be found through heterogeneous panel analysis. Figure is time tendency figure of different industries’ ΔIRP. ΔIRP means of most industries volatilize in [−200, 200] interval around zero, which indicate that most industries’ ΔIRP keep generally stable tendency from 2001 to 2015. Affected by financial crisis in 2008, all industries’ ΔIRP express significantly decreasing, among which the biggest decreasing industry is No.18 Diversified Financials. Because China’s government adopts economy stimulating plan in the later, these industries’ ΔIRP express rapidly increasing.
Random-coefficients regression of MVS three dimensions vector factor model indicates that P value of the model approximately equals to zero and total regression model is statistically significant. Test of parameter constancy indicates that P value approximately equals to zero and the model is varying coefficients panel model. Changes of MVS three dimensions variable factor lead to the difference of every industry’s ΔIRP and different sensitivity of every industry risk to MVS three dimensions variable factor. Total regression model indicates that ΔIRP is significantly correlative to macroeconomic climate factor and expectation confidence factor
of noise investor irrational sentiment and that macroeconomic condition and noise investor irrational sentiment have intense influences on industry risk. Total regression model also indicates that ΔIRP isn’t significantly correlative to industry scale increasing factor
, cost debt factor
and profit gaining and fluidity factor
and that industry value has a weak influence on industry risk. But for some industries, such as Software & Services, Diversified Financials, Banks and Retailing, ΔIRP is significantly correlative to industry scale increasing factor
, cost debt factor
or profit gaining and fluidity factor
, which indicates that these industries values have intense influences on industry risk. Furthermore, ΔIRP isn’t significantly correlative to heterogeneous beliefs factor
. But for some industries such as Transportation, Commercial and Special Services and Banks, ΔIRP is significantly correlative to heterogeneous beliefs factor
, which indicates that the influence of this factor to industry risk is weak (see Table ).
Table 9. Random-coefficients regression of MVS three dimensions vector factor model
On industry average level, ΔIRP is significantly correlative to macroeconomic climate factor , industry scale increasing factor
, profit gaining and fluidity factor
and expectation confidence factor
of noise investor irrational sentiment, which indicates that variable factors of macroeconomic dimension, industry value dimension and noise investor irrational sentiment dimension simultaneously have significant influences on industry average risk (see Table ).
Table 10. Random-coefficients regression on industry average level
Changes of macroeconomic dimension variable factors have influences on demand and supply of industries leading to changes of ΔIRP, and the influences generally affect most industries’ risks. The model indicates that ΔIRP is significantly correlative to macroeconomic climate factor and changes of
will make ΔIRP change. Changes of
usually lead to changes of ΔIRP in the same direction and risk comovements of different industries. In the stable growth stage of the economy, the returns of most industries also express tendency of stable growth and industry risk is commonly low. In the drastic declining stage of the economy, the returns of most industries also express declining tendency and industry risk is commonly high. This point is indicated by that industry average ΔIRP and all industries’ ΔIRP are negative correlative to macroeconomic climate factor
(see Table ). Some common factors can lead to the risk comovements of different industries (Chiu, Peña, & Wang, Citation2015; Chou et al., Citation2012).
Table 11. Coefficients of MVS variable factors
Changes of will make demand and supply of different industries express volatility of different ranges and produce different influences on these industries’ risks. To industries with different products demand elasticity, supply elasticity or income elasticity, changes of
will produce different ΔIRP influences. Schwartz and Altman (Citation1973) find that sensitivity differentials can cause price volatility differentials among different industries. The model indicates that the smallest influence industry of
changes to ΔIRP is Food & Staples Retailing, because this industry belongs to necessaries producing industry and its product demand elasticity is low. The biggest influence industry of
changes to ΔIRP is Consumer Durables & Apparel, because product demand elasticity of this industry is high and changes of
will lead to greater ΔIRP changes.
Changes of industry value dimension variable factors can also lead to ΔIRP changes of different ranges, and this kind of value changes mainly expresses in industry profit gaining ability, debt repaying ability and operating ability, etc. The biggest influence industry of industry scale increasing factor changes to ΔIRP is Food, Beverage & Tobacco, and the smallest influence industry is Utilities. The biggest influence industry of industry cost debt factor
changes to ΔIRP is Retailing, and the smallest influence industry is Commercial and Special Services. The biggest influence industry of industry profit gaining and fluidity factor
changes to ΔIRP is Transportation, and the smallest influence industry is Food, Beverage & Tobacco.
Changes of noise investor irrational sentiment dimension variable factors also can obviously affect changing ranges of ΔIRP and the model indicates that expectation confidence factor is significantly correlative to ΔIRP and changes of
will make ΔIRP change. There are massive noise traders in the market who have cognition deviations and heterogeneous beliefs to asset pricing and bring irrational investment behaviors, which make stock prices deviate rational intervals and create unusual market phenomenon that stock prices volatilize drastically and deviate from normal condition in capital market. This point is indicated by that industry average
and all industries’
are significantly positive correlative to ΔIRP, which means that changes of noise investor irrational sentiment will lead to industry risk volatility of great ranges.
Comparing with macroeconomic dimension and industry value dimension variable factors, changes of noise investor irrational sentiment dimension will lead to greater changes of ΔIRP, which indicate that noise investor irrational sentiment is decisive factor affecting industry risk. Industry average
coefficient is 176.67, which is obviously higher than macroeconomic dimension and industry value dimension factor coefficient. Except that
coefficient of Banks industry is 85.72,
coefficients of all the other industries volatilize in [107.25, 231.8] interval. The biggest
coefficient industry is Energy, which indicates that changes of noise investor irrational sentiment have the biggest influence on this industry’s ΔIRP. Comparing with the first common factor
, the second common factor
‘s influence is weaker. The smallest influence industry of this factor is Diversified Financials, and the biggest influence industry is Transportation.
6. Conclusions
Many factors can affect the levels and volatility ranges of industry risk. This paper constructs an industry MVS three dimensions vector factor model to analyze the factors consistent and affecting extent to industry risk. Furthermore, this paper analyzes simultaneously the linkage effect and working mechanism of multi-industries risks and gives an empirical research to determinants mechanism affecting industry risk.
Changes of macroeconomic variables have an important influence on industry risk. Changes of macroeconomic variables can lead to risk comovements of different industries. In the stable growth stage of the economy, the returns of most industries also express tendency of stable growth and industry risk is commonly low. In the drastic declining stage of the economy, the returns of most industries also express declining tendency and industry risk is commonly high. Macroeconomic variables can affect differently to industries with different products demand elasticity, supply elasticity, or income elasticity. Industry value variables can also affect change ranges of industry risk, and this kind of value changes mainly expresses in industry profit gaining ability, debt repaying ability, and operating ability, etc.
Data analysis indicates that noise investor irrational sentiment variables are significantly correlative to industry risk volatility. Noise investor irrational sentiment is that because investors’ personal knowledge, asset investment experience, risk preference, psychology change and others are different, they have cognition deviations and heterogeneous beliefs to asset pricing. Comparing with macroeconomic variables and industry value variables, noise investor irrational sentiment variables will lead to greater influences on industry risk, which are decisive factors affecting industry risk.
When investors give an analysis of industry risk for investment allocation, it is necessary for them to know the detailed factors affecting industry risk and the affecting extent. Using industry MVS three dimensions vector factor model, investors can give empirical data analysis for industry risk. Investors can identify separately the affecting extent to every industry’s risk for macroeconomic dimension, industry value dimension and noise investor irrational sentiment dimension, and give comparison and analysis among multi industries for further investment decision. An investor can select industries with suitable risk levels and risk affecting factors according to his/her own preferred investment principle. For example, a risk aversion investor will probably select industries with bigger affecting extent of macroeconomic factors or industry value factors.
Additional information
Funding
Notes on contributors
Feng Sun
Feng Sun is Doctor of Finance Engineering Department, Donlinks School of Economics and Management, University of Science and Technology Beijing. Professor Cheng Liu is Director of Finance Engineering Department, Donlinks School of Economics and Management, University of Science and Technology Beijing. He has abundant theory and practice experiences in finance field for many years. Xiaoguang Zhou is Associate Professor of Finance Engineering Department, Donlinks School of Economics and Management, University of Science and Technology Beijing. He is mainly good at behavior finance field.
References
- Alves, I. (2005). Investigating the relationship between the financial and real economy. Bank for International Settlements, 22, 450–480. https://doi.org/10.2139/ssrn.1188602
- Baker, M., & Stein, J. C. (2004). Market liquidity as a sentiment indicator. Journal of Financial Markets, 7, 271–299.10.1016/j.finmar.2003.11.005
- Baker, M., & Wurgler, J. (2006). Investor sentiment and the cross-section of stock returns. The Journal of Finance, 61, 1645–1680.10.1111/j.1540-6261.2006.00885.x
- Banz, R. W. (1981). The relationship between return and market value of common stocks. Journal of Financial Economics, 9, 3–18.10.1016/0304-405X(81)90018-0
- Basu, S. (1977). Investment performance of common stocks in relation to their price-earnings ratios: A test of the efficient market hypothesis. The Journal of Finance, 32, 663–682.10.1111/j.1540-6261.1977.tb01979.x
- Black, F. (1986). Noise. The Journal of Finance, 41, 528–543.10.1111/j.1540-6261.1986.tb04513.x
- Castrén, O., & Kavonìus, I. K. (2009). Balance sheet interlinkages and macro-financial risk analysis in the Euro area. Frankfurt: European Central Bank.
- Chiu, W.-C., Peña, J. I., & Wang, C.-W. (2015). Industry characteristics and financial risk contagion. Journal of Banking & Finance, 50, 411–427.10.1016/j.jbankfin.2014.04.003
- Chou, P.-H., Ho, P.-H., & Ko, K.-C. (2012). Do industries matter in explaining stock returns and asset-pricing anomalies? Journal of Banking & Finance, 36, 355–370.10.1016/j.jbankfin.2011.07.016
- Das, S., Markowitz, H., Scheid, J., & Statman, M. (2010). Portfolio optimization with mental accounts. Journal of Financial and Quantitative Analysis, 45, 311–334.10.1017/S0022109010000141
- De Bondt, W. F. M., & Thaler, R. (1985). Does the stock market overreact? The Journal of Finance, 40, 793–805.10.1111/j.1540-6261.1985.tb05004.x
- De Long, J. B., Shleifer, A., Summers, L. H., & Waldmann, R. (1990). Noise trader risk in financial markets. Journal of Political Economy, 98, 703–738.10.1086/261703
- Dumas, B., Kurshev, A., & Uppal, R. (2009). Equilibrium portfolio strategies in the presence of sentiment risk and excess volatility. The Journal of Finance, 64, 579–629.10.1111/j.1540-6261.2009.01444.x
- Escobar, M., Ferrando, S., & Rubtsov, A. (2015). Robust portfolio choice with derivative trading under stochastic volatility. Journal of Banking & Finance, 61, 142–157.10.1016/j.jbankfin.2015.08.033
- Fodor, A., Krieger, K., Mauck, N., & Stevenson, G. (2013). Predicting extreme returns and portfolio management implications. Journal of Financial Research, 36, 471–492.10.1111/jfir.v36.4
- Francis, B. B., Hasan, I., & Hunter, D. M. (2008). Can hedging tell the full story? Reconciling differences in United States aggregate-and industry-level exchange rate risk premium. Journal of Financial Economics, 90, 169–196.10.1016/j.jfineco.2007.10.007
- Froot, K. A., & Dabora, E. M. (1999). How are stock prices affected by the location of trade? Journal of Financial Economics, 53, 189–216.10.1016/S0304-405X(99)00020-3
- Hand, J. R. M., & Green, J. (2011). The importance of accounting information in portfolio optimization. Journal of Accounting, Auditing & Finance, 26(1), 1–34.10.1177/0148558X11400577
- Illeditsch, P. K. (2011). Ambiguous information, portfolio inertia, and excess volatility. The Journal of Finance, 5, 2213–2247. doi:10.1111/j.1540-6261.2011.01693.x
- Mehra, R., & Prescott, E. C. (1985). The equity premium- A puzzle. Journal of Monetary Economics, 15, 145–161.10.1016/0304-3932(85)90061-3
- Saldías, M. (2013a). Systemic risk analysis using forward-looking distance-to-default series. Journal of Financial Stability, 9, 498–517.10.1016/j.jfs.2013.07.003
- Saldías, M. (2013b). A market-based approach to sector risk determinants and transmission in the euro area. Journal of Banking & Finance, 37, 4534–4555.10.1016/j.jbankfin.2013.01.026
- Schwartz, Robert A., & Altman, Edward I. (1973). Volatility behavior of industrial stock price indices. The Journal of Finance, 28, 957–971.10.1111/j.1540-6261.1973.tb01418.x
- Shefrin, H., & Statman, M. (1994). Behavioral capital asset pricing theory. The Journal of Financial and Quantitative Analysis, 29, 323–349.10.2307/2331334
- Shiller, R. J. (2005). Behavior economics and institutional innovation. Journal of Southern Economic, 72, 269–283. doi:10.2307/20062111
- Spearman, C. (1904). ′General intelligence′ objectively determined and measured. The American Journal of Psychology, 15, 201–293.10.2307/1412107