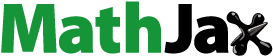
Abstract
In this paper, we develop and examine a simple interactive agent‐based model, where the distribution of returns generated from the model takes into account two stylized facts about financial markets: fat tails and volatility clustering. Our results indicate that the risk tolerance of fundamentalists and the relative funding rate of positive‐feedback traders versus fundamentalists are key factors determining the path of price fluctuations. Fundamentalists are more able to dominate the market when they are more willing than positive‐feedback traders to take risks. In addition, more crises occur as positive‐feedback traders face higher funding costs compared to fundamentalists. Our model suggests that fundamentalists cause heavier tails, and positive‐feedback traders cause the formation of speculative bubbles. Our model also indicates that traders’ attitudes toward risk vary across time and market. The generally low level of risk bearing by fundamentalists could explain the frequent occurrence of bubbles.
Public Interest Statement
Why do we observe ups and downs in stock markets? Does there exist a model to explain price fluctuations in financial markets? To answer these complicate questions, we propose a simple model but also rich enough to explain price movements in financial markets. We investigate various potential factors which could have impact affecting the path of price fluctuations. We also propose a new method to estimate key parameters in our model. Our simulations and empirical results indicate the importance of risk‐taking attitudes from fundamentalists in the market. As fundamentalists are more willing to take risks, the observed asset prices would be more close to their true values. Meanwhile, we would observe less speculative bubbles occurred in the market.
1. Introduction
Economists have investigated if rare events could explain the equity premium puzzle originally identified by Mehra and Prescott (Citation1985).Footnote1 De Long, Shleifer, and Summers (Citation1990a) proposed that the existence of noise traders could lead to a divergence between market prices and fundamental values such that the equity premium could be explained by noise‐trader risk. Shleifer and Summers (Citation1990) explained that the limits of arbitrage and movements in investor sentiment are important determinants of price movements. Frankel and Froot (Citation1990, Citation1991) showed that investors have heterogeneous expectations and shift from fundamentalists to chartists (technicians), potentially influencing price movements. Heterogeneous agent models which generate stylized facts of financial returns (unpredictability, fat tails, and volatility clustering) have been introduced and developed by several researchers.Footnote2 Several studies since 2005 have focused on the estimation of the parameters of agent‐based models, comparing estimated findings to that of observed historical data.Footnote3
In this paper, we extend the models of Frankel and Froot (Citation1990, Citation1991) and Kaizoji (Citation2002, Citation2004), and examine the simple interactive agent‐based modelFootnote4 in which the distribution of returns has fat tails and volatility clustering. The heterogeneous agent model of stock markets proposed by Kaizoji (Citation2002, Citation2004) predicts complicated endogenous price fluctuations leading to return distributions that are characterized by fat tails. In contrast to the model proposed by Kaizoji (Citation2004), fundamentalists in our model are able to survive in the market with the existence of noise traders. Moreover, we are able to simulate the scenarios of speculative bubbles and crashes. We examine the consistency of our model under shocks with different distributions, and search for key parameters which determine the path of price fluctuations. We then use historical data to estimate the key parameters of our model.
Our analysis is in line with those reported by Greenwood and Shleifer (Citation2013) and Edelen, Marcus, and Tehranian (Citation2010) who show that the relative risk tolerance between fundamentalists and positive‐feedback traders determines the path of price fluctuations. Instead of emphasizing intensity of choice to switch strategies which creates chaotic behaviors of price fluctuation, we focus on the impact of the risk tolerance of fundamentalists and the relative funding rate of positive‐feedback traders versus fundamentalists on the path of price fluctuations. We introduce Shannon entropyFootnote5 as a measure to investigate the differences of behaviors between fundamentalists and positive‐feedback traders. Kirchler and Huber (Citation2007) argued that heterogeneous fundamental information is the source of fat tails. Our results indicate that fundamentalists could be the source of heavier tails, and positive-feedback traders would cause the formation of speculative bubbles. We then propose a novel approach to estimate key parameters in our model. We find that traders’ attitudes toward risk may vary across time and markets, and the generally low level of risk bearing by fundamentalists could explain the frequent occurrence of bubbles.
The remainder of the paper is organized as follows. Section 2 reviews some more literature. Section 3 presents the interactive agent‐based model. Section 4 introduces the methodology for searching and estimating key parameters. Section 5 discusses the simulation and estimation results. Section 6 provides some more discussions. Section 7 concludes.
2. Related literature
Blanchard and Watson (Citation1982) defined rational bubbles as the difference between stock prices and the cumulative discounted value of future dividends. In contrast to rational bubbles, speculative bubbles are caused by amplification mechanismsFootnote6 in which rational speculators jump on the bandwagon, buying stock ahead of positive-feedback traders, and expecting to sell at a higher price later (De Long et al., Citation1990a). Well‐known speculative bubbles include the Dutch Tulip Mania of the 1630s, the Mississippi Bubble and South Sea Bubble of the 1720s, the Roaring Twenties of 1920s, the Dot‐com Bubble in 2000, and most recently the speculative real‐estate bubble in 2007.
Several studies have sought to identify the causes of speculative bubbles and the roles the different types of traders play in the financial market. De Long, Shleifer, Summers, and Waldmann (Citation1990b) claimed that the existence of rational speculators with positive‐feedback traders in the market would destabilize asset prices. Abreu and Brunnermeier (Citation2003) provided explanations in which an asset bubble persists with the presence of rational arbitrageurs. Brunnermeier and Nagel (Citation2004) found that riding a bubble can be the optimal strategy for sophisticated traders. Griffin, Harris, Shu, and Topaloglu (Citation2011) suggested that institutional investors drove and burst the 1997–2000 hi‐tech bubble. If fundamentalists are more responsive to price changes, Greenwood and Shleifer (Citation2013) found that the forces of these market participants would push prices back to near-fundamental levels.
Some research has focused on how investor sentiment could affect the financial market. Qiu and Welch (Citation2004) shared the same opinion that sentiment plays a role in the financial market. While consumer confidence qualifies as a proxy for investor sentiment, closed‐end fund discounts may be the wrong measure of sentiment. Baker and Wurgler (Citation2007) measured investor sentiment in the market and developed an index of sentiment levels. They argued that the wave of investor sentiment impacts individual firms and the entire stock market. Edelen et al. (Citation2010) showed that the sentiment of retail investors relative to that of institutional investors is a primary driver of equity valuations beyond that which can be explained by fundamentals.
3. Model
To extend the models of Kaizoji (Citation2004) and Frankel and Froot (Citation1991), we assume that there is a single stock in the market that is populated with three types of traders: fundamentalists, positive‐feedback traders, and noise traders.Footnote7 All traders in the market are myopic and possess beliefs on next period’s price for the stock. In contrast to the infinitely lived traders who maximize their expected utilities in Barberis, Greenwood, Jin, and Shleifer (Citation2015), traders in our model are short‐sighted, only maximizing their expected utilities for next period. Traders (both fundamentalists and positive‐feedback traders) maximize their utilities according to their beliefs. The expected utility for the next period is a function of profits, wealth, and costs, and the utility function is increasing and concave with respect to the demand for the stock. Traders are rationally bounded by identifying their own specific utility function: they are only able to observe and sum up marginal utilities of profits, wealth, and costs as a criteria for their utilities intuitively. In addition, there is a banking system to finance traders’ transactions. When traders do not trade, their next period’s expected utility is zero. An analysis of expected utility functions for fundamentalists and positive‐feedback traders is provided in Appendix A.
3.1. Noise traders
Different definitions of noise traders were introduced in several studies.Footnote8 We refer to noise traders as the aggregation of liquidity traders (Glosten & Milgrom, Citation1985; Kyle, Citation1985), traders with biased belief or sentiments (De Long et al., Citation1990a), and also the shock terms. The noise traders in Bloomfield et al. (Citation2009) are more like the positive‐feedback traders in our model.Footnote9
The accumulated noise traders’ demand for stock is as follows:
(1)
(1)
(2)
(2)
where γ denotes the strength of noise traders’ reaction to noisy information and ɛ1, t is related to the demand for the stock from liquidity traders and traders with biased belief or sentiments. In real‐world markets, liquidity traders tend to sell stocks for smoothing their consumptions, whereas traders with biased belief or sentiments tend to buy stocks when the market is agitated. Therefore, based on the law of large numbers and the central limit theorem, we assume that ɛ1, t is normally distributed. ɛ2, t represents the shock terms resulting from changes of policies and rare events. The changes of policies could arise as a result of unexpected dividend announcements, tight monetary policies, or restrictions on exports and imports; rare events could be the occurrence of severe snow storms, volcanic eruptions, earthquakes, or terrorist attacks. We allow the shock term ɛ2, t to vary with different probability distributions. ɛ2,t is public information to all traders in the market, but only fundamentalists and noise traders take the impacts of ɛ2, t into account. The accumulated noise traders’ demand for stock is positive when ɛt > 0, and
is negative when ɛt < 0.
3.2. Traders’ adaptive processes
Fundamentalists’ and positive‐feedback traders’ adaptive processes are as follows. The adaptive process for a fundamentalist can be written as(3)
(3)
where p* denotes the initial fundamental value of the stock. The fundamental value here is a martingale, so the best prediction for next period’s fundamental value is the current observed fundamental value.Footnote10 Fundamentalists believe that the market price move toward their fundamental value.Footnote11 The parameter ν captures the speed at which fundamentalists expect the market price to move back to fundamental value. In contrast to Kaizoji (Citation2004) who sets fundamental value as a constant across time, we assume that fundamentalists are able to observe information about policy changes and rare events but are not able to receive information regarding liquidity traders’ demand and noise traders’ biased beliefs and sentiments in the market. Thus, the fundamentalists correct their fundamental value each period by the shock term ɛ2, t.
For a positive‐feedback trader, the adaptive process is(4)
(4)
where μ is the error correction coefficient which represents how sensitive positive‐feedback traders are in correcting their expected stock price for the next period.
Both fundamentalists’ and positive‐feedback traders’ adaptive processes are similar to a first‐order autoregressive process AR(1), except that fundamentalists adjust their belief by the differences between the fundamental value and the current stock price pt while positive‐feedback traders adjust their belief by the differences between the last period expected price
and the current stock price pt.
3.3. Fundamentalists
Fundamentalists buy stocks when prices are below their expected price and sell stocks when prices exceed their expected price. They form their expected price based on the differences between fundamental value and current market price, and adjust their expected price each period. Fundamentalists maximize their expected utility for the next period as follows:(5)
(5)
where αf is the parameter indicating the eagerness of fundamentalists toward profitsFootnote12, and rf is the funding rate fundamentalists face for financing their positions. The first term of the expected utility function represents the expected profits realized in the next period and the second term is related to the costs which fundamentalists must pay for their transactions.Footnote13 One can view fundamentalists as sophisticated traders (institutional investors) who care only about their performance. Consequently, there is no wealth effect on the fundamentalists’ expected utility function.
The fundamentalists’ demand for stock can be obtained by solving Equation (5):
(6)
(6)
We can see that is positive when fundamentalists expect stock prices to go up for certain,
, and
is negative when they anticipate a decline in the stock price,
. Moreover, the expected utility for the next period
.
3.4. Positive‐feedback traders
According to De Long et al. (Citation1990a), positive‐feedback traders chase market trends, buying stocks when they anticipate price appreciation for the next period and selling stocks when expecting price depreciation. They adjust their forecasts based on the difference between the predicted price of the market trend and the current market price, correcting their prediction each period.
To see how positive‐feedback traders maximize their expected utility for the next period, we discuss two cases. In Case 1, we assume that the wealth effect is linear, meaning that utility does not diminish as wealth increases. Case 2 considers the diminishing gains of utility from increases in wealth, where we assume that the wealth effect exhibits constant relative risk aversion (CRRA).Footnote14
3.4.1. Case 1
In Case 1, positive‐feedback traders maximize their expected utility for the next period as follows.(7)
(7)
where αc is the parameter showing positive‐feedback traders’ eagerness toward profits, β is the coefficient of the wealth effect, and rc is the funding rate positive‐feedback traders pay for their transactions. The first term of the expected utility function given by Equation (Equation7(7)
(7) ) is the expected profits realized in the next period, the second term is related to the wealth effect, and the third term indicates the costs positive‐feedback traders face for their transactions. One can view positive‐feedback traders as individual traders (retail investors) where the wealth effect plays a role in their decision-making.Footnote15
Solving Equation (7), we have the positive‐feedback traders’ demand for stock in Case 1:
(8)
(8)
We can see from Equation (8) that is positive when positive‐feedback traders expect the price to increase and the wealth effect can compensate for the transaction costs,
;
is negative when positive‐feedback traders anticipate price depreciation,
. The expected utility for the next period
.
3.4.2. Case 2
In Case 2, positive‐feedback traders maximize their expected utility for the next period as follows:(9)
(9)
Similar to Case 1, the first term of the expected utility function given by Equation (9) is the expected profits realized in the next period, the second term is the wealth effect, and the third term shows the transaction costs.
Solving Equation (9), we have the positive‐feedback traders’ demand for stock in Case 2:
(10)
(10)
We require next period expected returns of positive‐feedback traders equal to as the regularity condition.Footnote16
is positive when
, and
is negative when
. We can see that as β increases, positive‐feedback traders trade more cautiously. Both Case 1 and Case 2 share similar properties. From now on, we will focus on the results for Case 1 and discuss Case 2 in detail in Section 6.
3.5. Market maker
Similar to the portfolio manager in Frankel and Froot (Citation1991), we assume that there exists a market maker whose role is to announce an execution price and to execute transactions. Let us assume the supply of stock in the market is fixed, so the stock prices are driven by traders’ demand for stock in the market. The market price each period is determined by the following process:(11)
(11)
where θ represents the market sensitivity by market makers in responding to changes in the demand for stocks, n is the total number of traders in the market, and κt is the proportion of positive‐feedback traders in the market at each period. We also assumed that noise traders exist in the market in a fixed weight ξ.Footnote17 The population fraction of fundamentalists in the market is equal to 1 − κt − ξ.
The market maker adjusts the price according to the orders received at the beginning of each period. There is a transaction cost associated with engaging a market maker to execute the orders. The rents that the market maker gains is related to the current stock price pt.Footnote18
3.6. Strategy switching
Several studies have investigated strategy switching between different types of traders.Footnote19 In Lux and Marchesi (Citation2000), strategy switching plays an important role resulting in asset returns with heavy tails and volatility clustering. Furthermore, Boswijk et al. (Citation2007) and de Jong et al. (Citation2010) demonstrate the regime shift of trading strategies using observed market prices.
The population fractions of fundamentalists and positive‐feedback traders are updated each period as follows:(12)
(12)
where φ is the intensity of choice to switch strategies, and φ > 0. Higher values of φ represent the situations in which agents switch more rapidly to a best‐performing strategy, whereas lower values of φ indicate agents are reluctant to switch strategy unless they observe large differences in the profits made between the strategies. are the cumulative profits realized by fundamentalists and positive‐feedback traders, respectively, each period. In contrast to Kaizoji (Citation2004),Footnote20 in our model, fundamentalists and positive‐feedback traders switch strategies based on the cumulative trading profits they made, thereby enabling us to generate different scenario.Footnote21
4. Methodology
To identify each parameter’s role in our model, we fix all the parameters at the initial settingsFootnote22 and allow the chosen parameter to vary within the considered range. We then observe the impact of each parameter in the artificial market under different shock terms based on the normal, student t (t), and α‐stable distributions, or GARCH processes. Table shows our initial settings.
Table 1. Initial settings
The initial settings mostly follow those used by Kaizoji (Citation2004). We can see in Table that the initial population fractions of fundamentalists and positive‐feedback traders are equal to 0.35. We start the simulations in which the fundamental value of the stock is equal to the market price. The existence of noise traders triggers the price fluctuations beyond the equilibrium.Footnote23 We run simulations for 10,000 steps and analyze the distribution of asset returns in the artificial market.
We then select the key parameters identified from our simulations and estimate their coefficients from January 1985 to September 2015. We move the window from January 1983 to September 2015 and estimate the coefficients of key parameter for each window. We set the window size equal to 500 trading days and the interval between each window equal to 20 trading days.Footnote24 Thus, the coefficients we estimate capture the monthly change of the chosen parameters. If the size of the simulated data is less than 500, we resample the historical data such that the size of the simulated data and the historical data is the same. The procedure is similar to a back‐testing process, where we estimate the coefficients based on the information of the past 500 trading days at the time period we observe the market.
Within the chosen parameters space, we run the simulations and apply the two‐sample Kolmogorov–Smirnov test and Anderson–Darling test with historical returns as the benchmark for estimating the coefficients of the parameters. Our null hypothesis is that the distribution of asset returns in the simulated data has the same continuous distribution as those for the historical data. We set the confidence interval equal to 95% and α = 5, and then run 500 trials. We then chose the set of parameters that minimizes the number of trials that reject the null hypothesis. If the number of trials where the null hypothesis is rejected is the same between two sets of parameters, we compare their average p‐value for the null hypothesis and choose the set with a larger average p‐value.
5. Results
We fix all the parameters at the initial settings as shown in Table and observe the impact of each parameter in the artificial market. In our model, we find that (1) the risk tolerance of fundamentalists (αf) and the relative funding rate of positive‐feedback traders (rc) versus fundamentalists are key parameters determining the path of price fluctuations, (2) fundamentalists most likely cause heavier tails, (3) positive‐feedback traders cause the formation of speculative bubbles, and (4) the existence of noise traders increases the probability that fundamentalists dominate the market. Moreover, the parameters which directly relate to traders’ desire toward wealth or profits, as measured by the wealth effect (β) and intensity to switch strategy (φ), would also affect the path of price fluctuations. The more wealth effect or expected profits affect traders’ next period’s expected utility, the higher the likelihood that those traders are able to dominate the market as a consequence. In addition, we then find that initial price (p0),Footnote25 positive‐feedback traders’ adjustment scale to expected price (μ), and initial positive‐feedback traders’ proportion in the market (κ0)Footnote26 do not have a significant influence in traders’ dominance of the market.Footnote27
5.1. Simulation results
We are able to generate the scenarios of bubbles, crashes, and normal times in our model. In our artificial market, we define bubbles as occurring when the stock price is more than eight times its fundamental value; crashes are defined as occurring when the stock price is (1) less than three, or (2) less than 57% of its fundamental value. Figure presents the scenarios for bubbles, crashes, and normal times. There are two situations in the normal time scenario. One situation (normal time‐no deviation) is the stock price close to its fundamental value and another situation (normal time‐deviation) is that the stock price deviates from its fundamental value but does not form a bubble.
We are also able to generate jump processes as shown in Figure . Jump processes tend to occur when fundamentalists or positive‐feedback traders rapidly dominate the market in the beginning of trading periods. The jump processes generated here are similar to a self‐exciting process. In our model, simulated returns are weakly autocorrelated while the absolute simulated returns display a positive, significant, and slowly decaying autocorrelations.Footnote28 Table presents the results of unit root tests, where the simulated returns are stationary.Footnote29
Table 2. Unit root test results
The relative‐risk aversion with respect to traders’ demand for stock in the market is equal to . Equations (13), (14), and (15) below represent the relative‐risk aversion coefficients of fundamentalists and positive‐feedback traders in Case 1 (linear wealth effect) and Case 2 (CRRA wealth effect), respectively.
(13)
(13)
(14)
(14)
(15)
(15)
In Case 1, fundamentalists perform better when they are more willing to take risk than positive‐feedback traders (αf increases while αc remains the same).Footnote30 Similarly, fundamentalists perform better than positive‐feedback traders do in Case 2 when αf is larger.Footnote31
To be more clear, let us look at the partial derivative of the relative‐risk aversion of fundamentalists’ demand for stock with respect to αf.(16)
(16)
when
since
. When
,
tends to be less than zero. Thus,
tends to be negative when
. An increase in αf causes the fundamentalists to be less risk‐averse and perform better by taking more risk than positive‐feedback traders.
We can see in Figure that fundamentalists are more able to dominate the market when αf increases with shock terms having different distributions.Footnote32 Figures and show the number of trials in which bubbles, crashes, and normal times (no deviation) occur. If the shock terms are α‐stable distributed or follow a GARCH process, crashes happen less as αf increases. We can observe more normal times (no deviation) when αf increases up to a threshold. However, when αf exceeds the threshold, bubbles resulting from the jump processes caused by fundamentalists occur frequently. In Figure , we can see that as fundamentalists dominate the market, the generated returns in our model have heavier tails and less volatility clustering,Footnote33 indicating that fundamentalists most likely cause heavier tails, and positive‐feedback traders may cause the formation of speculative bubbles.
Figure 6. ARCH effects, traders’ dominance versus kurtosis (Case 1, t‐distributed).
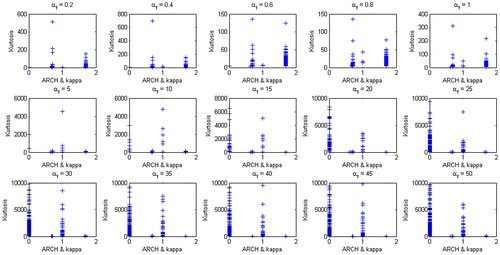
In our initial settings, fundamentalists and positive‐feedback traders trade against each other. As fundamentalists have more opportunities to dominate the market (αf increases), the Pearson’s correlation coefficient of stock demands between these two types of market participants increases (see Figure ).Footnote34 This indicates that the best strategy for fundamentalists would not be trading against positive‐feedback traders. Instead, fundamentalists are better off when they take advantage of positive‐feedback traders.
Figure 7. Average correlations of demands between fundamentalists and positive‐feedback traders (Case 1).
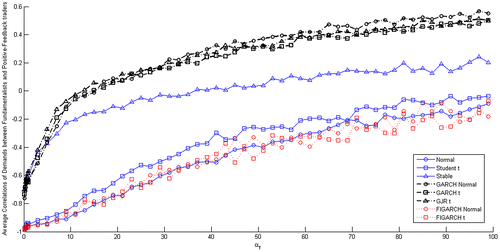
Entropy measures the variability of a sequence of discrete numbers. We measure the Shannon entropy of fundamentalists’ and positive‐feedback traders’ demand for stock.Footnote35 Figure shows the entropy measures of fundamentalists (Hf) versus positive‐feedback traders (Hc). We can see that when the fundamentalists are more determined (demand for stock is less variable relative to the positive‐feedback traders), they are more able to dominate the market. When fundamentalists trade against positive‐feedback traders (i.e. when αf is smaller), the average distance between Hf and Hc is smaller compared to the average distance when αf is larger (see Figure ).
A crisis occurs when market participants sell the stock and are reluctant to subsequently repurchase it. According to Thurner, Farmer, and Geanakoplos (Citation2012), leverage could cause fat tails and clustered volatility in the financial market. We then turn to examine the impact of funding costs in the artificial market. In our model, as the relative funding cost of positive‐feedback traders versus fundamentalists increases, more crashes occur (see Figure ) and the fundamentalists are more capable of dominating the market during the crisis (see Figure ).
Recall that the demand by positive‐feedback traders for stock is positive when
in Case 1 and
in Case 2. An increase in their funding rate (rc) would reduce their demand for a stock and at the same time reduce the liquidity of stock, leading to a higher probability of a crisis occurring. Although increasing the funding cost of positive‐feedback traders could preclude the formation of a speculative bubble and increase the probability that the stock price will trade close to its fundamental value, the trade off is the more frequent occurrence of crashes.
To investigate the dominance of fundamentalists as rc increases, we look at the partial derivative of the magnitude of ,
.
(17)
(17)
If , then
since
and
. If
, we can see that the first term is negative
and the second term tends to be positive. Then
tends to be positive when rc is small (i.e. rc = 0.1) but tends to be negative when rc is large (i.e. rc = 0.9).Footnote36 In other words, positive‐feedback traders sell more aggressively when rc is small and increasing, but sell less aggressively when rc is large and increasing when
. As rc increases, positive‐feedback traders are less active, which causes them to lose their influence on the market and perform worse than fundamentalists.
(18)
(18)
If , then
since
. Although
when
,
and the regularity condition
indicate that
is smaller as
than
is as
.Footnote37 Thus, positive‐feedback traders also tend to be less active as rc increases.
The existence of noise traders would increase the probability that fundamentalists dominate the market. We can see in Figure that fundamentalists require larger αf to dominate the market when the population fraction of noise traders in the market is smaller.
Other parameters related to profits and wealth (wealth effect, β; intensity to switch strategy, φFootnote38) show that when profits and wealth are more desirable toward traders, traders have more opportunities to dominate the market. We will discuss other parameters in more detail later in Section 6.
5.2. Empirical results
In this section we present the model’s estimate for the fundamentalists’ eagerness toward profits (αf), positive‐feedback traders’ funding rate (rc), and noise traders’ reaction strength (γ) in the US equity market as proxied by the Standard & Poor’s 500 index (S&P 500), the German equity market as proxied by the Deutsche Boerse AG German Stock Index (DAX), the British equity market as proxied by the Financial Times Stock Exchange 100 Share Index (FTSE 100), the French equity market as proxied by the Cotation Assistée en Continu quarante (CAC 40), the Swiss equity market as proxied by the Swiss Market Index (SMI), the Japan equity market as proxied by the Nikkei Stock Average (Nikkei 225), the Korean equity market as proxied by the Korea Composite Stock Price Index (KOSPI), the China equity market as proxied by the Shanghai Stock Exchange Composite Index (SSE), and the Hong Kong equity market as proxied by the Hang Seng Index (HSI). The time period covered is January 1985 to September 2015.Footnote39 We use the S&P 500, DAX, FTSE 100, CAC 40, SMI, Nikkei 225, KOSPI, SSE, HSI adjusted closing price from Bloomberg and adopt Case 1 (linear wealth effect) with t‐distributed shock terms to estimate the model.
Our estimating procedure is similar to a back-testing process, where we estimate the coefficients based on the information of the past 500 trading days for the time period we estimate the coefficients of the market.
Our chosen parameter space is:
Table
The calibrations in Section 5.1 suggest that as fundamentalists are more willing to take risks than positive‐feedback traders in the market up to some threshold, we are more able to observe asset prices as fundamental values. In Figure where we present the αf estimated for the nine markets, the dotted lines represent the estimated αf by the Kolmogorov–Smirnov (KS) test, and the solid lines are the stock index in the nine markets. We can see that fundamentalists did not have a large impact on the market before 1995, where fundamentalists traded actively without observing large trends in markets. This could be the result of the popularity of mutual funds and the growth of proprietary trading by banks after 1995. From Figure , we can see that the general level of risk bearing of fundamentalists was low during the periods January 1985 to September 2015 where stock prices increased, and that the fundamentalists traded aggressively after the burst of the Dot‐com Bubble (March 2000) and the announcement of the bankruptcy of Lehman Brothers (September 2008). The result is in line with the findings of Griffin et al. (Citation2011) who suggest that institutional investors drove and burst the 1997–2000 hi‐tech bubble. The fundamentalists became risk‐averse and rode the bubble once the market price rebounded. Compared to the results estimated by the KS test, the results estimated by the AD test are more sensitive.
Looking at the impact of fundamentalists on markets with respect to stock prices, returns, and absolute returns after 1995,Footnote40 our results indicate that they vary across markets. For example, we can see in Table that the αf is negatively correlated with stock prices for the CAC 40, but the αf is positively correlated with stock prices for the Nikkei 225. In general, αf tends to be negatively correlated with stock prices and returns except when fundamentalists strongly buy the stocks when the stocks’ prices are extremely undervalued. Moreover, the generally positive correlations between αf and absolute stock returns indicate fundamentalists have a significant impact on markets.
Table 3. Correlations between estimated αf and stock prices, returns, or absolute returns
Figure presents the estimated relative positive‐feedback traders’ funding rate (rc) versus fundamentalists for the nine markets based on the KS test. rc indicates the instability of the financial market.Footnote41 Figure compares the estimated rc with the U.S. federal funds effective rate from January 1985 to September 2015. Our results for rc identify the periods where positive‐feedback traders would face higher funding rate than fundamentalists.
Figure presents the estimated noise traders’ reaction strength (γ). The thick dotted and dotted lines represent the estimated γ by the KS test and the AD test, respectively, and the solid lines are the stock index in the nine markets. γ reflects noise traders’ reaction strength to news (e.g. the information they receive). Noise traders tend to be more active in markets exhibiting an upward trend, but are reluctant to trade during the downward trends. For instance, the rising price level of the S&P 500 ignited noise traders’ desire to purchase stocks, but the negative news after the burst of the Dot‐com Bubble and the announcement of Lehman Brothers bankruptcy greatly reduced their desire to purchase stocks.Footnote42
6. Discussions
6.1. Simulation results Case 2 (CRRA wealth effect)
Similar to the simulation results in Case 1 (linear wealth effect), fundamentalists are more able to dominate the market when αf and rc increase. An increase of rc could preclude the formation of a speculative bubble and increase the probability that the stock price will trade close to its fundamental value, but the trade off is the frequent occurrence of crashes. In addition, an increase of αf does not reduce the formation of a speculative bubble, which could result from the more aggressiveness of positive‐feedback traders in Case 2 than Case 1.
Let us consider the situation that fundamentalists would short the stock when they expect its price to fall and positive‐feedback traders have linear wealth effect, which we refer to as Case 3. Equations (19) and (20) show fundamentalists’ demand for stock and positive‐feedback traders’ demand for stock
.
(19)
(19)
(20)
(20)
Fundamentalists in Case 3 are more aggressive than the fundamentalists in Case 1, and positive‐feedback traders in Case 2 are more aggressive than the positive‐feedback traders in Case 1.Footnote43 As a result, larger αf is required for fundamentalists to dominate the market in Case 2 than in Case 1. In addition, the relative risk-bearing between fundamentalists and positive-feedback traders in Case 2 does not affect the outcomes. Figure compares the results of Cases 1, 2, and 3 as αf vary.
6.2. Other parameters
6.2.1. Wealth effect and strategy switching intensity
Positive‐feedback traders are less risk‐averse when β is larger in Case 1 or β is smaller in Case 2.Footnote44 When the wealth effect restricts positive‐feedback traders in Cases 1 and 2 or when the intensity of choice to switch strategies φ is larger in Case 1,Footnote45 we are able to observe the stock prices reflecting fundamental values with fundamentalists dominating the market.
6.2.2. Speed of adaptive process
We can think of the adjustment scale to expected price (ν, μ)Footnote46 as how fast information spreads among the same type of traders. Let us assume that αf is equal to 30 in Cases 1 and 2, so that fundamentalists have a greater chance to dominate the market than our default setting.Footnote47 Fundamentalists gain advantages when the information spreads more quickly among their group, and we are more able to observe stock prices as its fundamental value when ν increases up to a threshold in Case 1. However, the changes of μ do not have a significant impact on the market.
6.2.3. Noise traders’ reactions
In general, fundamentalists are able to dominate the market when markets are more agitated (i.e. γ and ξ are larger). Moreover, as γ and ξ become larger, we observe more violations of the regularity condition of positive‐feedback traders in Case 2.
6.2.4. Fundamentalists’ funding costs and market sensitivity to demands
Let us suppose αf equals 30 in Cases 1 and 2, so that fundamentalists have a greater chance to dominate the market than our default setting. An increase in funding costs (rf increases) would deter fundamentalists from trading against positive‐feedback traders. In addition, when the demand for stock has more impact on the stock price (market’s sensitivity to the demand of stock θ increases), positive‐feedback traders have a greater influence on the market in Case 1.
6.3. Goodness of fit, stability test, and identifiability of the model
Table shows the statistics for rejecting the null hypothesis in our empirical results. We can see that the goodness of fit based on the AD test has larger deviations than based on the KS test. We utilize the same methodology twice for the S&P 500 and look at the correlation between first‐trial and second‐trial estimated results. We can see in Table that the estimated αf is not stable. Figure provides an example of estimating the parameters of the same window for several trials. We can see that our methodology tends to be efficient (i.e. the same estimated results are obtained).
Table 4. Statistics for rejecting the null hypothesis
Table 5. Correlations between first‐trial and second‐trial estimated parameters
Figure provides an example of the likelihoods of rejecting the null hypothesis, where we fix two of the optimal estimated parameters and allow the rest one parameter to vary within our parameter choices. We can see that our estimated results are significant (i.e. the optimal estimated parameters have better fitness compared to alternative choices). To see the identifiability of the model, we use the estimated parameters, simulate the logarithmic returns, and compare the results to the real data. When αf is less than 3.5 or rc exceeds 0.3, our model tends to generate more rare events in the tails compared to the historical data (see Figure ).Footnote48
7. Conclusions
In this paper, we develop and examine a simple interactive agent‐based model, where the distribution of returns generated from the model take into accounts two stylized facts: fat tails and volatility clustering. Our simulation results indicate that the risk tolerance of fundamentalists and the relative funding rate of positive‐feedback traders versus fundamentalists are key factors determining the path of price fluctuations. Fundamentalists are more able to dominate the market when they are more willing than positive‐feedback traders to take risk. In addition, fundamentalists could be the source of heavier tails, and positive‐feedback traders cause the formation of speculative bubbles.
We provide a novel approach using a moving window methodology and applying the two‐sample Kolmogorov–Smirnov and Anderson–Darling tests to estimate the fundamentalists’ eagerness toward profits, positive‐feedback traders’ funding rate versus fundamentalists, and noise traders’ reaction strength in the markets over time. Our empirical results for the nine equity markets we choose are able to reflect market situations (e.g. bursting of the Dot‐com Bubble and the announcement of the Lehman Brothers bankruptcy). The generally low level of risk bearing by fundamentalists in the nine markets could explain the frequent occurrence of bubbles during the period from January 1985 to September 2015.
Our work shows the potential of agent‐based models’ application in finance, such as risk management and high‐frequency trading. Future research would be to generate a more general heterogeneous agent model and develop a more stable estimation methodology.
Funding
The authors received no direct funding for this research.
Supplementary material
Supplementary material for this article can be accessed here https://doi.org/10.1080/23322039.2017.1381370.
OAEF_Supplementary_materials.docx
Download MS Word (778.3 KB)Acknowledgement
We are grateful to Svetlozar Rachev, Frank Fabozzi, Sandro Brusco, Raphael Douady, Sergio Focardi, James Glimm, Chansog Kim, Andrew Mullhaupt, Remus Radu, Noah Smith, Stoyan Stoyanov, Keli Xiao, Svetlana Boyarchenko, and anonymous referees for insightful advice and comments.
Additional information
Notes on contributors
Po-Keng Cheng
Po-Keng Cheng received his doctorate degree from SUNY‐Stony Brook University, U.S.A. He has been working on research of interactive agent‐based models investigating traders’ behaviors and their trading strategies in financial markets. He currently also involves in studies related to probability, dynamical systems, and game theory.
Young Shin Kim
Young Shin Kim received his doctorate degree from Sogang University, Korea, and completed his Habilitation (Doctor of Science) in Karlsruhe Institute of Technology, Germany. His current professional and research interests are in the area of mathematical modeling and its application to finance. He is interested in mathematical models having fat‐tails, asymmetric dependence, clustering of volatility, and long-range dependence related to topics including financial risk management, portfolio management, and derivative pricing. He has published dozens of research papers in international reputable refereed journals. He is an expert in computer programming and shares basic libraries and tools developed by him.
Notes
1. See, for example, Rietz (Citation1988), Barro (Citation2006), and Julliard and Ghosh (Citation2012).
2. See, for example, Youssefmir and Huberman (Citation1997), Brock and Hommes (Citation1998), Lux (Citation1998), LeBaron, Arthur, and Palmer (Citation1999), and Lux and Marchesi (Citation1999, Citation2000).
3. See, for example, Alfarano, Lux, and Wagner (Citation2005), Boswijk, Hommes, and Manzan (Citation2007), and de Jong, Verschoor, and Zwinkels (Citation2010).
4. See, for example, Tesfatsion and Judd (Citation2006), Hens and Schenk-Hoppé (Citation2009), LeBaron (LeBaron, Citation2000, Citation2012).
5. See, for example, Sims (Citation2003), Peng (Citation2005), Van Nieuwerburgh and Veldkamp (Citation2010), Backus, Chernov, and Zin (Citation2014).
6. See, for example, Harrison and Kreps (Citation1978).
7. The existence of two expectation regimes, fundamentalists and trend following regimes, in the market is identified by Boswijk et al. (Citation2007) and de Jong et al. (Citation2010).
8. See, for example, Glosten and Milgrom (Citation1985), Kyle (Citation1985), Black (Citation1986), De Long et al. (Citation1990b), and Bloomfield, O’Hara, and Saar (Citation2009).
9. Positive‐feedback traders are viewed as noise traders in most studies. However, in order to identify the role of each type of trader in the market, here we classify traders into three groups: fundamentalists, positive‐feedback traders, and noise traders.
10. If the fundamental value is a submartingale, traders will buy the stock. Conversely, if the fundamental value is a supermartingale, traders will sell the stock.
11. Note that the best strategy for fundamentalists wouldn’t be to have their price belief equal to the fundamental value. Because of the existence of other types of traders, the market price wouldn’t move back to fundamental value in next period.
12. We can also interpret αf as how sensitive fundamentalists are to the differences between their next period expected price and the current stock price.
13. The logarithmic term for costs is to enable the expected utility function to be concave with respect to the demand for the stock. In practice, one can use the methodology introduced in Section 4 to find best fitted model for historical data.
14. Note that traders here are rationally bounded and intuitively sum up marginal utilities of profits, wealth, and costs as a criteria for their utilities.
15. If β = 0, then the positive‐feedback traders are not affected by the wealth effect. The existence of the wealth effect causes the positive‐feedback traders to be less risk‐averse and trade more aggressively compared to fundamentalists. The wealth effect could be the reason why the individual investors trade frequently and overconfidently. For discussions related to overconfidence, see , for example, De Long, Shleifer, Summers, and Waldmann (Citation1989), De Long et al. (Citation1990b), De Long, Shleifer, and Summers (Citation1991), Odean (Citation1998), Barber and Odean (Citation2000), Hirshleifer and Luo (Citation2001), and Chuang and Susmel (Citation2011).
16. The regularity condition is rarely violated in our simulations.
17. From our simulation results, ξ does not have a significant impact on the type of traders who would dominate the market when ξ < 0.5. However, as there are more noise traders in the market, fundamentalists perform better.
18. We can see in the utility function of fundamentalists in Equation (5) and positive‐feedback traders in Equations (7) and (9) that their transaction costs increase as pt increases.
19. See, for example, Lux (Citation1998), and Lux and Marchesi (Citation1999).
20. Fundamentalists and positive‐feedback traders switch strategies based on the prediction errors in Kaizoji (Citation2004).
21. For intuitions about different utility functions of fundamentalists and positive‐feedback traders, one can consider the inflows and outflows of mutual funds in which traders can trade by themselves or invest in mutual funds.
22. We will discuss the special cases in Section 6.
23. The equilibrium here means the stock price is equal to its fundamental value, which is different from the meaning of an equilibrium price cleared by the market.
24. We choose the window size being equal to 500 trading days from a statistical point of view. Further studies would be to investigate robustness and significance of the impact resulted from different window sizes. For descriptions of goodness‐of‐fit tests, see for example, Darling (Citation1957), Stephens (Citation1974), Crutcher (Citation1975), Dallal and Wilkinson (Citation1986) and Chernobai, Rachev, and Fabozzi (Citation2007) Chapter 10.
25. When the initial price is less than half of its fundamental value, the outcomes are different. This situation is similar to a crisis occurring where fundamentalists are more able to dominate the market.
26. We restricted the population fractions of positive‐feedback traders ranging from 0.05 to 0.65 in the initial periods, but observed the same outcome. Different outcomes could occur only when the performance differentials between the strategies are large enough such that the traders in the market all switch to the best performing strategy. However, this requires restricting the population fractions of each type of trader for a long time.
27. Because of space constraints, we cannot report all of the simulation results here. The codes are available from the authors.
28. All the simulation and estimation results are available from the authors.
29. We first find the best fitted AR process for the simulated returns, and then run unit root tests.
30. If αf increases and αc also increases equally (αf/αc remains the same), then the result would not change. For example, the results are similar when αf = αc = 6.5 and αf = αc = 1.5.
31. In Case 2, the magnitude of αcdoes not have significant impact on the outcomes.
32. Here we set and ɛ2, t varies with different distributions:
,
,
, GARCH normal with α0 = 0, α1 = 0.9, β1 = 0.05, GARCH t
with υ = 5, α0 = 0, α1 = 0.9, β1 = 0.05, GJR t with υ = 5, α0 = 0, α1 = 0.9, β1 = 0.05, γ = 0.1,
FIGARCH (1,d,1) normal with ω = 0.05, α = 0.05, d = 0.4, β = 0.45 and FIGARCH (1,d,1) t
with υ = 5, ω = 0.05, α = 0.05, d = 0.4, β = 0.45.
33. We use the ARCH test for the presence of volatility clustering.
34. When fundamentalists dominate the market, positive‐feedback traders update their price beliefs based on the market prices which fundamentalists have had an influential impact.
35. Here we measure the Shannon entropy by dividing the demand for stock into 10 levels at the range .
36. If rc is small (large), the second (first) term of would determine the sign of
.
37. Observe that the range of is much shorter when
compared to the range of
when
.
38. Intensity to switch strategy would also be a key factor causing a heavy tail and volatility clustering, but the investigation and estimation of the parameter are beyond the scope of this paper because our focus is on how risk aversion affects the behavior of traders in the market.
39. The time period covered in different markets vary due to the availability of data from Bloomberg. For instance, the adjusted closing prices for the SSE index are available since 12/19/1990.
40. From Figure , we know that fundamentalists did not have a large impact on the market before 1995.
41. Recall that crises have higher probability of occurrence when rc > 0.2 (see Figure ).
42. γ may have predictive power on next period’s price level of stocks, which is in line with Barber, Odean, and Zhu (Citation2009) who argue that retail investors push prices over short horizons.
43. The analysis is provided in Appendix A.
44. Taking the partial derivative of the relative risk aversion of positive‐feedback traders’ demand for stock with respect to β, we observe that tends to be negative and
tends to be positive.
when
, but
is positive when
since
and
is positive. However, we can see that the range of
when
is much smaller compared to the range of
when
, which restricts the positive‐feedback traders to trade aggressively when
.
The first term of tends to be positive if
since
from the regularity condition and the two terms,
, tend to be negative. If
, the first term of
tends to be negative since the two terms,
, tend to be positive. The second term of
is positive when
but is negative when
.
Alternative way to analyze is to look at the partial derivative of the magnitude of ,
.
is negative when
but is positive when
. However, we know that the range of
is much smaller when
compared to the range of
when
. Positive‐feedback traders tend to be more risk‐averse when β increases in Case 2.
45. Higher values of φ represent situations in which agents switch more rapidly to a best‐performing strategy.
46. Recall that ν captures the speed at which fundamentalists expect the market price to move back to fundamental value and μ is the error correction coefficient related to how sensitive positive‐feedback traders correct their expected stock price for the next period.
47. When αf is equal to 1, the results are not significant.
48. Recall that bubbles occur when fundamentalists are risk‐averse and crashes occur when rc is larger than 0.2 in our model.
References
- Abreu, D., & Brunnermeier, M. K. (2003). Bubbles and Crashes. Econometrica, 71(1), 173–204.10.1111/ecta.2003.71.issue-1
- Alfarano, S., Lux, T., & Wagner, F. (2005). Estimation of agent-based models: The case of an asymmetric herding model. Computational Economics, 26(1), 19–49.10.1007/s10614-005-6415-1
- Backus, D., Chernov, M., & Zin, S. (2014). Sources of entropy in representative agent models. The Journal of Finance, 69(1), 51–99.10.1111/jofi.12090
- Baker, M., & Wurgler, J. (2007). Investor sentiment in the stock market. Journal of Economic Perspectives, 21(2), 129–151.10.1257/jep.21.2.129
- Barber, B. M., & Odean, Terrance (2000). trading is hazardous to your wealth: The common stock investment performance of individual investors. The Journal of Finance, 55(2), 773–806.10.1111/0022-1082.00226
- Barber, B. M., Odean, T., & Zhu, N. (2009). Do retail trades move markets? Review of Financial Studies, 22(1), 151–186.10.1093/rfs/hhn035
- Barberis, N., Greenwood, R., Jin, L., & Shleifer, A. (2015). X-CAPM: An extrapolative capital asset pricing model. Journal of Financial Economics, 115(1), 1–24.10.1016/j.jfineco.2014.08.007
- Barro, R. J. (2006). Rare disasters and asset markets in the twentieth century. Quarterly Journal of Economics, 121(3), 823–866.10.1162/qjec.121.3.823
- Black, F. (1986). Noise. The Journal of Finance, 41(3), 529–543.10.1111/j.1540-6261.1986.tb04513.x
- Blanchard, O. J., & Watson, M. W. (1982). Bubbles, rational expectations and financial markets. In P. Wachtel (Ed.), Crises in the economic and financial structure (pp. 295–316). Lexington, MA: D.C. Heathand Company.
- Bloomfield, R., O’Hara, M., & Saar, G. (2009). How noise trading affects markets: An experimental analysis. Review of Financial Studies, 22(6), 2275–2302.10.1093/rfs/hhn102
- Boswijk, H. P., Hommes, C. H., & Manzan, S. (2007). Behavioral heterogeneity in stock prices. Journal of Economic Dynamics and Control, 31(6), 1938–1970.10.1016/j.jedc.2007.01.001
- Brock, W. A., & Hommes, C. H. (1998). Heterogeneous beliefs and routes to chaos in a simple asset pricing model. Journal of Economic Dynamics and Control, 22(8–9), 1235–1274.
- Brunnermeier, K. M., & Nagel, S. (2004). Hedge funds and the technology bubble. The Journal of Finance, 59(5), 2013–2040.10.1111/j.1540-6261.2004.00690.x
- Chernobai, A. S., Rachev, S. T., & Fabozzi, F. J. (2007). Operational risk: A guide to Basel II capital requirements, models, and analysis. Hoboken, NJ: John Wiley & Sons.
- Chuang, W.-I., & Susmel, R. (2011). Who is the more overconfident trader? Individual vs. institutional investors. Journal of Banking & Finance, 35(7), 1626–1644.10.1016/j.jbankfin.2010.11.013
- Crutcher, H. L. (1975). A note on the possible misuse of the Kolmogorov-Smirnov test. Journal of Applied Meteorology, 14(8), 1600–1603.10.1175/1520-0450(1975)014<1600:ANOTPM>2.0.CO;2
- Dallal, G. E., & Wilkinson, L. (1986). An analytic approximation to the distribution of Lilliefors’s test statistic for normality. The American Statistician, 40(4), 294–296.
- Darling, D. A. (1957). The Kolmogorov-Smirnov, Cramer-von Mises tests. The Annals of Mathematical Statistics, 28(4), 823–838.10.1214/aoms/1177706788
- de Jong, E., Verschoor, W. F. C., & Zwinkels, R. C. J. (2010). Heterogeneity of agents and exchange rate dynamics: Evidence from the EMS. Journal of International Money and Finance, 29(8), 1652–1669.10.1016/j.jimonfin.2010.05.007
- De Long, J. B., Shleifer, A., Summers, L. H., & Waldmann, R. J. (1989). The size and incidence of the losses from noise trading. The Journal of Finance, 44(3), 681–696.10.1111/j.1540-6261.1989.tb04385.x
- De Long, J. B., Shleifer, A., Summers, L. H., & Waldmann, R. J. (1990a). Positive feedback investment strategies and destabilizing rational speculation. The Journal of Finance, 45(2), 379–395.10.1111/j.1540-6261.1990.tb03695.x
- De Long, J. B., Shleifer, A., Summers, L. H., & Waldmann, R. J. (1990b). Noise trader risk in financial markets. Journal of Political Economy, 98(4), 703–738.10.1086/261703
- De Long, J. B., Shleifer, A., Summers, L. H. &, Waldmann, R. J. (1991). The survival of noise traders in financial markets. Journal of Business, 64(1), 1–19.
- Edelen, R. M., Marcus, A. J., & Tehranian, H. (2010). Relative sentiment and stock returns. Financial Analysts Journal, 66(4), 20–32.10.2469/faj.v66.n4.2
- Frankel, J. A., & Froot, K. A. (1990). Chartists, fundamentalists, and trading in the foreign exchange market. American Economic Review, 80(2), 181–185.
- Frankel, J. A., & Froot, K. A. (1991, October). Chartists, fundamentalists and the demand for dollars (No. r1655). Cambridge, MA: National Bureau of Economic Research.
- Glosten, L. R., & Milgrom, P. R. (1985). Bid, ask and transaction prices in a specialist market with heterogeneously informed traders. Journal of Financial Economics, 14(1), 71–100.10.1016/0304-405X(85)90044-3
- Greenwood, R., & Shleifer, A. (2013). Expectations of returns and expected returns (No. w18686). Cambridge, MA: National Bureau of Economic Research.10.3386/w18686
- Griffin, J. M., Harris, J. H., Shu, T., & Topaloglu, S. (2011). Who drove and burst the tech bubble? The Journal of Finance, 66(4), 1251–1290.10.1111/j.1540-6261.2011.01663.x
- Harrison, J. M., & Kreps, D. M. (1978). Speculative investor behavior in a stock market with heterogeneous expectations. Quarterly Journal of Economics, 92(2), 323–336.10.2307/1884166
- Hens, T., & Schenk-Hoppé, K. R. (eds.) (2009). Handbook of financial markets: Dynamics and evolution. Amsterdam: North-Holland.
- Hirshleifer, D., & Luo, G. Y. (2001). On the survival of overconfident traders in a competitive securities market. Journal of Financial Markets, 4(1), 73–84.10.1016/S1386-4181(00)00014-8
- Julliard, C., & Ghosh, A. (2012). Can rare events explain the equity premium puzzle? Review of Financial Studies, 25(10), 3037–3076.10.1093/rfs/hhs078
- Kaizoji, T. (2002). Speculative dynamics in a heterogeneous agent model. Nonlinear Dynamics, Psychology, & Life Sciences, 217–229.10.1023/A:1014070413635
- Kaizoji, T. (2004). Speculative bubbles and fat tail phenomena in a heterogeneous‐agent model. In International Symposia in Economic Theory and Econometrics (Vol. 14, 259–275). Emerald Group Publishing Limited.
- Kirchler, M., & Huber, J. (2007). Fat tails and volatility clustering in experimental asset markets. Journal of Economic Dynamics and Control, 31(6), 1844–1874.10.1016/j.jedc.2007.01.009
- Kyle, A. S. (1985). Continuous auctions and insider trading. Econometrica, 53(6), 1315–1335.10.2307/1913210
- LeBaron, B. (2000). Agent-based computational finance: Suggested readings and early research. Journal of Economic Dynamics and Control, 24(5), 679–702.10.1016/S0165-1889(99)00022-6
- LeBaron, B. (2012). Heterogeneous gain learning and the dynamics of asset prices. Journal of Economic Behavior & Organization, 83(3), 424–445.10.1016/j.jebo.2012.03.003
- LeBaron, B., Arthur, W. B., & Palmer, R. (1999). Time series properties of an artificial stock market. Journal of Economic Dynamics and Control, 23(9), 1487–1516.10.1016/S0165-1889(98)00081-5
- Lux, T. (1998). The socio-economic dynamics of speculative markets: Interacting agents, chaos, and the fat tails of return distributions. Journal of Economic Behavior & Organization, 33(2), 143–165.10.1016/S0167-2681(97)00088-7
- Lux, T., & Marchesi, M. (1999). Scaling and criticality in a stochastic multi-agent model of a financial market. Nature, 397(6719), 498–500.10.1038/17290
- Lux, T., & Marchesi, M. (2000). Volatility clustering in financial markets: A microsimulation of interacting agents. International Journal of Theoretical and Applied Finance, 3(4), 675–702.
- Mehra, R., & Prescott, E. C. (1985). The equity premium: A puzzle. Journal of Monetary Economics, 15(2), 145–161.10.1016/0304-3932(85)90061-3
- Odean, T. (1998). Volume, volatility, price, and profit when all traders are above average. The Journal of Finance, 53(6), 1887–1934.10.1111/0022-1082.00078
- Peng, L. (2005). Learning with information capacity constraints. Journal of Financial and Quantitative Analysis, 40(2), 307–329.10.1017/S0022109000002325
- Qiu, L., & Welch, I. (2004). Investor sentiment measures (No. w10794). Cambridge, MA: National Bureau of Economic Research.10.3386/w10794
- Rietz, T. A. (1988). The equity risk premium a solution. Journal of Monetary Economics, 22(1), 117–131.10.1016/0304-3932(88)90172-9
- Shleifer, A., & Summers, L. H. (1990). The noise trader approach to finance. Journal of Economic Perspectives, 4(2), 19–33.10.1257/jep.4.2.19
- Sims, C. A. (2003). Implications of rational inattention. Journal of Monetary Economics, 50(3), 665–690.10.1016/S0304-3932(03)00029-1
- Stephens, M. A. (1974). EDF Statistics for Goodness of Fit and Some Comparisons. Journal of the American Statistical Association, 69(347), 730–737.10.1080/01621459.1974.10480196
- Tesfatsion, L., & Judd, K. L. (Eds.). (2006). Handbook of computational economics: Agent-based computational economics (Vol. 2). Amsterdam: North-Holland.
- Thurner, S., Farmer, J. D., & Geanakoplos, J. (2012). Leverage causes fat tails and clustered volatility. Quantitative Finance, 12(5), 695–707.10.1080/14697688.2012.674301
- Van Nieuwerburgh, S., & Veldkamp, L. (2010). Information acquisition and under-diversification. Review of Economic Studies, 77(2), 779–805.10.1111/roes.2010.77.issue-2
- Youssefmir, M., & Huberman, B. A. (1997). Clustered volatility in multiagent dynamics. Journal of Economic Behavior & Organization, 32(1), 101–118.10.1016/S0167-2681(96)00021-2
Appendix A
Expected utility function in Case 1 and Case 2
In this appendix, we provide an analysis of the expected utility function for fundamentalists and positive‐feedback traders for Cases 1, 2, and 3.
Fundamentalists maximize their next period expected utility according to(A1)
(A1)
From the first‐order necessary condition (FOC), we can derive the fundamentalists’ demand for stock . We then check the second‐order necessary condition (SOC) of
. The derivations are provided below.
Let ,
then .
(i) | FOC Let | ||||
(ii) | SOC Let | ||||
(iii) |
|
then
Let and
, then
and
, which indicates that
is the minimum. Thus,
.
Let us consider the situation that fundamentalists would short the stock when they expect its price to fall in Case 3 and compare the when
. We can see that
in Case 3 is larger than
in Case 1, which indicates that fundamentalists are more aggressive in Case 3 than they are in Case 1 when αf ≥ 1. Following is the proof.
If ,
If .
Positive‐feedback traders maximize their next period expected utility as (A2) in Case 1 and (A3) in Case 2.(A2)
(A2)
(A3)
(A3)
Similar to the procedures that we derive for fundamentalists’ demand for the stock, we derive the positive‐feedback traders’ demand for stock by FOC and check SOC for the
we derived. The following provides the derivations.
Case 1
Let ,
then .
(i) | FOC Let | ||||
(ii) | SOC Let | ||||
(iii) |
then Let |
Case 2
Let ,
then
(i) | FOC Let | ||||
(ii) | SOC Let | ||||
(iii) | We can see that SOC holds either for
then Let
Comparing |
(i) |
When Thus, | ||||
(ii) |
|