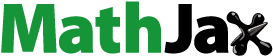
Abstract
The objective of the paper is to extend the results in Fournié, Lasry, Lions, Lebuchoux, and Touzi (1999), Cass and Fritz (2007) for continuous processes to jump processes based on the Bismut–Elworthy–Li (BEL) formula in Elworthy and Li (1994). We construct a jump process using a subordinated Brownian motion where the subordinator is an inverse -stable process
with
. The results are derived using Malliavin integration by parts formula. We derive representation formulas for computing financial Greeks and show that in the event when
, we retrieve the results in Fournié et al. (1999). The purpose is to by-pass the derivative of an (irregular) pay-off function in a jump-type market by introducing a weight term in form of an integral with respect to subordinated Brownian motion. Using MonteCarlo techniques, we estimate financial Greeks for a digital option and show that the BEL formula still performs better for a discontinuous pay-off in a jump asset model setting and that the finite-difference methods are better for continuous pay-offs in a similar setting. In summary, the motivation and contribution of this paper demonstrates that the Malliavin integration by parts representation formula holds for subordinated Brownian motion and, this representation is useful in developing simple and tractable hedging strategies (the Greeks) in jump-type derivatives market as opposed to more complex jump models.
Public Interest Statement
This paper entitled Bismut–Elworthy–Li Formula for Subordinated Brownian Motion Applied to Hedging Financial Derivatives provides pricing and risk management methods usable by quantitative risk analysts. It provides knowledge on how one can design and determine prices of new derivative products in a jump-type financial market. In addition, market risk can be managed using Greek representations to determine for instance, proportions in one’s investment portfolio, make informed decision based on the behaviour of volatility and/or potential extreme shocks in the market. It can be argued that simpler methods could be used instead to achieve the above objectives. Agreed, however, care must be taken to ensure that simple model of choice represents the market as closely as possible. This current paper is geared towards achieving exactly that. Stochastic volatility models with similar modelled jumps could be further innovations.
1. Introduction
The problem of computating the Greeks of derivatives with smooth pay-off functions has been extensively studied. The problem where the pay-off function is not necessarily regular poses a different level of difficult and requires a different approach. See for instance Fournié, Lasry, Lions, Lebuchoux, and Touzi (Citation1999) and Cass and Fritz (Citation2007). Notice that existing techniques avoid differentiating the pay-off function by introducing a weight function. The Bismut–Elworthy–Li (BEL) representation formula (Elworthy & Li, Citation1994) is one scenario of such innovations. In this paper we show that the known relationship between the Malliavin derivative and the first variation process still holds for an alpha-stable subordinated Brownian motion and results in an explicit martingale weight factor. This allows for an extension of the BEL formula to subordinated Brownian motion and as a result, Greeks can be easily computed in jump-type and/or emerging markets. The subordinator belongs to the Lévy family of four parameter alpha-stable distributions. Price dynamics of almost all financial instruments in the different financial markets are observed to deviate from the Gaussian distribution. Various models in literature have been developed to closely estimate the dynamics of these markets. The rich and robust family of alpha-stable distributions has proven successful over most traditional models in capturing skewed and heavy tailed distributions. An application to estimate the densities of solutions to stochastic differential equation with subordinated Brownian motion under Malliavin framework is discussed in Kusuoka (Citation2010). A rather different approach is discussed in Wyłomaǹska (Citation2012) where the authors, in addition to investigating the densities of subordinated Brownian motion, also discuss some properties related to transforms and averaged mean squared displacements of the process. They consider and compare both cases of stable processes and their inverses. In addition they provide some parameter estimation techniques. An intuitive study related to the work in this current paper is Elworthy and Li (Citation1994). However, this is limited to the Delta. The authors derived derivatives of solutions of diffusion equations and demonstrate that these derivatives exhibit and allow for the estimation of a diffusion equations’ smoothing properties. In addition, they use their results to study the logarithmic gradient of heat kernels. Their results can be extended to deriving representation formulas for more Greeks. A less related but still interesting work is by Song and Vondraček (Citation2003) where properties of a killed subordinated Brownian motion by an -stable are compared with those of an
-stable subordinated Brownian motion. They show possible comparability in their killing measures and propose bounds on the Green function and a jumping kernel of a subordinated (
) process. The work by Zhang (Citation2012) on the derivative and gradient estimate for SDEs driven by stable processes is another intuitive literature vital to our study. Other related works that are recent include Takeuchi (Citation2010), Khedher (Citation2012) and Kawai and Kohatsu-Higa (Citation2010). There is more existing literature on stable distributions that we cannot exhaustively discuss. Interested readers can refer to Sun and Xie (Citation2014) for a comprehensive literature on stable distributions.
In summary, the motivation and contribution of this paper demonstrates that Malliavin integration by parts representation formula holds for subordinated Brownian motion and, this representation is useful in developing simple and tractable hedging strategies (the Greeks) in jump-type derivatives market as opposed to more complex jump models.
The rest of the paper is organised as follows: In Section 2 we review some traditional techniques for computing the Greeks. In Section 3 we derive some useful results about stable processes and Malliavin Calculus with regards to subordinated Brownian motion. In Section 4 we analyse the differential calculus of our choice of process and show that the integration by parts formula exists. In Section 5 we present the BEL formula for subordinated Brownian motion and use the result to derive the main results of the paper. In Section 6 we discuss some applications. Section 7 concludes.
2. Sensitivity analysis
The theory of risk-neutral valuation asserts that given a complete filtered probability space with a subjective probability measure
and a filtration
satisfying the usual conditions, we can construct a pay-off function
of an option under an equivalent martingale measure whose price is given by
(1)
(1)
where is a discounted pay-off function by a risk free interest rate, r and
is the value of the underlying at maturity time T. A Greek is a derivative of (1) with respect to a certain model parameter p which could be the initial value
of the underlying, the volatility parameter
, time to maturity
, the option strike, E or the interest rate, r i.e.
(2)
(2)
Clearly, (2) poses a problem if is not differentiable. Different techniques in literature have been explored to resolve this challenge. For instance the Likelihood method (Fournié et al., Citation1999) suitable for known distribution of the underlying. It takes the form
(3)
(3)
where denotes the density of the underlying. Another widely known approach is the method of Malliavin calculus (see Bavouzet & Messaoud, Citation2006; Bayazit & Nolder, Citation2013; Bermin, Citation2000; Di Nunno, Øksendal, & Proske, Citation2008; Nualart, Citation1995). It eliminates differentiation of the pay-off function by introducing a weight term composed of Malliavin derivative and the Ornstein–Uhlenbeck operator terms, i.e.
(4)
(4)
where the weight factor consists of Malliavin derivatives of random variables X and G belonging to some space with nice properties e.g.
. This approach is more flexible than the previous one in the sense that the distribution of the underlying is irrelevant in computing the Greeks. However, it is computationally expensive. Another approach is the Bismut–Elworthy–Li representation formula Elworthy and Li (Citation1994), Cass and Fritz (Citation2007) and Baños, Duedahl, Meyer-Brandis, and Proseke (Citation2015),
(5)
(5)
where , the underlying initial spot price and
is some bounded function satisfying
(6)
(6)
The Bismut–Elworthy–Li Formula (5) applies to continuous diffusion processes but can be adapted to finite (see Cass & Fritz, Citation2007) and infinite (see Chen, Song, & Zhang, Citation2015) jump processes. The usefulness of this formula is its allowance for an explicit representation of the Delta of a financial derivative.
For instance by employing the theory of Malliavin calculus, the weight can be obtained explicitly for different Brownian motion functionals. Malliavin calculus for both continuous and jump diffusion processes has been extensively discussed in literature and there exist enormous applications on the subject (see Baños et al., Citation2015; Bavouzet & Messaoud, Citation2006, Bayazit & Nolder, Citation2013; Cass & Fritz, Citation2007; Di Nunno et al., Citation2008; Elworthy & Li, Citation1994; Kusuoka, Citation2010). The focus of this paper is to compute the Greeks for a wide range of pay-offs irrespective of their structure by employing the BEL formula in the framework of subordinated Brownian motion by
-stable distributions, with
.
3. Stable distributions
In this section we shall adapt some of the definitions and notations from Kateregga, Mataramvura, and Taylor (Citation2017).
Definition 3.1
A stable distribution is a four-parameter family of distributions denoted by where
is the characteristic exponent responsible for the shape of the distribution.
is responsible for skewness of the distribution.
is the scale parameter (it narrows/extends the distribution around
).
is the location parameter (it shifts the distribution to the left or the right).
In this paper we are only interested in positive, non-decreasing, stable cádlág processes denoted by whose density functions are defined on
. For simplicity, we shall denote by
the set of all such processes. More so, we shall denote the inverse of
by
. The purpose is to introduce jumps in asset returns through subordinated Brownian motion and in turn, provide pricing and hedging (the Greeks) representation formulas.
However, the density of does not have a closed-form representation formula except for Gaussian (
), Cauchy (
) and Inverse Gaussian or Pearson (
) distributions.
Essentially, densities of stable processes are estimated using characteristic functions through Fourier transforms.Footnote1 The characteristic function of is obtained using its domain of attraction and the Lévy-Khinchine representation formula (see Applebaum, Citation2004) and it’s given by
(7)
(7)
Consequently, the density of is given by
(8)
(8)
Definition 3.2
Let . Its inverse
,
is defined by (see Meerschaert & Straka, Citation2013):
(9)
(9)
For , it is easy to see that the following equivalence relation holds:
(10)
(10)
The process is also interpreted as the first passage time of S. Moreover
(11)
(11)
Let denote the density function of
. Using relation (10) we deduce
which implies
(12)
(12)
We can therefore approximate by estimating the integral in (12) using the density
in (8) and its characteristic function (7).
3.1. Subordination
Let , denote standard Brownian motion and
be an inverse stable process independent of B. Then the process
is referred to as stable subordinated Brownian motion.
Definition 3.3
(Joint Probability Space) Suppose is standard Brownian motion defined on
and let
be the space of non-decreasing càdlàg processes
,
starting at zero. We define a joint product probability space
(13)
(13)
where endowed with the natural filtrations:
(14)
(14)
We further introduce a separable Hilbert space defined by(15)
(15)
to obtain a complete abstract probability space .
The first and second moments of subordinated Brownian motion follow by applying semigroup properties. Literature on semigroups and infinitesimal generators is detailed in Song and Vondraček (Citation2003) and Applebaum (Citation2004).
Definition 3.4
Suppose where
is standard Brownian motion in
. The transition density
of B is given by
(16)
(16)
and its semigroup given by
(17)
(17)
where f is a nonnegative Borel function on .
Definition 3.5
Suppose is a subordinated Brownian motion. Then we define the operator
satisfying
(18)
(18)
The operator has a transition density
defined by
(19)
(19)
Lemma 3.6
The mean and variance of are computed by
(20)
(20)
(21)
(21)
Proof
Suppose f in Definitions 3.4 and 3.5 is such that . Using (17) and (18) we immediately observe that
Equation (21) follows immediately by definition of variance of . That is
Note that for ,
exists and can be computed. If
in (20) and (21) we recover standard Brownian motion with mean 0 and variance t. In general, the k-th moment of
is given by
(22)
(22)
where and
is the Gamma function.
Lemma 3.7
The covariance of is given by
Proof
Let then
and we have
Since for all ,
has independent increments with zero mean, we have
Similarly for we have the covariance as
. Then, we write
4. Malliavin derivative in the direction of jump processes
In this section we explore the differential calculus of using directional derivatives. Little has been done on this, a few references include Kusuoka (Citation2010) and Zhang (Citation2012).
Lemma 4.1
Following Definition 3.3, we let f be an -adapted right-continuous process with left limits satisfying
(23)
(23)
Then its -martingale stochastic integral exists, it’s well defined and can be expressed as an
-martingale stochastic integral i.e.
(24)
(24)
where is the inverse stable process of
.
Proof
This follows from the standard change of time.
Following Definition 3.3, we denote by D the Malliavin derivative operator defined on such that
represents differentiation of h with respect to
. In addition,
will denote Malliavin differentiation in direction h.
Lemma 4.2
Let be subordinated Brownian motion associated with
. Then
, for all
and
. Moreover, for a Càdlàg process f we have
(25)
(25)
where is the inverse stable process of
.
Proof
It is easy to see that since by definition
(26)
(26)
for , provided the limit exists.
For the second part of the lemma we notice that since is of bounded variation, the contribution of its small jumps is almost negligible and the number of jumps is finite. We partition
as
where
are the jump times of
and let
be a partion of
. Suppose
, we have
(27)
(27)
Now if we let then
for
and as a consequence we write
Note that is constant on
. Therefore
The result follows immediately, i.e.(28)
(28)
Lemma 4.3
Suppose f is a right continuous function with left limits, then(29)
(29)
where is the inverse stable process of
.
Proof
This follows from standard change of time computations.
Lemma 4.4
Let g be an -adapted right-continuous process with left limits satisfying,
(30)
(30)
then(31)
(31)
This holds for all .
Proof
This follows a similar argument as in the previous Lemma.
4.1. Discrete multiple stochastic integral
In this section we derive the integration by parts formula associated with our choice of random process . We denote by
the time between the k-th and
-th jumps of
.
We start with the triplet , a joint probability space introduced in Definition 3.3 with a real seperable Hilbert space
, with a scalar product
and the norm for an element
, denoted by
,
is the completion of
and
is the extension to the Borel
algebra of
of a cylindrical measure. We define independent stable random variables
which are canonical projections from
to
. We assume
is a form of subordinator introduced in Janicki and Weron (Citation1993, p. 33) which is an
-stable totally skewed Lévy motion with increasing sample paths
. This is a symmetric alpha-stable process (
) with positive Poisson jumps. Therefore,
belongs to a class of
Lévy motion processes with its jumps only at the jump times of
. As a consequence, we use Charlier polynomials to define the multiple stochastic integrals with respect to our process.
Definition 4.5
The Charlier polynomials are defined as(32)
(32)
They can also be expressed explicitly as
where .
The Charlier polynomials form an orthogonal basis of with respect to the Poisson measure
. Moreover, we have:
where when
and
for
. Therefore any function
can be uniquely represented as
with the corresponding norm given by .
Next, we construct the discrete multiple stochastic integral using the Wick product following similar arguments in Privault (Citation1990).
Suppose is a set of all functionals of the form
where Q is a real polynomial and
, we regard
as an algebra generated by
and define the Wick product in the following manner.
Definition 4.6
The Wick product of two elements denoted by
is defined (relaxing
for simplicity) as:
where for ,
and
,
and
.
Let be a space of square-summable sequences. There exists a discrete chaotic decomposition of
whose elements F can each be represented as a sum of multiple stochastic integrals of kernels of
. That is,
(33)
(33)
where and
is the discrete multiple stochastic integral of symmetric functions of discrete variable.
The stochastic integral of is an isometry from
to
as follows:
Proposition 4.7
Let and define
. Then
(34)
(34)
Proof
Consider a partition where
are the jump times of
and let
be a partion for each
. Suppose
, we have
where . Consequently
We notice that for on each
, we have
which is arrived at by conditioning with respect to and applying the tower property. Meanwhile for
we have
The last equation follows from the law of total expectation. The result follows immediately by combining both cases.
The discrete multiple stochastic integral ,
symmetric in
with finite support can be defined directly using the Wick product.
Definition 4.8
The symmetric tensor product is defined as
(35)
(35)
where and
is the set of all permutations of
. Moreover, suppose
with finite supports, we have
where ,
.
The definitions above suggest the results (Privault, Citation1990, Prop. 2 and 3) for the Poisson process, also hold for our choice of process with similar proofs.
Lemma 4.9
(1) | Suppose
| ||||||||||||||||||||||
(2) | Let |
Proof
See Privault (Citation1990).
Lemma 4.10
Suppose defines chaos of order
in
given by
. Then
has a chaotic decomposition:
Moreover, if is the tensor product
, endowed with the norm
equivalent to then the Fock space
is isometrically isomorphic to
.
Proof
The ’s are orthogonal according to Lemma 4.9. Secondly, Q is dense in
since the polynomials of
are dense in
: Suppose
and
, for any
, then
. This implies
since is a discrete-time martingale. Therefore
and
is dense in
.
The annihilation operator defined in (26) has an equivalent in the discrete chaotic decomposition of given by
(36)
(36)
Moreover, the following lemma holds.
Lemma 4.11
Suppose denotes a dense set of elements of
such that
where
has finite support in
and define an operator
by
Then for any and
, we have
(37)
(37)
where Dom represents domain and the operators D and are also closable and adjoint to each other.
Proof
See Privault (Citation1990).
The above results can be extended to
yielding:
(38)
(38)
This in turn leads to the following duality relation:(39)
(39)
The duality formula is an important tool for finding alternative representations of derivatives of expectations of irregular functions. We discuss this later.
Note that for the rest of the article we shall use abbreviations SDE and SSDE to represent stochastic differential equation and subordinated stochastic differential equation respectively.
4.2. Malliavin derivative of solutions to subordinated SDEs
In the following, K is defined as a Hilbert space, as a Sobolev space of K-valued functions associated with the H-derivative,
as a total set of a K-valued linear operator of Hilbert-Schmidt class on H,
as the total set of
-predictable
-valued functions
such that for
,
with
(40)
(40)
where h is given in (15). We denote as the total set of
-predictable
-valued functions b satisfying
,
with
(41)
(41)
Let represent a joint probability space introduced in the previous sections and consider the following stochastic differential equation:
(42)
(42)
A scenario of (14) with Lipschitz coefficients and standard Brownian motion has been discussed in Fournié et al. (Citation1999) and Bayazit and Nolder (Citation2013) to compute financial Greeks. The case of non-Lipschitz coefficients with subordinated Brownian motion is handled in Bavouzet and Messaoud (Citation2006), Sun and Xie (Citation2014) and much less related in Cass and Fritz (Citation2007) and Di Nunno et al. (Citation2008). For non-Lipschitz with standard Brownian motion, (see for instance Baños et al., Citation2015). In the current paper we are interested in a model with Lipschitz coefficients and subordinated Brownian motion.
Proposition 4.12
Suppose in the stochastic differential equation (42) has Lipschitz coefficients and assume and
. Then the solution
to (42) exists, is unique and belongs to
for all
.
Proof
The proof is based on Picard’s successive approximation and it follows similar steps of (Kusuoka, Citation2010, Thm 3.1 and Prop 2.1).
Let denote the dot product endowed on
in (15). A representation of the derivative of
follows in the following proposition.
Proposition 4.13
Denote the directional derivative of by
,
where
is the Malliavin derivative operator. Then from (42) we have
(43)
(43)
If we assume is a complete orthonormal basis in H, then
(44)
(44)
Moreover, if , then
(45)
(45)
Proof
First, represent (42) in its integral form:(46)
(46)
Then apply the product rule and (26) to the second term on the RHS of (46) to obtain (43).
If is an orthogonal basis, we can express
as
(47)
(47)
where and
is a unit vector of h. For h orthonormal gives (44).
If the last term of (45) is zero and by the Grönwall’s inequality, we have:
(48)
(48)
Also note from (46) that the first variation process can be deduced as:(49)
(49)
Combining (48) and (49) results into the following useful relation(50)
(50)
Alternatively,(51)
(51)
5. BEL formula for subordinated stochastic differential equations
Bismut–Elworthy–Li formula for general Lévy processes is studied in Cass and Fritz (Citation2007). We derive representations for subordinated Brownian motion based on (Elworthy & Li, Citation1994).
Proposition 5.1
Let be the solution to (42) on the horizon
where
(see for instance Baños et al., Citation2015; Cass & Fritz, Citation2007) and let
denote some bounded function. Suppose we can define a functional
of
by
(52)
(52)
Then the derivative of V with respect to x is given by(53)
(53)
where is some bounded function satisfying
(54)
(54)
Proof
Apply the classical chain rule on and use the relation (51) followed by the chain rule in the Malliavin sense. Finally apply the duality relation (39), in that order. A similar proof is provided in Sturm (Citation2004) using the identity
, where
,
.
Equation (53) is Bismut–Elworthy–Li formula for Geometric Brownian motion.
Proposition 5.2
Suppose (46) has Lipschitz coefficients. Let denote the right inverse of
where
is elliptic. For any function
and
, we have (the x argument is relaxed for simplicity)
(55)
(55)
where(56)
(56)
Proof
Working backwards and using the results obtained above we have
Next we apply the duality relation (39), Equation (50), Grönwall’s inequality and (45) for some arbitrary h not necessarily an orthonormal basis and in that order, where we have chosen
We provide a detailed analysis on the above result in the following section.
5.1. Main results
This section presents the main results of the paper. The idea is to extend the results by Fournié et al. (Citation1999) to a subordinated stochastic differential equation model by deriving the first- and second-order derivative representation for the expectation of a function that is not necessarily regular. Specifically, the idea is to by-pass the derivative of the expected (irregular) function by introducing a weight term in form of an integral with respect to subordinated Brownian motion. The results in this section are employed in the following section to estimate the Greeks using MonteCarlo simulations.
In this section, operators and D will be used interchangeably to represent weak derivatives, and
shall denote the first variation process given by
(57)
(57)
shall denote a space of bounded integrable functions,
shall denote the semigroup of the solution
to (46). Lastly,
shall denote a non-decreasing cádlág
-stable process and
its inverse with
. We require
to be complete to enforce some integrability conditions on
.
Corollary 5.3
Let with bounded first derivative such that
and define
. Then the weak derivative of
with respect to the initial state x is given by
(58)
(58)
provided the last term exists. Moreover(59)
(59)
Since is non-explosive, the weak derivative
is well defined.
Proof
This follows directly from the application of a weak chain rule.
Corollary 5.4
Suppose is non-degenerate and elliptic, there exist an inverse
of
smooth in
such that
for all
for some
. Moreover, if
(60)
(60)
then,(61)
(61)
Proof
Recall that the result holds for the case of continuous processes (Cass and Fritz, Citation2007; Elworthy & Li, Citation1994). We can therefore employ similar arguments of partitioning as in the second part of the Proof of Lemma 4.2 and apply similar steps as in the continuous case but piece-wise, to arrive at the required result.
Theorem 5.5
Let with its first derivative bounded and continuous:
(62)
(62)
Moreover, for ,
, the derivative with respect to x is given by
(63)
(63)
where is a martingale.
Proof
From Corollary 5.4, let , applying Itô’s formula to
(64)
(64)
yields(65)
(65)
As , and applying the knowledge from Lemmas 4.3 and 4.4 yields
(66)
(66)
Multiplying (66) by a martingale yields
(67)
(67)
Since and
. The required result follows.
Corollary 5.6
Suppose and indexes
, then
(68)
(68)
and(69)
(69)
and(70)
(70)
where denotes the Euclidean norm.
Proof
The proof follows directly by applying (5) where .
Theorem 5.7
From Corollaries 5.4 and 5.6, let be such that its first and second derivatives are bounded and continuous and,
(71)
(71)
such that for almost all for
,
(72)
(72)
where(73)
(73)
Then
Proof
From Equation (72) we deduce
Suppose and
then
Applying Itô formula to at
, yields
(74)
(74)
Multiply (74) by and
. Next, taking expectations and applying the identity (see Elworthy & Li, Citation1994)
(75)
(75)
yields the required result.
6. Applications
This section is dedicated to estimating the Delta, Gamma and Vega from two stochastic models of the asset price namely; the subordinated stochastic differential equation (SSDE) and the Geometric Brownian motion (GBm).
6.1. Hedging discontinuous-pay-off type options in Black–Scholes framework
We focus only on the digital option but the analysis could be extended to other discontinuous-pay-off type or irregular pay-off options (see Fournié et al., Citation1999 for instance). The Delta and Gamma follow directly from Theorems 5.5 and 5.7 respectively.
Let satisfy the stochastic differential equation
(76)
(76)
with the solution given by(77)
(77)
Figure shows the dynamics of solutions to SSDE and GBm.
The Greeks are computed by conditioning on , as follows :
6.1.1. Delta
According to Theorem 5.5(78)
(78)
Assume a discounted pay-off of a digital option i.e. where E is the strike price, then we can express the Delta by
(79)
(79)
Figure shows the digital option delta from both the SSDE and GBm models. Observe that the delta from SSDE is slightly higher due to existence of jumps and its convergence is slightly slower.
6.1.2. Gamma
Consider and let
in Theorem 5.7 then we deduce
(80)
(80)
Suppose . We obtain a similar expression of the Gamma as in the case of continuous Brownian motion by applying the identity
and simplying. That is
(81)
(81)
Figure shows digital option gamma from the SSDE and GBm models. Again we observe that the gamma from SSDE is slightly higher than that from GBm.
6.2. Vega
The Vega can be deduced similarly using integration by parts. That is(82)
(82)
Figure shows the Vega from the SSDE and GBm models. Note that the Vega from SSDE is slightly higher. Observe that despite fact the SSDE model has jumps, the convergence rate for the estimation of the Greeks from the model is as good as in the GBm model.
As a matter of interest, we apply the finite-difference method on the subordinated Brownian motion model to estimate the Greeks for a call option from the SSDE model. Recall from Fournié et al. (Citation1999) that the finite-difference method is recommended for computing the Greeks from European options compared to the BEL formula, it performs better in this case. Figure shows the estimation of the Greeks of a European call option using the SSDE model.(83)
(83)
7. Conclusion
We extended the integration by parts formula approach to computing the Greeks of options with discontinuous pay-offs presented in Fournié et al. (Citation1999) to markets with jumps. As an application, we estimated Greeks from the SSDE model and observed that BEL formula still performs well for SSDE as in the continuous diffusion models. As a concrete practical application, our model can be applied by investors in emerging/illiquid markets to construct hedge portfolios.
8. Cover image
Source: Authors.
Additional information
Funding
Notes on contributors
M. Kateregga
M. Kateregga is a PhD student at the University of Cape Town in South Africa. His research is in the field of mathematical finance and his PhD thesis is entitled Stable Distributions with Applications in Finance. The current paper is a chapter in his thesis. He is also a researcher at the African Collaboration for Quantitative Finance and Risk Research (ACQuFRR) which is the research section of the African Institute of Financial Markets and Risk Management (AIFMRM), which delivers postgraduate education and training in financial markets, risk management and quantitative finance. Mr Kateregga also works with Mira Networks in South Africa as a Software Engineer.
Notes
1 Note that the characteristic function will always exist.
References
- Applebaum, D. (2004). Lévy processes and stochastic calculus. Cambridge studies in advanced mathematics. Cambridge: Cambridge University Press.
- Baños, D. R., Meyer-Brandis, T., Proseke, F., & Duedahl, S. (2015). Computing deltas without derivatives. Finance and Stochastics, 21, 509. doi:10.1007/s00780-016-0321-3 Preprint.
- Bavouzet, M.-P., & Messaoud, M. (2006). Computation of Greeks using Malliavin’s calculus in jump type market models. Electronic Journal of Probability, 11(10), 276–300.
- Bayazit, D., & Nolder, C. A. (2013). Malliavin calculus for Lévy markets and new sensitivities. Quantitative Finance, 13(8), 1257–1287.
- Bermin, H.-P. (2000). Hedging lookback and partial lookback options using Malliavin calculus. Applied Mathematical Finance, 7(2), 75–100.
- Cass, T. R., & Fritz, P. K. (2007). The Bismut-Elworthy-li formula for jump-diffusions and applications to Monte Carlo methods in finance. arXiv:math/0604311 [math.PR].
- Chen, Z. Q., Song, R., & Zhang, X. (2015). Stochastic flows for Lévy processes with Hölder drifts. arXiv:1501.04758v1 [math-ph].
- Di Nunno, G., Øksendal, B., & Proske, F. (2008). Malliavin calculus for Lévy processes with applications to finance. Berlin: Universitext--Springer.
- Elworthy, K. D., & Li, X.-M. (1994). Formulae for the derivatives of heat semigroups. Journal of Functional Analysis, 125, 252–286.
- Fournié, E., Lasry, J.-M., Lions, P.-L., Lebuchoux, J., & Touzi, N. (1999). Applications of Malliavin calculus to Monte Carlo methods in finance. Finance and Stochastics, 3(4), 391–412.
- Janicki, A., & Weron, A. (1993). Simulation and chaotic behavior of alpha-stable stochastic processes. Boca Raton, FL: Chapman & Hall/CRC Pure and Applied Mathematics. Taylor & Francis.
- Kateregga, M., Mataramvura, S., & Taylor, D. (2017). Parameter estimation for stable distributions with application to commodity futures log-returns. Cogent Economics & Finance, 5(1), 1318813.
- Kawai, R., & Kohatsu-Higa, A. (2010). Computation of greeks and multidimensional density estimation for asset price models with time-changed brownian motion. Applied Mathematical Finance, 17(4), 301–321.
- Khedher, A. (2012). Computation of the delta in multidimensional jump-diffusion setting with applications to stochastic volatility models. Stochastic Analysis and Applications, 30(3), 403–425.
- Kusuoka, S. (2010). Malliavin calculus for stochastic differential equations driven by subordinated Brownian motions. Kyoto Journal of Mathematics, 50(3), 469–644.
- Meerschaert, M. M., & Straka, P. (2013). Inverse stable subordinators. Mathematical Modelling of Natural Phenomena, 8(2), 1–16.
- Nualart, D. (1995). Malliavin calculus. Probability and Its applications. New York, NY: Springer-Verlag.
- Privault, N. (1990). Chaotic and variational calculus in discrete and continuous time for the posisson process. Stochastics, 51, 83–109.
- Song, R., & Vondraček, Z. (2003). Potential theory of subordinate killed Brownian motion in a domain. Probability Theory and Related Fields, 125, 578–592.
- Sturm, S. (2004). Calculation of the greeks by Malliavin calculus (Technical report Institut für Matematik, Universität Wien. Diplomarbeit zur). Vienna: Erlangung des Akademischen Grades.
- Sun, X., & Xie, Y. (2014). Smooth densities for SDEs driven by degenerate subordinated Brownian motion with state-dependent switching. arXiv:1410.5913v1 [math.PR].
- Takeuchi, A. (2010). Bismut-elworthy-li-type formulae for stochastic differential equations with jumps. Journal of Theoretical Probability, 23(2), 576–604.
- Wyłoma\‘{n}ska, A. (2012). Arithmetic Brownian motion subordinated by tempered stable and inverse tempered stable processes. arXiv:1203.0892v1 [math-ph].
- Zhang, X. (2014). Derivative formula and gradient estimate for SDEs driven by α-stable processes. arXiv:1204.2630[math.PR].