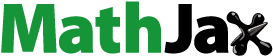
Abstract
The efficacy of four different portfolio allocation strategies is evaluated according to their absolute returns during different economic conditions over a period of 10 years. A comparison is drawn between the Most Diversified portfolio (MD) and three alternatives; a Minimum Variance portfolio, an Equally-Weighted portfolio and a Tangent (or Maximum Sharpe ratio) portfolio. The aim is to assess portfolio performance using cumulative returns, the Sharpe ratio and the daily volatilities of each portfolio. The four asset allocation methods are governed by multiple constraints. Although previous work has shown that MD portfolios exhibit greater diversification and a higher Sharpe ratio than other investment strategies, this was not found using developed market index data.
Public Interest Statement
Many investment portfolios lost substantial amounts of capital during the 2007/8 credit crisis. Some, like hedge funds, claimed to be effective “hedges” against risk because they were invested in highly diversified assets which would almost never all experience simultaneous downturns. These funds were, in fact, highly correlated with each other and their constituent assets were also strongly interrelated—hence, the substantial losses. Fund managers have subsequently worked on building alternative investment strategies, ones which would satisfy the promise of diversification, but not at the expense of diminished returns or increased volatility. The maximum diversification (MD) portfolio claims to fulfil these requirements.
This article explores whether an MD investment strategy would have been effective during the credit crisis, had it been then in place. The research shows this would not have been the case: the standard “efficient” tangent portfolio always outperforms other tested strategies.
1. Introduction
Mean–variance optimisation, developed by Markowitz (Citation1952), has become the foundation of modern finance theory. The technique considers the preferences of the investor as well as the return, risk and diversification effects, which together help to lower the overall risk of the portfolio. Markowitz’s (Citation1959) portfolio theory has been used to formulate an ex post framework of international portfolio diversification, but a flaw in this approach is that it assumes that all inputs are reliably known. These inputs (expected returns, variances and covariances) are estimated and not known with certainty. This uncertainty in the parameter values result in “estimation risk” which, in turn, often lead to suboptimal investor choices.
The financial crisis of 2008 forced practitioners to realise that existing investment strategies needed to change (Bennett & Sias, Citation2010). Hedge fund performance was generally poor: adequate protection from extreme market volatility was not realised, despite their vaunted diversification benefits via the use of different asset classes and investment strategies.
Modern portfolio theory was blamed for the suboptimal performance of many institutional portfolios during the 2008/9 financial crisis (Choueifaty, Tristan Froidure, & Reynier, Citation2011), because most portfolios were based on Markowitz’s portfolio optimisation, a scheme which would, it was widely believed, provide the requisite diversification necessary for the prevention of severely negative portfolio performance. Although the allocation method was deemed culpable, the scale of the misjudgement was also attributed to incorrect input parameters. Expected portfolio constituent returns were based on historical performance figures which overemphasised the equity component. Models also promoted assets with low historical correlations with equities, but during the crisis, the correlations of most asset classes increased and promised diversification benefits were insignificant. Alternative investment ideas were clearly needed (Jorion, Citation1992).
The Maximum Diversification (MD) portfolio, introduced by Choueifaty and Coignard (Citation2008), aims to maximise a metric which defines the degree of portfolio diversification and thereby create portfolios which have minimally correlated assets, lower risk levels and higher returns than other, “traditional” portfolio strategies. Using assets selected from developing economies, this article aims to investigate the claim that the MD portfolio’s performance is superior to three other, well-known investment strategies (namely MV, TG and EW portfolios). Component weights were calculated for each strategy and changes in these weights evaluated overtime. This analysis is of considerable interest to portfolio managers because, as prevailing economic conditions change, optimal weights adjust accordingly and shift from one industry (index) to another. These shifts alert portfolio managers to signs of changing economic conditions. Strategies that are more sensitive to the economic milieu will indicate shifts earlier than other strategies—this could provide savvy portfolio managers with a considerable advantage.
Possible reasons for concentrations during different economic conditions were also explored including the effect of the index level, return volatility and correlation (of index returns with entire portfolio returns) on portfolio weights.
The remainder of this article proceeds as follows. Section 2 presents a review of the relevant literature pertinent to the study. Section 3 discusses the data used and the methodology applied and Section 4 presents the results and provides a discussion of these results. Section 5 concludes.
2. Literature survey
Markowitz’s (Citation1952) portfolio theory asserts that a portfolio is diversified if its variance could not be reduced any further at the same level of expected return. This definition implies that a portfolio’s variance maybe used as a proxy for the fund’s diversification level. Maximum diversification was introduced by Choueifaty (Citation2006) along with the concept of a Diversification Ratio (DR). Choueifaty (Citation2006) claimed that portfolios with maximal DRs were maximally diversified and that such portfolios provided an efficient alternative to market cap-weighted portfolios. Choueifaty and Coignard’s (Citation2008) ideas are grounded in the maximisation of the portfolio’s Sharpe ratio because the mathematical properties governing the DR and MD portfolio were derived in a mean–variance framework. Choueifaty et al. (Citation2011) added the constraint to the thesis that no short selling of constituent assets was permitted; this reduced potential estimation errors and ensured positive exposure to the equity risk premium.
The MD portfolio generated the highest Sharpe ratio and highest annualised return for each period under investigation. The MD portfolio was also found to capture a bigger part of the risk premium by delivering higher (long run) returns at lower volatility levels (Choueifaty et al., Citation2011). The MD portfolio was also found to be more efficient ex post than the market benchmark, EW or MV portfolio (Choueifaty & Coignard, Citation2008). The analysis was not only applicable to the equity market: it could be adapted to other asset classes. The intention was to provide an effective alternative to other non-market-cap benchmarks.
Clarke, de Silva, and Thorley (Citation2006) showed that if asset returns increase in relation to risk, the MD portfolio is the highest Sharpe ratio portfolio on the efficient frontier. If asset returns decrease with an increase in risk, the MD portfolio is suboptimal and lies on the lower half of the frontier: further investigation is needed to exploit the low-risk anomaly considering transaction costs based on historical data (Clarke et al., Citation2006). The MD portfolio is equivalent to the risk parity portfolio when correlations are constant (Choueifaty & Coignard, Citation2008).
The MV portfolio typically suffers from high levels of concentration (Chan, Karceski, & Lakonishok, Citation1999) and is exposed to considerable estimation error, leading to high turnover and sometimes substantially different solutions when the estimation window is moved even a single step forward, if left unconstrained (Roncalli, Citation2014).
The EW portfolio is that portfolio that maximises the Herfindahl index. It has been shown that this portfolio often dominates many others in terms of risk-adjusted return and turnover (DeMiguel, Garlappi, & Uppal, Citation2009).
The TG portfolio is “optimally efficient”, defined as the portfolio which maximises the Sharpe ratio to create a portfolio with the best historical risk-adjusted performance. The capital asset pricing model combines the efficient portfolio with a risk-free asset to attain higher expected returns than those on the efficient frontier by combining the risk-free asset with the tangent portfolio containing all invested assets (Britten-Jones, Citation1999).
3. Data and methodology
The data selected were six broad equity indices from the US market (the Bond, Banking, Industrial, Biotech, REITS, NYSE TMT (technology, media and telecommunication) and Gold indices), so all were based in USD currency. These indices are themselves diversified, each comprises a minimum of 45 stocks (Statman, Citation1987). The simulation exercise involved “purchasing” the indices and holding them for the entire duration of the exercise. The portfolios were entirely passive, that is, no rebalancing occurred during the test phase.
Daily index data spanning 30 November 2006–30 December 2016 were employed to improve the granularity of the results. The period was chosen due to the significant economic fluctuations that took place during this time (the global financial crisis struck in 2008 which resulted in devastating financial impact on global markets and this period also embraces the onset and impact of the—principally European—sovereign crisis of 2011).
All data were sourced from Bloomberg and all data originated in the US. All index prices are quoted in USD, so returns are based on USD: this ensures for minimal timing differences, copious market liquidity and accurate pricing.
This analysis used index price data rather than individual stock price data. This simplified the analysis by mitigating the impact of selection and survivorship bias. An index is often recalculated and the composition of it changes overtime which excludes no-longer-active stocks and thus mitigates survivorship bias which could otherwise occur in stock selection. The index shifts and reallocates weighting to stay in line with its objectives as an index.
Four portfolio approaches were selected. These are discussed in Sections 3.1–3.4:
3.1. Equally weighted portfolio
Assume n assets, equal weights:(1)
(1)
where are the EW portfolio weights. This is the portfolio that maximises the Herfindahl index,
, defined as:
and where represents a maximally concentrated portfolio and
a fully diversified one.
3.2. Minimum variance portfolio
MV portfolio weights are calculated using a closed form equation, they are not “solved” parametrically like other portfolio weights. The mathematics governing the MV portfolio weights always generates positive weights. These are given by:(2)
(2)
where are the MV portfolio weights,
is the inverse of the n × n variance covariance matrix of relevant asset returns, 1 is a n × 1 vector of 1s.
3.3. Tangent portfolio
The unconstrained TG portfolio weights, for portfolios with no risk-free asset are:(3)
(3)
where is the vector of TG portfolio weights, and
is the n × 1 vector of the asset’s expected returns. For portfolios with a risk-free asset with return rf, the portfolio weights are:
(4)
(4)
To calculate the weights of the unconstrained TG portfolio an analytical solution is required. Excel’s Solver (or similar) must be used to derive these.
3.4. Maximum diversification portfolio
This optimisation of the MD portfolio has the following Lagrange function (Pemberton & Rau, Citation2007):
where is a Lagrange multiplier,
represents the matrix of portfolio weights to be optimised,
is the n × n variance covariance matrix of returns, and
is the n × 1 vector of asset volatilities:
L is the Lagrangian.
First-order conditions dictate that
This gives which means that
(5)
(5)
Also know that . Multiplying (5) by
gives
which gives:
(6)
(6)
Substituting into (6) into (5) gives:(7)
(7)
Using (7) and with , the diversification ratio, D, is defined as:
(8)
(8)
D maybe decomposed into its volatility-weighted correlation () and volatility-weighted concentration,
, measures.
where represents the volatility-weighted correlation of portfolios assets:
(9)
(9)
and is the volatility-weighted concentration ratio of the portfolio, with:
(10)
(10)
A fully concentrated, long-only portfolio has C = 1 (a single asset portfolio), while an equal volatility weighted portfolio has the lowest C, equal to the inverse of the number of component assets. C measures not only the concentration of portfolio weights, but also the concentration of risks; assets are weighted proportionally to their volatilities (Choueifaty et al., Citation2011).
Table summarises the weight formulae for the four approaches.
Table 1. Weight calculation summary for the four approaches
3.5. Assumptions and constraints
Investors are assumed to be rational, risk-averse market participants and there are no trade or taxation costs. Short-selling (positive and negative component weights) and long-only (positive-only component weights) are permitted for all portfolios. For short selling, (1), (2), (4) and (7) are used for the weight calculations, while for long-only portfolios, an optimisation, root-finding tool must be used such as Excel’s Solver (Gennotte, Citation1985).
4. Results and discussion
4.1. During financial crisis (2008)
During the period from 2008 to the end of 2009, there was a discrepancy of only approximately 20% between the cumulative returns of either of the allocation strategies. This is largely because these four portfolios were invested in similar indices. The TG portfolio was wholly invested in the Gold index until early August 2008. After this, it was fully invested in the Biotech index until late October 2009.
From earliest point within the data-set (14 December 2007), the TG portfolio short sold REITs. This was the start of the US housing crisis during which house prices declined precipitously. The Gold index was either shorted or invested in within a range between −14 and 12% which means it is not a significantly weighted component in the MV composition. Biotech index and NYSE TMT formed a larger component as both experienced weights more than 50% in comparison to other indices included in the analysis. Fittingly, it was long in the Gold and Biotech indices and short for REITS and the Industrial index. The long-only MD portfolio was positively weighted in the Gold, Biotech and Banking.
During the period between January 2008 and 2009 no portfolio could consistently maintain positive returns. All four allocation strategies generally exhibited negative cumulative returns more than −25%. There is a clear indication of a downward trend in cumulative returns following the onset of the crisis, with the lowest cumulative returns being seen during the early stages of 2009.
4.2. Post financial crisis (2009–2016)
The financial crisis had a devastating impact on financial markets around the world, and the data obtained reflect the poor economic performance in the US. Each asset allocation method exhibited declining returns to the investor and daily volatility was more than 1.75% in January 2009. Since then there has been a general trend of lower daily volatility levels which could indicate an improvement in market stability.
Furthermore, the returns showed lower levels of volatility after the decline in 2008–2009. Evidence suggests that there has also been an improvement in the Sharpe ratios which is in line with the belief of improved market conditions.
4.3. Diversification ratio (MD portfolio)
The principle of the DR was introduced Choueifaty and Coignard (Citation2008). The ratio will be >1 unless the portfolio consists of only a single asset. The objective of the MD portfolio is to maximise this ratio which means to maximise the ratio of the weighted average of volatilities and the portfolio’s volatility (see (8)).
4.3.1. Constrained (long-only) MD portfolio
The aim of the analysis is to maximise the DR with respect to the given constraints. The lowest observed ratio during the assessed period for the long-only MD portfolio was 1.21 which occurred during the final stages of 2007. The highest ratio of 1,651 was observed on the most recent date included in the data-set. Figure illustrates the upward trend of the Diversification Ratio (D) over the assessed period. Figure also shows the low level of volatility (0.015) of D over this period.
4.3.2. Comparison of MD portfolios (constrained vs. unconstrained)
Figure compares the D performances of the two MD portfolio variations over the observation period.
The unconstrained MD portfolio consistently outperformed the constrained alternative even though their performances were almost identical over the period. The only significant difference in performance was at the start of 2009 when the unconstrained D exceeded the constrained D by approximately 0.08. Figure does, however, indicate that the long-only constraint limits the achievable D.
Further comparison between the constrained and unconstrained MD portfolio can be done by comparing the volatility-weighted concentration ratios (C) of each portfolio as seen in (3). Intuition tells us that “concentrated” weights or strong correlation is detrimental to the efficient diversification of a portfolio. Figure shows the relationship between the constrained and unconstrained CR (note the different scales).
Important to note is the difference in axis measurement. While the unconstrained C is measured along the left y-axis and starts at zero, the constrained C is measured on the y-axis to the right and does not start at zero. This was done to more clearly illustrate the similarities in movement of both varieties.
On 9 September 2008, the initiation of the credit crisis, the mathematics behind the model failed. C for the constrained portfolio fell to zero: the market was in such a state of turbulence and market returns were so different from the previous day’s returns that the mathematics behind the model could not handle the abrupt change on that day. The only significant difference between the two varieties came about during the period between late 2011 and 2013. This coincides with the sovereign crisis, but further investigation is needed before any correlation can be drawn between these events.
Further analysis comparing the two variations of the MD portfolio involved the comparison of the DR correlation. Figure shows the results of the analysis.
Figure 4. Comparison of diversification correlations using constrained and unconstrained portfolio weights.
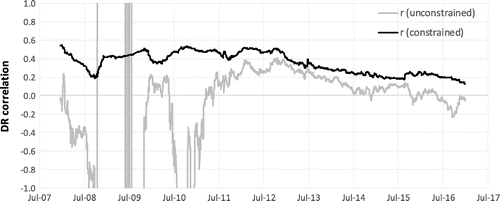
Following the disruption in September 2008, the correlation ratio returns to normal values, i.e. those observed prior to the onset of the crisis. This event had such drastic financial and economic ramifications that the market turbulence meant that the mathematics supporting the model could, as with the concentration ratio, not function properly. The constrained D concentration ratio fell to zero and the unconstrained D concentration ratio rose to zero.
The unconstrained D correlation ratio is considerably more volatile and prone to changes. Interesting to note is that these changes coincide with the dates of the credit crisis and sovereign crisis. It can also be deduced that from 2012 both D correlations tend to move along the same trend and within a similar volatility range.
The MD portfolio was calculated differently to the other three asset allocation portfolios included in the analysis. Due to the low levels of volatility shown by the Bond Index (2.79%), the model initially showed bias towards this index. It was decided to exclude the Bond index from the calculation of the MD portfolio and therefore the Bond Index has a 0.00% weighting in the composition of the MD portfolio. The omission of the Industrials index and the NYSE TMT by the model for most of the assessed period is due to the correlation level. Table shows that the correlations between the two indices is 0.88: this is the primary reason why the model has excluded these two variables.
Figure provides a graphical representation of the composition of the MD portfolio over the assessed period.
Figure 5. Portfolio weights for the maximum diversification portfolio overtime.
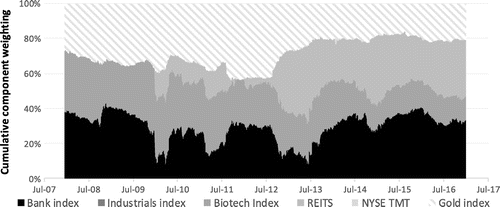
The MD portfolio favours the Bank, Biotech and Gold indices up until early 2010, at which point, the REITs is introduced significantly into the composition of the portfolio. The only unusual event would be the inclusion of the NYSE TMT for a brief period during the early stages of 2014.
4.4. Sharpe ratio
The Sharpe ratio determines the risk-adjusted return and is widely used as the industry standard. The Sharpe ratio is the quotient of the difference between the excess portfolio return (expected portfolio return () less the risk-free rate rf) and the standard deviation of the portfolio, σp.
(11)
(11)
Figure shows the Sharpe ratios over the assessed period.
The data show that, after the financial crisis in 2008, all the asset allocation methods in this analysis had negative Sharpe ratios. The interpretation of this is that there were no positive excess returns for the given level of portfolio risk. Each portfolio had positive Sharpe ratios by the end of 2009 which indicates that the market had started to recover from the disastrous events in the prior year.
There is a general market consensus that any Sharpe ratio more than three is considered “excellent” and the only portfolio which could achieve such a feat was the TG portfolio. This portfolio consistently outperformed all other alternatives from 2010 through to late 2015.
The Sharpe ratio improves over the analysis period. The four asset allocation methods all indicate negative Sharpe ratios at the onset of 2009, but these improve during that year. The EW, MV and MD portfolios all performed similarly throughout the assessed period, with the only portfolio outperforming the rest being the TG portfolio which reached a Sharpe ratio >4 during the late stages of 2013.
An important component in determining portfolio weights is the volatility of the index returns. Certain asset allocation methods tend to favour more stable indices whereas others are more interested in volatile returns. Table shows the respective volatilities for each index over the period. In this instance, the volatility is a representation of fluctuations in the price level of the index. Volatility levels will have an impact in the way in which the portfolios are constructed, with some portfolios tending towards indices with lower levels while others may seek indices with greater risk. Volatility is one way of measuring an index’s risk, with larger volatility levels being undesirable as it could indicate a degree of price instability.
Table 2. Vector of portfolio volatilities
The Bond index displayed the lowest volatility levels while the gold index was the most volatile of all indices by a significant margin. The low volatility levels displayed by the bond index are a contributing factor to why it was excluded in the calculation of the MD portfolio. If it were to be included then the model would be biased in its favour which could distort the analysis.
Figure shows the daily volatility levels of the four asset allocation methods. This information is important as volatility is a vital component in the process of portfolio composition.
Figure 7. Daily volatility for the various investment strategies. TG portfolio weights are constrained to be >0.
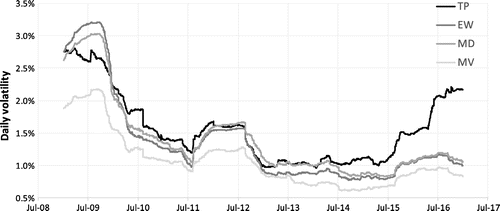
The data for daily volatility start in January 2009 and the MD portfolio has the greatest volatility levels. Even though it had relatively high daily volatility it still managed to have the highest Sharpe ratio at the time. After the financial crisis, the volatility levels all tended to reduce, except for the impact of the sovereign crisis. This downward trend indicates improved market stability: reassuring to investors and investment professionals.
The TG does not follow the trend of the other three portfolios in 2016: the increased volatility results in the Sharpe ratio of the TG being lower than the other allocation methods.
The relationship between indices is shown by the correlation between the two variables. The correlation is a fundamental consideration that portfolio managers or asset allocation strategies must take into account because of the significant impact it can have on a portfolio. Table shows the relationship between variables in the analysis.
Table 3. Correlation matrix
The interpretation of Table is important: the MD portfolio as the objective is to have a highly diversified portfolio which is diversified. The MD portfolio cannot be sufficiently diversified if it has highly correlated assets in its composition. The model thus excludes the NYSE TMT and the Industrials index due to the strong, positive correlation of 0.88 between the two variables. Evidently, there are only nine days within the entire analysis period which includes the NYSE TMT in the MD portfolio; the Industrials index on the other hand, was not included at all in calculating the MD portfolio.
These results suggest that certain asset allocation methods perform better than others in different economic conditions. Ultimately what can be said is that the MD portfolio did not portray the expected performance in terms of returns or Sharpe ratio. The discussion shows that D was inadequate due to the small data-set and high degree of correlation between indices. The analysis does, however, align with the portfolio theory that the TG portfolio provides optimal risk-adjusted returns. The TG portfolio could significantly outperform the other alternatives while still maintaining a high Sharpe ratio. The only downside to the TG portfolio in this analysis would be the increase in daily volatility during 2016, but further analysis would have to be done to establish why this occurred.
If a greater universe of data could be accessed then certain outcomes would likely be different. D ought to improve and C should decrease. Furthermore, the Sharpe ratios should improve for certain portfolios such as the TG and MD portfolios.
4.4.1. Suggestions for future work
Future analysis could focus on individual stocks as opposed to indices. This would give a wider scope for diversification and allow for better portfolio performance. Another alteration would be to increase the universe of stocks in the analysis. This would allow for improved returns, diversification ratio, concentration ratio and Sharpe ratio, as well as a more accurate outcome in general as the use of indices may result in a slight distortion of the outcome. Further analysis would include any transaction costs associated with investing in a specific stock as this better reflects real market conditions and can then provide the investor with a cost-adjusted return figure.
Figure shows the cumulative returns of all investment strategies with the long-only constraint applied to the TG portfolio.
All investment strategies displayed negative returns more than 30% between 2008 and 2009. Since then the MV, MD portfolio and EW portfolio allocation methods have had less volatility with respect to their returns. The TG portfolio, however, could attain positive cumulative returns by late 2010 and has subsequently gone on to achieve cumulative returns more than 200% at times. The TG portfolio significantly outperformed the other allocation methods by over 100% from 2014 onwards.
4.5. Unconstrained TG portfolio
The unconstrained TG portfolio was introduced to the analysis to establish whether the “optimal” portfolio could yield superior results to those from the constrained TG portfolio. Due to the reasons highlighted in the Data & Methodology section of the analysis, the unconstrained TG portfolio may not be applicable in practice yet, but the results warranted further discussion.
Figure shows the cumulative returns of the unconstrained TG compared to the alternatives over the period.
Figure shows the unconstrained TG portfolio outperforms the other allocation methods in terms of cumulative returns by over 2,500% over the period. This has been achieved while maintaining acceptable volatility levels relative to the other alternatives. Even if transaction costs were to be imposed, the portfolio would still perform significantly better than others as those costs are imposed on each portfolio. The cumulative transaction costs are also unlikely to offset returns. This formidable cumulative return was also achieved using 400% leverage (four times), which is not uncommon in market practices today (2018).
Figure highlights the Sharpe ratio performance of the unconstrained TG portfolio.
Figure shows how the unconstrained TG portfolio had a Sharpe ratio of approximately −0.3 after the financial crisis while the alternatives had Sharpe ratios >−0.5. The unconstrained TG portfolio could achieve Sharpe ratios >5 on multiple occasions in 2012 and 2013. This portfolio sustained Sharpe ratios >4 for 10 months (mid-2013–mid-2014).
Figure is a graphical representation of the daily volatility levels.
The unconstrained volatility is five times the size of other portfolio volatilities following the aftermath of the crisis and then drops to similar level in 2011–2016; except for an elevated period from mid-2012 to mid-2013, during the European sovereign crisis. At all times, the TG portfolio volatility was greater than all other strategies’ volatility. Unconstrained TG portfolio weights elevate portfolio risk, but not at the expense of diminished returns. TG portfolios achieve considerable return outperformance over other investment strategies even when accompanied by inflated daily volatility levels.
5. Conclusions and suggestions
The portfolios’ performance varied considerably. The TG portfolio outperformed the other portfolios in terms of cumulative returns for most of the period. The unconstrained TG portfolio displayed considerable returns of over 2,500%. The portfolio with the lowest cumulative returns was the MV portfolio, which also exhibited the lowest daily volatility levels. The MD portfolio underperformed as assets could not be diversified as effectively as required and thus lacked diversification benefits. Table shows that few assets have ≈ 0 correlations.
The best performing portfolio was the TG portfolio: it is the only risk-based portfolio which consistently yielded positive returns as is evident from Figures and . Not only did this portfolio outperform with respect to returns, but it also maintained relatively low daily volatility levels and a positive Sharpe ratio. The worst-performing portfolio was the MV portfolio. It exhibited negative cumulative returns for the entire assessed period and performed poorly with respect to its Sharpe ratio. This portfolio displayed the lowest daily volatility.
During the early stages following the financial crisis, the MD portfolio had the highest, albeit negative, Sharpe ratio and its cumulative returns were relatively stable around 0%. This gives us an indication that during this disastrous financial period the MD portfolio could negate many of the exogenous shocks which impacted the market at that time.
The outcome of this analysis highlights the nature of the diversification problem. The difficulty lies in accessing a large enough universe of stocks and then ensuring that these data are cleaned effectively. Furthermore, this only ensures that the MD portfolio outcome conforms to the theoretical assumptions and is not applicable to the “real” world due to its constraints. Calculating all hidden and transaction costs will involve considerable enterprise. Future work could also involve accurate forecasting of market prices and deriving what would be the future MD portfolio and which stocks would be included in such a portfolio.
Further analysis of the MD portfolio could incorporate more complete data from a wider universe. The larger universe will allow for more scope in terms of diversification benefits as well as the portrayal of a better view of the principle.
The future manipulation of the given set of constraints and assumptions is also an avenue for exploration. Relaxing the long-only constraint with a large data-set could yield interesting results as the short-selling of assets may prove to be advantageous to the portfolio.
Incorporating the concept introduced by Statman (Citation1987) could be useful if the data-set is large enough. The correct data-set would allow the fundamental assumption of no transfer costs to be relaxed. If the data-set were to be informative enough, further investigation could study transfer costs associated with each asset included. This information would allow investors to select the correct assets/stocks while considering their associated costs. These costs would accumulate with an increase in the number of constituent portfolio stocks, and this total would then be deducted from the returns to give real returns for the investor.
Extensive back testing of the analysis should be conducted to confirm the validity of the results. Back testing ensures the viability of the relevant trading strategies and enable those strategies to be optimised prior to the investor risking any capital.
Accounting for timing differences between global stocks could be investigated. These could give rise to biases or inaccurate portfolio choices. Additionally, the data could be analysed and tested to ensure that no survivorship or selection bias has entered the model.
Measuring the MD portfolio against other quantitative measures such as the risk parity approach, or an adapted version of it could also yield interesting results. The risk parity approach has become popular in recent times, but questions have been raised surrounding its applicability moving forward if stable market conditions are not maintained. Another measure to which the MD portfolio can be compared is the Inverse Volatility portfolio which may also lead to a well-diversified portfolio (Jensen, Citation2013).
Ultimately these new conditions will allow the investor to have an MD portfolio under the condition of that portfolio being optimal. There would be no real benefit in discovering the MD portfolio if it were not optimal for one to do so. Theoretically one could have an infinite amount of stocks in a portfolio, but in the real world, the associated costs would outweigh the benefits of such a portfolio. The real goal is thus to have an optimally diversified portfolio which incorporates and reflects real market conditions.
Funding
The authors received no direct funding for this research.
Additional information
Notes on contributors
Ludan Theron
Ludan Theron is a postgraduate student (master’s in risk management) at the School of Economics, North-West University, Potchefstroom campus in South Africa. He recently completed his honours degree at the University of Cape Town, South Africa, in Financial Analysis and Portfolio Management.
Gary van Vuuren
Gary van Vuuren is a professor of risk management at the School of Economics, North-West University, Potchefstroom campus in South Africa. He has worked for retail and investment banks and now consults for the ECB.
References
- Bennett, J. A., & Sias, R. W. (2010). Portfolio diversification. Retrieved January 22, 2017, from SSRN https://ssrn.com/abstract=728585
- Britten-Jones, M. (1999). The sampling error in estimates of mean-variance efficient portfolio weights. The Journal of Finance, 54(2), 655–671.10.1111/0022-1082.00120
- Chan, L. K. C., Karceski, J., & Lakonishok, J. (1999). On portfolio optimization: Forecasting co-variances and choosing the risk model. Review of Financial Studies, 12(5), 937–974.10.1093/rfs/12.5.937
- Choueifaty, Y. (2006). Methods and systems for providing an anti-benchmark portfolio. Patent number: USPTO 60/816, 276. Retrieved from https://www.google.com/patents/US7958038.
- Choueifaty, Y., & Coignard, Y. (2008). Toward maximum diversification. The Journal of Portfolio Management, 35(1), 40–51.10.3905/JPM.2008.35.1.40
- Choueifaty, Y., Tristan Froidure, T., & Reynier, J. (2011). Properties of the most diversified portfolio. Journal of Investment Strategies, 2(2), 49–70.
- Clarke, R., de Silva, H., & Thorley, S. (2006). Minimum-variance portfolios in the US equity market. The Journal of Portfolio Management, 33(1), 10–24.10.3905/jpm.2006.661366
- DeMiguel, V., Garlappi, L., & Uppal, R. (2009). Optimal versus naïve diversification: How in-efficient is the 1/N portfolio strategy? Review of Financial Studies, 22(5), 1915–1953.10.1093/rfs/hhm075
- Gennotte, G. (1985). Optimal portfolio choice under incomplete information. The Journal of Finance, 41(3), 733–746.
- Jensen, J. B. (2013). Risk parity approach to asset allocation, Copenhagen Busi-ness School ( MSc thesis). Retrieved from http://studenttheses.cbs.dk/bitstream/handle/10417/4819/jacob_buhl_jensen.pdf?sequence=1
- Jorion, P. (1992). Portfolio optimization in practice. Financial Analysts Journal, 48(1), 68–74.10.2469/faj.v48.n1.68
- Markowitz, H. (1952). Portfolio selection. The Journal of Finance, 7(1), 77–91.
- Markowitz, H. (1959). Portfolio selection: Efficient diversification of investments (p. 345). New York, NY: John Wiley & Sons, Inc.
- Pemberton, M., & Rau, N. (2007). Mathematics for economists: An introductory textbook. Manchester, NH: Manchester University Press.
- Roncalli, T. (2014). Introduction to risk parity and budgeting (Chapman & Hall/CRC Financial Mathematics Series). Abingdon: Taylor & Francis Group.
- Statman, M. (1987). How many stocks make a diversified portfolio? The Journal of Financial and Quantitative Analysis, 22(3), 353–363.10.2307/2330969