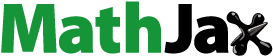
Abstract
This paper argues that the link between poverty and drug-related crime might be spurious. We take an empirical approach to investigate the causality and plausibility of this link. Firstly, we regress crime against envisaged explanatory variables in order to estimate the contribution of poverty to crime. Secondly, data is analysed using an ARDL ECM. The quarterly sample data for our estimation is for the period 1995–2016. We found a strong association between crime and poverty both in the short and long run. We recommend the government should focus on none income linked factors in dealing with the scourge of drug-related crime. As demonstrated in this study, various drug-related crimes are driven by socio-economic factors.
PUBLIC INTEREST STATEMENT
Over 50 percent of South Africans live in poverty, according to the Poverty Trends Report. This is way below the national poverty live of ZAR992 per month. Meanwhile, statistics show that 1 out of every 5 adults abuse substances, with codeine, alcohol and dagga the worst offenders. This work empirically evaluates the effects of drug-related crimes on poverty and vice versa. The study finds plausible results. Firstly, there is a strong association between drug-related crimes and poverty both in the short and long run. As demonstrated in this study, various drug-related crimes are driven by socio-economic factors. This study is worth noting because it is a pioneer in revealing linkages between drug-related crimes and poverty in the post-apartheid South Africa.
1. Introduction
South Africa is dogged by three social evils, namely, high crime rate, inequality and poverty. There have been claims that poverty promotes criminal activity; however, there is little evidence to back that claim. While crime has a direct impact on discouraging investment, it remains a challenge on how it is influenced by poverty. This paper examines if crime and poverty are linked.
Previous studies (Bourguignon, Citation2000; Fajnzylber, Citation2002) on crime and poverty have failed to provide compelling reasons for the link between the two. The reason being that poverty is multidimensional, while crime is caused by a number of factors not linked to poverty. For instance, studies (Chen & Yi, Citation2009; Hu, Hu, & Xu, Citation2005) conducted in China found that the crime rate increases substantially along with economic growth. Bourguignon (Citation1999) pointed out that property crime and violence activities were partly a consequence of excessive inequality and poverty. While factors causing poverty may be the same factors contributing to inequality, literature is divided in terms of the impact of these factors on crime. The cost of crime has damaging effects on the society leading to poor social integration and substantial amounts spent by governments in reducing it. These costs include prisons, courts, healthcare and private security expenditure. Yet the indirect costs include a decline in national productivity, employment and human capital (Fajnzylber, Lederman, & Loayza, Citation2000).
Only a few studies have been done to test the relationship between crime and poverty due to a lack of data in a number of African countries. Currently, the major conclusion from a number of studies is that poverty has little effect on crime (Ludwig, Duncan, & Hirschfield, Citation2001; Morgan, Citation2000; Oreopoulos, Citation2003). Fortunately, all these studies were done in developed countries where poverty is very minimum. Meanwhile, a study conducted by Fajnzylber et al. (Citation2000) on developing countries found that crime rates are related to growth, and poverty is driven by demographic factors. Similarly, Demombynes and Özler (Citation2005) noted that inequality is correlated with violent crime and property crime. Meanwhile, Drèze and Reetika (Citation2000) pointed out that murder rates in India were not related to poverty. Turning to Africa, Fafchamps and Moser (Citation2003) find that crime is higher in isolated, less populated places and in non-urban areas. This finding suggests that the geographic terrain found in certain countries exhibits a different outcome with regard to crime. Consequently, pointing out that in Africa, the geographic location of people has a direct impact on crime. Such a scenario is expected in South Africa as well, considering that most crimes occur outside urban areas.
We use quarterly data from 1995 to 2016 to examine the linkage between the two social evils, namely, crime and poverty. The observation that crime comes from economic segregation needs to be tested. Therefore, this paper argues that youth in poor areas have been the most affected by hardships—as a result, their anger is revealed by an increase in crime. It further argues that neglecting of poverty of unemployed youth has contributed to a rise in crime. Webster and Kingston (Citation2014) recorded that there is a relationship between youth poverty and policy changes in welfare, housing and employment. Subsequently, there is a view that those who live in poverty are more likely to be the victims of crime and offend.
South Africa has undergone numerous policy changes since the democratisation in 1994, most policies were targeted at redressing previous harms that were created by the apartheid system. Despite these policies, crime has continued to rise with poverty showing its impact on the youth. South Africa slipped from the 122nd position in 2014 to 136th in 2015 in the world in terms of crimes and ranks 37th of the 44 sub-Saharan nations. The Institute of Economic and Peace (Citation2014) pointed out that South Africa ranks as the 15th worst country in terms of societal safety and security, and the 8th most violent with a murder rate of 34 per 100,000 people. It was further pointed out that conflicts in South Africa were driven by poverty, inequality and slow pace in reform by the government, which in turn fuels crime and political confrontation.
This paper contributes to the literature by examining the relationship between drug-related crime and poverty. To the best of our knowledge, this is a first attempt to study this relationship in South Africa. We focus on South Africa as it represents a unique case among African countries. The country is one of the most unequal in the world and has high crime rates. While positive strides have been made in reducing poverty, the Gini index remains stubbornly high at over 0.65 in 2017 compared to 0.63 in 2015, pointing out that inequality has increased. Therefore, the availability of crime and poverty data makes it easier to follow its trends.
The rest of the paper is organised as follows. Section 2 outlines the theories that link poverty and crime and discusses empirical studies. The economic specification of the equations to be tested is given in Section 3. Section 4 discusses the regression results, and the last section concludes the study.
2. Theoretical framework
Economic determinants of crime and poverty may be considered too empirical; however, without some theoretical perspective, a little can be said about their impact. As a result, the theoretical need arises because of the lack of clarity in terms of the relationship among the stated factors. While at prima facie inequality may seem to be the driving factor between crime and poverty, the effect is not clear as shown by a number of studies that would be mentioned in the coming sections. Another concern that justifies the use of a theoretical perspective is the types of crimes committed under substance abuse or alcohol, drug dealing in large cities in developing countries at most, and violence. Data scarcity in developing countries makes it harder to estimate the linkage between the crime and poverty and even comparisons across countries. While this is a challenge for policy, the available data points to the fact that there is a possibility that inequality and poverty may have a significant positive effect on criminality. At the same time estimates indicate that the cost of crime is substantially high in countries with high levels of criminality Thus, our point of departure starts with understanding the effect of inequality on poverty and crime.
2.1. Economic theory
The linkage between crime and inequality is stressed by three ecological crime theories, namely, Shaw and McKay social disorganisation theory, Becker’s economic theory of crime and the strain theory by Merton. Becker’s (Citation1968) economic theory of crime points out that individuals allocate time between market and criminal activity by comparing the expected return from each crime, and taking into account the severity of punishment. In this case, inequality leads to crime by placing low-income individuals, who have low returns from market activity, in proximity to high-income individuals, who have things worth taking. This leads to low-income individuals committing a crime in order to improve their economic situation.
The strain theory (Merton, Citation1983) points out that individuals who are low in the social structure are frustrated by their failures to attain material attributes of success; this failure is manifested when surrounded by successful individuals. The theory states that the higher the inequality, so is the strain; in other words, individuals placed next to successful individuals feel frustrated at their situation. As inequality broadens, so is the strain in such individuals and the greater the likelihood of them committing a crime. As a result, they commit a crime in response. This is more common when it is income inequality.
According to Shaw and McKay (Citation1942), poverty, ethnic heterogeneity and residential mobility weaken social controls and undermine the willingness of communities to control their members. This situation can be exacerbated by a lack of family stability (Sampson, Citation1987). Of concern is that when social controls are undermined, it becomes harder to control crime.
These three theories are complementary in that they focus on different aspects of individuals; yet, the goal is to explain why certain individuals commit crime because of their social status. The strain theory focuses on pressures to commit crimes; the social disorganisation theory considers social deterrents to crime, and the economic theory is concerned with the incentives to commit a crime and the determent of the justice system.
Extensive literature review addresses the relationship between crime and numerous economic deprivations such as inequality, poverty and unemployment. The forthcoming section does not attempt to provide an extensive literature review on the current subject under study, but provide a basis for a proper understanding of the current problem under investigation. It is important to stress that the objective of the study differs in many aspects of existing literature on crime and poverty. Most existing literature focuses on the linkage between crime and poverty without considering the income status of the victims. While such approaches may seem direct, they do not provide policymakers with solutions on how to deal with crime caused by inequality in a certain area. Consequently, this study diverts from the norm and considers variables such as the Gini index and GDP, so as to understand how they influence drug-related crime. We strongly suspect that inequality can influence crime when there is economic growth in a certain area.
This we demonstrate by using a hypothetical example: there is a sudden rise in economic growth in a certain province, suggesting that people’s income per capita may arise in relation to this change. Suddenly people find themselves with extra income, something that was previously hard to achieve. Some may increase their saving power, while others may find it easy to spend it on gambling and partying. Such a situation increases their risk of buying drugs and drunk driving. Given that the economic growth cannot be sustained for a long time, those who were dissaving may find themselves with little to spend in maintaining their previous lifestyle. As their assets base is eroded, there is a likelihood that they may find it easy to do illegal things as a means of survival and sustaining their extravagant lifestyle. This can be explained using Friedman’s (Citation1957) permanent income hypothesis. We consider an individual who lives for T periods, and whose lifetime utility is as follows:
where is consumption in period t, and u (*) is the utility function. Assuming the individual has a wealth function of
and labour income of
in the T periods of his or her life. The individual is subject to the constraint that any outstanding debt must be repaid at the end of his or her life. Furthermore, the individual can borrow or save at an exogenous interest rate. Given that we assume zero interest rate, the individual budget constraint is as follows:
The individual, therefore, satisfies the budget constraint with equality if the marginal utility of consumption is positive. The Lagrangian maximisation problem is as follows:
where the first order condition of is
If Equation 4 holds in every period, the marginal utility from consumption is constant. Therefore, the level of consumption needs to be constant. We assume , and we put this into the budget constraint to form
for all t.
Equation 5 states that the individual divides his or her lifetime resources equally in each period of life. The term in parenthesis is the individual’s total lifetime resources. The distinction between permanent and transitory income is important in instances where the individual gains a windfall of amount M in his or her first period of life. This raises the current income by M and permanent income by M/T. Therefore, if the individual’s horizon is long, the impact of the windfall will be small. For instance, a temporary tax cut has little impact on consumption. The implication in our analysis is that the time pattern of income is not important to consumption but to saving. Thus, the individual’s saving in period t is the difference between income and consumption . When transitory income is high, so is the saving. On the contrary, when the current income is less than the permanent income, saving is negative.
Demombynes and Özler (Citation2002) found evidence in South Africa that consumption inequality within and between neighbourhoods led to higher crime rates. Similarly, Bourguignon (Citation2001) pointed out that higher poverty and inequality promote crimes. Our standpoint is viewed from Hirschman’s (Citation1973) “tunnel effect” that draws attention to the impact of inequality and growth in the following ways:
In the early stages of growth and development there is a high tolerance for inequalities.
This tolerance erodes over time if low-income groups fail to benefit from the growth process.
Therefore, in the long run, persistent inequalities are likely to lead to internal tensions caused by inequality.
According to Hirschman, individuals assess their individual welfare by comparing their own income to others. In other words, the poor, disregarding their gains in real income, will feel deprived. This may lead to the disgruntled poor individuals resorting to other means of improving their income status, and this includes both legal and illegal activities. The author points out that it is unclear how low-income people are more likely to be addicted to substance; however, they are more likely to break the law while under influence.
3. Overview of crime determinants
Webster and Kingston (Citation2014), focusing on post-war economic inequality and poverty in Britain, found that crime corresponded with long-term patterns of change in the national rate of property crime. For instance, during the 1980s and early 1990s, the rate of property crime increased coinciding with high levels of inequality and unemployment. Similarly, the same authors found that crime and poverty increased after the 2008 recession, but this time it was not property crime. They witnessed an upsurge in violent crimes as well. This finding is consistent with recent studies on the similar topic, who noted that poverty went hand in hand with violent crimes.
While studies have shown that there is a link between crime and poverty, this relationship does not occur simultaneously, but sequentially. For instance, the witnessing of violent crimes does not simultaneously occur with high poverty rates or inequality. We note that most studies on crime, inequality and poverty rely on cross-sectional analysis, more commonly focusing on American states, dating back to 1980s (see, Bailey, Citation1984; Glaeser, Sacerdote, & Scheinkman, Citation1996). Some of the studies (Land, McCall, & Cohen, Citation1990; Sampson, Citation1985) find a positive relationship between crime and inequality. Farrall and Jennings (Citation2012) find that rising unemployment and inequality correspond to long-term patterns of change in the national rate of property crime, suggesting that poverty and inequality contribute to criminal behaviour. Metz and Burdina (Citation2016) find that the wider the median income gap between the poorest neighbouring block group, the greater the property crime in richer block groups. Nonetheless, empirical evidence has found an ambiguous direction of causality (Pridemore, Citation2011). Yet, some studies like (Bailey, Citation1984) find no link between these two. Wikstrom and Butterworth (Citation2013) find a weak link between socio-economic status and crime between parents’ income and children’s offending. Nonetheless, Bjerk (Citation2007) finds a strong link between crime and socio-economic status. The opposing view on this linkage seems relatively determined by the study area largely. However, Valdez (Citation2007) points out that the causality between poverty and crime involves a complex interrelationship among the community and individual variables. Clearly, literature is inconsistent with regard to the causality between crime and poverty.
For instance, Jarjoura, Triplett, and Brinker (Citation2002) posit that the longer a young person lives in poverty the more likely would they engage in violent behaviour. In this study, we also hold a similar view with regard to South African crimes. Lack of personal growth and employment opportunities force individuals to commit criminal activities as a means of survival. Raphael and Winter‐Ebmer (Citation2001) indicated that there is a strong relationship between unemployment and crime. Similarly, a number of drug-related crimes are also committed by people who are unemployed and find solace in drugs. SAPS (Citation2016) pointed out that most drug-related crimes in South Africa were committed by uneducated youths. Jarjoura et al. (Citation2002) also noted that other factors influencing criminality included the education status of the individual, and parent occupation added more strain on such individuals.
Some studies (McMillan, Enns, Asmundson, & Sareen, Citation2010; Sareen, Afifi, McMillan, & Asmundson, Citation2011) point out that income level is linked to substance abuse. Similarly, children of parents in the lowest income decile are said to have a high propensity to commit crime compared with their peers in the fifth decile. Yet, on the other hand, behaviourists have noted violent offending and substance abuse are greatly influenced by genetics, although family environmental factors come into play (Sariasian et al., Citation2013). When viewed holistically, the relationship between violence and poverty holds in various crimes including domestic violence, murder and assault (Pridemore, Citation2011). Hipp and Yates (Citation2011) find that the relationship between crime and poverty is not always direct, instead, crime follows poverty. This is a norm in numerous studies focusing on crime and poverty; as it was stated before, the link is very unclear.
In certain instances, the linkage between crime and poverty is very complex. Kaylen and Pridemore (Citation2013) found that poverty effects led to a strong poverty-homicide relationship, while the homicide-inequality relationship was weak. Given the variations in terms of crime determinants, it becomes a bit clear that certain factors, which were overlooked by numerous studies, also contribute to making this relationship complex. For example, Hällsten, Szulkin, and Sarnecki (Citation2013) study in Sweden on immigrants’ children concluded that ethnicity and culture were unlikely to be the cause of crime, but, instead, socio-economic resources, and neighbourhood segregation—which expresses itself as an economic disadvantage—were major contributors. Such views are held by a number of studies (Lee, Citation2010; Valdez, Citation2007), who claim that people living in poor neighbourhoods were likely to be involved in criminal activities, especially when there are economically disadvantaged. Hayden (Citation2007) pointed out that the youth offending in the United Kingdom were from social housing. In other words, those youths staying in low-income neighbourhoods were more likely to commit crime than youth in non-low-income areas. While this finding may seem distant, the reality is that income status pushes youth to commit a crime in certain areas; yet, economic segregation plays a role in some areas.
4. Data
We use annual data from 1995 to 2016. The crime data is extracted from the South African Police Service database, which comprises numerous crimes ranging from robbery, violent crimes, murder and property theft. The Gini Index is extracted from the World Bank Database; Poverty headcount ratio from Quantec database; and GDP from the World Bank Poverty database. We omit the deterrent effect of police expenditure on crime, intuitively; areas with high crime rates are usually apportioned high expenditure. In terms of demographics, we note that most people who commit a crime, especially in South Africa, are males; as a result, we omit this variable. Yet, people in poverty are mostly children, single mothers and old people who have limited means to resort to crime as a mechanism to move out of poverty. Furthermore, most black Africans are in poverty, as a result, we expect them to be contributing a sizeable amount of offenders. Consequently, we exclude a number of demographic variables in our regression estimation. We focus on variables of interest, namely, Gini Index, GDP and Poverty Headcount ratio.
5. Model choice
Numerous studies on crime and poverty or inequality employ the ordinary least square (OLS) estimation. However, there is no clear justification except that the regression model to be estimated is assumed to be lognormal. Some applications of the OLS ignore the feedback effect that can be caused by the determinants of crime. Approaches such as Error Correction Models allow estimation of co-integrated variables, in the process permitting dynamics to be modelled. Recent studies have focused on using the Fixed Effects and Random Effects Models to control certain estimators. While on the other hand, some use the GMM estimator. Verbeek (Citation2008) highlighted the limitations of the model especially when there is over instrumentation of the control variables. Nonetheless, there is no perfect method; it all depends on which one provides plausible results in order to answer the objectives set forthwith. Fajnzylber (Citation2002) used a GMM estimator because of the assumption that if crime is among low-income individuals, possible crime positively affects inequality. Hauner, Kutan, and Spivey (Citation2012), while estimating inequality and crime in Russia also took a similar route. The model was based on its power of lagged differences on explanatory variables. In this case, Hauner et al. (Citation2012) implement GMM finite-sample SE correction because the GMM SEs are biased downwards.
However, we follow Pesaran, Shin, and Smith (Citation2001) in estimating an autoregressive distributed lag model (ARDL) because of numerous advantages. For example, the ARDL overcomes the challenges faced by traditional models in estimating the different order of integration such as purely I(0), I(1), and so on. Haug (Citation2002) points out that the ARDL approach is more suitable for small samples and provides long and short-run parameters simultaneously. This is a shift from the basic approaches in testing cointegration (see Engle & Granger, Citation1987) and the system-reduced ranks (Johansen, Citation1995). Pesaran et al. (Citation2001) point out that the ARDL follows a generalised Dickey-Fuller regression to test the lagged levels of variables under consideration in a conditional unrestricted correction model. Furthermore, the model has shown that the asymptotic distributions of statistics are nonstandard under the null hypothesis (no relationship exists in levels between variables) when the included variables of purely order I(0)or l(1) or mutually co-integrated. Therefore, the model uses a critical bound test, where, if the F statistic that falls outside the critical value bounds testing procedure, it can be inferenced without needing to know integration/cointegration of regressors. Based on our small sample size, our parsimonious conditional ARDL model takes the following form:
where is crime rate (Crime Ratio per 100,000 of the population) and in time period t,
is the measure of inequality using the Gini coefficient,
represent poverty measures (poverty headcount),
represents the per cent of GDP (Percent of GDP),
is the idiosyncratic error term.
Drugs are related to crime in various ways. In a direct way, it is a crime to possess, manufacture and distribute any form of drugs. Marijuana, cocaine, heroin and amphetamines are some drugs which are illegal. On the other hand, drugs are related to crime through the effects they have on the end user or generating any other illegal activity. The following is a summary list of the relationship between drugs and crime.
6. Results and discussions
The selected model choice does not require stationarity in time series since cointegration among variables can be tested using the Wald test and the F-test. However, to know the order of our variables, we performed the ADF test to the first difference of the variables, and the variables were of order 1(0) and l(1) as shown in Table . This suggested that we can continue with the ARDL model since the only situation that would affect the model estimation would be if the variables were of order l(2) variables.
Table 1. Unit root test (augmented Dickey-Fuller test)
First, we conduct an F statistic in order to test the null hypothesis that states that there is no level relationship. The null hypothesis is rejected on the basis that the F statistic (5.32) is over the critical value at all levels. This is also confirmed by the t-bounds tests, which test the lagged value on the dependent variable. Thus, we can conclude that a long run relationship exists, or there is cointegration among variables. Having established that there are possible cointegration relation inbound tests, we then perform an ARDL-ECM approach to estimate the level of relationships among variables. The selected ARDL model is 6,1,0,6.
Figure reveals, using the Hannan-Quinn criteria, the procedure selected an ARDL (6, 1, 0, 1). The selected model ARDL (6, 1, 0, 1) showed its relative superiority over other selected models using the Hannan-Quinn Criteria. It was also slightly better than an ARDL model (6, 1, 0, 2) as shown in Figure . However, all the top two models use six lag of the dependent variable.
The short-run estimates of the ARDL in Table show that lagged dependent variable LOG(Y) is negative and significant at 1% level. Meanwhile, the LOG(GINI) was also negative and a significant at 1% level. The negative coefficient (−0.02) means that a decline in the Gini index by 1% will lead to a decline in drug crime by 0.02%. Likewise, the LOG (POV) lagged in period one has a positive relationship with drug-related crimes by 0.10%. The findings are in line with Fafchamps and Minten (Citation2012) study, which found that poverty, has a positive effect on criminal activities besides crop theft. However, based on the literature review, we found that living in poverty increases someone’s chances of being involved in criminal activity. On the other hand, LOG (GDP) has a positive coefficient and significant at 1% level.
Table 2. ARDL long run form and Bounds Test.
Using the Hannan-Quinn criteria, a maximum of 8 lags of both the dependent and regressors were selected. Out of the possible 5832 models evaluated, the procedure selected an ARDL(6,1,0,6) model-6 lags of the dependent variable LOG(Y), the single lag of the LOG(GINI) and 6 lags of the regressor LOG(POV). Each of the lagged dependent variables LOG(Y(−1)), LOG(Y(−2)) LOG(Y(−4)) LOG(Y(−5)) LOG(Y(−6)) and regressors LOG(GINI(−1)), LOG(GDP) LOG(POV) LOG(POV(−1)) LOG(POV(−4)) LOG(POV(−5)) and LOG(POV(−6)) were significant, and that the coefficient of the lag of dependent variable of LOG (Y(−1), and (−5) were quite high, over 1.
The levels equation (Table ) reveals the long run relationship between variables. We find that LOG(POV) and LOG(GDP) is linked to increases in drug-related crimes in the long run. Meanwhile, LOG(GINI) has a negative relationship with the dependent variable in the long run. The ECM model equation is shown as follows:
Table 3. Long run regression results
EC = LOG(Y)—(−0.1616*LOG(GINI) + 0.3943*LOG(GDP) + 0.7808*LOG(POV) + 5.8463)
The positive coefficients between LOG(POV) and LOG(GDP) suggest a positive elasticity among those variables in the long run. When LOG(POV) increases by 1%, drug-related crimes increase by 0.79%. Meanwhile, a similar increase in LOG(GDP) is expected to lead to 0.39% elasticity in drug-related crimes. We point out two possibilities on how this unfolds in an economy. If GDP increases this leads to a rise in per capita income, and if individuals’ purchasing power is enhanced, they may increase consuming non-essential products since their capacity to save has increased. Likewise, the poverty headcount ratio increases because of other factors, either exogenous or endogenous; individuals may try to survive by resorting to drugs. This may be a survival strategy considering that drugs are usually easy to sell and addictive to victims. Therefore, considering that the drug-related crimes have been rising at an alarming rate in South Africa, one possible explanation may be that since unemployment is high in South Africa, people are trading drugs to survive.
The validity of the results is confirmed by the F-test and t-test as shown in Table . The null hypothesis of no level relationship is not rejected for both tests. We see that the F-statistic for the Bounds Test is 5.32, and this clearly exceeds even the 1% critical value for the upper bound. Accordingly, we strongly reject the hypothesis of “No Levels Relationship”.
Table 4. F bound tests
We then examine the stability of the short-run and long run coefficients by performing the CUSUM stability tests for ECM. The tests reveal that there is no instability of the coefficients because the plots of the CUSUM statistics lie within the 5% critical bounds of parameter stability (Figure ).
7. Diagnostic and stability tests
Table summarises the results of various stability tests. The validity of the results is always dependent on the stability of the model. According to the Ljung-Box Q statistics, there is no autocorrelation. The model passes all heteroscedasticity tests, implying that there is no heteroscedasticity.
Table 5. Stability diagnostics checks
8. Discussions
The effect of poverty on drug-related crime was present in both the short and long run. Sariasian et al. (Citation2013) found that low income in one’s family of origin was associated with violent offending and substance misuse, especially during adolescence. Meanwhile, the Gini index, a measure of inequality reveals that it has an effect on drug-related crime. Some studies (Brush, Citation2007; Choe, Citation2008) in the US find little evidence of inequality effects on crime. This, therefore, means that evidence of inequality effect on crime may be data-dependent. Baier (Citation2015) points out that when using lagged variables, the Gini coefficient shrinks tremendously and loses significance, suggesting that inequality contributed to the model is very little. Baier (2015) concludes that there is no relationship between crime and inequality—our analysis points otherwise. However, when Baier (2015) adds more control variables to control for a spurious regression, they find no link between crime and inequality. Adopting Neumayer’s (Citation2005) procedure, Baier (2015) tests the model using a fixed effects model and finds no relationship between the two variables. Contrary, Pare and Felson (Citation2014), Fajnzylber (Citation2002) and Neumayer (Citation2005) find a significant relationship between crime and inequality. Our analysis finds a strong negative association between drug-related crimes and inequality.
Baier (2015) then replicates Neumayer’s (Citation2005) estimation using variables at levels; in this case, all the additional variables are significant and signs are consistent with theory. However, the author fails to provide a viable explanation on what is driving the model except for deterministic trends in both the dependent explanatory variables and concludes that all the three models that were estimated found no causality between crime and inequality. The findings of this study establish dissimilar results; however, the focus is on drug-related crime. While Baier (2015), Neumayer (Citation2005) and Fajnzylber (Citation2002) found no relationship between crime and inequality in Latin America, this study finds evidence between these two variables in South Africa.
Aliber (Citation2003) contends that high inequality and poverty is a legacy of South Africa’s apartheid and colonial past. This is supported by the fact that poverty and inequality in South Africa seem to be ethnic-driven. Therefore, it would not be surprising that drug-related crimes are more prominent to non-white races, where poverty is mainly concentrated. Nevertheless, the association between crime and poverty may not be clear-cut as shown by the findings. Neff (Citation2007) finds no association between income or expenditure and South Africa ethnic groups. However, the author noted that the racial classification in South Africa was artificially created to generate racial differences. A similar case is in India, where economic activities and professions are linked to race, ethnic and religious background, popularly known as the caste system. Thorat (Citation2010) noted that poverty is significantly higher in lower castes and selected tribes. Likewise, Cheteni, Khamfula and Mah (Citation2017) found a similar conclusion, whereby black Africans and the-so-called coloureds in South Africa are in the lower caste; implying that these two races have a high likelihood to commit a crime, a view supported by the South Africa Police Service Crime Statistics Report 2014/15.
Statistics South Africa Report (2017) claimed that unemployment levels have risen to over 27%, its highest percentage in the last decade. Unemployment is more prominent among the youth, and, coincidentally, youth are the major offenders in drug-related crimes. Badiora’s (Citation2015) study found that gang activities and participants staying in high-density areas encounter more drug and alcohol abuse. Consequently, they are involved in gang activities and in constant street fights. In the Cape Flats in Cape Town, the BBC Documentary, “Ceasefire for Cape Town Gangs in South African drug war”, documented gang violence linked to drugs. It was found that gang violence was prominent in certain areas where rival gangs fight for the drug market. A similar trend exists in major cities in the whole of South Africa, where there are gang cartels selling drugs. Martinez, Rosenfeld and Mares (2008) found that drug trafficking was more prevalent in disadvantaged neighbours where the risk of engaging in crime was high. On the other hand, Freisthle, Needell and Gruenewald (2005) point out that the likelihood of obtaining drugs and alcohol was linked to adolescent antisocial behaviour.
Based on the view that antisocial behaviour can contribute to crime, we argue that drug-related crimes motivate offenders to commit various crimes. We support this view by using the crime opportunity theory, which states that opportunity determines the outcome of environments prone to crime. In other words, offenders look for an opportunity before committing a crime, and cannot do so unless the opportunity is available to break the law. Therefore, drug-related crimes are committed because of weak law enforcement throughout South Africa. As pointed out by the SAPS (2017), the youth find it easy to acquire drugs. Sindiyandiya, Cheteni and Ncube (2016) study on road accidents in South Africa found that most offending motorists were either intoxicated or under substance abuse.
Conversely, not every opportunity to commit crime translates into crime. According to the rational choice theory, offenders aim to maximise benefits and minimise costs to a decision. Consequently, the offenders look for a crime that offers immediate satisfaction and requires little effort to complete. The fact that drug-related crimes are on the rise highlights a weakened moral fibre in the society. At the beginning of 2017, communities in the Gauteng province staged xenophobic marches, blaming foreign nationals for contributing to drug-related crimes. This was reminiscent of the 2008 xenophobic attacks that leftover 48 people dead. At any rate, the drug marches turned violent. Accordingly, we conclude that drug offenders are rational beings who explore the vulnerability of the criminal justice system to their advantage. Our view is backed by the routine theory, which focuses on societal factors that have an impact on crime opportunism, i.e., where crime is likely to occur at a given point. Zabyelina (2017) stated that crime is relatively not affected by poverty, inequality and unemployment. That is, for crime to occur there needs to be space or time for a motivated offender to find a suitable target. Put differently, offenders tend to follow people’s routines as they go on about their lives.
The other avenue is that drug-related crime in South Africa has a complex network. Usual drug members adapt to investigation methods of law enforcement agencies making it hard for them to deal with organised crimes. Spapens (Citation2011) point out that those apprehended by police and serving jail time replace new criminal groups. However, corrupt contacts among law enforcement agencies have contributed to the creation of organised crime groups.
The contact issues an early warning to drug criminals if the investigative agencies apply new methods or if whether the law enforcement is doing random patrols and search. This leads to a situation whereby the less-protected drug groups disappear, and the protected ones escape arrests. Reuter (Citation1983) once stated that organised crime adopts a market perspective whereby criminal groups run on a principle of survival of the fittest. For instance, Rhino poaching syndicates run an efficient market whereby it is hard to detect the main owners of such a system. The poaching gangs are involved in organised crime as pointed out by Cheteni (Citation2014), where rhino horns are easily smuggled out of Africa through airports. While efforts on anti- rhino poaching efforts have intensified, little has changed. Drug criminals operate a similar network.
9. Conclusions and recommendations
This article furthers the discourse on the link between drug-related crime and poverty. Building on certain theories, this study argued that drug-related crimes are usually linked to poverty and inequality. We find evidence that poverty, inequality and income have an effect on drug-related crimes. Drug-related crimes are committed because offenders find an opportunity to do so. In other words, there are a few means of dealing with drug-related crimes in South Africa.
Our findings, therefore, indicate that preventive efforts should specifically aim to decrease substance abuse and target family-linked factors, rather than income inequality alone. We recommend that law enforcement should monitor and seize drugs. This includes follow-up checks on repeat offenders who are able to resume their involvement in the production or wholesale dealing of drugs. As pointed out by the SAPS (2017), 80% of crimes in South Africa are mainly committed by repeat offenders. The existence of drug gangs highlights a major societal problem and this point to a weak policing system. Therefore, the government should focus on creating special units that deal with drug crime until such a point where drugs are eradicated from the streets. In cases of gang activities, it is known that investigations ending in apprehensions and convictions of both the producers of drugs and the buyers reduce chances of future offending.
Future research can thus focus on identifying how governments can effectively reduce the use of drugs, and how poverty eradication efforts can be used to create formal employment.
Additional information
Funding
Notes on contributors
Priviledge Cheteni
Priviledge Cheteni is a PhD candidate at the North West University in South Africa. His PhD research examines poverty dynamics in South Africa. His research interests include developmental economics, demography, agricultural economics, climate change and environmental economics. He has published widely in various peer-reviewed journals.
Gisele Mah
Professor Gisele Mah is currently a Senior Lecturer in the School Economic and Decision Sciences, at the North West University in South Africa. She holds a PhD in Economics from the North West University in South Africa.
Yohane Khamfula Yohane
Professor Yohane Khamfula holds Master degrees from the University of York and Durham University in the UK, and a PhD in Economics from the Tilburg University in Netherlands.
References
- Aliber, M. (2003). Chronic poverty in South Africa: Incidence, causes and policies. World Development, 31(3), 473–490. doi:10.1016/S0305-750X(02)00219-X
- Badiora, A. I. (2015). Regional differences and developmental implications of security challenges in Nigeria. African Security Review, 24(l), 55–62. doi:10.1080/10246029.2014.990394
- Bailey, W. C. (1984). Poverty, inequality, and city homicide rates. Criminology, 22(4), 531–550. doi:10.1111/j.1745-9125.1984.tb00314.x
- Baier,J. (2015). Education, Corruption and Violent Crime in Mexico. Retrieved from http://www.ehes.org/ehes2015/papers/Baier.pdf
- Becker, G. S. (1968). Crime and punishment: An economic approach. Journal of Political Economy, 76, 169–217. doi:10.1086/259394
- Bjerk, D. (2007). The differing nature of black-white wage inequality across occupational sectors. Journal of Human Resources, XLII(2), 398–434. doi:10.3368/jhr.XLII.2.398
- Bourguignon, F. (1999). Absolute poverty, relative deprivation and social exclusion. Berlin: DSE.
- Bourguignon, F. (2000). The pace of economic growth and poverty alleviation. The World Bank and Delta, Paris. Unpublished
- Bourguignon, F. (2001). The distributional effects of growth: micro vs. macro approaches. Sede de la CEPAL en Santiago (Estudios e Investigaciones) 34859, Naciones Unidas Comisión Económica para América Latina y el Caribe (CEPAL).
- Brush, J. (2007). Does income inequality lead to more crime? A comparison of cross-sectional and time-series analyses of United States counties. Economics Letters, 96, 264-268.
- Chen, C., & Yi, J. (2009). Effect of income inequality on criminal behavior: Evidence from China. The Journal of World Economy (In Chinese), 32, 13–25.
- Cheteni, P. (2014). An analysis of anti-poaching technique in Africa: A case of rhino poaching. Environmental Economics, 5(3), 63–70.
- Cheteni, P., Khamfula, Y., & Mah, G. (2017). Vulnerability to Poverty: An Empirical Analysis using a Community Survey. Unpublished Manuscript, North West University, Mahikeng, South Africa.
- Choe, J. (2008). Income inequality and crime in the U.S. Economic Letters, 31–33. doi:10.1016/j.econlet.2008.03.025
- Demombynes, G., & Özler, B. (2002). Crime and local inequality in South Africa. World Bank Policy Research Working Paper 2925. doi:10.1044/1059-0889(2002/er01).
- Demombynes, G., & Özler, B. (2005). Crime and local inequality in South Africa. Journal of Development Economics, 76(2), 265–292. doi:10.1111/j.1728-4457.2000.00335.x
- Drèze, J., & Reetika, K. (2000). Crime, gender, and society in India: Insights from homicide data. Population and Development Review, 26(2), 335–352.
- Engle, & Granger. (1987). Cointegration and error correction: Representation, estimation and testing. Econometrica, 55, 251–276. doi:10.2307/1913236
- Fafchamps, M. (2006). Development and social capital. Journal of Development Studies, 42(7), 1180–1198. doi:10.1080/00220380600884126
- Fafchamps, M., & Minten, B. (2012). Impact of SMS-based agricultural information on Indian farmers. The World Bank Economic Review, 26(3), 383–414. doi:10.1093/wber/lhr056
- Fafchamps, M., & Moser, C. (2003). Crime, isolation, and law enforcement. Journal of African Economies, 12(4), 571–625. doi:10.1093/jae/12.4.625
- Fajnzylber, P. (2002). Minimum wage effects throughout the wage distribution: Evidence from Brazil’s formal and informal sectors. Unpublished paper, Department of Economics and CEDEPLAR, Universidade Federal do Belo Horizonte, Belo Horizonte, Brazil. doi:10.1044/1059-0889(2002/er01).
- Fajnzylber, P., Lederman, D., & Loayza, N. (2000). Crime and victimization: An economic perspective. Economia, 1, 219–302.
- Farrall, S., & Jennings, W. (2012). Policy feedback and the criminal justice agenda: An analysis of the economy, crime rates, politics and public opinion in Post-War Britain.Contemporary British History, 26, 467–488.
- Freisthler, B., Gruenewald, P. J., Johnson, F. W., Treno, A. J., & LaScala, E. A. (2005). An exploratory study examining the spatial dynamics of illicit drug availability and rates of drug use. Journal of Drug Education, 35(1), 15–27. doi:10.2190/25QY-PBC3-B1EB-JB5Y
- Friedman, M. (1957). A theory of the consumption function. Princeton: Princeton University Press.
- Glaeser, E., Sacerdote, B., & Scheinkman, J. (1996). Crime and social interactions. Quarterly Journal of Economics, 101(2), 507–548. doi:10.2307/2946686
- Hällsten, M., Szulkin, R., & Sarnecki, J. (2013). Crime as a price of inequality? The gap in registered crime between childhood immigrants, children of immigrants and children of Native Swedes. The British Journal of Criminology, 53(3), 456–481. doi:10.1093/bjc/azt005
- Haug, R. (2002). Forced migration, processes of return and livelihood construction among pastoralists in northern Sudan. Disasters, 26(1), 70–84. doi:10.1111/disa.2002.26.issue-1
- Hauner, D., Kutan, A. M., & Spivey, C. (2012). Inequality and crime: Evidence from Russia’s regions. Applied Economics Letters, 19(17), 1667–1671. doi:10.1080/13504851.2011.652773
- Hayden, C. (2007). Children in trouble. The role of families, schools and communities. Basingstoke: Palgrave/Macmillan.
- Hipp, J. R., & Yates, D. K. (2011). Ghettos, thresholds, and crime: Does concentrated poverty really have an accelerating increasing effect on crime? American Society of Criminology, 49, 955–990. doi:10.1111/crim.2011.49.issue-4
- Hirschman, A. O. (1973). The changing tolerance for income inequality in the course of economic development. The Quarterly Journal of Economics, 87, 544–566. doi:10.2307/1882024
- Hu, L., Hu, A., & Xu, S. (2005). A case study of the impact of the disparity between the rich and the poor upon criminal offences. In Management world (in Chinese) (pp. 34–44).
- Jarjoura, G. R., Triplett, R., & Brinker, G. (2002). Growing up poor: Examining the link between persistent childhood poverty and delinquency. Journal of Quantitative Criminology, 18(2), 159–187. doi:10.1023/A:1015206715838
- Johansen, S. (1995). Likelihood-based inference in cointegrated vector autoregressive models. New York, NY: Oxford University Press.
- Kaylen, M. T., & Pridemore, W. A. (2013). Social disorganization and crime in rural communities: The first direct test of the systemic model. British Journal Criminology, 53(5), 905–923. doi:10.1093/bjc/azt031
- Land, K. C., McCall, P. L., & Cohen, L. (1990). Structural covariates of homicide rates: Are there any invariances across time and social space? American Sociological Review, 95, 922–963. doi:10.1086/229381
- Lee, M. R. (2010). Concentrated poverty, race, and homicide. The Sociological Quarterly, 41(2), 189–206. doi:10.1111/j.1533-8525.2000.tb00091.x
- Levitt, S. (1997). Using electoral cycles in police hiring to estimate the effect of police on crime. American Economic Review, 87, 270–590.
- Ludwig, J., Duncan, G., & Hirschfield, P. (2001). 0Urban poverty and Juvenile crime: Evidence from a randomized housing mobility experiment.1. Quarterly Journal of Economics, 116(2), 655–679. doi:10.1162/00335530151144122
- Martínez, R., Rosenfeld, R., & Mares, D. (2008). Social disorganization, drug market activity, and neighborhood violent crime. Urban Affairs Review, 43(6), 846–874. doi:10.1177/1078087408314774
- McMillan, K. A., Enns, M. W., Asmundson, G. J., & Sareen, J. (2010). The association between income and distress, mental disorders, and suicidal ideation and attempts: Findings from the collaborative psychiatric epidemiology surveys. Journal of Clinical Psychiatry, 71(9), 1168–1175. doi:10.4088/JCP.08m04986gry
- Merton, R. (1983). Social structure and anomie. American Sociological Review, 3(5), 672–682.
- Metz, N., & Burdina, M. (2016). How neighbourhood inequality leads to higher crime rates. USApp – American politics and policy blog. Blog Entry.
- Morgan, K. (2000). Inequality and crime. The Review of Economics and Statistics, 82(4), 530–539. doi:10.1162/003465300559028
- Neff, D. (2007). Subjective well-being, poverty and ethnicity in South Africa: Insights from an exploratory analysis. Social Indicators Research: an International and Interdisciplinary Journal for Quality-of-Life Measurement, 80(2), 313–341. doi:10.1007/s11205-005-5920-x
- Neumayer, E. (2005). Does high indebtedness increase natural resource exploitation? Environment and Development Economics, 10(2), 127–142. doi:10.1017/S1355770X04001901
- Noriega, A. E., & Ventosa-Santaulària, D. (2007). Spurious regression and trending variables. Oxford Bulletin of Economics and Statistics, 69, 439–444. doi:10.1111/j.1468-0084.2007.00481.x
- Oreopoulos, P. (2003). The long-run consequences of living in a poor neighbourhood. The Quarterly Journal of Economics, 118(4), 1533–1575. doi:10.1162/003355303322552865
- Pare, P. P., & Felson, R. (2014). Income inequality, poverty and crime across nations. The British Journal of Sociology, 65, 434–458. doi:10.1111/1468-4446.12083
- Pesaran, M. H., Shin, Y., & Smith, R. J. (2001). Bounds testing approaches to the analysis of level relationships. Journal of Applied Econometrics, 16, 289–326. doi:10.1002/(ISSN)1099-1255
- Pridemore, W. A. (2011). Poverty matters: A reassessment of the inequality-homicide relationship in cross-national studies. The British Journal of Criminology, 51(5), 739–772. doi:10.1093/bjc/azr019
- Raphael, S., & Winter‐Ebmer, R. (2001). Identifying the effect of unemployment on crime. The Journal of Law and Economics, 44(1), 259–283. doi:10.1086/320275
- Reuter, P. (1983). Disorganized crime: The economics of the visible hand. Cambridge: The MIT Press.
- Sampson, R. J. (1985). Race and criminal violence: A demographically disaggregated analysis of urban homicide. Crime & Delinquency, 31, 47–82. doi:10.1177/0011128785031001004
- Sampson, R. J. (1987). Urban black violence: The effects of male joblessness and family disruption. American Journal of Sociology, 93, 348–382. doi:10.1086/228748
- Sareen, J., Afifi, T. O., McMillan, K. A., & Asmundson, G. J. (2011). The relationship between household income and mental disorders: Findings from a population-based longitudinal study. Arch Gen Psychiatry, 68(4), 419–427. doi:10.1001/archgenpsychiatry.2011.15
- Sariasian, A., Långström, N., D’Onofrio, B., Hallqvist, J., Franck, J., & Lichtenstein, P. (2013). The impact of neighbourhood deprivation on adolescent violent criminality and substance misuse: A longitudinal, quasi-experimental study of the total Swedish population. International Journal of Epidemiology, 42(4), 1057–1066. doi:10.1093/ije/dyt066
- Shaw, C. R., & McKay, H. D. (1942). Juvenile delinquency and urban areas. Chicago: University of Chicago Press.
- South Africa Police Services. (2016). Crime Statistics. Pretoria: Government Printer.
- Spapens, T. (2011). Interaction between criminal groups and law enforcement: The case of ecstasy in the Netherlands. Global Crime, 12(1), 19–40. doi:10.1080/17440572.2011.548955
- Thorat, A. (2010). Ethnicity, caste and religion: Implications for poverty outcomes. Economic and Political Weekly, 45(51), 47–53.
- The Institute of Economic and Peace (2014). http://economicandpeace.org.
- Valdez. (2007). Aggressive crime, alcohol and drug use, and concentrated poverty in 24 U.S. Urban Areas. American Journal of Drug and Alcohol Abuse, 33(4), 595–603. doi:10.1080/00952990701407637
- Verbeek, M. (2008). A Guide to Modern Econometrics. USA: John Wiley & Sons.
- Webster, C., & Kingston, S. (2014). Poverty and crime review. Available at http://eprints.lancs.ac.uk/71188/1/JRF_Final_Poverty_and_Crime_Review_May_2014.pdf
- Wikstrom, P. H., & Butterworth, D. A. (2013). Adolescent crime (1st ed.). Publisher-Willan.
- Zabyelina, Y. G. (2016). Can criminals create opportunities for crime? Malvertising and illegal online medicine trade. Global Crime. doi:10.1080/17440572.2016.1197124