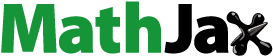
Abstract
The poor use of innovations for financial service delivery among African banks has limited the extent of financial development in the continent. Consequently, financial authorities seeks for a technology-enabled financial solution; an area not well covered in literature. This study therefore, examines the determinants of financial development in a panel of thirty-one heterogeneous African markets for the period 2002–2019 with emphasis on financial technology (Fintech). The liquidity-preference and credit-creation theories were used to model a dummy variable interactive equation to capture possible heterogeneities. The study hypothesized that Fintech transmits directly to financial development among emerging Africa but indirectly through bank-efficiency and financial inclusion among the frontier and fragile groups, respectively. Results from both the dynamic system GMM and the static techniques support this hypothesis with significant heterogeneities in both the intercept and slope coefficients of Fintech among the various groups. The study concludes that on average, emerging African markets report higher financial depth than the frontier and fragile groups; however, with higher slopes, they can converge with emerging markets’ in the long-run through Fintech adoption. The study recommends the collaboration of Fintech with banks, improved bank efficiency and financial inclusion as panaceas to promote financial development in Africa.
PUBLIC INTEREST STATEMENT
The perceived risk in extending credit to the public in Africa is high due to their poor compliance with innovation. This has limited the extent of financial development in the continent. It is on this background that this study examined the various Fintech transmission channels to finance in a panel of five emerging/largest, twenty-one frontier and five fragile African markets for 2002–2019 period. This study argues that African financial development is heterogeneous, hence Fintech transmits directly to finance among emerging markets given the relatively developed state of their financial system; but indirectly through bank efficiency and financial inclusion among frontiers and fragile African markets, respectively. Results from the dynamic and static techniques accentuates to this fact with greater financial development among the emerging Africa compared to frontier and fragile groups. Therefore, there is need to strengthen financial technology transmission mechanism to financial development in African countries through greater financial openness.
1. Introduction
The financial system in Africa has been held back by currency fluctuations, lack of innovation, over-reliance on the conventional banking style, and low supply of products for savings (Beck & Cull, Citation2013; Honohan, Citation1997). These have worsened with the widespread financial exclusion and low coverage of banks and Automated Teller Machines (ATMs) in the continent (Andrianaivo & Kpodar, Citation2012), leading to retarded development and the ineffectiveness of monetary policy in Africa (Allen et al., Citation2014). As a result, many financial authorities have sought for a technology-enabled financial solution to mitigate these problems and promote financial inclusion and overall financial development in the continent. Therefore, with the advent of financial technology, the need to identify its various channels of transmission to financial development among the heterogeneous African markets is the focus of this study.
The financial sectors in Africa are as heterogeneous as the cultural diversities in the continent. As a result, the relevance of financial technology to Africa has been doubtful especially as it comes with both prospects and problems. Therefore, as financial technology (Fintech hereafter) designs and delivers financial services, it becomes imperative to understand the peculiarities of the emerging, frontier and the fragile African markets for a better transmission to financial development. Again, Fintech can close the financial exclusion and digital-divide gaps in Africa, thereby improving the financial depth if channeled through the right source. Moreover, Africa has high propensity to save, but financial expansion and deepening has been constrained by lack of access to financial services and absence of depth of financial instruments (Andrianaivo & Kpodar, Citation2011). This suggests that the reason savings intermediation have not yet transmitted into potential investment in Africa is because of lack of innovation/Fintech, especially as the perceived risk in extending credit to the public in Africa is high (Stone & Grønhaug, Citation1993).
To circumvent these challenges, policies such as the removal of credit ceilings, interest rates liberalization, and privatization of state-owned banks were put in place by many African countries. Despite these, financial markets in Africa are still less developed than markets elsewhere on virtually all indicators of financial development (Green, Citation2013). This, therefore, suggests that financial development in Africa is more of a structural issue such as the use of Fintech in credit extension rather than policy driven. The use of financial innovation/technology in credit extension is the panacea to the widespread financial exclusion and development problems in Africa.
Moreover, the fact that MPESA (mobile money) grew in Kenya and other African markets like Tanzania but failed to launch in South Africa (Alexander et al., Citation2017; Vodafone, Citation2016) further strengthens the concept of heterogeneity among African markets. Its difficulty to replicate its success in South Africa was blamed on excessive financial regulations within South Africa financial system (Alexander et al., Citation2017). Therefore, the role of Fintech could actually be country’s specific. Therefore, this study aims to identify the determinants of financial development among the heterogeneous African markets with emphasize on Fintech.
2. Theoretical models
The demand for money or the liquidity preference theory by Keynes (Citation2016) and the credit creation theory as discussed by Jhingan (Citation2003) were employed to examine Fintech’s heterogeneous transmission channels to financial development. The first theoretical model is used to investigate the real and financial sectors’ variables that determine financial development; the second model is used to capture the various transmission mechanisms through which this impact can occur. Hence, they make up for each other’s weaknesses.
2.1. Liquidity preference (LP) theory
This theory was attributed to Keynes (Citation2016) as postulated in his work on the general theory of employment, interest, and money. Keynes argued that credit requirement is heavily dependent on three unique but interrelated motives. They are the transactions motive, the precautionary motive and the speculative motive. The first two motives are increasing functions of income in the previous period and the frequency of income, while the last motive is a decreasing function of interest rate or the spread of interest rate. Individuals hold their wealth in terms of money or bond. As the level of income increases and the more infrequent income is, the more money households will hold for transaction and precautionary purposes, whereas an increase in interest rate will reduce speculative balance. In other words, households are likely to hold more money for speculative purposes if the lending interest rate exceeds the deposit rate and vice versa.
Keynes (Citation2016) also believes that changes in the institutional and structural conditions of the economy also determine the household’s LP. This means that Keynes acknowledges the roles of institutions as an intermediary in credit extension. Thus, the extent of credit extension by financial institutions depends on their level of efficiency among other things. Therefore, this study argues that financial institutions efficiency could be one of the transmission channels to financial development. Based on the foregoing assertions, the liquidity balance is modelled thus:
Where lp represents the liquidity balance in the current period as a function (f) of income growth rate in the current period (yit), interest rate spread (rit), and financial institutions efficiency (fe) in the current periods. Therefore, based on Keynes theory, we postulate the following functional relationship:
2.2. Credit creation theory
On the other hand, the credit creation theory of banks, according to Jhingan (2003), is a useful theory that explains finance and its determinants. According to this theory, the ability of financial institutions to create credit through loan advancement depends largely on the reserve requirement ratio, total deposits, the number of bank excess reserves as well as the innovations/technologies used in the delivery of financial services. The reserve requirement ratio which is set by the central bank is inversely related to the amount of loan to be advanced, and by implication, the total credit a bank can create at a time. The total credit created equals the product of the initial deposit and the inverse of the required reserve ratio thus:
EquationEquation (1.2)(1.2)
(1.2) is the credit extension formula. It determines to what extent the banking system can create credit, given the initial demand deposit and the deposit expansion multiplier (
). The above equation can also be expressed as follows:
EquationEquations (1.3(1.3)
(1.3) ) and (Equation1.4
(1.4)
(1.4) ) are the extended version of (1.2). Therefore, credit creation through loan advancement depends on the discounted flows of successive required reserves multiplied by the initial deposit (ID). Moreover, since this role of intermediation is done by banks, their level of competence and technology can influence this process. Based on this, this study acknowledges financial institutions’ efficiency and Fintech inherent in a bank as the transmission channels to financial development. Therefore, the total credit created is then a function of the total credit in the previous period, initial deposit (ID), required reserve ratio (Rr), bank efficiency measured in terms of bank profit to assets ratio (Roa) and Fintech (ft) thus:
3. Literature review
Two different streams of empirical view are discussed in this study. First, is the interrelationship between financial development and technology adoption and the second is on whether to follow the supply-leading hypothesis or the demand-leading view to assess finance and real sector performance/technology adoption.
The channels through which technological advancement can spur finance are through an efficient banking system and a well-developed capital markets (Tadesse, Citation2005). This is because technology adoption is capital intensive and this could be mobilized through an efficient financial system. Financial markets, therefore, provide investors with the required capital to make this investment depending on the extent of financial openness in the country. Tadesse (Citation2005) proposed that the level of countries’ technical progress and the development of their financial systems in mobilizing funds are directly proportional. Moreover, the perceived liquidity risks of potential investors on technology adoption can be reduced when the state of the country’s capital markets and institutions are well development (Bencivenga et al., Citation1995). Bencivenga and Smith (Citation1991) added that financial intermediation promotes real growth when the process is designed in such a way that it reduces liquidity risk. Therefore, technology adoption and interest rate are inversely related. This suggests that a well-functioning banking/financial system makes fund available for technology adoption at a reduced risk or interest rate thereby serving as a precondition for technology to adoption to drive finance.
On the other hand, there has been an on-going debate in economic literature on whether to follow the supply-leading hypothesis or the demand-leading view to model the finance-growth nexus. Empirical pieces of evidence on this issue have being inconsistent. While some (H. C. Huang & Lin, Citation2009; Leitao, Citation2010;Tadesse, Citation2005; Van Nieuwerburgh et al., Citation2006) support the supply-leading view that finance has a positive impact on growth; others (Benyah, Citation2010; Chinn & Ito, Citation2006; Falahaty & Law, Citation2012; W. Huang & Temple, Citation2005) follow the demand-leading hypothesis and conclude that growth variables actually respond to changes in financial development.
Financial development promotes productivity by interacting with technological innovation and vice versa (Tadesse, Citation2005). This interrelationship is more of a heterogeneous process across the different African countries or industries that differ in their needs for financing innovation due to the multiplicity of diversities within the African financial system. Therefore, with greater financial openness and external financing, there can be a rapid growth rate of technological change in countries with more developed banking sector (Tadesse, Citation2005). This suggests that whereas financial openness could accelerate technology adoption and financial development, especially in financially repressed economies, a developed or efficient banking system is a precondition for these technologies to transmit to greater financial development and vice versa. These findings were consistent with those of Falahaty and Law (Citation2012), Hassan et al. (Citation2011), Benyah (Citation2010), and Chinn and Ito (Citation2006) who followed the demand leading hypothesis to investigate the determinants of financial development. According to Chinn and Ito (Citation2006), higher levels of financial openness, trade openness and banking system development can spur financial development when it interacts/transmits with a threshold level of legal development.
Irrespective of the direction of causality, finance-real sector performance nexus will not occur directly without a transmission channel (De-Gregorio & Guidotti, Citation1995). It is on this background that Mundial (Citation2014) pointed out that, there have been works of literature that linked finance and growth without a clear transmission mechanism. The literature rarely attempts to identify the particular mechanism through which finance-growth nexus can emerge (Arestis et al., Citation2006). Based on this, Sassi and Goaied (Citation2013) asserts that ICT adoption is a transmission channel through which finance can spur growth; otherwise, finance-growth nexus can be ambiguous. Benyah (Citation2010) confirmed this by reporting a negative impact of economic growth and financial openness on financial development in Africa. Therefore, it follows that finance and real sector performance can spur each other when transmitted through the right channel (Manizheh & Law, Citation2010). Later studies that addressed the determinants of financial development found legal, political and cultural factors, bank ownership, bank concentration and geographical differences across countries as important factors (Manizheh & Law, Citation2010). Invariably, it follows that for real sector performance to drive financial development, there has to be an efficient structure that will diversify excess savings into more potential real investments “at the highest available rates of return, and with minimum transactions costs” (Killick & Martin, Citation1990).
Despite the number of possible variables that have been outlined, there has not been a consensus (Benyah, Citation2010) either on the determinants of financial development or on its transmission channels. This is continuously updated in the literature. The major argument put forward in this study is that the extent of development in the financial system of an economy determines whether Fintech follows any transmission channel to financial development and what should constitute the transmission channel. Therefore, based on this argument, this study hypothesized that Fintech transmits to financial development directly among the emerging markets given their relatively developed financial system/structure but indirectly through bank efficiency (ROA) and financial inclusion (DBB)Footnote1 among the frontier and fragile categories, respectively.
4. Methodology
This study employs the dynamic system generalised method of moments (GMM) to estimate the model. This was implied by the theoretical framework under two instances. First, the theoretical model proposes that current period’s credit creation depends on its pervious period’s, and second, most of the variables in the model are endogenously determined. Therefore, a dynamic system GMM estimation technique will best capture the variability in the model. A dynamic GMM technique is efficient when the individual observation of the panel is more than or equal to its time observation. The individual units in this study is thirty-one countries (N = 31) and the number of years is eighteen (T = 18) years. It is capable of correcting for unobserved panel heterogeneity, omitted variable bias, measurement error and endogeneity problems of the lagged dependent variable (Bond et al., Citation2001). Moreover, in addition to the dynamic model, the static fixed and random effects techniques were also employed as a robustness check. The Hausman test was used to select between the fixed and the random effects techniques. The null hypothesis that the random effect model is a better estimate will not be rejected if probability value for the chi2 Hausman test is greater than 5 per cent otherwise, the fixed effect is preferable.
4.1. Model specification
The econometric equation is based on the two theoretical models reviewed above. The two models suggest the roles of both real sector and financial variables as the determinants of credit extension. It comprises the properties of Equationequations (1.1(1.1)
(1.1) ) and (Equation1.5
(1.5)
(1.5) ) in its general form thus:
Where: FD = vector of the different measures of financial development (the ratio of Credit to
private sector to GDP and the ratio of liquid liability to GDP);
Y = GDP growth rate;
IRS = Interest rate Spread;
ROA = Bank returns to assets ratio (a measure of financial efficiency);
DBB = Sum of deposits and borrowers from banks/Initial deposit (a measure of
financial inclusion);
ft = Vector of the measures of Fintech, Mobile Phone Banking (MPB), Internet
Banking (INTB), and Automated Teller Machines (ATM); while
FO = the economies financial openness (control variable),
The level form of Equationequation (1.6)(1.6)
(1.6) is specified thus:
Where: FDit, and αi0 are N X 2 vectors of the two measures of financial development and the average financial development for country i in period t, respectively. vi and μit are also N X 2 vectors of the country’s specific fixed effect and the unexplained portion of the dependent variable, respectively; hence μit ~ IID (0, σɛ2). δ0i and δji are K Χ 2 vectors of unknown parameters to be estimated on the explanatory variables of Fintech and three control variables, respectively. FDit-1, ftit and Xit are N X K matrixes of lagged dependent variable, explanatory variables of Fintech, and three control variables (Xit) respectively. The GMM estimation technique assumes another matrix Z that is N x M because of the presence of endogeniety; where, M > K, so that matrix Z are strictly exogenous {i.e. E(Z` μit) = 0}. Therefore, the Z matrix is a set of valid instrumental variables assumed to be highly correlated with the explanatory variables but orthogonal to the error term. Orthogonality in this sense means that the Z matrix consists of variables that are not correlated with the error term. Therefore, for the estimated parameters to be unbiased, the instrumental variables Z must be less than or equal to the number of groups (N).
By taking the first difference of Equationequation (1.7)(1.7)
(1.7) and transforming it to incorporate heterogeneity in both the average (intercept) and the slope Fintech determinants of financial development among the emerging, frontier and fragile economic groups, a dummy variable interactive System GMM equation is therefore modelled thus:
The variables remain as defined above. However, for clarity of purpose, the following explanations were necessary. δji’s are K Χ 2 vectors of unknown parameters, whereas FDit-1, ftit, ftit(ROAit),
ftit(DBB) and Xit are N X K matrixes explanatory variables and dt is the year dummies The dummy variables were used to ascertain which economic group is being modelled. Therefore,
is a dummy variable taking 1 if the economy group belongs to i group and 0 otherwise; i0 is a reference category and i1 and i2 represents frontier and fragile markets, respectively. EquationEquation (1.8)
(1.8)
(1.8) nests the equation by equation estimation. Take for instance, for a given economic group i, the equation is reduced to a regression of agent i’s intercept, the slope coefficients and the control variables. Since three groups of economies are considered (the emerging, frontier and fragile economies), two dummies were used for frontier and fragile groups to avoid the problem of dummy variable trap. This study used emerging markets as the reference category because they have a well-developed financial sector relative to the other markets in the model. Therefore, if the reference category is i0, then αi0 and δ0i are its corresponding intercept and slope coefficients, respectively, with respect to the impact of Fintech on financial development. The intercept and the slope coefficients of group i1 economy (frontier group) are given by (αi0+ α1) and (
+
), respectively, whereas that of the fragile group (i2) are (αi0+ α2) and (
+
), respectively. Note that the country’s specific-fixed effect disappears after the first differencing because it does not vary with time thus:
EquationEquation (1.8)(1.8)
(1.8) is a six-model equation for the three economic groups for two dependent variables. Its uniqueness is because it allows heterogeneity in the intercept and the slope coefficients among the three different economic groups thereby enabling us to treat each economic group as unique from the others. This makes it easy to ascertain the actual level of financial development and its determinants among each economic group for comparative analyses.
4.2. Data
The analysis is based on twenty four African economies comprising of five/largest emerging,Footnote2 twenty-one frontiers and five fragile marketsFootnote3 for the period 2002–2019. The different countriesin each group are presented under the Appendix Table . The data is sourced from the World Bank database (WBD) and International Financial Statistics (IFS). Two measures of financial development were used. They are bank credit-to-private sector as a ratio of gross domestic product (CPS) and liquid liability as a ratio of gross domestic product (LLGDP). Most studies among African economies used credit to private sectors and liquid liability as measures of financial development (Beck et al., Citation2003). Measures of Fintech include automated teller machine (ATM) per 100,000 people, individuals using internet to total population ratio, a proxy for internet banking and mobile phone subscription per 100,000 people, a proxy for mobile banking. The variables are briefly summarized in Table .
Table 1. Description of variable measurement and expected signs based on EquationEquation (1.8(1.8)
(1.8) )
Table 2. System GMM results of EquationEquation (1.8(1.8)
(1.8) )
Table 3. Static fixed and random effects models of EquationEquation (1.8)(1.8)
(1.8) for robustness check
5. Results and discussions
This section began with the presentation and discussion of the system GMM one-step results as modelled in EquationEquation (1.8)(1.8)
(1.8) . Financial development was measured with two indicators of domestic credit to private sector as a ratio of gross domestic product (CPS) and liquid liability to GDP (LLGDP). Six unique equations were estimated for the intercept coefficients only, the intercepts and slope coefficients and the intercept, slope and control variable coefficients each for CPS and LLGDP. This was necessary to see how the intercepts and slope determinants of Fintech impacts on financial development among the various economic groups.
In models 1 and 2, the results reveal that there is significant heterogeneity on the average financial development among the three economic groups. Particularly, in model 1, the average financial development among the emerging group α0 = 18.85% whereas those for the frontiers and fragile groups were (α0 + α1) = 7.82 and (α0 + α2) = 2.73 per cents, respectively. This shows that emerging markets report higher levels of domestic credit to private sector than the frontier African markets and the latter reports higher average than the fragile group. The same is true between emerging and frontier markets when financial development is measured in terms of LLGDP in model 2 and under models 4, 5 and 6. Therefore, the null hypothesis that α1 = α2 = 0 is rejected for models 1, 2, 4, 5 and 6 but accepted for model 3Footnote4; hence the average financial development for all the economic groups is 21.76% under model 3. This supports our assumption that emerging African markets’ financial state is relatively more developed than those of the frontier and fragile groups. These models (1 and 2) accounted only for the intercept variations for the three economic groups (αi0, α1 and α2) and the lag of financial development indicator. It did not account for the slope effect of Fintech. As a result, their explanatory power is too small to generalise for the entire groups. Given this weakness, models 3 and 4 were estimated to accommodate this weakness by accounting for both the intercepts and slope effects of Fintech transmission channels among the three economic groups.
The next two models (3 and 4) therefore assessed whether there is heterogeneity in the slope coefficients of Fintech impact on financial development among the three economic groups. The results reveal a consistent conclusion with the restricted version of 1 and 2. In addition to finding heterogeneity in the intercepts among the different groups, model 4 also revealed that there is significant heterogeneity in the slope coefficients of Fintech at 1% significant level. To be more specific, model 4 reveals that the slope impact of internet banking on financial development is on average and all things being equal δ0i = 0.21% at 1-% significance level while those of frontier and fragile groups are (δ0i + δ01) = 0.133 and (δ0i + δ02) = 0.209 per cents, respectively. Moreover, mobile banking raises financial development among the three economic groups at the rate of 0.045 per cents all things being equal. This is because (δ01) = (δ02) = 0 when Fintech is measured in terms of mobile banking under model 4. Again, the average slope coefficients for the impact of ATM on financial development stood at 0.645 and 0.228 per cents for emerging and frontier markets, respectively, while that of mobile banking among the fragile groups is −0.011% under model 3. These results suggest that an efficient banking system (ROA) and improved financial inclusion are the transmission channels through which Fintech can promote financial development among the frontier and fragile African markets, respectively.
Furthermore, apart from the impact of Fintech on financial development as were assessed in models 3 and 4; models 5 and 6 incorporated the role some control financial and macroeconomic variables could play on financial development. The result reveals some ambiguous conclusions. First, the inclusion of the control variables led to the violation of the heterogeneous slope assumption among the different economic groups. Second, the growth rate of output (GDP) significantly dampens the level of financial development. In model 6 for instance, the violation of the heterogeneous slope coefficients of Fintech is evident from the fact that δ01 = δ02 = 0, therefore virtually all the economic groups had similar slope of δ0i for the three measures of Fintech. This means that (δ0i) = (δ0i + δ01) = (δ0i + δ02). This could be attributed to the high significant and positive impact of financial openness at 1% significance level, thereby indicating that the more financially opened the financial market becomes, the more likely fragile and frontier markets will attract foreign direct investment and converge with their emerging counterpart in financial development. The fact that the reverse was the case with financial development measured in terms of CPS in model 5 is an indication that there were significant differences between the two measures of financial development in Africa.
Again, apart from internet banking that significantly dampens liquid liability/GDP at average rates of 0.384 and 0.389 per cents among the emerging and fragile groups, respectively, all economic groups had same slope for ATM and mobile banking at −0.394 and 0.264, respectively,Footnote5 under model 6. Likewise, the assumption of heterogeneous slope coefficients under model 5 was also violated as the coefficients of Fintech for emerging (δ0i) and frontier markets (δ0i + δ01) were statistically zero. In other words, whereas mobile banking and ATM significantly raises credit to private sector to the tune of 0.341 and 0.473 at 10% significance level among the frontier and fragile markets, respectively, internet banking significantly dampens it at 1% significance among the fragile groups. Therefore, Fintech could not impact on credit to private sector among the emerging group under model 5.
In addition, results from models 5 and 6 further reveal that the real sector variable of gross domestic product growth rate (GDPR), interest rate spread, and financial openness significantly impacts on financial development in Africa at 1-% significance level. The ambiguous result of a negative impact of GDP growth rate on financial development was consistent with that of Benyah (Citation2010) who also found that GDP growth rate negatively impacts financial development in Africa. This suggests the need for real sector variables to interact with institutional variables to drive financial development in Africa as were proposed by some authors (Chinn & Ito, Citation2006; Manizheh & Law, Citation2010; Sassi & Goaied, Citation2013).
Findings from models 1 to 6 reveal that the nexus between financial development and financial technology is a dynamic heterogeneous process. This was inferred from the strong significance of the first lag of the dependent variable at 1-% significance level for all the models. This suggests that various factors other than financial and economic can drive the level of an economy’s financial development through different channels. Therefore, as a dynamic GMM model, it is susceptible to two unique drawbacks. They are the problems of endogeneity or serial correlation and instrument validity. This study employs two specification diagnostic tests of the Sargan test of the overall validity of the instruments and the absence of serial correlation as were proposed by Blundell and Bond (Citation1998) and Arellano and Bond (Citation1991) respectively. Blundell and Bond (1998) believe that when the independent variables are not strictly exogenous, that means they are correlated with past and possibly current realisations of error with fixed individual effects and as such could produce biased parameter estimates. Therefore, the null hypothesis is that all instruments as a group are strictly exogenous; hence a higher p-value is desirable. On the other hand, the serial correlation test examines the null hypothesis that the error term (Ɛit) of the differenced Equationequation (1.8)(1.8)
(1.8) is not serially correlated at least at the second-order; hence a higher p-value is also desirable. While we cannot reject the null hypotheses of absence of serial correction for models 1 to 6 because their p-values are all more than 5%, only models 5 and 6 suggest that the instruments are indeed strictly exogenous because their p-value is more than 5%. Since the inclusion of the control variables validates the use of instruments, it suggests that real sector performance, interest rates, and the extent of an economies financial openness are necessary policy indicators to promote financial development among African markets. However, models 1 to 4 cannot be used for policy measures because their parameter estimates are biased.
On the other hand, the static fixed and random effects models were also used to investigate this nexus between financial development and financial technology among the heterogeneous African markets as a robustness check. The use of the static model was informed by the non-validity of instrumental variables particularly in models 1 to 4 and the negative non-significant-lagged dependent variable of domestic credit to private sectors in model 5. These suggest that the assumptions of endogeneity and instrument validity could be actually violated for these models and as such, static technique could useful too.
The findings from the static output results in Table were consistent with those of the system GMM estimator. Like the dynamic system GMM, it found heterogeneity in both the intercept and slope coefficients especially for models of 7, 8 and 12 below.Footnote6 Under model 7, the average financial development among the emerging, frontier and the fragile markets are 51.61, 24.62, and 9.99 per cents, respectively. Emerging markets report higher average of financial development than the frontier group which also reports higher average than the fragile group. This suggests that the more developed an economy becomes, the more likely they will diversify excess funds optimally from their surplus to deficit points. This consistent conclusion holds for models 8 and 12 as well.
Although, models 9 to 11 show that the assumption of heterogeneous average financial development is violated, thereby suggesting common intercept for the three economic groups; however, there is heterogeneity in its slope coefficients for internet banking, particularly for model 10. In other words, emerging African markets report higher level of the impact of internet banking on financial development more than the frontier (0.059) and fragile (0.167) groups. However, the explanatory power of this model is weak as the variability in liquid liability/GDP that was attributed to the independent variables was only 9.6%. Model 12 augmented this weakness by introducing some control variables. The inclusion of control variables raised the explanatory power of the independent variables to 57.85% with strong heterogeneity in both the intercept and slope coefficients of the impact of Fintech were found. It reveals that emerging markets reports higher impact of Fintech in driving financial development than the frontier markets which in turn reports higher intercepts and slopes than the fragile group. This result is consistent with those under the dynamic models of 1 to 6 and it also follows prior expectation that emerging African markets on average has higher financial development compared to their frontier and fragile groups. This could be used for policy implication especially as the explanatory power of the independent variable is high at 57.85%.
Finally, the coefficients of the year dummies was used to examine the growth process of financial development among African markets over the years, with emphasis from 2008 (year seven) being the first time the word “Fintech” was used in financial literature (Gimpel et al., Citation2018). This marked the year when financial authorities began to seek and implement a technology-enabled financial solution in financial services delivery, following the global financial crisis of 2008. The result as presented under the appendix Table B1 reveals that overall; there is a steady decline in the average level of financial development in Africa since the adoption of Fintech in 2008 for financial service delivery.
The estimates were based on models 1 – 6 being the main models of this study. With emphasis on only the significant coefficients, the result shows that in 2012, financial development measured in terms of credit to private sector to GDP ratio were on average and ceteris paribus -22.9 per cent (-21.9 – 1)% lower than the base year (2002) average rate. However, financial development measured in terms of liquid liability to GDP ratio in 2015, is about 20 per cent (1.20 - 1)% on average and ceteris paribus higher than its average rate in 2002. Furthermore, the results suggest that there is a continuous decline in financial development since the advent of Fintech in 2008 till the recent time in 2019. In model 4 for instance, the level of financial development in 2010 was about 3.7% lower than its average in 2002 but 4.7 and 9.4 per cents lower in 2011 and 2019 respectively in comparison to its base year average. This implies that between 2010 and 2019, financial development in Africa further declined by about 5.7%. This explains why Fintech has not being able to translate into improved financial system development in Africa. Therefore, the financial authorities in the continent should seek to implement a macro-prudential policy to forestall possible financial crisis that might arise due to Fintech adoption.
6. Conclusion and policy implications
This study follows the demand leading hypothesis of finance-growth nexus to empirically examine the various channels through which Fintech transmits to financial development among the various economic groups in Africa for the period 2002–2019. This study was motivated by the role Fintech plays in creating and extending credit and other financial services better than the conventional financial intermediation process by banks. Three economic groups were identified such as emerging, frontier, and fragile markets among a sample of thirty-one African economies based on FTCH (2019) countries’ classifications. Therefore, this study hypothesised that Fintech transmits to financial development directly among emerging markets, but indirectly through increased bank efficiency and financial inclusion among frontier and fragile markets, respectively. The rationale behind this is that emerging markets has an established financial structure with greater financial inclusion and bank efficiency compared to the other groups. Following the liquidity preference and the credit creation theories, a demand leading finance-growth dummy variable interactive model was specified and estimated with the dynamic system GMM and the static fixed and random effects techniques.
Major findings from the dynamic system GMM technique reveal that the impact of Fintech on financial development in Africa is a dynamic heterogeneous process. This was inferred by the strong heterogeneity in the intercepts and slope of Fintech among the three economic groups. The emerging group reports higher average and slope impact of Fintech than the frontier and fragile groups in almost all the models. This implies that emerging African markets have an established financial structure that promotes financial depth; whereas for the frontier group, a significant interaction between Fintech and bank is the panacea to raise financial development among them. On the other hand, greater financial inclusion among the fragile group through Fintech adoption will improve their financial depth.
The results further reveal under the static random effect model 12 that frontier and fragile markets have higher slope in terms of mobile banking at [(δi0 + δi1) = 0.032] and [(δi0 + δi2) = 0.002] than the emerging markets’ at [(δi0) = 0].Footnote7 This implies that, all things being equal, frontier, and fragile African markets can still converge with the emerging group financial development by using mobile banking to advance financial depth in the long run. The same is true between the emerging and fragile groups in terms of internet banking under the dynamic system technique of model 6. Therefore, the implication of this is that while policies tailored towards greater Fintech adoption and bank efficiency can promote finance among the frontier group, financial inclusion strategy, and structural transformation is the panacea to advance it among the fragile group, whereas economic diversification to new innovations in finance is the solution for emerging markets to stay afloat. In other words, whereas bank financial institutions’ collaboration with Fintech companies is a precondition for frontier markets to develop finance and catch-up with emerging markets, the ability of African fragile markets to develop finance is basically moored to financial inclusion, structural transformation, political stability, and the development of legal and education systems.
Finally, the fact that real sector variable of GDP growth rate and interest rate spread significantly dampen finance in Africa further suggests the need for a transmission channel between finance and real sector performance. This result is consistent with that of Sassi and Goaied (Citation2013) who found that the nexus between finance and growth is strengthened through ICT diffusion as a transmission channel. On the other hand, the positive significant impact of financial openness is an indication that financial regulations and reforms in Africa can negate the smooth running of the financial system in Africa and the potential benefits that comes with financial-technology adoption.
Additional information
Funding
Notes on contributors
Tochukwu Timothy Okoli
Tochukwu Timothy Okoli is a postgraduate student at the University of Zululand, South Africa and the principal researcher of this paper. This paper which aimed at finding what constitutes Fintechs’ transmission channels to financial development among the heterogeneous Africa was written in collaboration with my Supervisor. My research interest includes macroeconomic modeling, financial-technology, financial risk, financial stability, and development. I also excel in quantitative economics, systemic risk analysis, and econometric modeling and I have cognitive experience in teaching and research both locally and internationally.
Devi Datt. Tewari
On the other hand, Professor Devi Datt Tewari is a Professor of financial economics and the principal PhD Supervisor of Tochukwu Timothy Okoli. He has cognitive experience of more than thirty years in teaching and research. He served as the Dean of the faculty for a period of five years (2014-2018) and he has produced over twenty PhD students and has lots of publications. He is a researcher, a reviewer, a mentor, and a supervisor.
Notes
1. See variable description under Table 1
2. The emerging African markets are basically Egypt, Morocco and South Africa; however, the study also included Algeria and Nigeria in this category because of their well-developed financial and stock markets (Nili and Rastad, Citation2007). This made it a total of five countries within this category.
3. Moreover, due to the problem of non-availability of data, only twenty-one frontiers and five fragile African markets made the list under this study.
4. Note that Model 1 and Model 2 as presented in Table represents the same equation (1.8), both under credit to private sector and Liquid liability to GDP ratios as the dependent variables respectively.
5. Note that the slope coefficients of ATM and mobile banking among the frontier and fragile groups under model 6 were not significant even at 10% level of significance. Hence, their impact is statistically zero. This means that their impact is the same as those of the emerging group.
6. Note that the Hausman test was used to select between the fixed and the random effect models. The null hypothesis of the Hausman test is that the random effect model is better therefore a higher p-value suggests that we cannot reject the null hypothesis, otherwise we accept the alternative hypothesis that fixed effect model is better.
7. Note that the slope of mobile banking (δi0 = 0.008) under model 12 is statistically insignificant therefore, its true value is zero.
References
- Alexander, A. J., Shi, L., & Solomon, B. (2017). How Fintech is reaching the poor in Africa and Asia: A Start-Up Perspective. EMCompass, 34. International Finance Corporation, World Bank Group. http://hdl.handle.net/10986/30360
- Allen, F., Carletti, E., Cull, R., Qian, J. Q., Senbet, L., & Valenzuela, P. (2014). The African financial development and financial inclusion gaps. Journal of African Economies, 23(5), 614–18. https://doi.org/10.1093/jae/eju015
- Andrianaivo, M., & Kpodar, K. (2011). ICT, financial inclusion, and growth evidence from African countries (No. 11–73). International Monetary Fund.
- Andrianaivo, M., & Kpodar, K. (2012). Mobile phones, financial inclusion, and growth. Review of Economics and Institutions, 3(2), 30. https://doi.org/10.5202/rei.v3i2.75
- Arellano, M., & Bond, S. (1991). Some Tests of Specification for Panel Data: Monte Carlo Evidence and an Application to Employment Equations. The review of economic studies, 58(2), 277–297. doi:10.2307/2297968
- Arestis, P., Chortareas, G., & Desli, E. (2006). Financial development and productive efficiency in OECD countries: An exploratory analysis. The Manchester School, 74(4), 417–440. https://doi.org/10.1111/j.1467-9957.2006.00502.x
- Beck, T., & Cull, R. (2013). Banking in Africa. The World Bank. https://doi.org/10.1596/1813-9450-6684
- Beck, T., Demirgüç-Kunt, A., & Levine, R. (2003). Law and finance: Why does legal origin matter? Journal of Comparative Economics, 31(4), 653–675. https://doi.org/10.1016/j.jce.2003.08.001
- Bencivenga, V. R., & Smith, B. D. (1991). Financial intermediation and endogenous growth. The Review of Economic Studies, 58(2), 195–209. https://doi.org/10.2307/2297964
- Bencivenga, V. R., Smith, B. D., & Starr, R. M. (1995). Transactions costs, technological choice, and endogenous growth. Journal of Economic Theory, 67(1), 153–177. https://doi.org/10.1006/jeth.1995.1069
- Benyah, F. E. K. (2010). The determinants of financial development: A focus on African countries.
- Blundell, R., & Bond, S. (1998). Initial conditions and moment restrictions in dynamic panel data models. Journal of econometrics, 87(1), 115–143. doi:10.1016/S0304-4076(98)00009-8
- Bond, S. R., Hoeffler, A., & Temple, J. R. (2001). GMM estimation of empirical growth models. https://ssrn.com/abstract=290522
- Bzhalava, E. (2014). Determinants of financial development.
- Chinn, M. D., & Ito, H. (2006). What matters for financial development? Capital controls, institutions and interactions. Journal of Development Economics, 18(1), pp. 163–192. https://doi.org/10.1016/j.jdeveco.2005.05.010
- De Gregorio, J., & Guidotti, P. E. (1995). Financial development and economic growth. World development, 23(3), 433–448. doi:10.1016/0305-750X(94)00132-I
- Falahaty, M., & Law, S. H. (2012). The effect of globalization on financial development in the MENA region. Transition Studies Review, 19(2), 205–223. https://doi.org/10.1007/s11300-012-0242-6
- Gimpel, H., Rau, D., & Röglinger, M. (2018). Understanding FinTech start-ups–a taxonomy of consumer-oriented service offerings. Electronic Markets, 28(3), 245–264. doi:10.1007/s12525-017-0275-0
- Green, C. J., (2013). Financial reform and financial development in Africa. Commissioned proposal prepared for AERC. University of Georgia: Africa Growth Institute.
- Hassan, M. K., Sanchez, B., & Yu, J. S. (2011). Financial development and economic growth: New evidence from panel data. The Quarterly Review of Economics and Finance, 51(1), 88–104. https://doi.org/10.1016/j.qref.2010.09.001
- Honohan, P. (1997). Banking system failures in developing and transition countries: Diagnosis and predictions (January 1997). BIS Working Paper No. 39. https://ssrn.com/abstract=860624
- Huang, H. C., & Lin, S. C. (2009). Non‐linear finance–growth nexus. Economics of Transition, 17(3), 439–466. https://doi.org/10.1111/j.1468-0351.2009.00360.x
- Huang, W., & Temple, J. (2005) Does external trade promote financial development? Bristol Economics Discussion Papers 05/575.
- Ito, H. (2005). Notes on the calculation of the Chinn-Ito financial openness variable. www. ssc. wisc. edu/~ mchinn/Readme_kaopen163. pdf.
- Jhingan, M. L. (2003). Macroeconomic Theory, © ML Jhingan. Vrinda Publications, New Delhi.
- Keynes, J. M. (2016). General theory of employment, interest and money. Atlantic Publishers & Dist.
- Killick, T., & Martin, M. (1990). Financial policies in the adaptive economy. British Library, United Kingdom. http://hdl.handle.net/10068/517924
- Leitao, N. C. (2010). Financial development and economic growth: a panel data approach. Theoretical and Applied Economics, 15–24.
- Luintel, K. B., & Khan, M. (1999). A quantitative reassessment of the finance–growth nexus: Evidence from a multivariate VAR. Journal of Development Economics, 60(2), 381–405. https://doi.org/10.1016/S0304-3878(99)00045-0
- Manizheh, F., & Law, S. H. (2010). The determinants of financial development: New evidence from the Middle East and North Africa region.
- Mundial, B. (2014). Global Financial Development Report 2014: Financial Inclusion. World Bank.
- Nili, M., & Rastad, M. (2007). Addressing the growth failure of the oil economies: The role of financial development. The Quarterly Review of Economics and Finance, 46(5), 726–740. doi:10.1016/j.qref.2006.08.007
- Otto, J. A. (2003). U.S. Patent No. 6,578,760. Washington, DC: U.S. Patent and Trademark Office.
- Sassi, S., & Goaied, M. (2013). Financial development, ICT diffusion and economic growth: Lessons from MENA region. Telecommunications Policy, 37(4–5), 252–261. https://doi.org/10.1016/j.telpol.2012.12.004
- Stone, R. N., & Grønhaug, K. (1993). Perceived risk: Further considerations for the marketing discipline. European Journal of marketing, 27(3), 39–50
- Tadesse, S. A. (2005). Financial development and technology. University of Chicago - Booth School of Business; University of Michigan at Ann Arbor; University of Pennsylvania - Wharton Financial Institutions Center, https://papers.ssrn.com/sol3/papers.cfm?abstract_id=681562
- Van Nieuwerburgh, S., Buelens, F., & Cuyvers, L. (2006). Stock market development and economic growth in Belgium. Explorations in Economic History, 43(1), 13–38. https://doi.org/10.1016/j.eeh.2005.06.002
- Vodafone, N. N. (2016). M-Pesa Reaches 25 million Customers Milestone, vodafone.com, April 25, 2016; Mbele, Lerato, Why M-Pesa Failed in South Africa, bbc.com. Game publisher Game Mine.
- Yartey, C. A. (2008, May 11). The determinants of stock market development in emerging economies: Is South Africa different? (No. 8–32). International Monetary Fund.