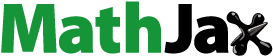
Abstract
This study is the first to analyse the relative importance of a number of the most cited determinants of bank risk and profitability using random forest’s relative value importance measure. The results show that a bank’s profitability is largely determined by bank-specific factors, while a bank’s risk is predominantly impacted by country-level factors. The results also suggest that proxies for market power and size play significant roles in impacting both the bank’s profitability and its risk profile. The analysis also confirms the presence of a major role for a country’s financial development status and regulatory quality in impacting the bank’s riskiness. Lastly, the analysis confirms the presence of a small number of dominant determinants of a bank’s profitability in contrast to the absence of clear dominant determinants of a bank’s riskiness.
PUBLIC INTEREST STATEMENT
We find that a bank’s profitability is largely determined by bank-specific factors, while a bank’s risk is predominantly impacted by country-level factors. We also find that proxies for market power and size play significant roles in impacting both the bank’s profitability and its risk profile. Furthermore, our analysis confirms the presence of a major role for a country’s financial development status and regulatory quality in impacting the bank’s riskiness. Lastly, our results suggest the presence of a small number of dominant determinants of a bank’s profitability in contrast to the absence of clear dominant determinants of a bank’s riskiness.
1. Introduction
The purpose of this study is to provide a comprehensive evaluation of the relative importance of the various determinants of a bank’s profitability and risk. While a number of previous studies have attempted to study the various factors contributing to a bank’s performance and/or risk (e.g., Bourke, Citation1989; Mirzaei et al., Citation2013; Molyneux & Thornton, Citation1992; Pasiouras & Kosmidou, Citation2007), we are aware of no study that attempts to study the relative importance of each factor. We use a well-known machine learning technique from other fields of research, namely random forest’s (RF) relative variable importance (RVI), to measure the comparative significance of each factor in determining a bank’s risk profile or profitability.
Our research adds to the growing stream of research focusing on understanding banks’ performance and risk profiles through measuring and comparing the relative contribution of each individual variable to the process. It also provides equity and credit analysts with better insights on the importance of the role of each studied determinant of a bank’s performance and riskiness. This will allow both groups of analysts to improve their analyses and forecasts of future performance through using findings on relative importance to assign unequal weights to the various factors used in their models based on importance.
The findings in this study also have some important policy implications. The insights from the new empirical evidence provided in this study provide regulators with a better understanding of the importance of each factor and help guide future regulatory decisions through focusing on those factors with the biggest impact. Similarly, the results from this study can help regulators monitor banks’ performance more efficiently through directing their attention towards factors with higher contribution.
Using the random forest’s relative value importance measure we find that the bank’s profitability and risk are largely determined by bank-specific factors and country-level factors, respectively. We also find that market power and size proxies play an important role in impacting both the bank’s profitability and risk. Lastly, the results also confirm a major role for a country’s financial development status and regulatory quality in determining the bank’s riskiness.
The remainder of this study is organized as follows. Section 2 provides a brief discussion of the past literature, while sections 3 and 4 explain the data collection and the methodology, respectively, including a short introduction to the random forest models and the relative value indicator. Section 5 discusses our results and findings, whereas section 6 provides some concluding remarks and suggestions for future research.
2. Literature review
A large number of studies have endeavoured to empirically examine determinants of a bank’s performance and risk profile. Generally, a bank’s performance is measured by the return on assets (ROA), return on equity (ROE) and net interest margin (NIM), whereas its risk profile is usually assessed by risk-weighted-assets (RWA) ratio, non-performing loans (NPL) ratio and the standard deviation of ROA or ROE (Li et al., Citation2021; Mirzaei et al., Citation2013; Molyneux & Thornton, Citation1992; Yanikkaya et al., Citation2018).
One of the first studies to examine determinants of a bank’s profitability is Short (Citation1979) who finds a significant role for market concentration, government ownership and assets growth in influencing a bank’s profitability. A more comprehensive empirical assessment is provided by Bourke (Citation1989) who examines the role of a number of internal (overhead expenses, liquidity, ownership and growth) and external (concentration, interest rates and inflation) factors in impacting a bank’s performance as measured by the return on assets and return on equity. Bourke reports that the internal factors as well as concentration have a positive association with the bank’s performance. A similar study by Molyneux and Thornton (Citation1992) on a sample of European banks reports comparable findings. Molyneux and Thornton also find that banks with higher market power tend to exhibit higher risk avoidance.
More recent studies report significant roles for various bank-specific factors such as interest rates spread, non-interest income, off-balance sheet exposure, product diversification, loan provisions, and capital in determining the bank’s profitability and risk profile. For instance, Goddard et al. (Citation2004) report the existence of a significant positive relationship between capital to asset ratio and a bank’s profitability, while Pasiouras and Kosmidou (Citation2007) document the presence of a negative effect of cost-to-income ratio on a bank’s performance in contrast to the positive impact of capital adequacy ratios on the performance (see also, Athanasoglou et al., Citation2008; Berger & Bouwman, Citation2013). The relationship between capital and profitability is found to be directly linked to the bank’s market power and ability to extract economic rent and operate more efficiently. Additionally, Berger and Bouwman (Citation2013) document a significant role for capital in improving small banks’ probability of survival during hard times.
In a related study, Mirzaei et al. (Citation2013) observe that a greater market share in developed markets tend to lead to higher profitability, while also suggesting that policies targeted towards promoting competition may lead to destabilising the individual banks (see also, Saunders & Schumacher, Citation2000). They also report that higher interest-margin revenues in developing markets lead to more profitable and stable banks as banks use these higher margins to cover potential credit losses. In a global study covering 23 developed countries, Berger et al. (Citation2009) report the presence of a negative relationship between market power and overall risk exposure. They also find evidence that while loan portfolio risk increases with market power the additional risk tends to be offset by the higher capital ratios. Further, Valverde and Fernández (Citation2007) report that product diversification boosts profitability and improves market power as it compensates loss in interest-income due to increased competition and shrinking interest rate spreads. Additionally, Angbazo (Citation1997) reports that off-balance sheet (OBS) exposure explains cross-sectional differences in interest rate risk and liquidity risk, which can be attributed to the off-balance sheet hedging activities. Angbazo finds that OBS activities help create a more diversified revenue generating base which reduces overall risk profile.
A number of studies also report the presence of a significant impact on a bank’s performance and riskiness due to several country factors such as gross domestic product (GDP) growth, inflation, regulations, domestic credit and interest rate (Bourke, Citation1989; Molyneux & Thornton, Citation1992). For instance, Pasiouras and Kosmidou (Citation2007) report that European banks’ profitability is significantly impacted by GDP growth and inflation and that this effect differs for domestic versus foreign banks operating in the region (see also, Bolt et al., Citation2012; Dietrich & Wanzenried, Citation2014). Saunders and Schumacher (Citation2000) find that regulatory policies along with macro interest rate volatility play a significant role in determining a bank’s interest margin. They also document the presence of a trade-off between ensuring bank’s solvency through imposing higher capital requirements and lowering the cost of banking services to consumers (i.e. lower interest margins). Similarly, Bolt et al. (Citation2012) document a significant role for long-term interest rates in determining bank profitability during times of high economic growth. Further, Mirzaei et al. (Citation2013) report the presence of a negative relationship between domestic credit as percentage of GDP and bank profitability in emerging markets versus a positive relationship in advanced markets. Mirzaei et al. also document that higher domestic credit leads to significantly higher bank risk in developing markets which could be attributed to the higher default probability and the less recovery potential in these markets (see also, Ash & Huizinga, Citation1999).
Previous studies use different statistical techniques to model the relationship between the bank’s profitability or risk and its determinants. Earlier studies such as Bourke (Citation1989) and Molyneux and Thornton (Citation1992) employ simple linear regression models with variables such as ROA or ROE as the dependent variable and the rest of the determinants as explanatory variables. Later studies, utilize the General Methods of Moments (GMM) models on the basis that such models are able to better address some of the well-known problems present in such settings compared to other techniques such as fixed-effect models (Dietrich & Wanzenried, Citation2014; Maudos, Citation2017; Mirzaei et al., Citation2013; Pasiouras & Kosmidou, Citation2007; Tregenna, Citation2009). For instance, while fixed-effect models account for cross-sectional differences, they fail to account for potential endogeneity with regards to the dependent variable which is addressed by GMM models through employing a dynamic panel data approach (Yanikkaya et al., Citation2018). Moreover, a number of recent studies which focus on bank efficiency utilize various parametric (e.g., stochastic frontier approach) and non-parametric (e.g., data envelopment analysis) frontier analysis methods to determine benchmark or best practice frontiers against which the performance of various banks is then measured (Claeys & Vander Vennet, Citation2008).
3. Data
We obtain the entity-level data needed for our analysis from the Worldscope Database. The Worldscope Database contains detailed profile and financial data on public companies around the world. We extract year-end financial data for the period 2000 to 2019 for all active publicly traded banks with a minimum market capitalization of USD 500 million or equivalent as of the end of 2019.Footnote1 The country-level data is obtained from the International Monetary Fund (IMF) and the World Bank databases. The various variables and their sources are explained in . Our final sample contains 1,245 banks from 66 countries.Footnote2
Table 1. Variable definitions
4. Methodology
4.1. Random forest and relative value importance
We use the relative value importance (RVI) indicator from the random forests (RF) model introduced by Breiman (Citation2001) to assess the contribution of the various studied factors to a bank’s risk and profitability. RF is an ensemble learning method that combines several random algorithms, or decision trees, to arrive at the final output. The RVI is calculated as the average weighted squared improvement to the model as a result of selecting the particular variable at each split (Friedman & Meulman, Citation2003). As highlighted by Biau and Scornet (Citation2016), Hastie et al. (Citation2009) and others, RF gained its popularity from requiring little intervention from researchers as well as its applicability to a wide array of classification and prediction tasks. Furthermore, RF models were found to be significantly less affected by everyday data challenges such as the presence of outliers and missing values (Biau and Scornet, Citation2016; Hastie et al., Citation2009). RF is also robust against many statistical issues impacting the performance of parametric models (e.g., regression analysis) such as multicollinearity or heteroskedasticity. Additionally, RF, like many other machine learning methods, is able to process a large number of input variables even in small samples, while also being largely unaffected by insignificant input variables (Hastie et al., Citation2009). Past studies have found that RF outperforms similar machine learning techniques such as decision trees and has comparable performance to other methods such as neural networks and generalized boosting (Jones et al., Citation2017).
4.2. Model specification and variables
The purpose of this study is to evaluate the relative importance of the different determinants of bank risk and profitability that were identified in past studies. To achieve this, we use a series of RF regressions with a number of profitability and risk proxies as dependent (output) variables:
Where all variables are defined in along with a brief description of each variable and an explanation of the relationship with bank profitability and/or risk. Since risk and return are related with each indicator having an impact on the other, we include the profitability dependent variables (i.e. ROA and NIM) as independent variables in the models using a risk proxy as the dependent variable and vice versa (i.e. including risk-dependent variables as independent variables in the models using a return proxy as a dependent variable). We calculate the RVI values by assigning the most important variable a value of 100 and then re-express the values of all other variables on the same relative scale. We exclude the year and country dummies from the calculation of the RVI values as they are irrelevant to our study and have insignificant contributions to the percentage of variation explained in all cases (less than 2%).
5. Results and discussion
5.1. Descriptive statistics and correlation analysis
reports the summary statistics for the full sample. We can see that the values of some of the variables vary significantly which is expected given the period covered by our sample which includes the early 2000s recession related to the dot-com bubble, the global financial crisis in 2008 and the European debt crisis in 2010 in addition to several other smaller events.
Table 2. Descriptive statistics
In order to provide a preliminary assessment of the relationship between the various indicators, we report the correlation coefficients between the various dependent variables and the independent variables in . First of all, the vast majority of the correlation coefficients appear to be highly significant at the 1% significance level. Profitability measures (ROA/NIM) seem to be highly positively correlated with capital size (CAP), Lerner’s index (LRN), interest rate spread (IRS), non-interest income (NIC), GDP growth (GDP) and policy rate (POL). Further, Lerner’s index (LRN), financial development (FDI) and regulatory quality (REG) appear to be significantly negatively correlated with the bank’s risk profile as measured by two risk indicators (NPL and SVL). They are also positively correlated with the third risk indicator (ZSC) which tends to have higher values for less risky banks. Many of these observations seem to be in line with prior findings in the literature. For instance, several studies have documented the presence of significant relationships between the bank’s riskiness on one side and market power, local financial markets and regulatory environment on the other (Berger et al., Citation2009; Mirzaei et al., Citation2013; Saunders & Schumacher, Citation2000). Other studies have also linked indicators such product diversification, interest rate spread and business cycles to bank’s profitability (Valverde & Fernández, Citation2007; Pasiouras & Kosmidou, Citation2007). While most of the reported correlations are highly significant, many of the correlation coefficients are relatively low especially in the cases including any of the risk indicators (NPL, ZSC and SVL). In the context of our study, this suggests that a bank’s risk and profitability are likely to be impacted by a large group of variables as opposed to a few variables only. We examine such relationships in more details in the next section.
Table 3. Spearman’s rank correlation
5.2. RVIs of the determinants of bank risk and profitability
We report the results of the RVI values from the various RF models in in order from the most important (top) to the least important (bottom) variable. Before discussing the RVI values, it is worth highlighting that three of the five models explain more than half of the total variance. The values of the percentage of variance explained are (the dependent variable is specified in the parentheses): 70.41% (ROA), 65.54% (NIM), 42.14% (NPL), 25.25% (ZSC) and 57.16% (SVL).
show the RVI values for the models containing our profitability indicators (ROA and NIM, respectively) as dependent variables. First important observation is that none of the top five determinants of ROA are country-level variables which indicates that ROA is largely determined by bank-specific characteristics and performance. Next, it is also important to note that Lerner’s index (LRN), a proxy of market power, has almost twice as much importance in determining a bank’s ROA as the next best indicator, ZSC, which is an indicator of a bank’s risk profile. This observation is supported by past findings in the literature regarding the role of market power in determining the bank’s profitability (Berger et al., Citation2009; Maudos & Solís, Citation2009). The rest of the indicators show that bank risk profile (ZSC), operating efficiency (OPX), product diversification (NIC) and capital strength (CAP), play an important role in determining a bank’s ROA. Further, the five most important determinants of a bank NIM are (in order of importance): interest rate spread (IRS), operating efficiency (OPX), policy interest rate (POL), financial development index (FDI) and size (SIZ). Once again, albeit it is now the first two variables rather than the first one only, IRS and OPX, are twice as important as the next best variable, POL, in determining the bank’s NIM. Also, as expected, two of the top five indicators are related to interest rate (IRS and POL). Interestingly, the results show OPX as one of the top five indicators again, which confirms that a bank’s efficiency with regards to its expenditures plays a significant role in impacting its performance as measured by both ROA and NIM. Further, the results also indicate that a bank’s NIM is largely dependent on the development status of the market in which it operates as well as market power as measured by FDI and SIZ, respectively. A highly developed financial market is more likely to offer cheaper and more diversified funding resources for banks which explains the important role played by such indicator. Moreover, a bank’s size and market power will determine its ability to obtain financing at attractive rates thus affecting its NIM. One interesting factor is non-interest income (NIC) which appears among the top ten determinants of NIM despite having no direct relation to interest rates. This observation is explained by the fact that banks rely on financing products to attract other non-interest related sources of income such as structuring, issuance, advisory and other fee-based products and vice versa, therefore creating a strong link between NIM and NIC.
show the RVI values for the models containing our risk indicators (NPL, ZSC and SVL, respectively) as dependent variables. In contrast to the results of the models using the profitability measures, the current models show that country-level variables dominate in terms of their relative importance in determining the various risk indicators with at least four of the top five determinants being country-level variables. This suggests that the riskiness of the bank’s portfolio is largely determined by macro rather than micro-related factors, especially those related to the growth of domestic credit and the business cycle as well as the development of the local financial markets and regulatory environment. Overall, it is worth noting that while models using profitability measures appear to have some dominant top determinants with twice the relative value of other determinants, the models using risk measures, appear to have more distributed RVI values with no significant domination by any one or two variables. There are several economic and policy implications arising from our findings in this study. First, the relatively high importance of country-level variables in determining the bank’s riskiness suggest that regulators and policy makers have a big role to play in the process. It also suggests that steps to improve the stability of the banking system should start at the level of the regulations and the environment in which the banks conduct their business. Second, our results show that size and market power play an important positive role in impacting a bank’s profitability and riskiness. This implies that smaller banks may have relatively lower profitability and riskier assets, which suggests the need for some policies and regulations to be specifically targeted towards ensuring the soundness and stability of this group of institutions. This is in contrast with the recent regulatory changes which focused primarily on regulating the systemically important banks. Lastly, the finding regarding the relatively important role played by operating efficiency in impacting the bank’s profitability indicates the presence of some disparity in how efficiently banks operate. This suggests that significant improvements in profitability can be brought by improving operational efficiency in some banks.
6. Conclusion
We use the relative value importance indicator from the random forest model to analyse the comparative importance of a number of determinants of bank risk and profitability. The analysis shows that a bank’s profitability is largely determined by bank-specific factors, while a bank’s risk is mainly impacted by country-level factors. We find that market power and size proxies play a significant role in determining both the bank’s profitability and its risk profile. The results also suggest a major role for a country’s financial development status and regulatory quality in impacting the bank’s riskiness. Lastly, the analysis shows that a bank’s risk profile is determined by a number of variables with close relative importance levels, while a bank’s profitability is determined by small number of dominant variables with the remaining variables having much less importance. Future research can focus on studying changes in banks’ profitability and riskiness arising from sudden or structural changes to some of the important variables identified in this study. Analysing such events will provide better insights into the role of these variables in influencing the bank’s profitability and riskiness and the magnitude of this impact. This will also provide regulators and policy makers with better understanding of what areas to focus on in order to ensure the soundness and stability of the banking system and its participants.
Disclosure statement
No potential conflict of interest was reported by the author(s).
Additional information
Funding
Notes on contributors
Nawaf Almaskati
Dr. Nawaf Almaskati is an active researcher in financial markets, corporate governance and machine learning with several publications in the field. Dr. Almaskati’s research focuses on empirically examining corporate governance and capital markets topics using various econometric and machine learning techniques to provide new insights into existing problems.
Notes
1. We select the entities based on the industry classification (Banks) in the Thomson Reuters Business Classification (TRBC) field.
2. We exclude countries with less than three banks in our final sample.
References
- Angbazo, L. (1997). Commercial bank net interest margins, default risk, interest-rate risk, and off-balance sheet banking. Journal of Banking & Finance, 21(1), 55–15. https://doi.org/10.1016/S0378-4266(96)00025-8
- Ash, D.-K., & Huizinga, H. (1999). Determinants of commercial bank interest margins and profitability: Some international evidence. The World Bank Economic Review, 13(2), 379–408. https://doi.org/10.1093/wber/13.2.379
- Athanasoglou, P. P., Brissimis, S. N., & Delis, M. D. (2008). Bank-specific, industry-specific and macroeconomic determinants of bank profitability. Journal of International Financial Markets, Institutions and Money, 18(2), 121–136. https://doi.org/10.1016/j.intfin.2006.07.001
- Berger, A. N., & Bouwman, C. H. S. (2013). How does capital affect bank performance during financial crises? Journal of Financial Economics, 109(1), 146–176. https://doi.org/10.1016/j.jfineco.2013.02.008
- Berger, A. N., Klapper, L. F., & Turk-Ariss, R. (2009). Bank competition and financial stability. Journal of Financial Services Research, 35(2), 99–118. https://doi.org/10.1007/s10693-008-0050-7
- Biau, G., & Scornet, E. (2016). A random forest guided tour. TEST, 25(2), 197–227. https://doi.org/10.1007/s11749-016-0481-7
- Bolt, W., de Haan, L., Hoeberichts, M., van Oordt, M. R. C., & Swank, J. (2012). Bank profitability during recessions. Journal of Banking & Finance, 36(9), 2552–2564. https://doi.org/10.1016/j.jbankfin.2012.05.011
- Bourke, P. (1989). Concentration and other determinants of bank profitability in Europe, North America and Australia. Journal of Banking & Finance, 13(1), 65–79. https://doi.org/10.1016/0378-4266(89)90020-4
- Breiman, L. (2001). Random forests. Machine Learning, 45(1), 5–32. https://doi.org/10.1023/A:1010933404324
- Claeys, S., & Vander Vennet, R. (2008). Determinants of bank interest margins in Central and Eastern Europe: A comparison with the West. Economic Systems, 32(2), 197–216. https://doi.org/10.1016/j.ecosys.2007.04.001
- Dietrich, A., & Wanzenried, G. (2014). The determinants of commercial banking profitability in low-, middle-, and high-income countries. The Quarterly Review of Economics and Finance, 54(3), 337–354. https://doi.org/10.1016/j.qref.2014.03.001
- Friedman, J. H., & Meulman, J. J. (2003). Multiple additive regression trees with application in epidemiology. Statistics in Medicine, 22(9), 1365–1381. https://doi.org/10.1002/sim.1501
- Goddard, J., Molyneux, P., & Wilson, J. O. S. (2004). The profitability of European banks: A cross-sectional and dynamic panel analysis. The Manchester School, 72(3), 363–381. https://doi.org/10.1111/j.1467-9957.2004.00397.x
- Hastie, T., Tibshirani, R., & Friedman, J. (2009). The elements of statistical learning: Data mining, inference, and prediction. Springer Science & Business Media.
- Jones, S., Johnstone, D., & Wilson, R. (2017). Predicting corporate bankruptcy: An evaluation of alternative statistical frameworks. Journal of Business Finance & Accounting, 44(1–2), 3–34. https://doi.org/10.1111/jbfa.12218
- Li, X., Feng, H., Zhao, S., & Carter, D. A. (2021). The effect of revenue diversification on bank profitability and risk during the COVID-19 pandemic. Finance Research Letters, 101957.
- Maudos, J., & Solís, L. (2009). The determinants of net interest income in the Mexican banking system: An integrated model. Journal of Banking & Finance, 33(10), 1920–1931. https://doi.org/10.1016/j.jbankfin.2009.04.012
- Maudos, J. (2017). Income structure, profitability and risk in the European banking sector: The impact of the crisis. Research in International Business and Finance, 39, 85–101. https://doi.org/10.1016/j.ribaf.2016.07.034
- Mirzaei, A., Moore, T., & Liu, G. (2013). Does market structure matter on banks’ profitability and stability? Emerging vs. advanced economies. Journal of Banking & Finance, 37(8), 2920–2937. https://doi.org/10.1016/j.jbankfin.2013.04.031
- Molyneux, P., & Thornton, J. (1992). Determinants of European bank profitability: A note. Journal of Banking & Finance, 16(6), 1173–1178. https://doi.org/10.1016/0378-4266(92)90065-8
- Pasiouras, F., & Kosmidou, K. (2007). Factors influencing the profitability of domestic and foreign commercial banks in the European Union. Research in International Business and Finance, 21(2), 222–237. https://doi.org/10.1016/j.ribaf.2006.03.007
- Saunders, A., & Schumacher, L. (2000). The determinants of bank interest rate margins: An international study. Journal of International Money and Finance, 19(6), 813–832. https://doi.org/10.1016/S0261-5606(00)00033-4
- Short, B. K. (1979). The relation between commercial bank profit rates and banking concentration in Canada, Western Europe, and Japan. Journal of Banking & Finance, 3(3), 209–219. https://doi.org/10.1016/0378-4266(79)90016-5
- Tregenna, F. (2009). The fat years: The structure and profitability of the US banking sector in the pre-crisis period. Cambridge Journal of Economics, 33(4), 609–632. https://doi.org/10.1093/cje/bep025
- Valverde, S. C., & Fernández, F. R. (2007). The determinants of bank margins in European banking. Journal of Banking & Finance, 31(7), 2043–2063. https://doi.org/10.1016/j.jbankfin.2006.06.017
- Yanikkaya, H., Gumus, N., & Pabuccu, Y. U. (2018). How profitability differs between conventional and Islamic banks: A dynamic panel data approach. Pacific-Basin Finance Journal, 48, 99–111. https://doi.org/10.1016/j.pacfin.2018.01.006