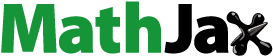
Abstract
This study examines the factors affecting farmers’ access to formal credit in Basona Worana District, North Showa Zone, Amhara Regional State, Ethiopia. The study used cross-sectional data while conducting a survey. It was conducted from November 2019 to June 2020 G.C. A multistage random sampling technique was used, and 299 smallholders’ farmers were selected and fully took part in the survey. Quantitative data were collected using a structured questionnaire to identify the main factors of formal financial credit access. Of the total 299 participants, only 72 (24.1%) took formal financial credit from lending institutions. The data were analyzed by descriptive statistics and logistic regression analysis. The regression result reveals that age, sex of household head, family size, extension contacts, off-farm income, interest rate, lending procedure, group lending and Rapid Repayment Period are the main determinant factors that affect formal financial credit access at the household level, and these variables are found to be statistically significant at 1 % and 5 % levels of precision. On the other hand, the other remaining variables like education status, attitude towards credit risk, experience on credit use, farm land size, livestock ownership and distance from lending institutions are found to be statistically insignificant. Thus, policy makers should work to improve credit service supply and amount of credit and assist smallholders to invest the credit on their farming. Lending institutions should revise their group lending system, interest rate, lending procedure and rigid repayment periods.
PUBLIC INTEREST STATEMENT
In Ethiopia, agricultural finance is regarded as a decisive factor in farming production, helping poor farmers to maintain consumption of basic necessities, adopt advanced technology and raise their incomes. But the accessibility of good financial services and access to formal credit are very low in its distribution and amounts. So, this study tried to examine the factors affecting farmers’ access to formal credit in Basona Worana District, North Shoa Zone, Amhara Regional State, Ethiopia. The results revealed that age, sex of household head, family size, extension contacts, off-farm income, interest rate, lending procedure, group lending and Rapid Repayment Period are the main factors that affect formal financial credit access at the household level.Therefore, good access to credit is an effective tool to enhance agricultural productivity, encourage technological adoption and ultimately alleviate poverty.
1. Introduction
Agricultural finance is regarded as a decisive factor input in farming production, helping poor farmers to maintain consumption of basic necessities, adopt advanced technology and raise their incomes (Hoda & Terway, Citation2015). The accessibility of good financial services is considered as one of the engines of economic development. The establishment and expansion of financial serves is also one of the instruments to break the vicious circle of poverty. Governments of less-developed countries have frequently practiced the policies of providing cheap credit to the agricultural sector through financial intermediaries. This cheap credit, it was hoped, would lower the dependence on rural money lenders (Rizwan et al., Citation2019). Therefore, access to credit is an effective tool to enhance agricultural productivity, encourage economic development and thereby alleviate poverty. Accordingly, governments in most developing countries have ambitious efforts aimed at improving credit accessibility to farmers, particularly in rural areas. Moreover, the growing attention in this regard is derived from the view that the provision of credit to rural population is a very effective strategy for poverty reduction (Zeller, Citation1998; Assifaw & Adeba, Citation2016). However, the majority of farmers in developing countries have only limited access to commercial banks and other formal financial institutions.
Credit is crucial in the agricultural sector to enhance the productivity of crops and animals used as food for human beings. Farmers usually obtain low crop production due to the lack of capital, and credit is a capital alternative to enhance productivity in developing countries (Akmal et al., Citation2012; Sattar, Citation2012; Rizwan et al., Citation2019). Numerous factors are involved in low productivity: a low dosage of fertilizer is caused by the lack of capital, and this is one of the major factors involved in low productivity in developing countries like Pakistan (Sattar, Citation2012). Poor farmers generally borrow capital because their income and margins are low (Njeru et al., Citation2016). It is also important in the agricultural sector to make use of advanced technologies to enhance farm productivity. The demand for agricultural credit appears not just among small farmers but also among medium and large farmers, due to the low margins associated with agriculture (Das et al., Citation2009; Julien et al., Citation2021).
Besides, smallholder farmers in developing countries, especially in low-income African countries like Ethiopia, are at the forefront of those who lacked access to formal credit. In that, only 4.7% of adults in rural areas have a loan from formal sources and only 5.9% own a bank account (International Financial Corp (IFC), Citation2014). Regardless of what has been all said, most African farmers still are ignored not only by banks but also by formal Microfinance Institutions (MFIs). As a result, to own resources, these farmers rely on incomes of friends and relatives, remittances and informal money lenders (Gollin, Citation2014; Rizwan et al., Citation2019). It is also noted as it will be harder to farmers who use informal credit use to make improvements, because of high interest rates they are obliged to pay (Ojiako et al., Citation2014; Julien et al., Citation2021). Besides, there is also emerging evidence that showed low utilization of formal financial credits irrespective of the availability of formal financial institutions in different African countries, such as Ethiopia (Getnet, Citation2014), Uganda (Richard & Ramzy, Citation2016), Rwanda (Kopparthi & Alice, Citation2016), Nigeria (Samson & Obademi, Citation2018) and many Asian Countries (Rizwan et al., Citation2019).
The government of Ethiopia has made financial sector reforms in the past two decades, and the financial sector of Ethiopia currently consists of only 3 public and 16 private banks, 31 microfinance institutions, 14 private and 1 public insurance companies, and over 8000 Saving and Credit Cooperatives (SACCOs; Samuel, Citation2020). In Ethiopia, taking this ground into account, the main role of formal financial credit provision is left for the 31 microfinance institutions, which all may not be as equal as a single public bank in capacity. Besides, distribution of the microfinance institutions in the country is also not equitable and most are residing in urban areas than rural. In that, 14 microfinance institutions are working only in Addis Ababa, where almost no farming is practiced, and the rest are owned by regional governments (Aderaw and Singh, Citation2016; Samuel, Citation2020).
Among the smallholder farmers in different parts of Ethiopia, those in the Amhara region, where the present study area Basona Worana district is found, smallholder farmers faced credit constraints, due to limited outreach capacity of microfinance institution in the region; Specifically, the one popular in the region called Amhara Credit and Saving Institute (ACSI) with its limited outreach capacity is only available in the district (Aderaw and Singh, Citation2016; Samuel, Citation2020). In addition, farmers in the Basona Worana district are almost all smallholders at the subsistence level, who do not possess commercially intensive farming. Besides, poor credit access coverage (20% from total population) is due to lack or less access for different formal financial sectors in the study areas. Thus, they mainly need to get improved access to formal financial credit so as to make improvements of their livelihood. As well, the use of formal financial credit and determinants that affect access to formal credit was not studied at all to the best of the knowledge of researchers. Therefore, based on these grounds, this study was performed to consider the status of smallholder farmers' access to formal credit and examine the main determinant factors that affect access among smallholder farmers in the Basona Worana district.
To address these, the study answers the following two questions: what are the main determinant factors that affect smallholder farmers' access to formal credit in the Basona Worana district what are the major obstacles encountered among smallholder farmers in the Basona Worana District in order to access formal financial credit? Finally, the rest of this paper is organized as follows: Section 2 presents different reviews of literature, and Section 3 discusses methodology with an emphasis on the description of the logit models applied in the study. Section 4 emphasizes discussion of results with its interpretation, and finally, Section 5 presents conclusions and key policy recommendations.
2. Literature review
The objective of this section is to explore the empirical studies that seek to establish the linkages between the main determinant factors that affect smallholder farmers' access to formal credit in the agriculture financial sector. Different theories are essential tools that logically frame to see the relationships of variables in a formal way that can be expressed to empirical models. It becomes suitable to make econometric analysis. This study used random utility model/theory to frame the farmers' choice for different input options for their production maximizations. It is commonly based on different assumptions as the study assumed that farmers are rational decision-makers who maximize the utility in their farming activities, so do are to use credit from formal credit institutions. Second, it assumed that smallholder farmers usually run out of resource inputs in and will look for a variety of mechanisms to alleviate the shortage and improve their productivity, with a special aspiration to financial credit from formal sources. Finally, the study assumed that farmers who fail to access/use formal credit will continue searching for other mechanisms, most probably seeking for informal credits’ sources. Thus, based on those three assumptions, the model can be written as
where i = 1, 2, 3 … n are the individual smallholder farmers (Household heads) and j = 0 and 1 are the alternatives that a farmer's (i) formal credit ís constrained or unconstrained; Xij, vector, are the determinant factors that influence farmers’ access to formal credit, and ij is the random error term (disturbance term).
Besides, different studies have been performed by different researchers to address the factors that determine smallholder famers' access to formal credit. A majority of studies in the literature focused on factors that affect smallholder farmers' access to formal credit in rural areas especially for developing counties (see Amjad & Hasnu, Citation2007; Dzadze et al., Citation2012; Chauke et al., Citation2013; Muhongayirea et al., Citation2013; Nouman et al., Citation2013; Baiyegunhi & Fraser, Citation2014; Sebatta et al., Citation2014; Anang et al., Citation2015; Nyaga & Nzulwa, Citation2017; Rizwan et al., Citation2019; Masaood & Keshav, Citation2020; Julien et al., Citation2021). In these studies, the results showed that sex, age, membership in a financial solidarity group, sown area, marital statuses, type of association and interest rate are the main significant variables influencing the demand for formal credit directly.
We also find many research studies focused on the relationship between the dependent variables (access to formal credit) and other explanatory variables or factors like Demographic factors such as age, sex and family size and Socioeconomic factors such as education status, attitude towards credit risk, experience on credit use, extension contract, off-farm income, farmland size and livestock ownership (Anang et al., Citation2015; Assogba et al., Citation2017; Elias et al., Citation2015; Gbigbi, Citation2017; Kiplimo et al., Citation2015; Musabanganji et al., Citation2015; Nyaga & Nzulwa, Citation2017; Samson & Obademi, Citation2018). Other works of Julien et al., Citation2021; Masaood & Keshav, Citation2020; Rizwan et al., Citation2019; Samuel, Citation2020, considered the Institutional/political factors such as interest rate, distance from lending institutions, lending procedures and rigid repayment period. In their findings, many variables significantly affect formal credit access in many African Countries and other developing countries.
In the Ethiopian context, most of the existing researches/studies have only descriptively examined the role of formal credit access in agricultural production in rural areas. The studies considered the demographic, socioeconomic and Institutional factors for their analysis (Dube et al., Citation2015; Mebrate, Citation2015; Abafita et al., Citation2016; Assifaw & Adeba, Citation2016; Samuel, Citation2020). After critical reviewing empirical literature studies, the present study identified the following gaps. Most of the studies in Ethiopia and other countries used the direct elicitation theoretical approach to measure the status of households’ access to credit, as it is recommended for ease of practicality. But those studies did not further attempt to categorize households based on their credit rationing except simply identifying households as credit constrained or no. Those studies confirmed that classifying households based on their credit rationing type will have an additional value to clearly investigate “why” a given household was credit constrained in a very simplest way.
In addition, due to methodological limitations, especially smaller sample sizes used in some of the studies, inconsistencies of results among studies regarding the determinant were exaggeratedly widened. This can make the possibility of driving facts at the meta level difficult. However, this was limited in Ethiopia’s context; hence, many more empirical studies need to be performed by using a large number of sample sizes and large study area coverage, use more relevant and related variables in the model, incorporate the descriptive study with the inferential model to understand the issue well and drive better policy implications that can aid in improving the livelihoods of smallholder poor farmers in Ethiopia.
3. Methodology
This section presents the methodology parts of the research. Among these, in the first step, we discussed the Research Design and Rationale; second, Description of the study Area, Sample Size Determination and Sampling Procedure; then, Data source and Collection Procedure, Model Specification and Estimation and finally, the Diagnostic Tests for a given model.
3.1. Research design and rationale
А research design is the master plan of a research that shows the exact ways a researcher should use to precisely answer the research questions and attain the objectives (Snauders et al., Citation2009). Regarding this, the questions and objectives of our study were clearly defined. Some of the objectives such as examine farmers' access to formal credit and tagging farmers into the respective type of credit rationing need a descriptive approach, while, investigating associations between the dependent and independent variables entail an explanatory approach. Therefore, our study utilized both descriptive and explanatory design approaches. In addition, apart from its descriptive and explanatory nature, our study collected data at specific period of time in order to assess smallholder farmers' access to formal credit and investigate the determinants. Thus, based on the time horizon dimension of research design classification, our study used the cross-sectional data type for this study.
3.2. Description of the study Area
. shows the map of Basona Worana district, North Showa zone, Amhara region, Ethiopia. The district is closely bordering the North Showa zone capital: Debre Berhan town. It is located 130 km far from the country’s capital city Addis Ababa and at 695 km from the region’s capital Bahir Dar. The altitude of the area ranges from 1500 to 3400 meter above the sea level. In addition to the rural kebeles of Debre Berhan, the district is a neighbor of other districts, such as Tarma Ber and MojanaWodera in the north, Angolelana Tera in the south, Ankober in the east and Siyadebirna Wayu, Moretna Jiru and Abichuna Gnea in the west. The district covers an area of 1208.17 km2, which is divided into 31 rural kebeles. Its average annual daily temperature ranges from 9 to 15 °C, with a rainfall of 1100 mm (https://en.m.wikipedia.org/wiki/Basona Worana). According to the 2018 population projection estimation, the district has a total population of 140,386.0, in which 71, 439.0 are male and 68, 947.0 are female. Besides, of the total population, 138,264.0 are rural dwellers and the rest 2,122.0 are urban residents (Central Statistical Agency (CSA), Citation2018).
3.3. Sample size determination
To make generalization about the whole population, different sampling designs and procedures are used to get the truly representative sample. Thus, this section presents the sampling designs and procedures that were employed for this study. The sample size for the present study was determined using the single population proportion cross-sectional formula and the assumptions indicated (Singh & Masuku, Citation2014),
, where
•n is the desired sample size.
•Z is the standard normal deviation at the 95% confidence level (1.96)
•P is the proportion of expected formal credit access farmers (22.78%) *
•q = 1-P (1-0.2278) =0.7722, which is the proportion of expected formal credit not accessible by farmers. By definition, q is equal to 1–P (the expected proportion)
•d is the degree of accuracy desired, 5% (0.05).
Based on this, 270.31 ≈ 271, and the study considered that the nonresponse/refusal rate was 10%: 271*0.10 = 27.1 ≈ 28;
Therefore, our study sample size was 271 + 28 = a total of 299 households (household heads).
NB: The proportion of expected formal credit users (P) was determined based on secondary data obtained with permission: from ACSI, which is the main formal MFI in the region as well as in the district. The total credit for user smallholder farmers (households), in the year 2016/17 from the three branches of ACSI in the Basona Worana district, i.e., Debre Berhan Zuria, Keyt and Goshe Bado was, 2700, 2650 and 2028, respectively. Using this and the total number of smallholder households in rural kebeles of the district, equal to 32, 389, obtained from the Basona Worana District Agriculture Office, the researcher roughly estimated the proportion of expected formal credit users in the district as *100 = 22.779 ≈ 22.78%.
3.4. Sampling procedure
A total of thirty-one kebeles are found in the Basona Worana district. These kebeles cover a wide area, so including all of them in the study population was impractical due to time and resource limitations. To get the representative sample, our study applied a multistage sampling method.
indicates the sampling stages of this study: such that, in the first stage kebeles were selected by simple random sampling (lottery method). Before the selection, one of the kebeles called Gudoberet town was considered as urban kebele and hence excluded from the source population. From the total thirty rural kebeles of the district, eleven kebeles that accounted nearly 36.7% were selected. The kebeles were classified into Dega, Woina Dega and Kolla agro ecological zones of 14, 10 and 6 rural kebeles in each, respectively. Based on this, the eleven sample kebeles were proportionally allotted to each agro-ecological zone, that is, (14 × 11)/30 = 5.13 ≈ 5 kebeles from Dega; (10 × 11)/30 = 3.67 ≈ 4 kebeles from Woinadega and (6 × 11)/30 = 2.20 ≈ 2 kebeles from Kolla.
Table 1. Selected kebeles of Basona Worana District, all households and sample size
In the second stage, households were selected by systematic random sampling. That is, the first household in a given kebele was selected randomly, and then the next household was the (1 + k)th household, the third (1 + 2k)th, fourth (1 + 3k)th, etc., where k was calculated by dividing the total number of households in a given kebele by the number of households allotted for the kebele. The list of households in each of the Rural Kebele Administration Office was also used as a frame.
Key:HHs = Households, AEZ = Agro Ecological Zone
3.5. Data source and collection procedure
Primary data were collected through the administration of a semistructured and personal interview by a team of 6 trained enumerators to 299 farmers considered. Furthermore, secondary data were collected from published and unpublished reports, researcher’s website and already available documents of secondary sources such as Basona Worana District Office of Agriculture and Rural Development and other related offices.
The data collection procedures are as follows: first, the questionnaire was first prepared in English, translated to Amharic and back to English to ensure language use consistency. Second, all the data collection tools were pilot tested to check reliability and validity before the actual data collection. Therefore, taking the information of the pilot study, reliability was tested by Cronbach's alpha, with a value of 0.785 indicating strong reliability. Finally, the actual data collection was performed with the formal permission written letter from Debre Berhan University with approval of Basona Worana district administrative office and each rural kebele. The quantitative data collection was carried out by the face-to-face interview of smallholder farmers by trained data collectors. The data collectors were agriculture extension workers (development agents), selected from a different rural kebele, and were assigned for each sampled kebele to avoid interviewer and interviewee bias. For ensuring data quality, all the interviews were performed in the local language (Amharic). As well, on spot checks, reinterviewing and vigilant examination of completed questionnaires and quality of the recordings were performed through daily supervisions with the close attendant of the researcher.
3.6. Model specification and estimation
Theoretical models are essential tools that logically frame relationships of variables in a formal way that can be expressed mathematically to empirical models and become suitable to perform econometric analysis. As a result, this study develops a theoretical framework on smallholder farmers' access to formal credit using the random utility model specified by Greene (Citation2003). The random utility model is commonly used as a framework for determining of farmers' choice for different input options. Based on three assumptions that are mentioned in the literature part and the used random utility model, the study model can be written as
The probability of the status of a particular farmer to be a particular alternative j(0 or 1) is given by the probability that the utility of that alternative to the farmer is greater than the utility to that farmer of other alternative J and alternative K and the determinant factors that can affect the farmers' access to source J. These determinant factors set by the present study to affect smallholder farmers' access to formal credit were specified as demographic factors, institutional factors and economic factors.
Hence, Uij is the total of all factors that enable a farmer to have access to formal credit, which is greater than the Uik factors that hinder a farmer to have access to formal credit. Therefore, the probability of farmer (i) to access the formal credit source is the Function (F) of Uij and can be rewritten as
- (3)
where Uij is the utility function of j factors including the determinant set, as demographic factors, socioeconomic and institutional factors on the (i)th farmer, and it can be written as
-, (4)
Thus, as the present study applied the direct elicitation theoretical approach of measuring households' access to formal credit,
- (5)
Therefore, based on Equation 5, the study estimates the model by the logistic regression model. The logistic regression model has got advantage over the others in the analysis of the dichotomous outcome variable in that it is an extremely flexible and easily used model from the mathematical point of view and results in a meaningful interpretation (Greene, Citation2003) Finally, the logit model was selected or considered for estimation as follows:
(6)
where Pi is the the probability that an individual has access to formal credit;
-e denotes the base of natural logarithms, which is approximately equal to 2.718;
-Xi represents the ithexplanatory variables;
-α is the constant to be estimated and β is the coefficient to be estimated.
The logit model can be written in terms of the odds and log of odds, which enables one to understand the interpretation of the coefficients. The odds ratio implies the ratio of the probability (Pi) that an individual would choose an alternative to the probability (1-Pi) that he/she would not choose it,
If Ui is then considered as the farmer (i) status of having access or not having access credit from formal sources representing the dichotomous dependent variable and again denoted by (Y) and the disturbance term i is taken into account, the logit model became
Therefore, presents all explanatory variables grouped into three categories, i.e., Demographic:age, sex and family size; Socioeconomic: education status, attitude toward credit risk, experience on credit use, extension contract, off-farm income, farmland size and livestock ownership and Institutional factors: interest rate, distance from lending institutions, lending procedures and rigid repayment period was set as follows
Table 2. Description variables used in the logit model
Y(1/0) = α+ β1AGE+β2SEX+β4FAMS+β3EDU+β5ACR+β6ECU+β7EC+β8OFFIN+β9FLS +β10LIVA+β11IR+β12DFLI+β13LP+β14GL+β15RRP+
3.7. Diagnostic tests
Before testing important variables into the multiple regression models, it is necessary to test multicollinearity problems among continuous variables and check associations among discrete variables, which seriously affect the parameter estimates. According to Gujarati (Citation2009), multicollinearity refers to a situation where it becomes difficult to identify the separate effect of independent variables on the dependent variable because of the existing strong relationship among them. In other words, multicollinearity is a situation where explanatory variables are highly correlated. There are two measures that are often suggested to test the existence of multicollinearity. These are Variance Inflation Factor (VIF) for association among the continuous explanatory variables and Contingency Coefficients (CC) for dummy or discrete variables. As another diagnostic test for heteroskedasticity: it has diverse variances between residual terms. To detect the heteroscedasticity problem, the study uses the Breusch-Pagan/Cook-Weisberg test. The result shows that even variance is constant at H0Prob>chi2 = 0.000, resulting in rejection of H0, which means that there is problem of heteroscedasticity. To minimize this problem, the study applied the robust logistic regression.
4. Results and discussion
4.1. Descriptive analyses
The study uses descriptive statistics and econometric models. The study used continuous and dummy variables for its descriptive analyses. For the continuous variable, consider the mean value of the variables and standard deviation, which is a measure of dispersion or spread of variable(s), whereas for dummy variables, we used the frequency table for their number and percentages. According to survey results, the age of smallholder household heads participated in the study ranged from 18 to 49 years with an average of 31.43. The average number of family size is 5.27. The family size of a household varies from two to twelve family members who are living in the study area. Besides, 76.3% of the sample households are male-headed family, while the remaining 23.7% of the households are female-headed. Out of 299 households, the education level, 164 (54.8 %) of the household heads are literate. 142 (47.8%) households do not anticipate that the use of credit is risky, i.e., attitude toward credit risk. Besides, 72(24.1%) households have more experience on formal credit access. But the majority, 226 households, have no experience of how to use formal credit for their economic activities. In the case of extension contact, there are 2.44 days on average contract with an extension officer that the household head has within the year.
In relation to off-farm income availability of the household, only 109 (36.5%) of the total smallholder farmer household heads participated in our study replied, and they had additional income from nonfarm activities, whereas the rest of the participants (nearly two-third of total) had no income except farming. For the farm land size, the average farmland size was 1.88 hectare and its ranges from 1.2 hectare to 3.0 hectare. Besides, the average livestock owned is 3.2 TLU (Total Livestock Unit). The survey result shows that the average distance of households from formal credit lender institutions is 6.6 kilometer and it ranges from one to nine kilometer. From 299 households, 247 (82.6%) of households said that the lending interest rate of formal credit is high. In case of the lending procedure, 141(47.4%) household’s perception the availability of formal credit institutions in the area says easy or simple. In the case of group lending, 184 (61.5%) household’s perceptions toward forming group to take credit from formal sectors say that it is difficult to take credit. Finally, in regard to Rapid Repayment rate, the majority of households, 236 (78.9%) households, usually agree to repay their loans when their crops are harvested (see Appendices B and C).
4.2. Factors affecting access to formal financial credit by households
This section presents the econometric estimation of the logit model, in which variables that significantly affect the use of formal financial credit. In logit estimation of the access to formal financial credit: sex of household head, family size, extension contacts, off-farm income, interest rate, lending procedure, group lending and Rapid Repayment Period are statistically significant at 1 % and 5 % levels of precision. According to the logit output results, the significant variables are the main determinant factors for accessing formal financial credit estimation. On the other hand, the remaining variables are found to be statistically insignificant (see ).
Table 3. Logit estimation for accessing formal financial credit
The data were estimated using Stata software application version 11.2. From the logit regression result depicted in the table, we can observe that the explanatory variables identified in the model sufficiently explain the variation in the dependent variable, which was shown by the high value of Pseudo R2 (=0.3628). Moreover, probability of chi2 is statistically significant at 1 percent, which indicates that all explanatory variables taken together are significant in explaining the dependent variable in the model.
Before estimating, the study used different diagnostic tests forFootnote1 multicollinearity, which is tested by theFootnote2 VIF,Footnote3 TOL test andFootnote4 Spearman’s correlation coefficient matrix for access to the formal financial credit model. From the findings, the correlation coefficient values are not found to show perfect correlation (negative and positive) between the variables.Footnote5 Heteroscedasticity is also tested by the Breusch-Pagan test. The test assures the presence of heteroscedasticity (has no constant variance in εi) and study regressed by robust logistic regression to solve the problems. Besides, Conducted Ramsey test for omitted variables and its result F (20,263) = 1.04(0.4159). The result indicates that it is statistically insignificant, i.e., there is no omitted variable during the study model setting. Therefore, the model can be valid to determine variables that significantly determine access to formal financial credit by households in the study area.
After identifying the main factors that affect access to formal financial credit by the logit model, the marginal effect of the method was used to estimate the explanatory variables on dependent variables. As can be seen from , age, sex of household head, family size, extension contacts, off-farm income, interest rate, lending procedure, group lending and Rapid Repayment Period are the main determinant factors to access the formal financial credit at the household level, and those variables are found to be statistically significant at 1 % and 5 % levels of precision. On the other hand, the remaining variables like education status, attitude toward credit risk, experience on credit use, farm land size, livestock ownership and distance from lending institutions are found to be statistically insignificant. Thus, the discussion for all significant variable mentioned as below,
The coefficient of age is positive; this indicates that as household age increases, more Baiyegunhi, L. S., & Fraser, G. G. (2014) probability to access formal financial credit is present than the young one. This means that, if household age increases by one more age, keeping other variables constant, the probability of accessing formal financial credit increases by 0.7%. This can be due to the fact that as household head age increases, the head becomes more and more experienced and aware of credit access and use. As well, household heads build more social acceptance while they living in the village for more year and he can easily make groups for credit. This result is consistent with the finding of Sebatta et al., Citation2014; Anang et al., Citation2015: Mebrate, Citation2015; Samuel, Citation2020.
The coefficient of sex is positive; this indicates that the men-headed households have more probability to access formal financial credit than female-headed households. This means that, if the family member headed by men, keeping other variables constant, the probability of accessing formal financial credit rises by 11%. This can be due to the fact that women have rare or less access to formal financial credit and women have less opportunities to control economic resources in most societies of developing countries (Baiyegunhi, L. S., & Fraser, G. G. (2014) Mebrate, Citation2015; Gbigbi, Citation2017; Julien et al., Citation2021).
The coefficient of family size is positive; this indicates that the more family member households have more probability to access get formal financial credit than less family members. This means that, if the family member increases by one more individual, keeping other variables constant, the probability of accessing or getting formal financial credit rises by 3%. This can be due to the fact that the large family size can self-engage in cultivation of farm crops and have an opportunity to get more family labor for production and generating more income than less family members. This finding is similar to the works of Baiyegunhi, L. S., & Fraser, G. G. (2014) Mebrate, Citation2015; Assifaw & Adeba, Citation2016; Masaood & Keshav, Citation2020.
The coefficient of extension contact is negative; This indicates that as the number of contact days of smallholder farmers with agriculture extension workers increases, their likelihood to access formal financial credit will decline. This means that, if the number of contact days increases by one more contact, keeping other variables constant, the probability of getting formal financial credit decreases by 4%. This might be due to the fact that the agricultural extension workers never recommend smallholder farmers in the study area to use formal credit with their current status because the farmers use their credit for home consumption purposes and the creditors need big collateral for their credit in addition to group landings. As a result, the study finding is different from the findings of Dzadze et al., Citation2012; Anang et al., Citation2015; Dube et al., Citation2015: Masaood & Keshav, Citation2020; Samuel, Citation2020.
The coefficient of off-farm income is positive; this indicates that the households with more off-farm income have more probability to access formal financial credit than those without off-farm income. This means that, if households with more off-farm income, keeping other variables constant, the probability of accessing formal financial credit increases by 20%. This can be due to the fact that most of the credit users utilize the credit taken on household consumption and start nonfarm tasks for getting off-farm income to cover their credit. This finding is similar to the works of Muhongayirea et al. Citation2013 Dube et al., Citation2015; Kiplimo et al., Citation2015; Gbigbi, Citation2017; Masaood & Keshav, Citation2020.
The coefficient of interest rate is negative; this indicates that credits with a high interest rate have less probability to access formal financial credit than credit with a low interest rate. This means that, if the credit interest rate increases by 1%, keeping other variables constant, the probability of accessing formal financial credit decreases by 19%. This can be due to the fact that the higher level of interest rate can discourage household’s appetite to take credit and it results in less demand to credit by rural households. This finding is consistent with the works of Baiyegunhi, L. S., & Fraser, G. G. (2014) Mebrate, Citation2015; Assifaw & Adeba, Citation2016; Gbigbi, Citation2017; Julien et al., Citation2021.
The coefficient of lending procedure is negative; this indicates that credits with a long-lending procedure have less probability to access formal financial credit than credit with a low short-lending procedure. This means that, if credit with a long-lending procedure increases by one more procedure, keeping other variables constant, the probability of getting formal financial credit decreases by 14%. This can be due to the fact that the long-lending procedure may discourage the farmers to use or get credit from lending institutions assumed if there is a high demand of credit. This finding is consistent with the works of Nouman et al., Citation2013; Mebrate, Citation2015; Assifaw & Adeba, Citation2016and; Julien et al., Citation2021.
The coefficient of group lending is negative; this indicates that credits with group lending have less probability to access formal financial credit than credit with individual lending. This means that, if credit with group lending increases by one more individual in the group, keeping other variables constant, the probability of accessing formal financial credit declines by 23%. This can be due to the fact that most farmers who are unwilling to form groups to take loan from institutions perceived as it may result in free riders‘ problems in the group. This finding is similar to the work of Nouman et al. (Citation2013); Muhongayirea et al. Citation2013 Dube et al. (Citation2015); Mebrate (Citation2015); Assifaw and Adeba (Citation2016) and Masaood and Keshav (Citation2020).
The coefficient of Rigid Repayment period is negative; this indicates that credits with a Rigid Repayment period have less probability to access formal financial credit by households than credit with no Rigid Repayment period. This means that, if credits with a Rigid Repayment period increase by one more rigid repayment period, keeping other variables constant, the probability of accessing formal financial credit declines by 26%. This can be due to the fact that farmers agreed to repay their loans when their crops are harvested. But sometimes, the harvesting time may not be good time on their outputs or may vary by climatic changes due to various reasons and farmers could not repay their loan periodically as per pre-arranged agreement. In this case, the rigid repayment period by formal intuitions does not encourage the farmers to use formal financial credit on rigid repayment period forms. This finding is similar to the work of Muhongayirea et al. Citation2013 Dube et al., Citation2015; Mebrate, Citation2015: Gbigbi, Citation2017; Masaood & Keshav, Citation2020.
5. Conclusion and recommendations
The overall objective of the present study was to examine the determinant factors that affect smallholder farmers’ access to formal credit in Basona Worana district of North Showa Zone, Amhara Regional State, Ethiopia. Data on formal credit use status of the smallholder farmer household heads in the past twelve months of the data collection period and on the potential determinants that assumed to affect households’ access to formal credit, such as demographic, socioeconomic and institutional factors, were collected from 299 smallholder farmer household heads using questionnaire. According to survey results, the age of smallholder household heads participated in the study ranged from 18 to 49 years with an average of 31.43. The average number of family size is 5.27. The family size of a household varies from two to twelve family members who are living in the study area. Besides, 76.3% of the sample households are male-headed family, while the remaining 23.7% of the households are female-headed. Out of 299 households, the education level, 164 (54.8 %) of the household heads are literate. 142 (47.8%) households do not anticipate the use of credit as risky, i.e., attitude toward credit risk. Besides, 72 (24.1%) households have access to formal credit use. In the case of extension contact, there are 2.44 days on average contract with an extension officer that the household head has within the year.
In relation to off-farm income availability of the household, only 109 (36.5%) of the total smallholder farmer household heads participated in our study replied, they had additional income from nonfarm activities and for farm land size, the average farmland size was 1.88 hectare. Besides, the average livestock owned is 3.2 TLU (Total Livestock Unit). The survey result shows that the average distance of households from formal credit lender institutions is 6.6 kilometers and it ranges from one to nine kilometers. Finally, in regard to the Rapid Repayment rate, the majority of households, 236 (78.9%) households, usually agree to repay their loans when their crops are harvested.
Moreover, the regression result reveals that age, sex of household head, family size, extension contacts, off-farm income, interest rate, lending procedure, group lending and Rapid Repayment Period are the main determinant factors that affect access to formal financial credit at the household level, and those variables are found to be statistically significant at 1 % and 5 % levels of precision. On the other hand, the remaining variables like education status, attitude toward credit risk, experience on credit use, farm land size, livestock ownership and distance from lending institutions are found to be statistically insignificant. From the finding, the following policy recommendations are applied to the credit sector in the districts. Thus, policy-makers, especially local leaders, should work toward improving the provision of credit services, improving the amount of credit granted for individual farmers and equitable distribution of lending institutions (branches). Policy-makers should plan ways that integrate different bodies, such as the agricultural sectors, education and other organizations focused on gender to work together. Besides, lending institutions that target smallholder farmers in rural kebeles should revise their lending procedures, the group lending system, their high interest rates, lending procedures and their rigid repayment periods.
Finally, in the areas of future research, this study is a cross-sectional study, which is a one-time snapshot and did not enable us to see the dynamics of the access of formal financial credit in the study areas as well as in the region and the country too. All mentioned factors are not also the only variables that predict smallholder farmers’ access to formal financial credit throughout the area or elsewhere in the country. Hence, similar other studies or future researchers should focus to cover unstudied areas so as to drive meta-data for the zone, the region as well as the country as a whole. If similar other studies need to be conducted in the district, they should stratify the rural kebeles other than agro-ecological zones (we used in the present study), so as to include unselected kebeles by our study. If similar other studies need to be conducted in the district, they need to be innovative enough to include more variables(Determinants-) and considers more sample size to big and large study.
Acknowledgements
We would like to thank the editor and anonymous reviewers for their supportive comments and suggestions.
Disclosure statement
No potential conflict of interest was reported by the author(s).
Additional information
Funding
Notes on contributors
Shewit Kiros
Shewit Kiros is a bank officer at Commercial Bank of Ethiopia, Mekele, Tigray. His research interest includes macroeconomics policy analysis, Monetary Economics, Financial Development issues and international trade.
Getamesay Bekele Meshesha
Getamesay Bekele Meshesha is an Assistant Professor of Economics in the Department of Development Economics, Faculty of Policy and Development Studies at African Leadership Excellence Academy, Ethiopia. His research interest includes microeconomic Policy analysis, Rural-Urban Energy Demand linkages, Health Care Demand, Agriculture and Rural development, Poverty especially Energy Poverty, Impact analysis of different projects and activities, Project Planning and Development, Population and development, and other related areas.
Notes
1. Multicollinearity means the existence of a perfect or exact linear relationship between two or more explanatory variables in the regression model.
2. Variance inflating factor measures the speed with which variance and covariance increase. It is computed as , where r2 is the correlation coefficient, and VIF = 1.82 and r2 = 0.451
3. TOL = 1/VIF or 1-r2 = 1/1.82 = 0.549
4. All Spearman’s correlation coefficients are below 0.8.
5. The probability distribution of random variables (ui) is the same over all observation of x, and in particular, the variance of each ui is the same for all values of the explanatory variables Var(ui) = E {(ui—E(ui) }2 = E(ui)2 = δ2 = Constant variance.
Heteroscedasticity is also tested by the Breusch-Pagan test as Chi2(1) = 41.68(0.000).
References
- Abafita, J., Atkinson, J., & Kim, C. S. (2016). Smallholder commercialization in Ethiopia: Market orientation and participation. International Food Research Journal, 23(4), PP1797–22.
- Aderaw, G., & Singh, M. (2016). Development of financial sector in Ethiopia: Literature review. Journal of Economics and Sustainable Development, 7(7), 9–20., http://www.iiste.org: ISSN 2222-1700 (Paper) ISSN 2222-2855 (Online).
- Akmal, N., Rehman, B., Ali, A., & Shah, H. (2012). The impact of agriculture credit on growth in Pakistan. Asian Journal of Agriculture and Rural Development, 2(4), 579. Asian Economic and Social Society ISSN (P): 2304–1455, ISSN (E): 2224–4433
- Amjad, S., & Hasnu, S. (2007). Smallholders’ access to rural credit: Evidence from Pakistan. The Lahore Journal of Economics, 12(2), 1–25. https://doi.org/10.35536/lje.2007.v12.i2.a1
- Anang, B., Sipiläinen, T., Bäckman, S., & Kola, J. (2015). Factors influencing smallholder farmers’ access to agricultural microcredit in Northern Ghana. Africa Journal of Agricultural Research, 10(24), 2460–2469. https://doi.org/10.5897/AJAR2015.9536
- Assifaw, L., & Adeba, G. (2016). Analysis of factors affecting smallholder farmers’ access to formal credit in Jibat district, West Showa Zone, Ethiopia. International Journal of African and Asian Studies, 25(1), 43–53. http://www.iiste.org ISSN-2409–6938
- Assogba, P. N., Kokoye, S. E. H., Yegbemey, R. N., Djenontin, J. A., Tassou, Z., Pardoe, J., & Yabi, J. A. (2017). Determinants of credit access by smallholder farmers in North-East Benin. Journal of Development and Agricultural Economics, 9(8), 210–216. doi:10.5897/JDAE2017.0814.
- Baiyegunhi, L. S., & Fraser, G. G. (2014). Smallholder farmers’ access to credit in the amathole district municipality, eastern Cape province, South Africa. Journal of Agriculture and Rural Development in the Tropics and Subtropics, 115(2), 79–89. ISSN: 1612-9830 – journal online: www.jarts.info
- Central Statistical Agency (CSA). (2018). Population projection of Ethiopia for all regions; At Woreda level from 2015. Addis Ababa. – 2019.
- Chauke, P. K., Motlhatlhana, T. K., Pfumayaramba, M., & Anim, F. D. K. (2013). Factors influencing access to credit: A case study of smallholder farmers in the Capricorn district of South Africa. African Journal of Agricultural Research, 8(7), 582–585.
- Das, A., Senapati, M., & John, J. (2009). Impact of agricultural credit on agriculture production: An empirical analysis in India. Reserve Bank of India Occasional Papers, 30(2), 75–107. https://www.rbi.org.in/scripts/bs_viewcontent.aspx?Id=2240
- Dube, L., Mariga, T., & Mrema, M. (2015). Determinants of access to formal credit by smallholder tobacco farmers in Makoni district, Zimbabwe. Greener Journal of Agricultural Sciences, 5(1), 034–042. https://doi.org/10.15580/GJAS.2015.1.011515003
- Dzadze, P., Mensah, O. J., Aidoo, R., & Nurah, G. K. (2012). Factors determining access to formal credit in Ghana: A case study of smallholder farmers in the Abura-Asebu Kwamankese district of central region of Ghana. Journal of Development andAgricultural Economics, 4(14), 416–423. doi:10.5897/JDAE12.099 ISSN 2006- 9774 ©2012 Academic Journals
- Elias, S., Ahmad, I. M., & Patil, B. L. (2015). The determinants of access to agricultural credit for small and marginal farmers’ in Dharwad district, Karnataka, India. Research Journal of Agriculture and Forestry Sciense, 3(5), 1–5. Corpus ID:55470541
- Gbigbi, T. M. (2017). Are there road blocks to access micro-credit from selected microfinance banks in delta state, Nigeria? Implications for small scale farmers sustainability. Journal of Food Industry, 1(1), 1–16. https://doi.org/10.5296/jfi.v1i1.11613
- Getnet, A. (2014). Financial inclusion, regulation and inclusive growth in Ethiopi a. Working paper. NBE, Ethiopia.
- Gollin, D. (2014). Smallholder agriculture in Africa: An overview and implications for policy IIED Working Paper. IIED, London, UK.
- Greene, H. W. (2003). Econometric analysis (5th ed.). Pearson Education, Inc.
- Gujarati, D. (2009). Basic econometrics 5th edition Tata Mc-Graw hill Companies, Tokyo.
- Hoda, A., & Terway, P. (2015). Credit policy for agriculture in India: An evaluation. Indian Council for Research on International Economic Relations.
- International Financial Corp (IFC). (2014). Access to finance for smallholder farmers, learning from the experiences of microfinance institutions in Latin America, Washington, Dc, USA.
- Johann, M. (2012). Managing agricultural commercialization for inclusive growth in Sub- Saharan Africa. Global Development Network –Policy brief Paper-No. 1.
- Julien, H., Kossi, A., & Aklésso, E. (2021). Analysis of factors influencing access to credit for vegetable farmers in the Gulf Prefecture of Togo. American Journal of Industrial and Business Management, Volume 11, 392–415. https://doi.org/10.4236/ajibm.2021.115026
- Kiplimo, J. C., Ngenoh, E., Koech, W., & Bett, J. K. (2015). Determinants of access to credit financial services by smallholder farmers in Kenya. Journal of Development and Agricultural Economics, 7(9), 303–313: doi:10.5897//JDAE2014.0591
- Kopparthi, M. S., & Alice, K. (2016). Impact of value chain financing on smallholder farmers’ livelihoods in Rwanda case study: Rwanda rice value chain. International Journal of Information Research and Review, 03(12), 3331–3340.
- Masaood, M., & Keshav, L. (2020). Factors affecting farmers’ access to formal and informal credit: Evidence from rural Afghanistan. Journal of Sustainability, 12(1268), 1–16.
- Mebrate, W. (2015). Determinants of access to formal credit to small holder farmers: The case of Dale Woreda, Sidama Zone. In Proceeding of the 36th Annual Research Review Workshop. (No 4); PP22-37, May, 2015. Hawassa University, College of Business and Economics.
- Miller, C., Richter, S., McNellis, P., & Mhlanga, N. (2010). Agricultural investment funds for developing countries. Food and agriculture organization of the United Nations. Available:http://www.fao.org/fileadmin/user-upload/ags/publications/investment-funds.pdf. Accessed July, 2/2018
- Muhongayirea, W., Hitayezub, P., Mbatiac, O. L., & Wangiad, S. M. (2013). Determinants of farmers’ participation in formal credit markets in rural Rwanda. Journal of Agricultural Sciense, 4(2), 87–94. https://doi.org/10.1080/09766898.2013.11884706
- Musabanganji, E., Karangwa, A., & Philippe Lebailly, P. (2015). Determinants of access to agricultural credits for small Scale farmers in the southern province of Rwanda. International Scientific Agricultural Symposium “Agrosym 2015, 1815–1820.
- Njeru, T. N., Mano, Y., & Otsuka, K. (2016). Role of access to credit in rice production in Sub-Saharan Africa: The case of Mwea irrigation scheme in Kenya. Journal of African Economies, 25(2), 300–321. https://doi.org/10.1093/jae/ejv024
- Nouman, M., Siddiqi, M. F., Asim, S. M., & Hussain, Z. (2013). Impact of socio-economic characteristics of farmers on access to agricultural credit. Sarhad Journal Agriculture, 29(3), 469–475: link.gale.com/apps/doc/A352710134/AONE?u=anon~d2d689ff&sid=googleScholar&xid=19df68a2
- NSZADB(North Shewa Zone Agricultural Development Bureaue) (2017). North Shewa Zone agricultural development Bureaue, annual report.
- Nyaga, J., & Nzulwa, J. (2017). Determinants of procurement performance in state corporations in Kenya. The Strategic Journal of Business & Change Management, 4(2), 865–888. www.strategicjournals.com
- Ojiako, I. A., Idowu, A. O., & Ogbuk wa, B. C. (2014). Determinants of loan repayment behavior of smallholder cooperative farmers in Yewa North local government area of Ogun state, Nigeria: An application of Tobit model. Journal of Economics and Sustainable Development, 5(16), 144–153 Corpus ID: 73656113.
- Richard, B., & Ramzy, M. (2016). Study on access to formal financial credit services by smallholder rural farmers for agricultural investment: A struggling option to rural development in Uganda. Proceedings of ISER International Conference, International Journal of Management and Applied Science, 2 (11), 58–63, ISSN: 2394–7926.
- Rizwan, M., Qing, P., Saleem, I., Adnan, N., & Qing, W. (2019).Why and for what? An evidence of agriculture credit demand among rice farmers inPakistan.ADBI working Paper Series: Number 995. www.adbi.org
- Samson, A., & Obademi, O. (2018). The determinants and impact of access to agricultural credit on productivity by farmers in Nigeria; Evidence from Oyo state, Nigeria. Advances in Social Sciences Research Journal, 5(3), 252–265. https://doi.org/10.14738/assrj.53.3588
- Samuel, S. (2020). Determinants of access to formal credit in rural areas of Ethiopia: Case study of smallholder households in Boloso Bombbe district, Wolaita zone, Ethiopia. Journal of Economics, 9(2), 40–48. http://www.sciencepublishinggroup.com/j/eco
- Sattar, T. (2012). A Sociological analysis of constraining factors of development in agriculture sector of Pakistan. Journal of Economics and Sustainable Development, 3(8), 8–24. ISSN 2222-1700 (Paper) ISSN 2222-2855 (Online) 0r www.iiste.org
- Sebatta, C., Wamulume, M., & Mwansakilwa, C. (2014). Determinants of smallholder farmers’ access to agricultural finance in Zambia. Journal of Agricultural Science, 6(11), 63–73. doi:10.5539/jas.v6n11p63
- Singh, A. S., & Masuku, M. B. (2014). Sampling techniques & determination of sample size in applied statistics research an overview. International Journal of Economics, Commerce and Management, 2(11), 1–22. http://ijecm.co.uk/
- Snauders, M., Lewis, P., & Thorn Hill, A. (2009). Research methods for business students fifth ed. Pearson Education Limited.
- Zeller, M. (1998). Determinants of repayment performance in credit groups: The role of program design, Intergroup risk polling, and social Cohesion. International Food Policy Research Institute. Reprint No. 384.
Appendix A:
Conceptual Definition of few terms
-Access to formal credit: the availability of the chance or the opportunity for a smallholder farmer to borrow money from financial institutions.
-Formal credit: financial credit from financial institutions.
-Credit: money that individuals borrow from lenders to be paid back in a certain time interval.
-A household: a group of rural persons who live together under the responsibilities of the head and eat from the same pot.
-Kebele: the lowest administrative body in Ethiopia, which comprises a population of at least 5000 people.
-Smallholder farmers: farmers who plough a small area of land for their agricultural practice or farmers who do not own an intensive commercial farm.
Appendix B:
Result for Continuous variables
Appendix C:
Result for Dummy variables
. tab sex educat attrisk offincom ir lendpro grouplen rapidrp affc
-> Tabulation of sex
-> Tabulation of educat
Tabulation of attrisk
Appendix D:
Results for Diagnostic Tests
Multicollinearity by VIF
estat vif
Diagonal matrix/Pairwise Correlation Matrix/Contingency Coefficient
iii.Heteroskedasticity Tests
estat hettest
Breusch-Pagan/Cook-Weisberg test for heteroskedasticity Ho: Constant variance
Variables: fitted values of uffc
chi2(1)=41.68
Prob > chi2 =0.0000
Ramsey test
. estat ovtest, rhs
(note: exper^3 dropped because of collinearity)
Ramsey RESET test using powers of the independent variables; Ho: model has no omitted variables
F(20, 263) =1.04
Prob > F =0.4159