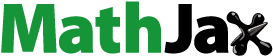
Abstract
This study examines the relationship between bank stock price and its selected fundamentals, namely, profitability, credit risk, and liquidity risk. Using the dynamic common correlated effect (DCCE) technique, we discover a mechanism error-correction between the stock price and the selected fundamentals. We estimate that the equilibrating process of stock price takes between 2.62 and 3.22 months. This study also provides significant support for hypotheses of the positive role of profitability (proxied by ROE and NIM) to bank stock price. Credit and liquidity risk measures do not significantly affect stock price.
1. Introduction
Banks are inherently hard to value (more opaque) than companies in other industries (Blau et al., Citation2017; Morgan, Citation2002). Existing empirical studies reveals the difficulty in assessing the value of banks by outsiders (Jones et al., Citation2012). This difficulty is arised from asymmetric information and moral hazard problem that is inherent in a bank business model (Greenbaum et al., Citation2019, page 8). These problems have caused substantial measurement error in the loan and scurities, which are the dominant part of banks asset (Freixas & Rochet, Citation2008 page 127).
Banks heavily apply risk management techniques to reduce the impact of asymmetric information and moral hazard to asset value (therefore, bank is often called a business of handling risk, Koch & MacDonald, Citation2014 page 22). Nevertheless, its efficacy is still fell short of expectation, which explains heavy handling of this industry by regulators (Barth et al., Citation2008, page 12). Due to previously explained narrative, market discipline (by investor) is also believed to be less effective for banks (Morgan & Stiroh, Citation2001).
There are several common profitability measures alternatives for banks like Earnings per Share (EPS), Return on Asset (RoA), Earnings Growth, and Return on Equity (ROE). In this study, we use ROE; as doccumented by Pennacchi and Santos (Citation2021), this measure is the most common form of profitability measurement, which is often used in setting management compensation contracts and investor communications.
As a business in handling risk, it is only natural to expect the response of its stock investors highly dependent not only on the level of profit achievement but more importantly on how is it achieved? Does the profitability bring hidden issues that could materially reduce or even subsequently reverse the achievement? The market power and pricing behavior of banks depend on the broader size of the bank’s margins beyond interest income, and because of the complexity of the bank’s business, any policy analysis of a bank’s market power must rely on different indicators and especially on indicators that reflect the full reality of the bank’s output mix and explicitly considers the prices of all outputs relative to their marginal costs (Valverde & Fernández, Citation2007). Investors face a high-dimensional forecasting problem; this are behavioral biases, and investors arguably face a high-dimensional prediction problem in the real world. Investor heterogeneity can result in the role of endogenous price-based signals from which investors can extract information about not only asset fundamentals but also the trading behavior of other investors and need to learn (Martin & Nagel, Citation2022). In this regard, it is standard practice by bank stock investors to view financial performance in conjunction with accompanying risks
Bank business is subject to various kinds of risks, but there are two most prominent risks that are relevant for Indonesia context, liquidity risk and credit risk (Saunders & Cornett, Citation2004 page 173).Footnote1 There are ample empirical studies that support this notion. Credit risk, liquidity risk, and bank capital were shown to affect bank profitability in either a positive or negative way. Banks need to change their credit policies, which aim to reduce credit risk, whereas good credit policies lead to reduced bad credit in banks and thus improved profitability. In addition, the banks should have more liquidity and higher capital in order to face any future situations that might have an effect on their profitability (Saleh & Abu Afifa, Citation2020). Credit risk and liquidity risk on determining banks’ cost of intermediation and their effects on bank margins are sensitive to economic fluctuations (Kesraoui et al., Citation2022). The impact of liquidity on profitability is positively correlated, while the credit risk negatively and significantly impacts the profitability (Abbas et al., Citation2019).
Information in a stock price is divided into three components, namely, past information, current information available to the public, and current private information. The extent and amount of this information reflected in stock price reflect market efficiency. Blau et al. (Citation2017) argued that since risk in the bank intermediation process is quite high, the information carried in its stock price is limited. Investors can only observe efforts of risk management, but not its efficacy, and hence, their decision is based on less then perfect information resulting in error. Investors learn from experience and therefore act more in accordance with the prescriptions of finance theory as long as outcomes are observable and salient. They view financial education, decision support, default options, salient disclosure, and private investments in financial literacy via investment mistakes as complements, not substitutes. Koestner et al. (Citation2017); Carlin and Robinson (Citation2012). Surely, investors learn from their mistakes and revise their decision accordingly.
Previous studies in the relationship of bank stock return and its determinants mostly cast in static design without accounting for feedback and learning effects. We contribute to the existing literature by a study conducted in a dynamic paradigm. Our dynamic empirical design allow us to not only learn about long-run relationship but also uncover the error-correction mechanism (ECM): a manifestation of feedback and learning process. To the best of our knowledge, we are the first to perform this kind of study.
To meet our research objective, we construct empirical design using listed bank stock price as a dependent variable and profitability, credit risk, and liquidity risk as our variables of interest and macroeconomic variables (interest rate, exchange rate change, and GDP growth) as control. We apply a novel econometric technique, namely, Dynamic Common Correlated Effect (DCCE) (Chudik & Pesaran, Citation2015), to a monthly long panel data set that comprises 21 banks the for period of July 2006 to August 2019. The flow of application of the econometric technique follows closely Ariefianto et al. (Citation2021).
Previous studies proposed that lack of transparency can reduce stock price stability and lead to a lower level of market efficiency (Blau et al., Citation2017; Haggard et al., Citation2008; Jin & Myers, Citation2006). Due to a lack of company-specific information, Veldkamp (Citation2006) created a model in which investors must rely on broad signals to estimate firm cash flows. Moreover, lack of information leads to greater heterogeneity among securities, and this results in uninformed share prices.
When two or more prices of security allow an investor to build a net investment portfolio that will produce a definite return, an arbitrage opportunity (risk-free) arises. These opportunities initiate a large trading volume that puts pressure on the security price. According to Bodie et al. (Citation2018; 326), this pressure will continue until the price reaches the level that prevents arbitrage.
Bank opacity may affect the ability of outsiders to precisely evaluate the bank, and hence, bank stock price information may also be inaccurate. According to Simoens and Vander Vennet (Citation2021), profitability was one of the primary drivers of bank M/B ratios from 2007 to 2017. This changed after the crises as improved cost efficiency was a major factor in increased profitability. Furthermore, an extended period of low interest rates in Europe could have a detrimental impact on M/B valuations and bank franchise values. This analysis suggests that higher deposit shares were related to lower performance in post-crisis Europe and could be attributed to the low-for-long monetary policy environment reducing the value of bank funding.
According to Yuksel and Zengin (Citation2017), the net interest margin is an estimate of the diference between the weighted average yield on interest income and expenses (Islam & Nishiyama, Citation2016). Bank profitability is usually measured by net interest margin because it is one of the useful indicators to describe the bank’s core income capability where net interest margin focuses on measuring profits from interest activities (Batten & Vo, Citation2019). Our hypothesis that higher profitability positively correlates with the stock price. For example, assuming that the net interest margin is not good will affect the net income and the bank’s stock price.
Hypothesis 1. Bank stock price positively correlates with profitability
The profitability variables adopted for the study were NIM and ROE. NIM measures the gap between what the bank pays savers and what the bank receives from borrowers. Thus, NIM focuses on the traditional borrowing and lending operations of the bank (Chen et al., Citation2018; Gadzo et al., Citation2014). NIM is also the difference between interest income generated (by banks) from loans and interest expense paid out to depositors, relative to average earning assets. It shows the profit a bank is making from its basic function, out of its average earning assets. Higher NIM convinces banks to give out more loans and hence increase its credit and operational risk management levels. Studies that have employed NIM as a profitability measure include Heffernan and Fu (Citation2008), Nguyen (Citation2012), Lee et al. (Citation2014), Noman et al. (Citation2015), and Gadzo et al. (Citation2019).
The bank would surely select ROE assuming that they want to create a rosy picture of its performance. The use of ROE in bank compensation contracts and investor communications is consistent with this decision (Pennacchi & Santos, Citation2021). Based on this, ROE is estimated to positively correlate with stock prices.
Hypothesis 2. Bank stock price negatively correlates with credit risk
Imbierowicz and Rauch (Citation2014) suggests that liquidity and credit risk jointly affect bank health in a sample of US commercial banks. According to the findings of Vazquez and Federico (Citation2015), simultaneous exposure to liquidity and credit risk increases bank troubles during the crisis based on a collection of European and American banks. Furthermore, liquidity and credit risks are believed to be regulated together (Acharya, Mehran and Thakor, Citation2016; Acharya & Mora, Citation2015; Brunnermeier et al., Citation2009; Calomiris et al., Citation2015; Distinguin et al., Citation2013; He & Xiong, Citation2012; Imbierowicz & Rauch, Citation2014; Vazquez & Federico, Citation2015). However, since banks are heavily reliant on the interbank market, increasing capital requirements might be seen as a prudential step to reduce liquidity and insolvency concerns.
Hypothesis 3. Bank stock price positively correlates with liquidity measures
According to Phung et al. (Citation2021), non-performing loans negatively correlate with bank efficiency. NPL can be seen as an indicator of a bank’s asset health. This is because the bulk of assets that might produce income to a bank are from loans. The liquidity risk of a bank increases mainly as the NPL ratio increases, and this reduces the value of productive assets and can decrease the value of the bank’s shares. Once the value of the productive assets decreases significantly higher, NPL can negatively correlate with the stock price.
Higher credit risk is negatively correlated with stock price, while liquidity risk positively is correlated with stock price.
2. Methodology and data
Based on hypothesis contruction explained in the previous section, we construct the relationship of stock price and the explanatory variables in the form of short-run (Error-Correction Model) and long-run regression as follows:
where SP is that stock price, Prof is the profitability measure, Cred is the Credit risk measure, Liq is the liquidity measure, and CV is a set of control variables (Size, Capital ratio/capi, Exchange Rate/EXCH, Interest Rate/INTR, and Riil Gross Domestic Product/GDPR). EquationEquation 1(1)
(1) is the short-run equation, and Equationequation 2
(2)
(2) is the long-run equation. EquationEquation 1
(1)
(1) and Equation2
(2)
(2) are connected by the error-correction term (ECT); it is also a lag one period long-run regression residual whose parameter
measures the pace of adjustment. This parameter should be negative with the absolute value strictly less than 1. A detailed description on variables, proxies, and their expected sign hypothesis (in relation to the dependent variable) is provided in .
Table 1. Variables, proxies, and expected sign. The research hypotheses are given as expected impact sign against the dependent variable from the correlation matrix, and we have also considered the real facts in the Bank and also used the previous study as a reference
Our data set comprises monthly series of 21 public banks from July 2006 to August 2019, 3,318 bank monthly observations. The data are collected from the Indonesia Financial Services Authority-OJK (for bank level data), Yahoo finance for the stock price, and Bank Indonesia for exchange rates and interest rates. Appropriate cleansing, interpolation, and winsorization (with cut of 1% and 99%) are applied in data preparation.
Since our data are the type of a long panel (the number of time series is greater than the cross-section), we should pay attention to several important feature: (a) non-stationary (unit root), (b) cointegration, (c) slope heterogeneity, and (d) cross-sectional dependence.
Ideally, our econometric method requires all variables to be included in the model to be non-stationary, i.e. possesses a unit root process. For (bank-level) panel variables, we employ Pesaran (Citation2007) cross-section Augmented Dickey Fuller-PESCADF. This method was developed to be resistant to cross-sectional dependence (Pesaran, Citation2015b). Unit root tests for time series variables were performed using the ADF approach (Dickey & Fuller, Citation1979).
Westerlund (Citation2007) developed a method for the cointegration test that takes into consideration the cross-sectional dependence. The rejection of the null hypothesis is interpreted as the presence of cointegration. Persyn and Westerlund (Citation2008) generalize the design to employ a variety of possible dynamic structures. Various cointegration test specifications were performed based on (a) the variable of dependent used (SP); (b) the lag amount used, leads, and LR windows; (c) use or disuse of the automated lag selection (method by Akaike’s information criteria; AIC); and (d) the use of the bootstrap approach to obtain robust inference (Blomquist & Westerlund, Citation2014).
Another issue with data from lengthy panels is the heterogeneity of the cross-section slope rather in addition to the residual component (Eberhardt & Teal, Citation2011). We test the homogeneity slope against heterogeneity assumption using the method developed by Pesaran and Yamagata (Citation2008) and Blomquist and Westerlund (Citation2013). The test detail covers (a) alternative long-run specifications (SP), (b) the use of standard errors of heteroscedasticity and consistent autocorrelation (HAC), and (c) inclusion of the cross-section mean of the descriptive variables and the lag amount (0–4).
Based on the slope heterogeneity test results, an appropriate version of estimator is selected. For the case of slope heterogeneity, we should use the mean group estimator (Pesaran & Smith, Citation1995). If the assumption of slope homogeneity is not rejected, then we could use set an identical slope for all cross-section units yielding a pooled mean group estimator reported by Pesaran et al. (Citation1999).
We conduct robustness check to support our empirical finding. The robustness check is performed by (a) running regressions on alternative proxies, (b) using samples based on bank types (state-owned banks: SOE; private-owned: PRIV). and (c) using subsamples based on the period: (i) up to April 2009 (714 observations) and (ii) May 2009—Aug 2019 (2,604 observations). April 2009 was selected as the cut-off period to reflect the condition before and after global financial crisis of 2008.
3. Results and discussion
3.1. Descriptive statistic and preliminary analyses
presents the descriptive statistics of the variables used in the study. We can see that the variables are quite well behaved as indicated by closeness of mean and median and no excessive skewness. Banks’ profit equals a company’s revenues minus expenses. Earning a profit is important to a business because profitability impacts whether a company can secure financing from a creditors, attract investors to fund its operations, and grow its business. Banks must have good governance in managing credit risk and liquidity risk so that banks can generate profits, which will certainly provide satisfaction to shareholders, investors, creditors, and all stakeholders. LAR is a liquid asset ratio, which provides an indication of the liquidity available to meet expected and unexpected demands for cash. The level of liquidity indicates the ability of the deposit-taking sector to withstand shocks to their balance sheet. LAR is calculated by using the core measure of liquid assets as the numerator and total assets as the denominator. LAR is a variable that deserves special attention since it has some degree of skewness. The SP average is 1.72, with a median of 0.60, and the LAR average is 28.38, with a median of 26.08.
Table 2. Descriptive statistics. This table reports descriptive statistics variables used in the study. The variables are in numeric (up to two digits behind the comma); SP in thousand Indonesian Rupiah (IDR), SIZE, EXCH, and GDPR in IDR; SIZE, EXCH, and GDPR in Logarithmic form; and NIM, ROE, NPL, LAW, LDR, ALR, CAPI, and INTR in percentage. Descriptive statistics presented are average, median, maximum, minimum, standard deviation, percentiles, and number of observation. The statistics are calculated over the whole sample data
shows the result of simple correlation (Pearson’s Correlation) between variables of interest in this study. The correlation data are represented in triangular form, and multicollinearity does not appear to be a possibility for explanatory variables. The correlation between variables of independence is significantly lower than 0.500. Simple correlation coefficients among SP are high with SIZE, at 0.52, and these statistics provide clues about the possibility of a large and significantly positive correlate with stock price measures. In summary, based on the analysis from the data set for subsequent analysis, the profile of data is not believed to have further negative implications.
Table 3. Correlation matrix. This table reports simple bivariate correlation statistics (Pearson) between variables used in the study. Correlation statistics are calculated over the whole sample data. Correlations are presented as a half-triangle matrix
summarizes the findings of the unit root check of the variables used in the study. Significant rejection of nonstationary null hypothesis is obtained for variables NIM, ROE, NPL, LAW, DLR, LAR, CAPI, and INTR. On the other hand, variables SP, SIZE, EXCH, and GDPR are significantly non-stationary.
Table 4. Unit root test. This table reports the summary of unit root tests performed on variables used in the study. The report covers (1) name of variables, (2) name of corresponding unit root test method used, and (3) conclusion of the test (reject or cannot reject the null hypothesis). The levels of significance used as a rule for null hypothesis rejection are 1%, 5%, and 10%, respectively
shows that null hypotheses of no cointegration are not rejected using subgroup and global criteria. Nevertheless we can see later, from ECM model estimates that the ECT is highly significant, indicating that there is an equilibrating mechanism, i.e. cointegration. We suspect that the apparent inalignment is caused by undetected multicollinearity or endogeneity among risk proxies used (credit risk and liquidity).
Table 5. Cointegration test. This table reports the results of the cointegration test based on Westerlund (Citation2007), Persyn and Westerlund (Citation2008), and Blomquist and Westerlund’s (Citation2013) methods. Cointegration is based on various specifications: with SP as the dependent variable. Null hypotheses are (1) global no cointegration (using Pt and Pa test statistics) and (2) subsets cointegration (using Gt and Ga) for all specifications
Finally, as shown in , the null hypotheses of slope homogeneity are soundly rejected. Therefore, the regressions should be estimated by the mean group type estimator. We do not report the exante cross-section dependence test as the result is similar with post-estimation procedure that strongly concludes the presence of cross-sectional dependence. The presence of cross-sectional dependence then called for the use of cross-section averaging of variables to improve the estimation of equation. With this result, we conclude our estimation technique as a Dynamic Common Correlated Error Estimator with Mean Group variant (DCCE-MG).
Table 6. Slope Heterogeneity Test. This table reports the slope heterogeneity test using the method proposed by Pesaran and Yamagata (Citation2008) and Blomquist and Westerlund (Citation2013). There are several specifications based on (1) whether SP is a dependent variable, (2) inclusion of cross-section average, and (3) heteroscedasticity treatment. Null hypotheses are slope homogeneity for all specifications
3.2. Regression result
The regressions provide sound support for the error-correction model. We find strong and significant evidence of an error-correction mechanism from the regressions. The MG estimate for ECT is −0.3094, which corresponds to a time lag of 3.2 months (=1/0.3094). The CD test yields significant statistics, indicating that cross-sectional dependence occurs and is significant.
In , ROE, LAW, DLR, and SIZE were positively correlated with SP, but ROE has signicance to SP in short-run equations and long-run equations, while DLR has significance in short-run equations only. Therefore, it seems that the DLR impact on SP is a more short-term reaction rather long-term adjustment.
Table 7. Baseliene regression result. This table reports estimations of the baseline model with a dependent variable. The table presents the estimated coefficients and standard error. The regression model corresponds to specific specifications inclusion of constant and application of cross-section average (DCCE class estimators). Statistical significance is denoted as * at the 10% level, ** at the 5% level, and *** at the 1% level
The findings show that macroeconomic (EXCH, INTR, and GDPR) is negatively correlated with SP and this result is in accordance with the literature from Huy et al. (Citation2020).
3.3. Robustness test
In , the outcome of ECT estimates might be considered qualitatively equal to regression for the robustness test using SP. The MG and DCCE criteria have an approximate range from −0.3103 to −0.3156, and these ECT estimations correlate with a time lag of 3.17 months (=1/0.3156) to 3.22 months (=1/0.3103). For all models, the CD test yields significant statistics, indicating that cross-sectional dependence occurs and is significant.
Table 8. Robustness test with other variables. This table reports estimations of the baseline model with the dependent variable. The table presents the estimated coefficients and standard error. Each regression model (denoted by a number in the first line of each column heading) corresponds to specific specifications inclusion of constant and application of cross-section average, by alternating proxies in the variables of interest (DCCE class estimators). Statistical significance is denoted as * at the 10% level, ** at the 5% level, and *** at the 1% level
According to the results, all independent variables (NIM, ROE, NPL, DLR, and ALR) except LAW in all models were positively correlated with SP and these results are the same as baseline regression. DCCE estimations in models 1 and 2 are preferred and as predicted ROE by the long-run equation in Models 1, 2, and 3 and the short-run equation in Models 1 and 2 was positively correlated and significant to SP. Control variables did not have a significant impact on SP.
In , the outcome of ECT estimates might be considered qualitatively equal to regression for the robustness test using SP and reveals that SOE banks are the most responsive in the event of a stock price shock. Using SP as a stock price gauge, the ECT estimates for SOE banks were −0.4694 or 2.13 months shock adjustment, and these are greater than PRIV banks, which were −0.3015 or 3.32 months shock adjustment. This may be due to SOE banks’ extraordinary reputation or credibility, which makes them purchase or release stock easily.
Table 9. Robustness test—Bank type. This table reports estimation of the robustness model: bank type regressions with the dependent variable using the DCCE technique with ECM format. The table presents the estimated coefficients and standard error. Each regression model (denoted by a number in the second line of each column heading) corresponds to specific specifications described by inclusion of constant and cross-section average. Estimations are applied to subsamples based on bank types (“SOE” and “PRIV”). Statistical significance is denoted as * at the 10% level, ** at the 5% level, and *** at the 1% level
According to the robustness test, the result of long-run and short-run equations, ROE in Model 5a, were positively correlated, significant to SP, and consistent with the baseline regression finding. The short-run equation of CAPI in Model 5b was negatively correlated with and highly significant to SP. This is probably because State-Owned Enterprises (SOEs) have a strong capital and different business model than National Private Bank (PRIV). Customer financing appears to be a focus of SOE banks’ activity and the signaling concept appears to work for both SOE and PRIV banks.
ROE and LAW have different algebraic signs, while our initial hypothetical sign of ROE is positive and LAW is negative, so they are still similar to our initial hypothesis. All control variables except CAPI were not significant to SP.
In , the sample in Model 6b (after April 2009) had lower ECT coefficients than the sample in Model 6a (up to April 2009). Therefore, this estimate is in line with the idea that after the 2008 economic crisis, there was a shock that fell stock prices after April 2009.
Table 10. Robustness test—Period. This table reports estimation of robustness check regression with the dependent variable using the DCCE technique with ECM format. The table presents the estimated coefficients and standard error. Each regression model (denoted by a number in the first line of each column heading) corresponds to specific specifications described by inclusion of constant and cross-section average. Estimations are applied to split samples (“Up to April 2009” and “after April 2009”). Statistical significance is denoted by * at the 10% level, ** at the 5% level, and *** at the 1% level
ROE by the long-run equation is positive and significant after April 2009, but lost relevance in the sample up to April 2009. The stock price signaling hypothesis appears to be valid just after April 2009. This finding is aligned with Simoens and Vennet, 2020 who concluded that one of the main drivers of bank M/B ratio from 2007 to 2017 was profitability, while cost efficiency improvement was a key driver of better profitability following the crisis.
LAW negatively correlated with SP is equal to the initial hypothetical sign, and according to the literature from Phung et al. (Citation2021), credit risk negatively correlate with bank efficiency and ultimately negatively correlate with stock prices, which is similar towith Abbas et al. (Citation2019), The impact of liquidity on profitability positively correlates, while the credit risk has a negative and statistically significant effect on bank profitability. All control variables were not significant.
In general, NPL and LAW negatively correlated with SP and are equal to the initial hypothetical sign. According to the literature from Phung et al. (Citation2021), non-performing loans negatively correlate with bank efficiency and ultimately negatively correlate with stock prices
4. Conclusion
Our paper has shown that profitability is the main driver of bank stock prices in Indonesia but have to be viewed with their risk features. Nevertheless, the conventional risk measures do not seem to have a significant effect. This result has important consequences for bank management, regulators, and investors, in which perhaps new more meaningful risk measures should be developed, tested, and imposed.
We have modelled the empirical relationship of bank stock price with its selected fundamental (profitability, credit risk, and liquidity risk) using DCCE in ECM format. The empirical design is then applied to a monthly panel data set composed of 21 banks from period July 2006 to August 2019 (3.318 bank month observations). Our empirical design is quite well supported. We found a highly significant coefficient of ECT between 0.31 and 0.38, corresponding to 2.62 to 3.22 months of equilibrating time. ECT estimates are proven to be robust under various checking schemes.
ROE has positive and statistically significant correlation with SP, and DLR has a positive and quite significant correlation with SP. Aligned with our initial hypothesis, profitability (NIM and ROE) and liquidity risk (DLR and ALR) consistent positively correlated with SP and these findings are consistent with the study by Pennacchi and Santos (Citation2021), “when the bank wants to create a rosy picture of its performance, ROE i selected.” This option is backed by the widespread use of ROE in bank compensation contracts and investor communications and consistent too with the study by Abbas et al. (Citation2019); the impact of liquidity on profitability positively correlates, while the credit risk negatively and significantly impacts the profitability. Results from the regression for credit risk, LAW, have different algrebraic signs but NPL consistently positively correlated with SP, and this finding is consistent with the study by Madugu et al. (Citation2020), credit risk positive (NPL) and significant effect on profitability. Robustness check performed by splitting samples: before crisis and after crisis provide support for the mentioned results.
Nevertheless, evidence of no cointegration found by an earlier stand-alone test should give us a note. There may be a undetected multicollinear structure in risk proxies that weakens the inference. This alignment could call for a revisit to panel cointegration test methodology since by theory, the results from ECT estimates and cointegration test should confirm each other. Therefore, future research should address this issue, finding credit risk and liquidity proxies that are orthogonal to each other while simultaneously being a correct measure.
Our results suggest that profitability is the main driver of stock prices in Indonesia but have to be viewed with risk implications. Nevertheless, the conventional risk measures do not seem to have a significant effect. This result has important consequences for bank management, regulators, and investors; perhaps new more impactfull risk measure should be developed, tested, and imposed .
Acknowledgements
We would like to thank several anonymous referees for inputs that have helped us to greatly improve the quality of this paper. All remaining errors are ours.
Disclosure statement
No potential conflict of interest was reported by the author(s).
Additional information
Funding
Notes on contributors

Hendrik Widjaja
Moch. Doddy Ariefianto Doddy is an Assistant Professor in Master Accounting, Binus Graduate Program, Binus University since August 2019. Before his tenure as a lecturer, he was a practitioner in the banking industry for more than 18 years holding various managerial positions. Doddy obtained Master of Science and Doctorate degree in Economics from Universitas Indonesia in 2007 and 2010, respectively. His research interest includes applied econometrics, banking, financial market, risk management, and financial technology. He has published his works in various reputable international journals.

Moch. Doddy Ariefianto
Hendrik Widjaja Hendrik is a General Manager of Finance in Sinarmas Group, since April 2015. Hendrik obtained a bachelor’s degree in computerized accounting from Bina Nusantara University in 1996, currently preparing a thesis to the graduate program in partial fulfilment of the requirements for Master Degree in Accounting Bina Nusantara University, Jakarta. Hendrik has had a career of more than 20 years in Big Four Accounting Firms, Barito Group, Parents Group, PSP Group, etc.
Notes
1. We also think Interest risk, arising from duration mismatch, as an important key risk, however, since we do not have access to the required data, hence, we refrain to include this risk in our study but acknowledge it as our study limitation.
References
- Abbas, F., Iqbal, S., Aziz, B., & Yang, Z. (2019). The impact of bank capital, bank liquidity and credit risk on profitability in postcrisis period: A comparative study of US and Asia. Cogent Economics & Finance, 7(1), 1605683. https://doi.org/10.1080/23322039.2019.1605683
- Acharya, V. V., Mehran, H., & Thakor, A. V. (2016). Caught between scylla and charybdis? Regulating bank leverage when there is rent seeking and risk shifting. The Review of Corporate Finance Studies, 5(1), 36–21 https://doi.org/10.1093/rcfs/cfv006.
- Acharya, V. V., & Mora, N. (2015). A crisis of banks as liquidity providers. The Journal of Finance, 70(1), 1–43. https://doi.org/10.1111/jofi.12182
- Ariefianto, M. D., Trinugroho, I., Lau, E., & Sergi, B. S. (2021). Banks’ liquidity management dynamics: Evidence from Indonesia. International Journal of Emerging Markets . https://doi.org/10.1108/IJOEM-06-2020-0715
- Barth, J. R., Caprio, G., & Levine, R. (2008). Rethinking bank regulation: Till angels govern. Cambridge University Press.
- Batten, J., & Vo, X. V. (2019). Determinants of bank profitability-Evidence from Vietnam. Emerging Markets Finance and Trade, 55(6), 1417–1428. https://doi.org/10.1080/1540496X.2018.1524326
- Blau, B. M., Brough, T. J., & Griffith, T. G. (2017). Bank opacity and the efficiency of stock prices. Journal of Banking & Finance, 76, 32–47. https://doi.org/10.1016/j.jbankfin.2016.11.026
- Blomquist, J., & Westerlund, J. (2013). Testing slope homogeneity in large panels with serial correlation. Economics Letters, 121(3), 374–378. https://doi.org/10.1016/j.econlet.2013.09.012
- Blomquist, J., & Westerlund, J. (2014). A non-stationary panel data investigation of the unemployment–crime relationship. Social Science Research, 44, 114–125. https://doi.org/10.1016/j.ssresearch.2013.11.007
- Bodie, Z., Kane, A., & Marcus, A. J. (2018). Investments . 2 Penn Plaza. 10121.
- Brunnermeier, M., Crocket, A., Goodhart, C., Persaud, A., Shin, H., Brunnermeier, M., Crockett, A., Goodhart, C. A., Persaud, A., & Shin, H. S. (2009). The fundamental principles of financial regulation (Vol. 11). ICMB, Internat. Center for Monetary and Banking Studies.
- Calomiris, C. W., Heider, F., & Hoerova, M. (2015). A theory of bank liquidity requirements. Columbia Business School Research Paper, (14–39). https://dx.doi.org/10.2139/ssrn.2477101
- Carlin, B. I., & Robinson, D. T. (2012). What does financial literacy training teach us? The Journal of Economic Education, 43(3), 235–247. https://doi.org/10.1080/00220485.2012.686385
- Chen, Y. K., Shen, C. H., Kao, L., & Yeh, C. Y. (2018). Bank liquidity risk and performance. Review of Pacific Basin Financial Markets and Policies, 21(1), 1850007. https://doi.org/10.1142/S0219091518500078
- Chudik, A., & Pesaran, M. H. (2015). Common correlated effects estimation of heterogeneous dynamic panel data models with weakly exogenous regressors. Journal of Econometrics, 188(2), 393–420. https://doi.org/10.1016/j.jeconom.2015.03.007
- Dickey, D. A., & Fuller, W. A. (1979). Distribution of the estimators for autoregressive time series with a unit root. Journal of the American Statistical Association, 74(366a), 427–431 https://doi.org/10.1080/01621459.1979.10482531.
- Distinguin, I., Roulet, C., & Tarazi, A. (2013). Bank regulatory capital and liquidity: Evidence from US and European publicly traded banks. Journal of Banking & Finance, 37(9), 3295–3317. https://doi.org/10.1016/j.jbankfin.2013.04.027
- Eberhardt, M., & Teal, F. (2011). Econometrics for grumblers: A new look at the literature on cross‐country growth empirics. Journal of Economic Surveys, 25(1), 109–155. https://doi.org/10.1111/j.1467-6419.2010.00624.x
- Freixas, X., & Rochet, J. C. (2008). Microeconomics of banking. MIT press.
- Gadzo, S. G., Gatsi, J. G., & Akoto, R. K. (2014). Post-merger analysis of the financial performance of SG SSB. International Journal of Financial Economics, 3(2), 80–91 http://www.rassweb.com.
- Gadzo, S. G., Kportorgbi, H. K., & Gatsi, J. G. (2019). Credit risk and operational risk on financial performance of universal banks in Ghana: A partial least squared structural equation model (PLS SEM) approach. Cogent Economics & Finance, 7(1), 1589406. https://doi.org/10.1080/23322039.2019.1589406
- Greenbaum, S. I., Thakor, A. V., & Boot, A. (2019). Contemporary financial intermediation. Academic Press.
- Haggard, K. S., Martin, X., & Pereira, R. (2008). Does voluntary disclosure improve stock price informativeness? Financial Management, 37(4), 747–768. https://doi.org/10.1111/j.1755-053X.2008.00033.x
- He, Z., & Xiong, W. (2012). Rollover risk and credit risk. The Journal of Finance, 67(2), 391–430. https://doi.org/10.1111/j.1540-6261.2012.01721.x
- Heffernan, S., & Fu, M. (2008 https://ssrn.com/abstract=1247713). The determinants of bank performance in China. Available at SSRN 1247713.
- Huy, D. T. N., Dat, P. M., & Anh, P. T. (2020). Building an econometric model of selected factors’impact on stock price: A case study. Journal of Security & Sustainability Issues, 9(M 77–93). https://doi.org/10.9770/jssi.2020.9.M(7)
- Imbierowicz, B., & Rauch, C. (2014). The relationship between liquidity risk and credit risk in banks. Journal of Banking & Finance, 40, 242–256. https://doi.org/10.1016/j.jbankfin.2013.11.030
- Islam, M. S., & Nishiyama, S. I. (2016). The determinants of bank net interest margins: A panel evidence from South Asian countries. Research in International Business and Finance, 37, 501–514. https://doi.org/10.1016/j.ribaf.2016.01.024
- Jin, L., & Myers, S. C. (2006). R2 around the world: New theory and new tests. Journal of Financial Economics, 79(2), 257–292. https://doi.org/10.1016/j.jfineco.2004.11.003
- Jones, J. S., Lee, W. Y., & Yeager, T. J. (2012). Opaque banks, price discovery, and financial instability. Journal of Financial Intermediation, 21(3), 383–408. https://doi.org/10.1016/j.jfi.2012.01.004
- Kesraoui, A., Lachaab, M., & Omri, A. (2022). The impact of credit risk and liquidity risk on bank margins during economic fluctuations: Evidence from MENA countries with a dual banking system. Applied Economics 54 35 , 4113–4130 https://doi.org/10.1080/00036846.2021.2022090.
- Koch, T. W., & MacDonald, S. S. (2014). Bank management. Cengage Learning.
- Koestner, M., Loos, B., Meyer, S., & Hackethal, A. (2017). Do individual investors learn from their mistakes? Journal of Business Economics, 87(5), 669–703. https://doi.org/10.1007/s11573-017-0855-7
- Lee, C. C., Hsieh, M. F., & Yang, S. J. (2014). The relationship between revenue diversification and bank performance: Do financial structures and financial reforms matter? Japan and the World Economy, 29, 18–35. https://doi.org/10.1016/j.japwor.2013.11.002
- Madugu, A. H., Ibrahim, M., & Amoah, J. O. (2020). Differential effects of credit risk and capital adequacy ratio on profitability of the domestic banking sector in Ghana. Transnational Corporations Review, 12(1), 37–52. https://doi.org/10.1080/19186444.2019.1704582
- Martin, I. W., & Nagel, S. (2022). Market efficiency in the age of big data. Journal of Financial Economics, 145(1):154–77 https://doi.org/10.1016/j.jfineco.2021.10.006.
- Morgan, D. P. (2002). Rating banks: Risk and uncertainty in an opaque industry. American Economic Review, 92(4), 874–888. https://doi.org/10.1257/00028280260344506
- Morgan, D. P., & Stiroh, K. J. (2001). Market discipline of banks: The asset test. Journal of Financial Services Research, 20(2), 195–208. https://doi.org/10.1023/A:1012464108248
- Nguyen, J. (2012). The relationship between net interest margin and noninterest income using a system estimation approach. Journal of Banking & Finance, 36(9), 2429–2437. https://doi.org/10.1016/j.jbankfin.2012.04.017
- Noman, A. H. M., Pervin, S., Chowdhury, M. M., & Banna, H. (2015). The effect of credit risk on the banking profitability: A case on Bangladesh. Global Journal of Management and Business Research 15 3 41–48 https://d1wqtxts1xzle7.cloudfront.net/40884692/5-The-Effect-of-Credit-Risk-with-cover-page-v2.pdf?Expires=1660120983&Signature=Eh3fShnONF09fSOzplIbr5ihfruU7FfiFYlT80Kjzr8EMpFHxr6H1cZFOTrhuGr5PMZ03Z~bHVjDeRag8z4m3W1Ayu13hUZozH2ARH2LJaK1C8zacj2lmD7o21qmhNJ1cUzYt9YqpJ24739J7CwohklA3rGWFe0glSbaffTiRQJKfVr41vSyUgOufNLZG8CXkRZ60FxuxV1wXlln-mSznymituQzXCPXGqQtANCUu-TsayqCrfzCMFJXDgJbaZAKIxmClzthln0Jww7YGirG4f-B5SuH7KFaypBrbnAPKGMvpm~aKEqbwhZW1wbd4lpJtcqkSDKUVKjSHDE2H8cnng__&Key-Pair-Id=APKAJLOHF5GGSLRBV4ZA .
- Pennacchi, G. G., & Santos, J. A. (2021). Why do banks target ROE? Journal of Financial Stability, 54, 100856. https://doi.org/10.1016/j.jfs.2021.100856
- Persyn, D., & Westerlund, J. (2008). Error-correction–based cointegration tests for panel data. The Stata Journal: Promoting Communications on Statistics and Stata, 8(2), 232–241. https://doi.org/10.1177/1536867X0800800205
- Pesaran, M. H. (2007). A simple panel unit root test in the presence of cross‐section dependence. Journal of Applied Econometrics, 22(2), 265–312. https://doi.org/10.1002/jae.951
- Pesaran, M. H. (2015). Time series and panel data econometrics. Oxford University Press.
- Pesaran, M. H., Shin, Y., & Smith, R. P. (1999). Pooled mean group estimation of dynamic heterogeneous panels. Journal of the American Statistical Association, 94(446), 621–634. https://doi.org/10.1080/01621459.1999.10474156
- Pesaran, M. H., & Smith, R. (1995). Estimating long-run relationships from dynamic heterogeneous panels. Journal of Econometrics, 68(1), 79–113. https://doi.org/10.1016/0304-4076(94)01644-F
- Pesaran, M. H., & Yamagata, T. (2008). Testing slope homogeneity in large panels. Journal of Econometrics, 142(1), 50–93. https://doi.org/10.1016/j.jeconom.2007.05.010
- Phung, Q. T., Van Vu, H., & Tran, H. P. (2021). Do non-performing loans impact bank efficiency? Finance Research Letters, 46 102393 Part B https://doi.org/10.1016/j.frl.2021.102393.
- Saleh, I., & Abu Afifa, M. (2020). The effect of credit risk, liquidity risk and bank capital on bank profitability: Evidence from an emerging market. Cogent Economics & Finance, 8(1), 1814509. https://doi.org/10.1080/23322039.2020.1814509
- Saunders, A., & Cornett, M. M. (2004). Financial markets and institutions: A modern perspective. McGraw-Hill Companies.
- Simoens, M., & Vander Vennet, R. (2021). Bank performance in Europe and the US: A divergence in market-to-book ratios. Finance Research Letters, 40, 101672. https://doi.org/10.1016/j.frl.2020.101672
- Valverde, S. C., & Fernández, F. R. (2007). The determinants of bank margins in European banking. Journal of Banking & Finance, 31(7), 2043–2063. https://doi.org/10.1016/j.jbankfin.2006.06.017
- Vazquez, F., & Federico, P. (2015). Bank funding structures and risk: Evidence from the global financial crisis. Journal of Banking & Finance, 61, 1–14. https://doi.org/10.1016/j.jbankfin.2015.08.023
- Veldkamp, L. L. (2006). Information markets and the comovement of asset prices. The Review of Economic Studies, 73(3), 823–845. https://doi.org/10.1111/j.1467-937X.2006.00397.x
- Westerlund, J. (2007). Testing for error correction in panel data. Oxford Bulletin of Economics and Statistics, 69(6), 709–748. https://doi.org/10.1111/j.1468-0084.2007.00477.x
- Yuksel, S., & Zengin, S. (2017). Influencing factors of net interest margin in Turkish banking sector. International Journal of Economics and Financial Issues, 7(1), 178–191.