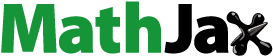
Abstract
Hedging being a predominant financial concern, is considered as a robust method of managing investment risks. Literature evinces that the covered call strategy provides nominal returns alongside effective hedging. However, studies have not compared the hedging effectiveness of covered call, covered put, collar, and synthetic long call strategies in the equity segment. The study evaluates the hedging effectiveness of option trading strategies by applying them to the companies of the top six National Stock Exchange (NSE) sector indices for twelve years, from 2009 to 2020 under volatile and neutral market conditions. The study collects data of 18,720 option contracts and considers 3744 observations for each strategy. The panel regression analysis approach is being incorporated into the study. Hausman test results determine the appropriate model between fixed and random effects. Furthermore, the study compares the mean scores of selected strategies to identify significant differences between the groups using the Games-Howell Post Hoc Tukey Test. The study recommended covered call and covered put strategies effectively hedge the investment in both market conditions. The study’s findings help retail investors choose a better hedging strategy and employ the same in their trading, specific to the market condition. Future studies can apply and compare the effectiveness of these strategies in other emerging derivative markets.
1. Introduction
Investors always seek strategies that hedge the equity risk (Antonakakis et al., Citation2020) and minimize the uncertainties in their investment (Fontanills, Citation2005; Samuel, Citation2017 and Kedžo & Šego, Citation2021). Derivatives are one of the financial instruments used for risk management (Dewobroto et al., Citation2010). Though the fundamental objective of derivatives is hedging, it has also been used for speculation. Forwards, futures, options, and swaps are derivative instruments; however, options have gained prominence due to their unique features and applicability in managing the risk of any underlying financial asset (Dewobroto et al., Citation2010and Kedžo & Šego, Citation2021). These options provide the right to buy or sell but not the obligation to buy or sell any specified financial asset at a specified price and time. Options trading allows to hedge the underlying asset and minimize the risks in the investment; it has attained huge momentum in the Indian capital market in recent years in terms of the number of contracts traded and premium turnoverFootnote1 (, ). However, it is noted that individual investors incur huge losses on equity investment due to market volatility (Kumar Meher et al., Citation2021). Therefore, option strategies allow investors to employ suitable hedging strategies to manage the equity price risk.
Table 1. Total number contracts traded and total premium turnover of index and stock options on national stock exchange from 2012 to 2022
Available studies on options hedging strategies focused on the performance of covered call or buy-write strategy (Diaz & Kwon, Citation2016; Hoffmann & Fischer, Citation2012; Mugwagwa et al., Citation2012 and Niblock & Sinnewe, Citation2018) and observed superior risk-adjusted returns. Limited attention is directed to other hedging strategies, such as collar strategy (Bartonova, Citation2012 and Israelov & Klein, Citation2016) and synthetic long call strategy (Figlewski et al., Citation1993; Dewobroto et al., Citation2010; and Mohil et al., Citation2020). Although studies have examined the performance of options strategies, such as covered call, straddle, and strangle strategies, the comparative study on options hedging strategies on covered call, covered put, collar, and the synthetic long call is not investigated much (Dewobroto et al., Citation2010 and Kedžo & Šego, Citation2021). Additionally, limited studies have compared the options strategies in the equity segment. Hence, evaluating and comparing the effective options hedging strategies is indispensable as it helps the investors in trading and hedging the investment.
Further in the literature, studies have explored the performance of options strategies in providing risk-adjusted returns. Studies by Chen & Leung (Citation2003), Guo et al. (Citation2020), and Fullwood et al. (Citation2021) found positive risk-adjusted returns using straddle strategies. However, limited studies have analyzed the performance of options strategies from the Indian perspective. Bangur (Citation2020) Bhat (Citation2021) and S.P. et al. (Citation2022) studied straddle and strangle strategies and observed that short straddle and short strangle strategies provide significant positive returns. Nevertheless, these strategies are applied to take advantage of market volatility and are considered as volatility strategies.
Prior studies express that a covered call strategy can be applied as a hedging strategy (Mugwagwa et al., Citation2012 and Niblock & Sinnewe, Citation2018; however, it is also noted that strategies like the collar and synthetic long call can hedge the equity price risk. The literature evinces that the covered call strategy is a prominent hedging strategy that provides nominal returns to retail investors. Hence, this opens the opportunity to analyze further the effectiveness/validity of the other option hedging strategies like the covered put, collar, and synthetic long call. Additionally, researchers have prescribed further enquiry to examine the efficacy of hedged investment in the equity market using hedging strategies against the equity price risk. Therefore, the study’s primary objective is to evaluate the influence of equity payoff and options premiums on options strategy’s payoff and, thereby, measure the hedging effectiveness under volatile and neutral market conditions. Further, the study compares the hedging effectiveness of options strategies. In order to evaluate and compare the hedging effectiveness of selected options strategies, the study considered the data from companies of the top six National Stock Exchange (NSE) sectoral indices for a period of 12 years from 2009 to 2020 and examined a total of 18,720 option contracts. The study follows a two-stage method where in the first stage, the study evaluates the influence of equity payoffs and options premiums on the options strategy’s payoff and measures the hedging effectiveness by applying the panel regression approach (Bhaduri & Sethu Durai, Citation2008). In the second stage, the study compares the mean scores of selected strategies to identify significant differences in the mean returns between the strategy (Goltz & Lai, Citation2009 and Dewobroto et al., Citation2010). Thus, the study justifies the appropriate hedging strategy for the investors.
The present study contributes to the literature in the following ways. Primarily, this study attempts to evaluate the hedging effectiveness of options hedging strategies using Indian equity options data and measure the performance of options strategies in the equity segment. Further, the study compares the hedging effectiveness of options strategies against the equity price risk by applying the panel regression approach (Bhaduri & Sethu Durai, Citation2008; Bonga-Bonga & Umoetok, Citation2016; Addae-Dapaah & Abdullah, Citation2020; Abou Elseoud et al., Citation2020and Badi Baltagi, Citation2021). This approach identifies the options strategy that hedges equity investment effectively. Thus, the investor can make informed decisions during their investment. Secondarily, the study compares the option strategy’s returns to identify the significant difference in mean scores between the strategies. A non-parametric tool, i.e., the Games-Howell Host Hoc Tukey Test, is applied to compare the mean returns of strategies. This approach helps in identifying the significant mean differences between strategies payoff.
2. Literature review
The literature review section mainly focuses on various option strategies and hedging methods.
2.1. Option strategies
There are studies on option trading strategies, where most of the studies concentrate on covered call strategy. This strategy holds a long position in the equity stock and protects against changes in the equity price of a stock by writing a slightly Out of The Money (OTM) call option (Mugwagwa et al., Citation2012 and Israelov & Nielsen, Citation2015). Theoretically, a covered call strategy should reduce both expected return and risk. Studies by Board et al. (Citation2000), Leggio & Lien (Citation2002), Whaley (Citation2002), Israelov & Nielsen (Citation2015), O’Connell & O’Grady (Citation2014), and Diaz & Kwon (Citation2016) opined that covered call strategy attracts investors by enhancing realized returns not much lower to index returns with minimal risk. However, these studies focused on applying a covered call strategy to equity index options. Mugwagwa et al. (Citation2012) and Niblock and Sinnewe (Citation2018) studied the performance of the covered call strategy using performance measures and observed that the strategy yields nominal returns at lower risk in the Australian equity market. Further, Israelov & Nielsen (Citation2015) opined that covered call strategy contributes to hedging effectiveness up to 64% of the market beta. Moreover, a covered put strategy is applied when the investors seek moderately bearish market by selling an OTM put option along with the short position in the equity (Kang et al., Citation2021). This strategy generates a maximum payoff of profit on the sale of equity plus the put option premium received for the put sold.
On the other hand, buying an ATM (At-The-Money) put option to the covered call strategy leads to constructing a collar strategy. Thus, this strategy holds a long position in the underlying security and put option, where buying a put option acts as insurance against the downside risk in the underlying asset’s price (El-Hassan et al., Citation2018). At the same time, the cost of the put option premium is mitigated by the premium received for the call option sold, thereby mitigating the cost of construction of the strategy. Bartonova (Citation2012) and Israelov & Klein (Citation2016) opined that selling a call option mitigates the cost of buying a put option in a collar strategy and covers 65% of loss risk to exhibit the best return-risk ratios. Further, Bartonova (Citation2012) highlighted the impact of employing a zero-cost collar strategy as hedging against a drop in the exchange rate. However, a study by El-Hassan et al. (Citation2018) debated Bartonova’s (Citation2012) findings and observed a negative effect on hedging using collar strategy as the put option was not exercised in any situations.
A synthetic long call strategy is an alternative hedging strategy adopted to protect the unexpected price drops in the underlying asset (Figlewski et al., Citation1993; Dewobroto et al., Citation2010; and Kedžo & Šego, Citation2021). This strategy is also known as the protective put strategy, where an investor buys a security in the open market, and to mitigate the loss of downside price risk, an investor buys an OTM put option (Mohil et al., Citation2020). Dewobroto et al. (Citation2010) compared stock hedging strategies for five years and declared that the covered call strategy is the best among other stock hedging strategies. Furthermore, Kedžo & Šego (Citation2021) analyzed the efficiency of hedging strategies such as covered call, collar, and other trading strategies by applying the stochastic dominance method. The study results depicted that unhedged portfolios never dominated portfolios with hedging strategies.
Studies are available on the performance of straddle, strangle, and butterfly options strategies. Studies by Chen & Leung (Citation2003), Guo et al. (Citation2020), and Fullwood et al. (Citation2021) analyzed the performance of straddle strategies and found significant positive returns. From the Indian perspective, Bangur (Citation2020) developed and compared the performance of the new trading strategy with the short straddle strategy. The study found that the new trading strategy’s performance is superior to the straddle strategy’s profitability and success rate. While a study by Bhat (Citation2021) has opined that short straddle and short strangle strategies provide significant positive returns. Basson et al. (Citation2018) suggested adopting butterfly strategies to earn limited profit with limited risk. Though there are studies on the performance of straddle, strangle and butterfly strategies, they are considered volatility strategies and applied to take advantage of the market volatility. There are studies on options pricing and performance of options hedging strategies; however, limited studies have compared the hedging effectiveness of covered call, covered put, collar, and synthetic long call strategies (Kedžo & Šego, Citation2021). Hence, this study attempts to compare the hedging effectiveness of selected strategies using an appropriate hedging tool in the equity segment and measure the hedge ratio.
2.2. Measuring hedging effectiveness
Different studies have employed diverse methods for analyzing hedging effectiveness. For example, EDERINGTON (Citation1979) estimated the hedging effectiveness of treasury bill futures contracts using the Ordinary Least Squares (OLS) method and concluded that the OLS method generates nominal portfolio variance. Further, FRANCKLE’s (Citation1980) study highlighted the EDERINGTON’s (Citation1979) findings by opining that the hedge ratio calculated using OLS improves the hedging effectiveness. Moreover, Bhaduri & Sethu Durai (Citation2008) study analyzed the effectiveness of stock futures index hedging and found 93.39% hedging effectiveness on spot index prices. Further, the study opined that the OLS method provides better hedging results at a shorter time horizon. Bonga-Bonga & Umoetok’s (Citation2016) study results concurred with Bhaduri & Sethu Durai (Citation2008) study findings, where their study determined that the least-squares approach provides effective results in a shorter time horizon. However, the study found 95.84% hedging effectiveness using index futures. Additionally, the study added the reason behind the effectiveness of the least-squares method as it attributed to the hedge ratio estimation using the slope of the independent variables, which is interpreted as the hedge ratio.
Lien (Citation2008), in his study, opined that determining hedge ratio using a regression model performs better, but researchers explore alternative methods to identify improved hedging effectiveness. Further adding to the prior study findings, G. Singh (Citation2017) suggests that hedge ratio calculated using OLS provides better results than hedging ratios estimated using Vector Error Correction Model (VECM), Vector Autoregressive (VAR), Generalized Autoregressive Conditional Heteroskedasticity (GARCH), and Exponential GARCH. Luo & Wang (Citation2018) studied currency derivatives to hedge currency risk using the least-squares method.
Bhatia et al. (Citation2020) examined the hedging effectiveness of metals and compared their relationship with the stock market by employing Dynamic Conditional Correlation—GARCH. Addae-Dapaah & Abdullah (Citation2020) studied the hedging effectiveness of exchange-traded funds using VECM and OLS. However, the study opined that the linear regression model is a relatively robust and simple technique. Major literature overviewed that the regression method is better for measuring hedging effectiveness. The primary purpose of this study is to determine the influence of equity payoffs on options strategy payoffs and measure the hedging effectiveness of selected options strategies. Further, the study compares the mean returns of these strategies to identify the significant differences in the mean returns and tests the below-mentioned hypotheses.
H1: There is a difference in hedging effectiveness of a covered call, covered put, collar, and synthetic long call strategies under volatile and neutral market conditions
H2: There is a difference between mean payoffs of a covered call, covered put, collar, and synthetic long call strategies
With the above hypotheses, the study attempted to present the panel data considered for the analysis and present the empirical analysis on hedging effectiveness and comparison of mean returns of selected strategies.
The methods section describes the data considered for the study and methods of calculating the payoffs of different strategies. Further, this paper reports the empirical analysis on the comparison of hedging performances and mean comparison of selected strategies and presents the evidence. The final section concludes the research article.
3. Research design
3.1. Data
This study considers monthly stock options data of companies that are part of the top six sectoral indices of the National Stock Exchange (NSE)Footnote2 available for trading in equity derivatives from 2009 to 2020. The study collects 18,720 option contracts for applying four option strategies. The required data is downloaded from the NSE repository. This study considers a one-month stock options contract and assumes that contracts are bought on the beginning day and held until expiry. The selected option strategies are constructed for twelve years. This study classifies the market conditions as volatile market conditions and neutral market conditions based on volatility levels is determined by considering the monthly volatility spread between S&P Nifty 50 implied volatility (IV) and realized volatility (RV) indexed over the total sample period (Niblock & Sinnewe, Citation2018).
If VSt > 0.036, it is deemed to be high volatility or volatile market condition, and If VSt < 0.036 is considered low volatility or neutral market condition (Niblock & Sinnewe, Citation2018).
3.2. Methodology
3.2.1. The method of payoffs calculation
The present study begins with calculating payoffs from selected option strategies and equities. The payoffs are calculated using the equations mentioned below:
The equity payoff is calculated as,
If the stock price at expiration is more than the OTM call strike price, the payoff from the covered call strategy is calculated as,
If the stock price at expiration is less than or equals the OTM call strike price, then the payoff from the covered call strategy is estimated as the OTM call premium.
where, is Price at the end of the holding period,
is the purchase price,
is the closing value of underlying security,
is the value of underlying security purchased,
is OTM call strike price and
is OTM call premium.
If the stock price at expiration is less than the opening stock price and is less than OTM put strike price, then the payoff from the covered put strategy is assessed as,
If the stock price at expiration is more than the opening stock price and is more than OTM put strike price, then the payoff from the covered put strategy is assessed as OTM put premium.
where is OTM put strike price and
is OTM put premium.
If the stock price at expiration is more than ATM put strike price and more than the OTM call strike price, the collar strategy payoff is calculated as,
If the stock price at expiration is more than ATM put strike price and less than the OTM call strike price, the collar strategy payoff is calculated as,
If the stock price at expiration is less than the ATM put strike price and less than the OTM call strike price, the collar strategy payoff is calculated as,
where, is the ATM put strike price and
is the ATM put option premium.
If the stock price at expiration is more than OTM put strike price and more than , the synthetic long call strategy payoff is calculated as,
If the stock price at expiration is less than OTM put strike price and less than , the synthetic long call strategy payoff is calculated as,
If the stock price at expiration is more than the OTM put strike price and less than , the synthetic long call strategy payoff is calculated as,
.
where, is the OTM put strike price and
is the OTM put option premium.
3.3. The panel regression method
The study’s primary objective is to investigate the hedging effectiveness of option trading strategies. The study considers twenty-six companies (cross-sectional) for twelve years (Time series). Badi Baltagi (Citation2021) opined that the cross-sectional observations are bound to be heterogeneous over time. However, the panel regression approach considers unobserved heterogeneity between the cross-section and provides better results with more information than the OLS approach. Hence, the study applies the panel regression method for measuring hedging effectiveness with three approaches. The Pooled Ordinary Least Squares (POLS) model is applied initially to measure the influence of independent variables on the dependent variable. Further, the Breusch - Pagan LM Test (Citation1980) identifies heteroskedasticity in the POLS model. If heteroskedasticity is identified in the POLS model, Fixed and Random Effect Models (FEM & REM) are applied, and the appropriate model is determined between FEM and REM using the Hausman test.
The panel regression analysis examines the influence of independent variables on the dependent variable. In this study, the payoffs of a covered call, covered put, collar, and synthetic long call strategies are considered dependent variables, whereas the payoff of equity stocks, option premiums, and market conditions are considered independent variables. The option premiums considered for the study are calculated by the NSE using the Black-Scholes model.Footnote3 This model considers other variables such as underlying asset price, option strike price, risk-free interest rate, time to expiration, dividends, and volatility for calculating option premium; hence, all these variables are integrated with options premium (Black & Scholes, Citation1973). However, brokerage or commission fee does not affect the options premium calculation. Besides, the objective of this study is to measure the influence of equity payoff and options premiums on a strategy’s payoff. Therefore, the study does not consider other factors separately. The study introduces two dummy variables for volatile and neutral market conditions and measures the effectiveness of selected option strategies in hedging the equity position.
The mathematical equation for the panel regression model can be given as follows (Rao et al., Citation2021):
where Cst is options strategy’s payoff and uit is error term.
3.4. Unit root tests
Econometric panel data analysis assumes the stationarity of the data. The Augmented Dickey-Fuller test (ADF) proposed by Dickey & Fuller (Citation1979, Citation1981) and the Phillips-Perron unit root test (PP unit root test) (Citation1988) that has been widely accepted to test the unit root or stationarity of the data. The stationarity of the data implies that statistical properties like means and variances should be consistent over the period. The rationality behind the stationarity of the data is that statistical properties will remain unchanged in the future.
If the data is stationary over the period, it is implied that statistical properties will remain unchanged in the future. The mathematical expression of ADF is as follows:
The mathematical expression of the Phillips-Perron unit root test (PP unit root test) is as follows:
Where yt = denotes the time series to be tested, a = constant, β = coefficient on a time trend, p = lag order of the autoregressive process, εt = error term.
4. Data analysis and interpretations
In this section, the study has reported the results of descriptive statistics, panel regression, Kruskal-Wallis Test, and Games-Howell Post Hoc Tukey Tests. The descriptive statistics of selected option strategies and the options premiums are presented in . The highest and lowest strategy payoff observed during the study period is ₹2012.20 and ₹-513.70 for the collar strategy. The covered put strategy showed the highest average return of ₹37.53 with a deviation of ₹41.73, and the collar strategy showed the lowest average payoff of ₹28.68 with a variation of ₹90.62. These results indicated that the covered put strategy generated a superior mean payoff at a lower risk than the equity payoff, whereas the collar strategy observed a lower mean payoff at comparatively higher risk than other strategies. The Shapiro Wilcoxon test is applied to determine the normality of the data and is hypothesized as H0: The data are normally distributed. The results showed that the p-value of the test statistics is not significant at a 5% significance level; thus, data is not normally distributed (H1).
Table 2. Descriptive statistics
4.1. Unit root test results
The ADF and PP unit root tests are applied to test unit root or stationarity in time series. It can be hypothesized as H0: Data of selected option strategies payoff, option premiums, and equity payoffs have unit root or are not stationary. The ADF and PP unit root test statistics are significant at a 5% significance level. Hence, H0 has been rejected, and thus, the data of payoffs of selected option strategies and selected equities do not have unit root or stationery (H1; ).
Table 3. Results of augmented Dickey-Fuller test and Phillips-Perron unit root test
4.2. Hedging effectiveness of option strategies
Further, the study investigated the hedging effectiveness of the selected option strategies under neutral and volatile market conditions by applying panel regression analysis. The study applied the POLS approach, and a further cross-sectional dependence test, i.e., the Breusch-Pagan LM test, is used to determine the heteroskedasticity in the POLS results. This test can be hypothesized as H0: The models have no cross-sectional dependence. The p-value of the Breusch-Pagan LM test is less than 0.05; hence, hypotheses are rejected at a 5% level of significance. Further, the redundant fixed effect tests are applied to examine the significant effects. The null hypothesis of this test can be written as H0: the effects are redundant in the pooled regression model. The results reject the null hypothesis at a 5% level of significance (p < 0.05) and accept that the effects are statistically significant. Hence, FEM and REM are applied, and the Hausman test is conducted to identify the appropriate model between FEM and REM. The Durbin-Watson test results indicated the absence of autocorrelation, and the F-statistics of the F-test were found to be significant at a 5% significance level for all the models, indicating that models are a good fit.
The panel regression results of the hedging effectiveness of the covered call strategy is demonstrated in . The Hausman test results observed that the REM is more appropriate than the FEM under volatile and neutral market conditions (Chi-square statistic of 0.0000). Equity payoff and OTM call premium have a significant positive influence on covered call strategy payoff. The coefficient values of equity payoff and OTM call premium showed that a one-rupee change in the equity payoff and OTM call premium influences covered call payoff by 0.133 and 1.166 rupees, respectively. Further, the study measures the goodness-of-fit for regression models using the R2 value. It tests the frequency of the interaction between the model and the dependent variable. The R2 explains how much the independent variables explain the variation of a dependent variable in a regression model. In this case, the R2 value for the covered call strategy is 0.6963, implying that 69.63% variation in the covered call payoff is explained by the equity payoff and OTM call premium variation. Any model having R2 values of 0.75, 0.50, or 0.25 can be described as the substantial or best effective, moderate, or weak effective for hedging (J. F. Hair et al., Citation2011and F. F. Hair et al., Citation2014). The R2 value of 0.6963 for the covered call strategy indicates moderate effectiveness for hedging under both market conditions.
Table 4. Covered call strategy
Thus, the panel regression model for REM in volatile and neutral market conditions takes the following form:
The panel regression results of the hedging effectiveness of the covered put strategy are exhibited in . The results of the Hausman test revealed that the FEM is more appropriate than the REM in neutral market conditions (Chi-square statistic of 37.5367), whereas the REM is more efficient in volatile market conditions (Chi-square statistic of 0.0000). The equity payoffs significantly negatively influence covered put strategy payoff in volatile and neutral market conditions. It indicates that an additional increase or decrease in equity payoff by one rupee impacts the covered put strategy’s payoff by −0.076 rupees. OTM put premiums significantly positively influence the covered put strategy’s payoff. Hence, an additional change in OTM put premium by one rupee influences the strategy’s payoff by 1.069 and 1.056 rupees under volatile and neutral market conditions. The R2 values have explained 87% and 90.45% variation in the covered put payoffs by the variation in equity payoff and OTM put premium under volatile and neutral market conditions. The R2 values of 0.87, and 0.9045 for the covered put strategy indicate best or substantial effectiveness for hedging under volatile and neutral market conditions.
Table 5. Covered put strategy
The panel regression model for the REM in volatile market conditions and FEM in neutral market conditions takes the following form:
The results of panel regression models for collar strategy under both market conditions are reported in . The FEM is considered as more appropriate than the REM under both market conditions (the Chi-square value of the Hausman test is 32.4213). The equity payoff and ATM put premium have a significant negative influence on collar strategy payoffs. The coefficient values indicate that an additional change in equity payoff and ATM put premium by one rupee influences the collar strategy payoffs by −0.417 rupees and −0.654 rupees, respectively, under volatile and neutral market conditions. However, OTM call premiums have a significant positive impact on the strategy’s payoff, and a one rupee increase in OTM call premium influence the strategy payoff by 1.624 rupees. The R2 value of 0.5390 indicated that 53.90% variation in collar strategy’s payoff is explained by equity payoff, OTM call premium, and ATM put premium under both market conditions. This strategy showed moderate effectiveness for hedging under volatile and neutral market conditions.
Table 6. Collar strategy
The panel regression model for FEM in volatile and neutral market conditions takes the following form:
The results of the hedging effectiveness of the synthetic long call strategy are exhibited in . The Hausman test results reported that the REM is more appropriate under volatile market conditions (Chi-square statistic of 0.0000), and the FEM is more efficient under neutral market conditions (Chi-square statistic of 26.3199). Equity payoffs negatively influence synthetic long call strategy payoff with a coefficient value of −0.026 rupees under both market conditions. However, OTM put premiums significantly positively impact synthetic long call strategy. It can be interpreted as an additional change in OTM put premium by one rupee influences the payoffs by 0.233 rupees and 0.164 rupees under volatile and neutral market conditions. The R2 values infer that the variation in equity payoffs and OTM put premiums explains 0.97% and 10.08% variations in synthetic long call payoffs in volatile and neutral market conditions. The R squared values of 0.0097 & 0.1008 indicated weak effectiveness for hedging under volatile and neutral market conditions.
Table 7. Synthetic long call strategy
The panel regression model for REM in volatile market conditions and FEM in neutral market conditions takes the following form:
4.3. Comparison of payoffs of selected option trading strategies
Further, the study attempted to compare the payoffs of different strategies to conclude the differences between one strategy’s payoff to another strategy’s payoff using a statistical approach. The Shapiro-Wilcoxon test results indicated that the data considered for the study is not normally distributed (); Hence, this study adopted a non-parametric method for comparison, i.e., the Kruskal-Wallis test, to find out whether the group medians are equal or not. The results indicate that there is a difference in the medians from one group to another group (Test statistic 721.584 and p =<.001; ). Hence it is essential to identify the groups with significant mean/median differences. For this, the Games-Howell Post Hoc Tukey Test is applied.
Table 8. Results of Kruskal-Wallis test
The Games-Howell Post Hoc Tukey Test results showed significant differences for covered call to covered put strategy and covered call to collar strategy at a 99% significance level (p =0.005 and 0.006, respectively). Significant differences were found for the covered put to collar strategy (p =<0.001) at a 99.9% significance level. Covered call to synthetic long call, covered put to synthetic long call, and synthetic long call to collar strategies have observed no significant mean differences between the groups. The study observed that only three groups have significant mean differences out of 6 groups ().
Table 9. Results of Games-Howell Post Hoc Test
5. Discussions
The hedging effectiveness of the covered call, covered put, collar, and synthetic long call strategies and a comparison of their mean payoffs are discussed in this section. The hedging effectiveness of the covered call strategy found that the OTM call premiums significantly influence covered call payoff more than equity payoff under both market conditions (). Besides, the results showed that selling an OTM call option provides an absolute benefit to the investor over equity payoff (Diaz & Kwon, Citation2016). The investor receives a premium for the call sold, which is an additional income for the risk taken. Thus, the study findings support the findings of (Dewobroto et al., Citation2010; Mugwagwa et al., Citation2012and Niblock & Sinnewe, Citation2018). The results of the covered put strategy found that equity payoffs have a negative influence on covered put strategy payoff under both market conditions. However, taking a short position in equity during the construction of the strategy restricts the payoff from equity (Kang et al., Citation2021). Hence, equity payoffs showed a significant negative influence on the strategy’s payoff. Moreover, OTM put premiums positively influence the strategy’s payoff. The findings indicated that the premium received for selling an OTM put provides an additional income to the investor (Kang et al., Citation2021).
Further, the results of the collar strategy showed that OTM call premiums have a significant positive influence, and selling an OTM call provides an absolute benefit to the investors (; Bartonova, Citation2012). However, ATM put premiums negatively influence collar strategy payoff and reduce the absolute benefit received for call option sold (Israelov & Klein, Citation2016). The reduction in absolute benefit is that the ATM put option expires worthless. Hence, this finding is consistent with the findings of (El-Hassan et al., Citation2018). The equity payoffs significantly negatively impact the synthetic long call strategy’s payoff. As a result, any changes in the equity prices impact the strategy payoff negatively. This finding supports the findings of (Mohil et al., Citation2020).
Holistically, the study observed the positive impact of equity payoff on strategy’s payoff only for the covered call strategy, and the remaining strategies showed negative influence (Dewobroto et al., Citation2010). Moreover, the covered put strategy evidenced a higher R square value, while the synthetic long call strategy reported a lower R square value ().
Table 10. Comparison of R squared and hedge ratios
The second approach of our study compared the payoffs of a covered call, covered put, collar, and synthetic long call strategy. For this purpose, the study has applied the Kruskal-Wallis Test and Games-Howell Post Hoc Tukey Test. The study found significant mean differences between the covered call to covered put strategy and the covered call to collar strategy (Dewobroto et al., Citation2010). However, synthetic long call strategy payoffs have equal mean payoffs compared to other option hedging strategies (no significant mean differences).
6. Robustness checks
Applying robustness checks or diagnostic tests to the analysis is essential to establish that the estimated model adequately describes an economic phenomenon (BEGGS, Citation1988). The study applied the Breusch-Pagan LM test for verifying heteroskedasticity in the POLS model, the Hausman test for selecting an appropriate model between FEM and REM, and the Durbin-Watson test for checking autocorrelation in the regression models. The Breusch-Pagan LM test reported the presence of heteroskedasticity in the POLS model; hence, FEM and REM models are applied. The Hausman tests have identified appropriate models between FEM and REM for all the hedging strategies. Further, the Durbin-Watson test statistic reported the absence of autocorrelation in the regression models. Hence, the results are verified with the assumptions of diagnostic tests and confirmed that the results are robust.
7. Conclusion
The present paper compared the hedging effectiveness of covered call, covered put, collar, and synthetic long call strategies in the Indian derivatives market for 12 years from 2009 to 2020 under volatile and neutral market conditions. The study addressed the applicability of options strategies for hedging the equity using the panel regression approach. The study results suggest that the covered put and covered call strategies have greater hedging effectiveness than other hedging strategies. The investors can earn nominal returns by employing a covered call strategy in both market conditions. The study has evidenced the applicability of the covered put strategy in hedging the equity position. However, collar and synthetic long call strategies offer lower hedging effectiveness against the equity price risk.
Additionally, the study compared payoffs of option hedging strategies and identified mean differences between the groups are equal or not equal. The study results found that the payoff of the covered call strategy has significant mean differences with covered put and collar strategies, whereas the payoff of the synthetic long call strategy has no statistically significant mean differences with other strategies. Hence, the study establishes a benchmark to help retail investors choose a better hedging strategy and employ it in their trading, specific to the market condition.
Further, this paper provides scope for expanding research work in this area. This study does not consider brokerage or commission charges payable to the brokering firm, which directly or indirectly affects the final strategy’s payoff. Future studies can consider other variables such as brokerage or commission and taxes paid while calculating the strategy’s payoff. Further, this research has compared the hedging effectiveness of four strategies for twenty-six companies that are part of the top six sectors of NSE and are available for trading in equity derivatives from 2009 to 2020. Future studies can apply and compare the effectiveness of these strategies in other emerging derivative markets.
Disclosure statement
There are no relevant financial or non-financial competing interests to report.
Additional information
Funding
Notes on contributors
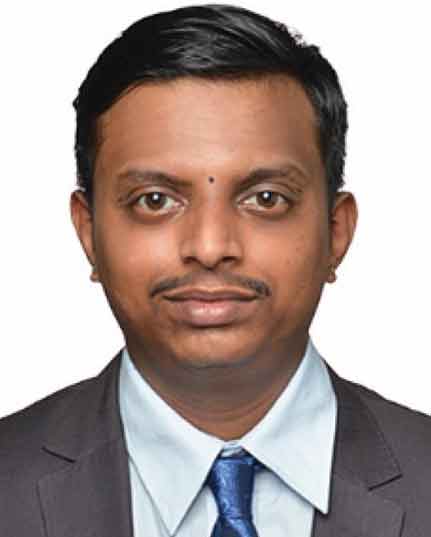
Shivaprasad S P
Mr. Shivaprasad S P (first author) is a research scholar at the Department of Commerce, Manipal Academy of Higher Education, Manipal, Karnataka, India. His areas of interest include Portfolio Management, Financial Derivatives, Financial Accounting, and Taxation.
Geetha E
Dr. Geetha E (corresponding author) is presently working as an Associate Professor at the Department of Commerce, Manipal Academy of Higher Education, Manipal, Karnataka, India. Her teaching areas include Financial Accounting, Portfolio Management, Financial Management, and Business Statistics.
Raghavendra Acharya
Dr. Raghavendra Acharya (co-author) has over 20 years of teaching and research experience in India and presently working as a Professor and Associate Dean at VIT-AP School of Business, VIT-AP University, Amaravati, Andhra Pradesh. He has published papers in esteemed academic journals, and his areas of interest include Capital Market, Financial Derivatives, Portfolio Management, Financial Management, and Management Accounting.
Vidya Bai G
Dr. Vidya Bai G (co-author) is working as an Associate Professor at the Department of Commerce, Manipal Academy of Higher Education, Manipal. Her teaching and research areas include Financial analytics, Financial modelling, and Operations Research.
Rajeev Matha
Mr. Rajeev Matha (co-author) is pursuing a Ph.D. under the Dr. T.MA Pai scholarship program at the Department of Commerce, Manipal Academy of Higher Education. His area of interest includes Behavioural Finance, Econometrics, Capital Market, and Structural Equation Modelling.
Notes
1. Historical growth in FO segment in Indian capital market. https://www1.nseindia.com/products/content/derivatives/equities/historical_fo_bussinessgrowth.htm
2. Sectoral indices considered for the study are Nifty Bank Index, Nifty Auto Index, Nifty Pharma Index, Nifty IT Index, Nifty PSU Bank Index and Nifty Private Bank Index.
References
- Abou Elseoud, M. S., Yassin, M., Ali, M. A. M., & McMillan, D. (2020). Using a panel data approach to determining the key factors of Islamic banks’ profitability in Bahrain. Cogent Business & Management, 7(1), 1831754. https://doi.org/10.1080/23311975.2020.1831754
- Addae-Dapaah, K., & Abdullah, K. (2020). Cross hedging effectiveness of real estate securities exchange traded funds. Journal of Real Estate Portfolio Management, 261, 1–24. https://doi.org/10.1080/10835547.2020.1826240
- Antonakakis, N., Cunado, J., Filis, G., Gabauer, D., & de Gracia, F. P. (2020). Oil and asset classes implied volatilities: Investment strategies and hedging effectiveness. Energy Economics, 91 , 104762. https://doi.org/10.1016/j.eneco.2020.104762
- Baltagi, B. (2021). Econometric analysis of panel data. Springer.
- Bangur, P. (2020). Strangle to resuscitate: Evidence from India. The Journal of Investment Strategies, 9(2), 1–14. https://doi.org/10.21314/jois.2020.121
- Bartonova, M. (2012). Hedging of sales by Zero-cost collar and its financial impact. Journal of Competitiveness, 4(2), 111–127. https://doi.org/10.7441/joc.2012.02.08
- Basson, L., van den Berg, L., van Vuuren, G., McMillan, D., & McMillan, D. (2018). Performance of two zero-cost derivative strategies under different market conditions. Cogent Economics & Finance, 6(1), 1492893. https://doi.org/10.1080/23322039.2018.1492893
- BEGGS, J. J. (1988). Diagnostic testing in applied econometrics. Economic Record, 64(2), 81–101. https://doi.org/10.1111/j.1475-4932.1988.tb02044.x
- Bhaduri, S. N., & Sethu Durai, S. R. (2008). Optimal hedge ratio and hedging effectiveness of stock index futures: Evidence from India. Macroeconomics and Finance in Emerging Market Economies, 1(1), 121–134. https://doi.org/10.1080/17520840701859856
- Bhat, A. (2021). The profitability of volatility trading on exchange-traded dollar-rupee options: Evidence of a volatility risk premium? Global Business Review, 097215092110461. https://doi.org/10.1177/09721509211046169
- Bhatia, V., Das, D., & Kumar, S. B. (2020). Hedging effectiveness of precious metals across frequencies: Evidence from wavelet based dynamic conditional correlation analysis. Physica A: Statistical Mechanics and Its Applications, 541, 123631. https://doi.org/10.1016/j.physa.2019.123631
- Black, F., & Scholes, M. (1973). The pricing of options and corporate liabilities. Journal of Political Economy, 81(3), 637–654. https://doi.org/10.1086/260062
- Board, J., Sutcliffe, C., & Patrinos, E. (2000). The performance of covered calls. The European Journal of Finance, 6(1), 1–17. https://doi.org/10.1080/135184700336937
- Bonga-Bonga, L., & Umoetok, E. (2016). The effectiveness of index futures hedging in emerging markets during the crisis period of 2008-2010: Evidence from South Africa. Applied Economics, 48(42), 3999–4018. https://doi.org/10.1080/00036846.2016.1150948
- Breusch, T. S., & Pagan, A. R. (1980). The lagrange multiplier test and its applications to model specification in econometrics. The Review of Economic Studies, 47(1), 239. https://doi.org/10.2307/2297111
- Chen, A.-S., & Leung, M. T. (2003). Option straddle trading: Financial performance and economic significance of direct profit forecast and conventional strategies. Applied Economics Letters, 10(8), 493–498. https://doi.org/10.1080/1350485032000095375
- Dewobroto, D., Febrian, E., Herwany, A., & Khresna Br, R. (2010). The best stock hedging among option strategies. Research Journal of Applied Sciences, 5(6), 397–403. https://doi.org/10.3923/rjasci.2010.397.403
- Diaz, M., & Kwon, R. H. (2016). Optimization of covered call strategies. Optimization Letters, 11(7), 1303–1317. https://doi.org/10.1007/s11590-016-1083-8
- Dickey, D. A., & Fuller, W. A. (1979). Distribution of the Estimators for Autoregressive Time Series with a Unit Root. Journal of the American Statistical Association, 74(366), 427. https://doi.org/10.2307/2286348
- Dickey, D. A., & Fuller, W. A. (1981). Likelihood Ratio Statistics for Autoregressive Time Series with a Unit Root. Econometrica, 49(4), 1057. https://doi.org/10.2307/1912517
- EDERINGTON, L. H. (1979). The hedging performance of the new futures markets. The Journal of Finance, 34(1), 157–170. https://doi.org/10.1111/j.1540-6261.1979.tb02077.x
- El-Hassan, N., Hall, A., & Tulunay, I. (2018). Methods and performances of collar strategies (pp. 1–39). https://www.researchgate.net/publication/331486055
- Figlewski, S., Chidambaran, N. K., & Kaplan, S. (1993). Evaluating the performance of the protective put strategy. Financial Analysts Journal, 49(4), 46–56. https://doi.org/10.2469/faj.v49.n4.46
- Fontanills, G. (2005). The options course: High profit & low stress trading methods. Wiley, Copyr.
- FRANCKLE, C. T. (1980). The hedging performance of the new futures markets: Comment. The Journal of Finance, 35(5), 1273–1279. https://doi.org/10.1111/j.1540-6261.1980.tb02211.x
- Fullwood, J., James, J., & Marsh, I. W. (2021). Volatility and the cross-section of returns on FX options. Journal of Financial Economics, 141(3), 1262–1284. https://doi.org/10.1016/j.jfineco.2021.04.030
- Goltz, F., & Lai, W. N. (2009). Empirical properties of straddle returns. The Journal of Derivatives, 17(1), 38–48. https://doi.org/10.3905/jod.2009.17.1.038
- Guo, B., Shi, Y., & Xu, Y. (2020). Volatility information difference between CDS, options, and the cross section of options returns. Quantitative Finance, 20(12), 2025–2036. https://doi.org/10.1080/14697688.2020.1814018
- Hair, J. F., Ringle, C. M., & Sarstedt, M. (2011). PLS-SEM: Indeed a silver bullet. Journal of Marketing Theory and Practice, 19(2), 139–152. https://doi.org/10.2753/mtp1069-6679190202
- Hair, F.,sJr, Sarstedt, J., Hopkins, M., Kuppelwieser, L., & V, G. (2014). Partial least squares structural equation modeling (PLS-SEM): An Emerging tool in business research. European Business Review, 26(2), 106–121. https://doi.org/10.1108/ebr-10-2013-0128
- Hoffmann, A. O. I., & Fischer, E. T. S. (2012). Behavioral aspects of covered call writing: An empirical investigation. Journal of Behavioral Finance, 13(1), 66–79. https://doi.org/10.1080/15427560.2012.657314
- Israelov, R., & Klein, M. (2016). Risk and return of equity index collar strategies. The Journal of Alternative Investments, 19(1), 41–54. https://doi.org/10.3905/jai.2016.19.1.041
- Israelov, R., & Nielsen, L. N. (2015). Covered calls uncovered. Financial Analysts Journal, 71(6), 44–57. https://doi.org/10.2469/faj.v71.n6.1
- Kang, B. J., Eom, C., Lee, W. B., Chang, U., & Park, J. W. (2021). A study of the performance of option strategy benchmark index in global option markets. Korean Journal of Financial Studies, 50(4), 439–472. https://doi.org/10.26845/kjfs.2021.08.50.4.439
- Kedžo, M. G., & Šego, B. (2021). The relative efficiency of option hedging strategies using the third-order stochastic dominance. Computational Management Science, 18(4), 477–504. https://doi.org/10.1007/s10287-021-00401-z
- Kumar Meher, B., Thonse Hawaldar, I., Spulbar, C., & Birau, R. (2021). Forecasting stock market prices using mixed ARIMA model: A case study of Indian pharmaceutical companies. Investment Management and Financial Innovations, 18(1), 42–54. http://dx.doi.org/10.21511/imfi.18(1).2021.04
- Leggio, K. B., & Lien, D. (2002). Covered call investing in a loss aversion framework. Journal of Psychology and Financial Markets, 3(3), 182–191. https://doi.org/10.1207/s15327760jpfm0303_6
- Lien, D. (2008). A further note on the optimality of the OLS hedge strategy. Journal of Futures Markets, 28(3), 308–311. https://doi.org/10.1002/fut.20297
- Luo, H. R., & Wang, R. (2018). Foreign currency risk hedging and firm value in China. Journal of Multinational Financial Management, 47-48, 129–143. https://doi.org/10.1016/j.mulfin.2018.11.002
- Mohil, S., Nayyar, R., & Patro, A. (2020). When is informed trading more prevalent?—An examination of options trading around Indian M&A announcements. Journal of Futures Markets, 40(6), 1011–1029. https://doi.org/10.1002/fut.22102
- Mugwagwa, T., Ramiah, V., Naughton, T., & Moosa, I. (2012). The efficiency of the buy-write strategy: Evidence from Australia. Journal of International Financial Markets, Institutions and Money, 22(2), 305–328. https://doi.org/10.1016/j.intfin.2011.10.001
- Niblock, S. J., & Sinnewe, E. (2018). Are covered calls the right option for Australian investors? Studies in Economics and Finance, 35(2), 222–243. https://doi.org/10.1108/sef-07-2016-0164
- O’Connell, D., & O’Grady, B. (2014). The buy-write strategy, index investment and the efficient market hypothesis:More Australian evidence. The Journal of Derivatives, 22(1), 71–89. https://doi.org/10.3905/jod.2014.22.1.071
- Phillips, P. C. B., & Perron, P. (1988). Testing for a unit root in time series regression. Biometrika, 75(2), 335–346. https://doi.org/10.1093/biomet/75.2.335
- Rao, P., Goyal, N., Kumar, S., Hassan, M. K., & Shahimi, S. (2021). Vulnerability of financial markets in India: The contagious effect of COVID-19. Research in International Business and Finance, 58, 101462. https://doi.org/10.1016/j.ribaf.2021.101462
- S. P. S, E. G, Raghavendra, L. K and Matha R. (2022). Choosing the right options trading strategy: Risk-return trade-off and performance in different market conditions. Investment Management and Financial Innovations, 19(2), 37–50. https://doi.org/10.21511/imfi.19(2).2022.04
- Samuel, Y. M. Z.-T. (2017). Option implied beta and option return. Applied Economics, 50(2), 128–142. https://doi.org/10.1080/00036846.2017.1313958
- Singh, G. (2017). Estimating optimal hedge ratio and hedging effectiveness in the NSE index futures. Jindal Journal of Business Research, 6(2), 108–131. https://doi.org/10.1177/2278682117715358
- Whaley, R. E. (2002). Return and risk of CBOE buy write monthly index. The Journal of Derivatives, 10(2), 35–42. https://doi.org/10.3905/jod.2002.319194
Appendix
Descriptive Box plots
Box plots represent the numeric data such as median, quartiles, upper and lower adjacent points, etc. Each strategy’s numeric data is described in different box plots. represents the box plots of a covered call, covered put, collar, and synthetic long call strategies.