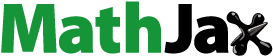
Abstract
Financial distress (FD) is a crucial issue that needs to be resolved in time; otherwise, it may adversely influence society’s areas. Therefore, identifying the factors responsible for FD and how these factors affect FD is an exciting area of research. Given this, this paper proposes investigating the effect of competition on the bank’s FD in India. This paper uses the data of 34 banks operating in India for the observation period (2016–2019). Three variants of Altman’s Z-score are used for the assessment of FD. Lerner’s index of the banks is developed for the competition measurement. A panel data analysis is further performed for regression analysis. The current study has an interesting outcome. The findings reveal that high market power or low competition lowers financial distress and adds more financial stability in banks in India. This paper provides fresh evidence on the connection between market power and FD of banks in India. Moreover, no other study has been observed to use all the three variants of Altman’s z-score to provide robustness and reliability in results. Therefore, the study has a significant contribution and a novelty in its approach.
1. Introduction
Financial distress (FD) is an issue that needs specialized attention because of its probable consequences (Habib et al., Citation2020; Lizal, Citation2002). Such consequences span all the areas of society, which may adversely affect all the firm’s stakeholders, including society and the environment (Barney & Harrison, Citation2020; Freeman et al., Citation2010). Financial distress may not always culminate into bankruptcy, but stress beyond a threshold is always deleterious for the firms (Gordon, Citation1971). Before embarking upon a discussion on FD, it is pertinent to define it for clarity so the context in which it is used in the study should be established. FD is defined as a situation in which a firm cannot meet its financial obligation as and when it comes up (Garlappi & Yan, Citation2011; Ikpesu, Citation2019). The definition of FD taken in the study discernably includes any outcome that encompasses failure, insolvency, bankruptcy, or default to meet firms’ financial obligations (Habib et al., Citation2020). However, the definition of FD is a generic definition apt for banks as well.
Among several types of firms, banks have a key role and work as the backbone of the economy. It handles the nation’s financial system by its twofold activity of collecting deposits and providing loans in different ways (Kishore, Citation2015; Pradhan, Citation2014). Hence, financial stability of banks should be under the radar of all concerned stakeholders. The major cause of the financial crisis of 2008 is found to be the failure of financial institutions. The recent bank failures in India such as Punjab and Maharashtra Co-operative Bank (PMC), Punjab and Sindh Bank, and Yes Banks, have created the serious concerns towards exploring the factors responsible for FD in banks (Jasrotia & Agarwal, Citation2021). The rising NPAs (one of the main causes of FD) in Indian banks is mentioned in . Therefore, researches in this area are the need of the hour to layout reliable evidence on responsible factors for bank’s financial health.
Figure 1. Rising NPA in India.
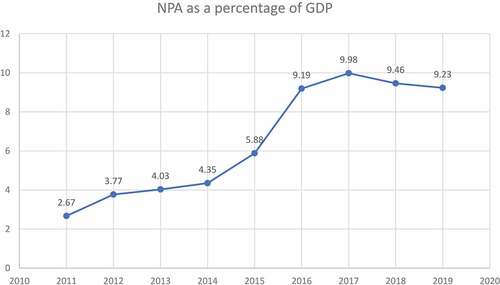
Literature does dwell into to explicate the reasons of FD for both firms (Habib et al., Citation2020) and banks (Zaki et al., Citation2011). The voluminous literature on the topic deduces the following three categories (Habib et al., Citation2020; Lizal, Citation2002) of the reasons for FD: 1) firm-specific financial reasons; 2) governance issues; and 3) exogenous factors. Out of the three reasons, we find that the last category, exogenous factors, are not explored well, and their association with FD is yet to be established (Amendola et al., Citation2015; Habib et al., Citation2020; Ikpesu, Citation2019; Zhang et al., Citation2016).
Exogenous factors are beyond the realm of banks or firms (Habib et al., Citation2020). Moreover, exogenous issues envelop many factors within the giant umbrella of exogenous issues for FD (Habib et al., Citation2020; Lizal, Citation2002). One of the essential factors for FD in the bigger basket of exogenous issues is competition. Surprisingly, many studies relate competition and distress in firms and banks (Majeed et al., Citation2018; Zaki et al., Citation2011). However, the impact of competition on financial stability (or financial distress) cannot be ascertained with unanimity. The literature is full of diverse views on the association between the two. One set of studies, across all types of firms, including banks, find evidence that competition increases financial stability and is helpful in the growth of the firms (Noman et al., Citation2018; Obembe & Soetan, Citation2015; Saha & Dutta, Citation2020). Another set of studies propounds that competition is determinantal for financial stability and increases the risk (Alam et al., Citation2019a; Beck et al., Citation2013; A. Berger et al., Citation2009; Gyimah et al., Citation2021). Another point of view advocates that competition does not impact financial stability at all (financial distress; Yazdanfar & Öhman, Citation2020).
There is no consensus on the role of competition on the firms’ financial stability, including banks. Hence a fresh set of evidence is required to establish the association between competition and financial distress in the banks in India. Thus, the following objective is framed for the current study: to assess the impact of competition on the financial distress of the banks (while controlling for regulation, size, profitability and valuation of the banks).
Undoubtedly, the policy on the competition is directly or indirectly linked to concentration banking (Alegria & Schaeck, Citation2008; Dávila & Walther, Citation2020; Deidda & Fattouh, Citation2005). The policy on the competition with FD will also have implications for policy on concentration banking. This output enhances the importance of the current study. This situation is the primary motivation to explore the association of competition on the FD of the banks.
Interestingly, the paper’s findings are unique as there is very thin literature on the role of competition for banks’ financial stability. Furthermore, the paper’s findings support less competition in the banking sector, which de facto support concentration banking (Alegria & Schaeck, Citation2008; A. N. Berger et al., Citation2004). Looking at the vast area to be covered in the countries like India with the vast population base needing a formal financial system, the findings of this paper can be construed to support differentiated banking structure (Carter & Mcnulty, Citation2005; Kishore, Citation2015). These findings are the significant contribution of the current study, which are fresh and novel evidence to support the less competition in the banking sector for their financial stability. The main implications of the study are: 1) The Government and policymakers would be decisively clear on the issue of competition in the banks in India (Alam et al., Citation2019b); 2) the recently started consolidation of banks may get a boost to accept it as a long-term policy, especially from the banks’ stability point of view (Jasrotia & Agarwal, Citation2021); 3) the existing differentiated banking system may also get accepted as a long-term policy (Jayadev et al., Citation2017; Misra, Citation2006; Reddy, Citation2018).
The rest of the paper is arranged into seven sections, including the Introduction section. The second section is on the theoretical background and financial distress in the Indian banks. The third section is on the relevant literature review to deep dive into the current work and identify gaps on the topic. The fourth section is on the data and methodology applied in the paper, followed by the results of the data analysis in the next section. The sixth section talks about the discussion on the results of the current study in comparison with the earlier research work. The paper is concluded in the seventh section.
2. Theoretical background and financial distress in India
2.1. Rising NPA and FD in banks in India
NPA (Non-performing Assets) levels in India are on the rise or not coming down. NPA are the assets which come under such credit or loan for which the interest or principal amount remains overdue for certain time-period (i.e. 90 days or more; RBI, Citation2021). In terms of percentage of GDP, it is close to 10% (World Bank, Citation2019). In another measure, it is in 2018 close to 14% of the total advances in the public sector bank which roughly three times more than their private counterparts (Oliver, Citation2017). Together (private and public banks), it is around 9% during the same period (Agarwal et al., Citation2020). It is a worrisome figure, especially when the public sector calls the shots in terms of total advances in India (The public sector has 58% higher advances than private sector banks; RBI, Citation2021). The problem of NPA seems to be more troublesome because of the quality of the assets and opaqueness in the definition of bad assets and its timely disclosures (Narula & Singla, Citation2014; Shajahan, Citation1998; K. v. N. Prasad & Ravinder, Citation2012). It is clear that banks, especially public sector banks, are financially stressed in India. Above all, bringing legislation to introduce newer kinds of banks exacerbates the condition of the banks in India because of increased competition (Maji & Hazarika, Citation2018; Reddy, Citation2018). However, there are instances of bank consolidation (Jasrotia & Agarwal, Citation2021), which is a move towards DBS (Differential Banking System; Kishore, Citation2015). The whole discussion can be deduced that India’s banks are financially stressed due to various reasons, including competition. Therefore, this study is justified to demist how competition affects the financial stress and stability of the banks in India.
2.2. FD concerning India in the aftermath of the 2008 world financial crisis
The world financial crisis of 2008 (Rastogi, Citation2014) was the last straw as it shook the tree and made everyone across the world recognize issues in the global banking and financial system (Moosa, Citation2010; Reinhart & Rogoff, Citation2011), including in India (Agarwal et al., Citation2020). Even BASEL is also severely criticized for its failure to address the problem of all types of risks in banking worldwide (Allen et al., Citation2012; Demirgüç-Kunt & Detragiache, Citation2011; Pakravan, Citation2014; Schwerter, Citation2011). Literature regarding the performance of BASEL post-2008 crisis puts a big question mark on the even sanctity of BASEL (Samitas & Polyzos, Citation2015). The insinuation made by the crisis of 2008 alerted everyone to preempt and proactively take stock of the situation. Even though India was least affected by the crisis (A. Prasad & Reddy, Citation2009), it made RBI and other policymakers recognize issues in the Indian banking and financial system, mainly rising NPA in the public sector banks (Agarwal et al., Citation2020).
India has a unique legal and institutional framework (Agarwal et al., Citation2020). Despite that, NPA gradually becomes a grave matter of concern (Joseph & Prakash, Citation2014). Besides other acts and legal frameworks in 2002, the SARFAESI Act was brought in India to facilitate lousy debt management and improve banks’ conditions concerning NPA (Singh, Citation2016). Having such a robust ecosystem, India falters concerning its NPA issues. India suffers from 4Rs, as aptly described by the Finance Minister of India in 2019: 1) lack of transparency in recognizing the distressed assets; 2) lack of resolve in recovering the bad debt despite the existence of the legal and institutional setup; 3) to recapitalize banks especially public sector banks; and 4) to bring reforms in the whole banking system so that procedures are followed in letter and spirit (Agarwal et al., Citation2020; Jain & Jain, Citation2020). Among other things, all the reforms and corrections, in 2016, India took a giant leap by introducing the Insolvency and Bankruptcy Act 2016 to rectify the situation of NPA in the long run (Gupta, Citation2018).
2.3 Insolvency and Bankruptcy Code (IBC): a failed attempt or a future possibility to the woes of FD in Indian banking
In India, a new era regarding bad and stressed assets is ushered by enacting the Insolvency and Bankruptcy Code Act 2016 (IBC, Citation2016). The basic theme of the Insolvency and Bankruptcy Code (IBC) act is to let a defaulting firm die its natural death if it can be converted into a healthy firm instead of artificially keeping the dying firm alive (IBC, Citation2020). However, the IBC Act has achieved very little success as yet in terms of achieving its goals. It would not be wrong to claim IBC Act as a missed opportunity (Deb & Dube, Citation2020; Gupta, Citation2018; Pryor & Carg, Citation2020).
As it is evident with the above discussion that India’s chequered history regarding FD is futile. India is still grappling with the issues of FD and its repercussions despite watershed legislation of IBC Act 2016 for it. Therefore, it is justified to explore further how competition exacerbates the already fragile and precarious condition of banks in India regarding FD.
3. Literature Review and Hypotheses formulation
Distress in the bank is studied well, and literature discusses it in length. We present a literature survey of the relevant studies on the topic through four categories to highlight the research gap and recognize the problems addressed in the study.
3.1. FD and Firm-specific financial factors
Financial distress and firm-specific financial factors are closely intertwined with one another. Both FD and firm-specific financial factors (henceforth, financial factors only will be used referring to firm-specific financial factors) are obvious bedfellows. Because FD may happen for any reason (financial, non-financial or exogenous), it will eventually be translated into financial numbers, which may give the false impression that only financial factors are the causes of FD. This situation may be the root cause of literature on firm-specific financial causes for FD of firms and banks alike.
In a study on FD of banks in UAE during 2000–2008 (before the world financial crisis of 2007–08) through 16 banks (12 conventional banks and 4 Islamic banks) by Zaki et al. (Citation2011), only firm-specific financial factors are found to be significant with financial distress. The main financial ratios identified in the study are cost-income ratio, provision coverage ratio and equity to total asset ratio. Paule-Vianez et al. (Citation2019) also find only firm-specific financial factors as the determinants of the FD in 148 credit institutions, including banks in Spain, during 2012–2016. They use Artificial Neural Network methodology and different definitions of FD to identify CAMEL (Kabir & Dey, Citation2012) based financial ratios as the right set of predictors of FD. In a study on 101 Islamic banks that operate globally, Halteh et al. (Citation2018) explore the association of 18 financial variables with FD using Machine Learning tools. They propound that working capital divided by total asset is the most crucial financial ratio to predict the FD.
Studies other than banks also give excessive importance to financial factors compared to anything else as the determinants of FD. Sehgal et al. (Citation2021) use five financial ratios, including cash to total liability, from 1742 listed Indian firms during 2010–2016 to describe the financial distress without acknowledging any other thing as the determinant of FD. Farooq et al. (Citation2018) use more than 400 Pakistani listed firms to advocate that mild liquidity and profit reduction are the early signs of FD in recovering firms. However, as mild liquidity issues become severe liquidity problems, recovering such forms from FD becomes rare. In a study on Ethiopian firms during 2002–2012, Gebreslassie (Citation2015) find financial factors (NPA, capital to loan ratio and net interest margin)m as the significant determinants of FD. Jahur and Quadir (Citation2012) also present financial factors determining FD in Bangladesh’s SMEs (Small and Medium Enterprises). Baimwera and Muriuki (Citation2014) in Nairobi firms, Jaafar et al. (Citation2018) in Malaysian firms and Ikpesu (Citation2019) in the Nigerian firms find evidence that financial factors are the determinants of FD in the respective firms.
3.2. Firm-Specific non-financial issues of FD (including governance)
The firm-specific non-financial factors of FD are critical. It is believed that the other reasons of FD (financial factors and exogenous factors) can be managed effectively provided issues related to the governance are in order (Madrid-Guijarro et al., Citation2011). It is believed that if governance issues are in place, both the firm-specific financial and non-financial issues can be responded to and managed well (Camacho‐Miñano et al., Citation2020; Elloumi & Gueyié, Citation2001). Similarly, the same logic can also be deduced for the exogenous factors for FD. Good governance of the firm obviously cannot change the exogenous factors but can respond more effectively to the challenges (Lee & Yeh, Citation2004; Pramudena, Citation2017).
Al‐Tamimi (Citation2012) advocate that corporate governance has a significant association with FD in UAE based national banks. Wanke et al. (Citation2015), in their studies in Brazilian banks during 1996–2011, find evidence that size and private ownership of the banks are significantly associated with an increase in the efficiency of the banks and inversely proportional to Financial distress. Bakar and Noordin (Citation2021) explore both financial and non-financial factors in Malaysian firms as the determinants for FD using Machine Learning tools (Neural Network Methods). They find ownership of independent directors and institutional ownership have harmful (reduces the financial distress) association with FD. A study from Jordon on 59 publicly listed firms during 1989–2002 found that government, foreign and top-5 large shareholders were negatively associated with FD (default risk). Yousaf et al. (Citation2021) present in their study on Chinese firms during 2007–2016 that diversity in the board based on gender, age, education, expertise, and independence significantly influence the firms’ FD using statistical and machine learning tools. In a study on Indonesian firms during 2008–2013, Kristanti et al. (Citation2016) advocate that corporate governance significantly influences FD reduction. In a landmark study on CG and FD, Whitaker (Citation1999) found evidence that the situation of FD is not linked to the governance of the firms. However, exit from FD in the early stages is significantly associated with the governance or managerial actions of the firms. It is even true for the historically poorly run firms from the governance point of view.
3.3. Holistic approach of determinants of FD including exogenous determinants
The reason for FD can also be exogenous (macroeconomic indicators, regulation, market competition and overall structure of the marketplace). It can also be reasonably and logically argued that combining all the three (firm-specific financial and non-financial factors and exogenous factors) could be the real reason for FD in the firms, including banks. However, there is relatively limited evidence in the literature to corroborate the same.
Wanderi (Citation2016) find evidence in a study on 43 Kenyan banks during 2011–2015 that corporate governance and macroeconomic factors (including globalization and information technology) are significantly associated with the FD. Zhang et al. (Citation2016), in their study on 629 US banks during 2010–2013, find evidence that all three sets of reasons determine FD. They find that FD in US banks is related to non-performing loans (firm-specific financial factor), diversification of ownership and board (firm-specific non-financial factors) and housing price indicator and capital regulation (exogenous factors). Au contraire, it is found in another study by Zaki et al. (Citation2011) that firm-specific financial factors have a significant association with FD in UAE banks during 2000–2008. However, macroeconomic indicators are not significantly associated with FD. In a non-bank study of 3865 Swedish firms during 2008–2015, Yazdanfar and Öhman (Citation2020) explain that macroeconomic indicators and firm-specific characteristics are significantly associated with FD in the banks. Another study on non-bank of 92 Canadian banks by (Elloumi & Gueyié, Citation2001) found that apart from financial factors, components of corporate governance are significantly associated with FD. The studies on this area are pretty more minor, primarily concerned with the banks. Hence, this current study can bridge the gap in this regard.
3.4. Competition and FD
The holistic approach to explaining the FD considers one significant factor competition as a cause of FD. There is literature that endorses this point of view (Beck et al., Citation2006; Cetorelli, Citation2001). Here, it is seen that the competition (an exogenous factor) does not come alone. Instead, it is part of the more extensive set of factors where competition happens to be one of the essential factors for FD in the firms, including banks. The literature is voluminous on the association between competition and FD. However, there is no unanimity on the role of competition for FD in the banks across the world. One set of literature opines that competition supports reducing the FD in the firms, including banks. Another set of literature says that the role of competition for FD is negative, and competition increases financial distress. Above all, another literature set presents their neutral view viz-a-viz competition and FD in banks.
Among the mixed empirical evidence of competition-fragility theory (competition enhances FD) and competition-stability theory (Competition improves financial stability), the studies are skewed towards the competition-fragility hypothesis. Alam et al. (Citation2019b), in a study on ten leading Islamic banking countries of more than 200 banks (both Islamic and conventional banks), find evidence in favour of the competition-fragility hypothesis but for conventional banks only. In a study on 308 European banks during 1996–2009, Cipollini and Fiordelisi (Citation2012) opine that bank power is one of the essential determinants of bank value in a stressed bank. In other words, they confirm the competition-fragility hypothesis. A. Berger et al. (Citation2009) evince in their study on 8235 banks of 23 nations support the competition-fragility point of view. Other studies share similar views (Hanggraeni, Citation2018; Leroy & Lucotte, Citation2017).
The proponents of the competition-stability hypothesis insist that increased competition in the banking sector increases efficiency and brings them stability. Boyd and De Nicolo (Citation2005) argue that the concentration of banks (less competition) increases the risky portfolio, and stability is compromised. Beck et al. (Citation2013) present, defying the mixed empirical evidence (competition-stability and competition-fragility), favourable evidence for competition-stability provided stringent regulation, and supervisory effectiveness is found in order. They show that the reverse may be true (competition-fragility hypothesis) if regulation is weak and supervisory failures are witnessed. Alam et al. (Citation2019b) also identified similar conditional views. They use bank data from 10 highly developed Islamic banking nations of 59 Islamic banks and 149 conventional banks during 2006–2016. They posit that high competition supports the competition-stability hypothesis in Islamic banks, whereas the competition-fragility hypothesis is accepted in conventional banks.
Thus, fresh evidence is desired to bring clarity to the mixed view on competition and FD. Therefore, the following hypothesis is framed in its alternate form:
Hypothesis (H): competition influences the financial distress in the Indian banks while controlling for regulation, size, profitability and valuation.
4. Data and methodology
4.1. Data and sample
The current study uses the secondary data of a cross-section of 34 banks operating in India. The list of banks is presented in Table A1 in the appendix. The data has been fetched from the CMIE Prowess database. In order to provide fresh evidence, this paper has considered the observation period of four years (i.e., 2016–2019) as per the requirement of a sufficient number of observations for reliable results. The reason behind choosing these 34 banks is the availability of valid data simultaneously for all sample banks. These banks (Table A1) are also important as they cover the majority of financial operations in India. As Indian banks are going through various changes day by day, thus the said time-period (i.e., 2016–2020) is very important to catch the recent scenario of banks in India. The introduction of IBC Act 2016 also make this period essential for investigation on Indian banks to dig out the fresh evidence on banks’FD.
4.2. Variables
Portrays the variables used in this study. The current study has used the following variables:
Table 1. List of variables
Dependent variables: The dependent variables are three variants of Altman’s Z-score (i.e., ZS_O, ZS_WS, and ZS_E). These variables represent the bank’s financial distress (Altman, Citation1968; Pradhan, Citation2014). He initially developed the ZS_O. The other two Z-scores are an extension of the original Z-score. These z-scores are used interchangeably (Altman & Hotchkiss, Citation2010; Max & Altman, Citation2011; Pradhan, Citation2014). Therefore, all variants of the Z-score are taken up to ensure robust results by specifying three models. The following sub-section presents a detailed description of the assessment of these scores.
Explanatory variables: LI is Lerner’s index (Agaroki et al., Citation2011; Lerner, Citation1934), and it is used as the explanatory variable in this study. LI is the measure of the market competition of banks in India. The measurement technique of LI is explained in the following subsection.
Control Variables: The current study has the following four control variables. The first is the CAR (capital adequacy ratio) indicator of bank regulation. It is measured as the ratio of a bank’s capital to its risk-weighted assets (Moyer, Citation1990; Bateni et al., Citation2014). The second is the bank’s assets representing bank size. The third is the NIM (net interest margin), indicating profitability. It is calculated as the difference between investment returns and interest expenses, divided by the average earning asset (Nguyen, Citation2012; Hamadi and Awdeh, Citation2012). The fourth is market capitalization (mc), indicating the valuation, and is calculated by multiplying the number of a bank’s equity shares with the price of the share (Kumar and Shah, Citation2009; Narayan et al., Citation2011).
These control variables are used to have a good fit model and to examine the sole impact of competition on FD for consistent and reliable outcome. These variable are chosen as control variables because they might directly or indirectly associate to bank’s financial stability (Beaver, Citation1966; Ohlson, Citation1980).
4.3. Methodology applied for data analysis
4.3.1. Measurement of financial distress
Altman (Citation1968) has the credit first to develop a multivariate model based on multiple discriminant analysis (MDA) using five factors to identify the firm’s financial distress position (Pradhan, Citation2014). The output obtained from the model is called the Z-Score, which signals the position of the firm’s FD depending on the Z-Score value coming under a particular range. At the academic level, studies on the firm’s FD, Beaver (Citation1966), Altman (Citation1968) and Ohlson (Citation1980) contributed immensely in this area in the beginning. They have suggested several determinants to predict the firm’s FD. Beaver (Citation1966) advocated for the ratio of cash flow to debt as the key indicator of FD, while Ohlson (Citation1980) has used his discrete variables as a proxy for the likelihood of bankruptcy by applying the logit model.
Altman (Citation1968) used the five most significant variables in his model to predict a firm’s FD. He also found approximately 80–90% accuracy in his model to predict FD. Hence, Altman’s Z-score model is considered the most reliable model for predicting the firm’s FD (Altman, Citation2013). For developing the model, Altman (Citation1968) chose 66 firms for a period of 20 years (1946–1965), in which 33 firms were failed, and the rest of 33 were matching firms. He recognized 22 significant variables. Out of these 22 variables, the five most significant variables are chosen to specify the discriminant model using the MDA. Originally, Altman (Citation1968) developed the following model.
Where
T1 = WC/TA. WC is working capital, and TA is total assets. It is the evaluation of liquid assets as a part of company size.
T2 = RA/TA. RA is retained earnings. It evaluates a firm’s profitability indicating the company’s age and its earning capabilities.
T3 = EBIT/TA. It evaluates a firm’s operating efficiency indicating the viability of the firm in the long run.
T4 = market value of equity/book value of total liabilities. It incorporates market elements reflecting the security price variations.
T5 = sales/TA. It evaluates the total asset turnover varying from industry to industry.
Based on the value of the Z-score, the firms are categorized in the following way:
Z > 2.67—reflects a safe condition of the firm regarding FD.
1.81 < Z < 2.67—reflects a grey signal indicating the firm is prone to FD soon.
Z < 1.81—reflects distress signal indicating the firm is in FD.
Only manufacturing firms were taken into account in developing the original model.
Hence, Altman and Hotchkiss (Citation2010) put forth the model developed initially. They revised it, including non-manufacturing firms. The sale–asset ratio was removed from the model for probable industry effect.
The revised model for non-manufacturing firms is as follows:
In Equationequation 2(2)
(2) , the dependent and the independent variables have the same meaning as the originally developed model (EquationEq.1)
(1)
(1) .
Classification of firms:
Z > 2.6—reflects a safe condition of the firm regarding FD.
1.1 < Z < 2.6—reflects a grey signal indicating the firm is prone to be in FD soon.
Z < 1.1—reflects distress signal indicating the firm is in FD.
However, this model was not found very much suitable for developing markets. Therefore, Max and Altman (Citation2011) further improved the model for the developing markets by adding a constant value (i.e., +3.25) in the above model equation. This exercise was done to standardize the scores to a score of zero, which indicates the default (D) rated bond. However, the cut off range for the classification of the firms remains the same as for the non-manufacturing firm. Following is the Z-score function specification for developing markets is as follows:
In Equationequation 3(3)
(3) , the dependent and the independent variables have the same meaning as the originally developed model (EquationEq.1)
(1)
(1) .
4.3.2. Measurement of competition
This paper has used Lerner’s index for the assessment of bank competition.
Lerner (Citation1934), in his paper, discussed the attributes of market power and concluded that the level of market power or competition is measured as the percentage deviation of price from the marginal cost. He proposed the following model for measuring the market power:
Where P is price, and MC is the marginal cost calculated in Eq.5 by generating the derivative of cost concerning the bank’s output q. LI is Lerner’s index indicating the degree of market power, and its value ranges from 0 to 1. A value of 0 or a closer value signals a high degree of market power or low competition, while a value of 1 or closer to one shows a low degree of market power or high competition. LI may have a negative value in some exceptional cases, gesturing very high product substitutability or no profit maximization (Agaroki et al., Citation2011; Spierdijk & Zaouras, Citation2017).
The measurement of marginal cost is based on the following equation:
where C represents the bank’s total cost (i.e. total expenses). The q and d are the values of the total earning asset (indicating the bank’s output) and bank deposits, respectively. w represents the input prices, and e is the error term. Bars over the variables show deviations from their mean value. It is specified to reduce the issue of multicollinearity (Agaroki et al., Citation2011; Brissimis et al., Citation2008). Further, w includes three input prices, i.e. the fund cost (calculated as dividing interest expenses by total deposits), the labour cost (calculated as dividing personnel expenses by total assets, and the cost of capital (calculated as dividing the depreciation and other capital expenses by total fixed assets). is the coefficient for the variables, and j can take the value from 0 to 9 where
is the constant term. Subscript (it) indicates bank i and time t.
4.3.3. Model specification for data analysis
In order to consider both time-series and cross-sectional behaviour of the dataset, the study has performed the panel data analysis because it serves the purpose best for analyzing both time-series and cross-sectional effects (Baltagi, 2008; Hsiao, Citation2007).
For model specification, the fixed-effect model is suitable for the data analysis because the Hausman test rejects the null of no connectivity of individual effect with the explanatory variables (Please refer to for the tests; Bell et al., Citation2019; Setodji & Shwartz, Citation2013). The fixed-effect model specifications for coefficients estimation are as follows;
Table 2. Descriptive statistics and correlation matrix
Table 3. Static panel data model (fixed effect)
Where ZS_O, ZS_WS, and ZS_E are the Altman’s Z-score and are taken as dependent variables. LI is the explanatory variable representative of competition. Although, the Altman original Z-score model is developed considering manufacturing firms, however many studies like Carson (Citation1995) and Mamo (Citation2011) have used it for financial firms as well. Therefore, we have also incorporated original model to provide robust results observing three different models (using all three type Z-scores). CAR, L_ASS, NIM, and L_MC are control variables used to have a good fit model and to examine the sole impact of competition on FD for consistent and reliable outcome. These variable are chosen as control variables because they might directly or indirectly influence bank’s financial stability (Beaver, Citation1966; Ohlson, Citation1980). L_ASS and L_MC are noted as the log value of the bank’s assets and market capitalization. α is the sum of β0 + μi and μi the individual unobservable term in one-way model (fixed effect). is the conventional error term.
5. Results
This section discusses the results obtained from running the specified models. First, it describes the data set’s descriptive statistics and correlation matrix. Second, it discusses the outcomes of the models with diagnostics. Finally, it puts justification on the robustness of the outcome.
5.1. Descriptive statistics
The current paper presents the descriptive statistic in . The average values of ZS_O, ZS_WS, ZS_E are −0.505, −0.922, and −4.706, respectively. These values indicate that banks in India are going through financial distress. This result is validated in all three cases for the observation period. The mean value of LI is 0.247, indicating banks in India have good market power and less competition. The average CAR value is 13.218, which shows reasonable regulatory measures in Indian banks. The assets have an average value of INR 361759 Crore showing, an average large bank size in India. The mean values of NIM and MCAP are 2.611 and INR 139338.3, respectively showing the average profitability and valuation of banks in India. The smaller standard deviation values of z-scores, LI, CAR, and NIM, indicate their observation values are closer to their mean values. Assets and MCAP have large standard deviations showing observation values have a significant deviation from their mean values. Hence, banks vary in terms of its size (Assets) and value (MCAP).
The correlation matrix in shows the correlation between independent variables. It indicates that LI does not correlate with any control variable because no significant p-value is found at 0.05. The CAR has a significant and positive correlation (at 5% significance) with NIM and LMC, while LASS and NIM only show a significant and positive correlation (at 5% significance) with LMC. It is also evident that no significant correlation has value greater than 0.80. Therefore, multicollearity is not a hurdle for consistent analysis.
5.2. Main results
5.2.1. Static panel data analysis (Model1)
portrays the results of static panel data analysis. On observing model diagnostics, the Hausman test confirms the fixed effect model (Both fixed effect and random effect are significant; therefore, the Hausman test is applied). Wald test is exhibiting significant values; hence it rejects null of homoscedasticity. It means model specification has the problem of hetero. In addition to this, the Wooldridge test for auto-correlation also has a significant value of 5%. This result also confirms the presence of auto-correlation. Due to the presence of heteroscedasticity and auto-correlation, robust estimates are taken. The adjusted R-square is 0.2758. It means the input variables can explain 27.58% variations in output variables. Adjusted R-square also indicates that adding more input variables increases the model’s predictive ability.
The coefficient of explanatory variable LI is both positive and significant at 5% significant level (.1237) (for the combination of ZS_O and LI). This result implies that the higher market power or lower competitiveness results in the firm’s higher financial distress score. It means that a higher level of market competitiveness improves the firm’s financial position. Thus, the outcome of the analysis is quite interesting regarding the association between LI and FD. Other combinations (i.e., ZS_S and LI, and ZS_WS and LI) have also been considered as specified in Model 2 and 3 (see, EquationEq. 7(7)
(7) and Equation8
(8)
(8) ). Only NIM is a significant control variable among control variables in all three models (having a negative coefficient at p-value<0.05). This indicates that NIM may influence FD negatively. These models also follow the static model specifications. The following sub-section puts light on the results of models 2 and 3.
5.2.2. Panel data analysis for model2 and model3
The study has also set two more models using ZS_WS and ZS_E as the dependent variables to test the robustness of the results. In both models, the coefficients of LI are positive (.0221 and .1237) and significant at 5% (Please see ). It again implies that higher market power or low competition shoots up the financial distress score reduces the firm’s financial distress position (low FD score means more financial distress and vice versa). F-test has been performed to confirm the fixed effect, which has a significant value confirming the suitability of the fixed-effect model over pooled OLS. The adjusted R-square is considered as it improves. The adjusted R-square indicates that the input variables in each case can explain 25.40% and 27.58% variations in output variables. In the robustness test, we have also estimated fixed effect and robust estimate of standard error for the consistency. Due to similar reasons (as in model 1), autocorrelation and heteroscedasticity tests are not repeated.
Table 4. Robustness of the results
5.3. Diagnostic checking and endogeneity
The study considers LI an endogenous explanatory variable and ROA an instrument variable in the model. The Durnin-Wu-Hausman Test of Endogeneity is performed to check endogeneity due to LI. The value of Chi-Square (1) and F (1,129) are 2.5738 and 2.4884, respectively, and both values are insignificant. Hence, null of no Endogeneity cannot be rejected. It confirms that there is no endogeneity issue in model specification due to LI.
5.4. Robustness of the output
For checking the robustness of the output, this study has used the three variants of Altman’s Z-score. Hence, three models are specified (EquationEq. 6(6)
(6) ,Equation7
(7)
(7) and Equation8
(8)
(8) ). Each model exhibits similar results. Since all the models provide similar outcomes (indicating market power improves financial stability or lowers a firm’s FD), it confirms the robustness of the results.
6. Discussion
6.1. Discussion on hypotheses
The alternate hypothesis of the current paper is that competition influences the financial distress in the Indian banks while controlling for regulation, size, profitability, and valuation. The data analysis output cannot reject the hypothesis (H). All the models have the same outcome regarding the hypothesis. It means a high market power or low competition adds financial stability. It also implies that concentration banking or low competition is suitable for bank stability in India. The finding is on expected framing which supports the mostly accepted theory of competition-fragility in literature. Therefore, the outcome is realistic which indicates higher level of competition among banks results in higher FD in Indian banks.
6.2. Comparison of the findings with the literature
The findings of the current study favour some earlier sets of studies (Alam et al., Citation2019a; Beck et al., Citation2013; A. Berger et al., Citation2009; Gyimah et al., Citation2021), propounding that competition is determinantal for financial stability and increases the risk. These studies are in support with competition-fragility theory which is highly accepted in existing literature. However, there exist some other studies in favour of competition-stability theory, such as Saha and Dutta (Citation2020), Obembe and Soetan (Citation2015), and Noman et al. (Citation2018), which are not in favour of the current study. These studies advocate that across all types of firms, including banks, the competition increases the financial stability, and it is helpful in the growth of the firms. Moreover, the outcome of the current study also contradicts the findings of Yazdanfar and Öhman (Citation2020), which argue that competition has no link with the firm’s financial stability.
6.3. Contribution
A vast volume of literature demonstrates the linkage between the competition and the firm’s financial health. However, there is no consensus regarding the effect of competition on a firm’s financial distress (Majeed et al., Citation2018; Zaki et al., Citation2011). Given this, the present study contributes to the existing body of knowledge by providing fresh and robust evidence on the competition level of Indian banks, their distress position, and the connectivity of market power and financial stability. The findings reveal that bank competition and FD are significantly associated. It further indicates that high market power or a low level of competition improves the financial stability of banks in India. Moreover, no other study has been observed to use all the three variants of Altman’s z-score to provide robustness and reliability in results. Therefore, the study has a significant contribution and a novelty in its approach.
6.4. Policy level and managerial implication
FD is an undesirable phenomenon that disturbs the firm and influences all society (Barney & Harrison, Citation2020; Freeman et al., Citation2010). Therefore, the timely recognition of such a challenging situation and its influencing factors is essential (Habib et al., Citation2020; Lizal, Citation2002). The present study recognizes that market competition is an essential determinant of a bank’s financial stability. The study’s findings imply that the market power improves financial stability, or the competition increases the FD. It means the banks operating with more market power can command the pricing to improve profits. Hence, the market gains benefit in enhancing financial stability and reducing the risk of bank failure.
The findings have significant implications in Indian banking as it has witnessed many reforms since its inception. The introduction of (IBC, Citation2016) and the current bank failures worldwide including India draw attention of all stakeholders to keep a bird eye view on financial stability. There might be several determinants which can influence a bank’s financial stability. Nevertheless, there is no empirical corroboration regarding bank competition for financial distress. Thus, the study’s findings provide empirical evidence that higher competition leads to higher FD in banks. The findings supports the competition-fragility theory. The current findings have noticeable implications for the policymakers to introduce such bank policies, which can improve the market power of the bank and reduce the overall level of competition to combat FD of banks. Furthermore, all stakeholders should monitor the management and ensure such bank’s managerial activities, which could have command over pricing to improve profits, enhance market power, or lower competition. This way, the present study provides managerial implications as well.
7. Conclusion, limitation, and future scope
In the present study, the authors have investigated the influence of competition on the bank’s financial stability (as FD) in India. Three models are developed to test the influence using panel data of 34 banks. These models are based on Altman’s Z-scores and LI while controlling the bank regulation, size, profitability, and value. The model outcomes are similar in all three cases, indicating that market competition positively relates to the firm’s FD. It implies that a lower degree of competitiveness or a higher degree of banks’ market power adds up the financial stability by reducing the distress. Hence, the study’s finding indicates that competition among banks or market power is an important cause of banks’ FD condition. It delivers notable implications for managers to sustain a strong position in a competition or have a grip of market power. Furthermore, policymakers need to keep competition measures essential for banks FD position. Hence, they should draft policies or regulations that ensure a healthy competitive environment. There exists two fundamental theories on competition and distress (1. Competition-fragility theory and Competition-stability theory). Hence, there is no unanimity for the association of competition and FD in banks. The present study contributes to the existing body of knowledge by providing fresh and robust evidence on the competition level of Indian banks, their distress position, and the connectivity of market power and financial stability.
The current paper has its scope limited to the banks operating in India. Therefore, the findings of this study should be carefully seen. However, the authors believe that the present paper will help provide insights for bank’s operating in other emerging economies of similar kind. The coverage of sample size is limited to certain number of banks. Furthermore, only one technique for competition assessment has been undertaken. Other techniques can also be employed for more robustness in the outcome of competition.
In consequence of the limitations, the present study provides clues for future studies on the topic. The current paper can be replicated for the investigation in other developed or developing economies. If possible the larger sample size should be incorporated in future studies. The other techniques for FD and competition assessment may be used. In addition to this, these techniques can be combined to design different models for examining and comparing the outcomes. For future studies, the introduction of moderating variable can also be undertaken to provide interesting outcomes.
Disclosure statement
No potential conflict of interest was reported by the author(s).
Additional information
Funding
References
- Agarwal, A. K., Das, A., Jacob, J., & Mohapatra, S. (2020). Introduction to the special issue on financial distress, bankruptcy, and corporate finance. Vikalpa: The Journal for Decision Makers, 45(2), 61–20. https://doi.org/10.1177/0256090920953995
- Agoraki, M. E. K., Delis, M. D., & Pasiouras, F. (2011). Regulations, competition and bank risk-taking in transition countries. Journal of Financial Stability, 7(1), 38–48. https://doi.org/10.1016/j.jfs.2009.08.002
- Al‐Tamimi, H. A. H. (2012). The effects of corporate governance on performance and financial distress: The experience of UAE national banks. Journal of Financial Regulation and Compliance, 20(2), 169–181. https://doi.org/10.1108/13581981211218315
- Alam, N., Hamid, B. A., & Tan, D. T. (2019a). Does competition make banks riskier in dual banking system. Borsa Istanbul Review, 19(1), S34–S43. https://doi.org/10.1016/j.bir.2018.09.002
- Alam, N., Hamid, B. A., & Tan, D. T. (2019b). Does competition make banks riskier in dual banking system? Borsa Istanbul Review, 19(Suppl. 1), 34–43 https://doi.org/10.1016/j.bir.2018.09.002.
- Alegria, C., & Schaeck, K. (2008). On measuring concentration in banking systems. Finance Research Letters, 5(1), 59–67. https://doi.org/10.1016/j.frl.2007.12.001
- Allen, B., Chan, K. K., Milne, A., & Thomas, S. (2012). Basel III: Is the cure worse than the disease? International Review of Financial Analysis, 25 (C) , 159–166. https://doi.org/10.1016/j.irfa.2012.08.004
- Altman, E. I. (1968). Financial ratios, discriminant analysis and the prediction of corporate bankruptcy. The Journal of Finance, 23(4), 589–609.
- Altman, E.I. (2013). Predicting financial distress of companies: revisiting the Z-score and ZETA® models. In Handbook of research methods and applications in empirical finance. Edward Elgar Publishing. available at https://www.elgaronline.com/view/edcoll/9780857936080/97 https://www.elgaronline.com/view/edcoll/9780857936080/9780857936080.00027.xml accessed during July 2021
- Altman, E. I., & Hotchkiss, E. (2010). Corporate financial distress and bankruptcy: Predict and avoid bankruptcy, analyze and invest in distressed debt (Vol. 289). John Wiley & Sons.
- Amendola, A., Restaino, M., & Sensini, L. (2015). An analysis of the determinants of financial distress in Italy: A competing risks approach. International Review of Economics & Finance, 37(C), 33–41. https://doi.org/10.1016/j.iref.2014.10.012
- Baimwera, B., & Muriuki, A. M. (2014). Analysis of corporate financial distress determinants: A survey of non-financial firms listed in the NSE. International Journal of Current Business and Social Sciences, 1(2), 58–80 https://citeseerx.ist.psu.edu/viewdoc/download?doi=10.1.1.1077.7173&rep=rep1&type=pdf.
- Bakar, S., & Noordin, B. A. A. (2021). The dynamic impacts of financial determinants and ownership concentration toward firm survival in Malaysia. In W. A. Barnett & B. S. Sergi (Eds.), Recent developments in Asian economics international symposia in economic theory and econometrics. Emerald Publishing Limited, 397. https://doi.org/10.1108/S1571-038620210000028023. .
- Barney, J. B., & Harrison, J. S. (2020). Stakeholder theory at the crossroads. Business and Society, 59(2), 203–212. https://doi.org/10.1177/0007650318796792
- Bateni, L., Vakilifard, H., & Asghari, F. (2014). The influential factors on capital adequacy ratio in Iranian banks. International Journal of Economics and Finance, 6(11), 108–116. https://doi.org/10.5539/ijef.v6n11p108
- Beaver, W. H. (1966). Financial ratios as predictors of failure. Journal of Accounting Research, 4 (1966) , 71–111. https://doi.org/10.2307/2490171
- Beck, T., De Jonghe, O., & Schepens, G. (2013). Bank competition and stability: Cross-country heterogeneity. Journal of Financial Intermediation, 22(2), 218–244. https://doi.org/10.1016/j.jfi.2012.07.001
- Beck, T., Demirgüç-Kunt, A., & Levine, R. (2006). Bank concentration, competition, and crises: First results. Journal of Banking & Finance, 30(5), 1581–1603. https://doi.org/10.1016/j.jbankfin.2005.05.010
- Bell, A., Fairbrother, M., & Jones, K. (2019). Fixed and random effects models: Making an informed choice. Quality & Quantity, 53(2), 1051–1074. https://doi.org/10.1007/s11135-018-0802-x
- Berger, A. N., Demirguc-Kunt, A., Levine, R., & Haubrich, J. G. (2004). Bank concentration and competition: An evolution in the making. Journal of Money, Credit, and Banking, 36(3), 433–451. https://doi.org/10.1353/mcb.2004.0040
- Berger, A., Klapper, L., & Turk-Ariss, R. (2009). Bank competition and financial stability. Journal of Financial Services Research, 35(2), 99–118. https://doi.org/10.1007/s10693-008-0050-7
- Boyd, J. H., & De Nicolo, G. (2005). The theory of bank risk taking and competition revisited. The Journal of Finance, 60(3), 1329–1343. https://doi.org/10.1111/j.1540-6261.2005.00763.x
- Brissimis, S. N., Delis, M. D., & Papanikolaou, N. I. (2008). Exploring the nexus between banking sector reform and performance: Evidence from newly acceded EU countries. Journal of Banking & Finance, 32(12), 2674–2683. https://doi.org/10.1016/j.jbankfin.2008.07.002
- Camacho‐Miñano, M. D. M., Muñoz-Izquierdo, N., Pincus, M., & Wellmeyer, P. (2020). Are key audit matter disclosures useful in assessing financial distress? Available at: https://www.researchgate.net/profile/Maria-Del-Mar-Camacho-Minano/publication/349168773_Are_Key_Audit_Matter_Disclosures_Useful_in_Assessing_Financial_Distress/links/6088194e2fb9097c0c12fb46/Are-Key-Audit-Matter-Disclosures-Useful-in-Assessing-Financial-Distress.pdf, Accessed on June 2021.
- Carson, M. J. (1995). Financial distress in the life insurance industry: An empirical examination. Illinois University, 1211–1240 https://www.actuaries.org/AFIR/colloquia/Orlando/Carson.pdf.
- Carter, D. A., & Mcnulty, J. E. (2005). Deregulation, technological change, and the business-lending performance of large and small banks. Journal of Banking & Finance, 29(5), 1113–1130. https://doi.org/10.1016/j.jbankfin.2004.05.033
- Cetorelli, N. (2001). Competition among banks: Good or bad? Economic Perspectives-Federal Reserve Bank of Chicago, 25(2), 38–48 https://fraser.stlouisfed.org/files/docs/historical/frbchi/economicperspectives/frbchi_econper_2001q2.pdf#page=40.
- Cipollini, A., & Fiordelisi, F. (2012). Economic value, competition and financial distress in the European banking system. Journal of Banking & Finance, 36(11), 3101–3109. https://doi.org/10.1016/j.jbankfin.2012.07.014
- Dávila, E., & Walther, A. (2020). Does size matter? Bailouts with large and small banks. Journal of Financial Economics, 136(1), 1–22. https://doi.org/10.1016/j.jfineco.2019.09.005
- Deb, S., & Dube, I. (2020). Insolvency and Bankruptcy Code 2016: Revisiting with market reality. International Journal of Law and Management, 63(1), 125–146. https://doi.org/10.1108/IJLMA-05-2020-0133
- Deidda, L., & Fattouh, B. (2005). Concentration in the banking industry and economic growth. Macroeconomic Dynamics, 9(2), 198–219. https://doi.org/10.1017/S1365100505040174
- Demirgüç-Kunt, A., & Detragiache, E. (2011). Basel core principles and bank soundness: Does compliance matter? Journal of Financial Stability, 7(4), 179–190. https://doi.org/10.1016/j.jfs.2010.03.003
- Elloumi, F., & Gueyié, J.-P. (2001). Financial distress and corporate governance: An empirical analysis. Corporate Governance: The Int J of Effective Board Performance, 1(1), 15–23. https://doi.org/10.1108/14720700110389548
- Farooq, U., Jibran Qamar, M. A., & Haque, A. (2018). A three-stage dynamic model of financial distress. Managerial Finance, 44(9), 1101–1116. https://doi.org/10.1108/MF-07-2017-0244
- Freeman, R. E., Harrison, J. S., Wicks, A. C., Parmar, B. L., & De Colle, S. (2010). Stakeholder theory: The state of the art. Cambridge University Press.
- Garlappi, L., & Yan, H. (2011). Financial distress and the cross‐section of equity returns. The Journal of Finance, 66(3), 789–822. https://doi.org/10.1111/j.1540-6261.2011.01652.x
- Gebreslassie, E. (2015). Determinants of financial distress conditions of commercial banks in Ethiopia: A case study of selected private commercial banks. Journal of Poverty, Investment and Development, 13(2422), 59–74 https://core.ac.uk/download/pdf/234695253.pdf.
- Gordon, M. J. (1971). Towards a theory of financial distress. The Journal of Finance, 26(2), 347–356. https://doi.org/10.1111/j.1540-6261.1971.tb00902.x
- Gupta, A. (2018). Insolvency and bankruptcy code, 2016: A paradigm shift within insolvency laws in India. The Copenhagen Journal of Asian Studies, 36(2), 75–99. https://doi.org/10.22439/cjas.v36i2.5650
- Gyimah, D., Siganos, A., & Veld, C. (2021). Effects of financial constraints and product market competition on share repurchases. Journal of International Financial Markets, Institutions and Money, 74 (C) , 101392. https://doi.org/10.1016/j.intfin.2021.101392
- Habib, A., Costa, M. D., Huang, H. J., Bhuiyan, M., Uddin, B., & Sun, L. (2020). Determinants and consequences of financial distress: Review of the empirical literature. Accounting and Finance, 60(S1), 1023–1075. https://doi.org/10.1111/acfi.12400
- Halteh, K., Kumar, K., & Gepp, A. (2018). Financial distress prediction of Islamic banks using tree-based stochastic techniques. Managerial Finance, 44(6), 759–773. https://doi.org/10.1108/MF-12-2016-0372
- Hamadi, H., & Awdeh, A. (2012). The determinants of bank net interest margin: Evidence from the Lebanese banking sector. Journal of Money, Investment and Banking, 23(3), 85–98 https://www.researchgate.net/profile/Ali-Awdeh/publication/325997470_The_Determinants_of_Bank_Net_Interest_Margin_Evidence_from_the_Lebanese_Banking_Sector/links/5b326c580f7e9b0df5ccac1b/The-Determinants-of-Bank-Net-Interest-Margin-Evidence-from-the-Lebanese-Banking-Sector.pdf.
- Hanggraeni, D. (2018). Competition, bank fragility, and financial crisis. Banks and Bank Systems, 13(1), 22–36. https://doi.org/10.21511/bbs.13(1).2018.03
- Hsiao, C. (2007). Panel data analysis—advantages and challenges. Test, 16(1), 1–22. https://doi.org/10.1007/s11749-007-0046-x
- IBC (2016).Insolvency and bankruptcy code act 2016, available at: https://www.mca.gov.in/Ministry/pdf/TheInsolvencyandBankruptcyofIndia.pdf
- IBC (2020). Implementation of insolvency and bankruptcy of code -pitfalls and solutions. 32nd report of standing committee of finance of 7th Lok Shabha, available at: https://www.ibbi.gov.in/uploads/whatsnew/fc8fd95f0816acc5b6ab9e64c0a892ac.pdf
- Ikpesu, F. (2019). Firm specific determinants of financial distress: Empirical evidence from Nigeria. Journal of Accounting and Taxation, 11(3), 49–56. https://doi.org/10.5897/JAT2019.0333
- Jaafar, M. N., Muhamat, A. A., Alwi, S. F. S., & Karim, N. A. (2018). Determinants of financial distress among the companies practise note 17 listed in Bursa Malaysia. INTERNATIONAL JOURNAL OF ACADEMIC RESEARCH IN BUSINESS AND SOCIAL SCIENCES, 8(11), 798–809. https://doi.org/10.6007/IJARBSS/v8-i11/4956
- Jahur, M. S., & Quadir, S. N. (2012). Financial distress in small and medium enterprises (SMES) of Bangladesh: Determinants and remedial measures. Economia. Seria Management, 15(1), 46–61 https://d1wqtxts1xzle7.cloudfront.net/81631854/4-with-cover-page-v2.pdf?Expires=1662643041&Signature=TFzHqQB-gUeGGF2XfcoftHVKEbNnKfoV-xCHw71rZYRtB7KuEaP8goMH8noiL9qAukHSmAJ9xg-0K3uys9EbAf9ciTS9u~jkEsM4WiPbn3ofe~h0byqIGgGLxZNzpn7F1tyhDiAOOUOiaTqymzWWDMFpZpuqPxXUmUoYPSlBggOQfoEuroTlEoyBAd9KzHu9qtsJCNuKvM01SISRJ8V7IPRd4cdXEgjfoEp3NcA5T~Aof5FnRVnSUcIbLI9N1pjX-tkWOfbbLc1A9t0ChsTYm9KqHZmf0SMym5r7s1piGUTQ13ZyRn-5b2EzrU2YIuj0jbK4lQ9PpvHH1BSCgVJWAQ__&Key-Pair-Id=APKAJLOHF5GGSLRBV4ZA.
- Jain, A., & Jain, P. (2020). The merger of banks in India: Boon or bane for the Indian economy. European Journal of Interdisciplinary Research, 1(1), 46–51 http://dx.doi.org/10.5281/zenodo.4006607.
- Jasrotia, S. S., & Agarwal, T. (2021). Consolidation of Indian PSU banks and the way forward. Journal of Public Affairs, 21(1), e2133. https://doi.org/10.1002/pa.2133
- Jayadev, M., Singh, H., & Kumar, P. (2017). Small finance banks: Challenges. IIMB Management Review, 29(4), 311–325. https://doi.org/10.1016/j.iimb.2017.10.001
- Joseph, A. L., & Prakash, M. (2014). A study on analyzing the trend of NPA level in private sector banks and public sector banks. International Journal of Scientific and Research Publications, 4(7), 1–9. https://citeseerx.ist.psu.edu/viewdoc/download?doi=10.1.1.571.9632&rep=rep1&type=pdf.
- Kabir, A., & Dey, S. (2012). Performance analysis through CAMEL rating: A comparative study of selected private commercial banks in Bangladesh. Journal of Politics and Governance, 1(2and3), 16–25. https://citeseerx.ist.psu.edu/viewdoc/download?doi=10.1.1.703.8799&rep=rep1&type=pdf#page=16.
- Kishore, K. (2015). Small finance banks: New category of differentiated banks. FIIB Business Review, 4(4), 13–20. https://doi.org/10.1177/2F2455265820150402.
- Kristanti, F. T., Rahayu, S., & Huda, A. N. (2016). The determinant of financial distress on Indonesian family firm. Procedia-Social and Behavioral Sciences, 219 (2016) , 440–447. https://doi.org/10.1016/j.sbspro.2016.05.018
- Kumar, V., & Shah, D. (2009). Expanding the role of marketing: From customer equity to market capitalization. Journal of Marketing, 73(6), 119–136.
- Lee, T. S., & Yeh, Y. H. (2004). Corporate governance and financial distress: Evidence from Taiwan. Corporate Governance: An International Review, 12(3), 378–388. https://doi.org/10.1111/j.1467-8683.2004.00379.x
- Lerner, A. P. (1934). The concept of monopoly and the measurement of monopoly power. The Review of Economic Studies, 1(3), 157–175. https://doi.org/10.2307/2967480
- Leroy, A., & Lucotte, Y. (2017). Is there a competition-stability trade-off in European banking? Journal of International Financial Markets, Institutions and Money, 46(1), 199–215. https://doi.org/10.1016/j.intfin.2016.08.009
- Lizal, L. (2002). Determinants of financial distress: What drives bankruptcy in a transition economy? The Czech Republic case. The Czech Republic Case (January 2002), Phare ACE grant, available at: http://intranet.ef.uni-lj.si/apps/95_AdministracijaRCEF/seminarji/datoteke/lizalfin4ljublj.pdf
- Madrid-Guijarro, A., García-Pérez-De-Lema, D., & Van Auken, H. (2011). An analysis of non-financial factors associated with financial distress. Entrepreneurship and Regional Development, 23(3–4), 159–186. https://doi.org/10.1080/08985620903233911
- Majeed, M. A., Yan, C., & Tauni, M. Z. (2018). How does competition shape managerial decisions? Product market competition and financial statement comparability. Management Decision, 56(11), 2437–2471. https://doi.org/10.1108/MD-04-2017-0319
- Maji, S. G., & Hazarika, P. (2018). Capital regulation, competition and risk-taking behavior of Indian banks in a simultaneous approach. Managerial Finance, 44(4), 459–477. https://doi.org/10.1108/MF-09-2017-0340
- Mamo, A. Q. (2011). Applicability of Altman (1968) model in predicting financial distress of commercial banks in Kenya ( Doctoral dissertation). School of Business, University of Nairobi.
- Max, L. H., & Altman. (2011). PREDICTING FINANCIAL DISTRESS OF COMPANIES: REVISITING THE Z-SCORE AND ZETA® MODELS. New York University.
- Misra, B. S. (2006). The Performance of regional rural banks (RRBs) in India: has past anything to suggest for future. Reserve Bank of India Occasional Papers, 27(1), 89–118 https://www.researchgate.net/profile/Biswa-Misra/publication/23777397_The_Performance_of_Regional_Rural_Banks_RRBs_in_IndiaHas_Past_Anything_to_Suggest_for_Future/links/547766500cf205d1687a7dac/The-Performance-of-Regional-Rural-Banks-RRBs-in-IndiaHas-Past-Anything-to-Suggest-for-Future.pdf.
- Moosa, I. A. (2010). Basel II as a casualty of the global financial crisis. Journal of Banking Regulation, 11(2), 95–114. https://doi.org/10.1057/jbr.2010.2
- Moyer, S. E. (1990). Capital adequacy ratio regulations and accounting choices in commercial banks. Journal of Accounting and Economics, 13(2), 123–154.
- Narayan, P. K., Mishra, S., & Narayan, S. (2011). Do market capitalization and stocks traded converge? New global evidence. Journal of Banking & Finance, 35(10), 2771–2781. https://doi.org/10.1016/j.jbankfin.2011.03.010
- Narula, S., & Singla, M. (2014). Empirical study on non-performing assets of bank. International Journal of Advance Research in Computer Science and Management Studies, 2(1), 194–199 https://d1wqtxts1xzle7.cloudfront.net/35504237/v2i1-0032-with-cover-page-v2.pdf?Expires=1662644463&Signature=eOSHpt~VkkeKdFU-33IfgQR-uDdaGT56~2zibz4db~IhjEWvO~MH5GRRIsdzEohPAOggj2xiza1cdWIF8CRuofud52qoviki6yZhwilbW3aVQY-Xu-1UiMGyhxNuca8yHfuLZxvmXPNA1W12DkpPJYMGTF81wzpTDa~N9itgLcXaoLUH~TuxkWKgkeRcF5qwNtYDpAgy~NHozLjCZmpzMWU1gx8VC~5apl0joRnns9TV610UUhmH-cfDX11~QjDwSynjzKsdW61SNJBHFazF2NODVkKmwQfXQOSFUXVEIfAT9Sd-bi-FuBtbN6dVxCTBfoFJ6-6D92yZY6qh9dBB0Q__&Key-Pair-Id=APKAJLOHF5GGSLRBV4ZA.
- Nguyen, J. (2012). The relationship between net interest margin and noninterest income using a system estimation approach. Journal of Banking & Finance, 36(9), 2429–2437. https://doi.org/10.1016/j.jbankfin.2012.04.017
- Nikolova, L. V., Rodionov, D. G., & Mottaeva, A. B. (2016). Securitization of bank assets as a source of financing the innovation activity. International Journal of Economics and Financial Issues, 6(S3), 1–7 https://dergipark.org.tr/en/pub/ijefi/issue/31980/352636.
- Noman, A. H. M., Gee, C. S., & Isa, C. R. (2018). Does bank regulation matter on the relationship between competition and financial stability? Evidence from Southeast Asian countries. Pacific-Basin Finance Journal, 48(C), 144–161. https://doi.org/10.1016/j.pacfin.2018.02.001
- Obembe, O. B., & Soetan, R. O. (2015). Competition, corporate governance and corporate performance: Substitutes or complements? Empirical evidence from Nigeria. African Journal of Economic and Management Studies, 6(3), 251–271. https://doi.org/10.1108/AJEMS-02-2012-0007
- Ohlson, J. (1980). FINANCIAL RATIOS AND THE PROBABILISTIC PREDICTION OF BANKRUPTCY. Journal of Accounting Research, 18(1), 109–131. https://doi.org/10.2307/2490395
- Oliver, W. (2017). INDIA’S PUBLIC-SECTORBANKING CRISIS Oliver Wyman. 8(9.21): 1–14. https://www.oliverwyman.com/content/dam/oliver-wyman/v2/publications/2017/aug/Indias_Public-Sector_Banking_Crisis_Whither_The_Withering_Banks.pdf
- Pakravan, K. (2014). Bank capital: The case against Basel. Journal of Financial Regulation and Compliance, 22(3), 208–218. https://doi.org/10.1108/JFRC-09-2013-0030
- Paule-Vianez, J., Gutiérrez-Fernández, M., & Coca-Pérez, J. L. (2019). Prediction of financial distress in the Spanish banking system: An application using artificial neural networks. Applied Economic Analysis, 28(82), 69–87. https://doi.org/10.1108/AEA-10-2019-0039
- Pradhan, R. (2014). Z score estimation for Indian banking sector. International Journal of Trade, Economics and Finance, 5(6), 516. https://doi.org/10.7763/IJTEF.2014.V5.425
- Pramudena, S. M. (2017). The impact of good corporate governance on financial distress in the consumer goods sector. J. Fin. Bank. Review, 2(4), 46–55. https://sgsrjournals.co.in/paperdownload/3.pdf)
- Prasad, K. V. N., & Ravinder, G. (2012). A CAMEL model analysis of nationalized Banks in India. Journal of Venture Capital & Financial Services, 6(1), 5–15 https://sgsrjournals.co.in/paperdownload/3.pdf.
- Prasad, A., & Reddy, C. P. (2009). Global financial crisis and its impact on India. Journal of Social Sciences, 21(1), 1–5. https://doi.org/10.1080/09718923.2009.11892744
- Pryor, C. S., & Carg, R. (2020). Differential treatment among creditors under India’s Insolvency and Bankruptcy Code, 2016: Issues and solutions. The American Bankruptcy Law Journal, 94(1), 123–153. https://ssrn.com/abstract=3676489.
- Rastogi, S. (2014). The financial crisis of 2008 and stock market volatility–analysis and impact on emerging economies pre and post crisis. Afro-Asian Journal of Finance and Accounting, 4(4), 443–459. https://doi.org/10.1504/AAJFA.2014.067017
- RBI (2021). available at: https://rbi.org.in/Scripts/PublicationsView.aspx?id=20270
- Reddy, S. (2018). Announcement of payment banks and stock performance of commercial banks in India. Journal of Internet Banking and Commerce, 23(1), 1–12. https://www.icommercecentral.com/open-access/announcement-of-payment-banks-and-stock-performance-of-commercial-banks-in-india.php?aid=86885&view=mobile.
- Reinhart, C. M., & Rogoff, K. S. (2011). From financial crash to debt crisis. American Economic Review, 101(5), 1676–1706. https://doi.org/10.1257/aer.101.5.1676
- Saha, M., & Dutta, K. D. (2020). Nexus of financial inclusion, competition, concentration and financial stability: Cross-country empirical evidence. Competitiveness Review: An International Business Journal, 31(4), 669–692. https://doi.org/10.1108/CR-12-2019-0136
- Samitas, A., & Polyzos, S. (2015). To Basel or not to Basel? Banking crises and contagion. Journal of Financial Regulation and Compliance, 23(3), 298–318. https://doi.org/10.1108/JFRC-11-2014-0045
- Schwerter, S. (2011). Basel III’s ability to mitigate systemic risk. Journal of Financial Regulation and Compliance, 19(4), 337–354. https://doi.org/10.1108/13581981111182947
- Sehgal, S., Mishra, R. K., Deisting, F., & Vashisht, R. (2021). On the determinants and prediction of corporate financial distress in India. Managerial Finance, 47(10), 1428–1447. https://doi.org/10.1108/MF-06-2020-0332
- Setodji, C. M., & Shwartz, M. (2013). Fixed-effect or random-effect models: What are the key inference issues? Medical Care, 51(1), 25–27. https://doi.org/10.1097/MLR.0b013e31827a8bb0
- Shajahan, K. (1998). Non-performing assets of banks: Have they really declined? And on whose account? Economic and Political Weekly, 33(12), 671–674. https://www.jstor.org/stable/4406554.
- Singh, V. R. (2016). A study of non-performing assets of commercial banks and it’s recovery in India. Annual Research Journal of SCMS, Pune, 4 (1), 110–125. https://www.scmspune.ac.in/chapter/2016/Chapter%209.pdf.
- Spierdijk, L., & Zaouras, M. (2017). The Lerner index and revenue maximization. Applied Economics Letters, 24(15), 1075–1079. https://doi.org/10.1080/13504851.2016.1254333
- Wanderi, R. G. (2016). Influence of corporate governance practice on financial distress among commercial Banks in Kenya. University of Nairobi. availabe at http://erepository.uonbi.ac.ke/bitstream/handle/11295/100230/Wanderi_Influence%20of%20Corporate%20Governance%20Practice%20on%20Financial%20Distress%20Among%20Commercial%20Banks%20in%20Kenya.pdf?sequence=1&isAllowed=y
- Wanke, P., Barros, C. P., & Faria, J. R. (2015). Financial distress drivers in Brazilian banks: A dynamic slacks approach. European Journal of Operational Research, 240(1), 258–268. https://doi.org/10.1016/j.ejor.2014.06.044
- Whitaker, R. B. (1999). The early stages of financial distress. Journal of Economics and Finance, 23(2), 123–132. https://doi.org/10.1007/BF02745946
- World Bank (2019). available at: https://data.worldbank.org/indicator/FB.AST.NPER.ZS?end=2019&locations=IN&start=2008&view=chart
- Yazdanfar, D., & Öhman, P. (2020). Financial distress determinants among SMEs: Empirical evidence from Sweden. Journal of Economic Studies, 47(3), 547–560. https://doi.org/10.1108/JES-01-2019-0030
- Yousaf, U. B., Jebran, K., & Wang, M. (2021). Can board diversity predict the risk of financial distress? Corporate Governance: The International Journal of Business in Society, 21(4), 663–684. https://doi.org/10.1108/CG-06-2020-0252
- Zaki, E., Bah, R., & Rao, A. (2011). Assessing probabilities of financial distress of banks in UAE. International Journal of Managerial Finance, 7(3), 304–320. https://doi.org/10.1108/17439131111144487
- Zhang, Z., Xie, L., Lu, X., & Zhang, Z. (2016). Determinants of financial distress in large financial institutions: Evidence from US bank holding companies. Contemporary Economic Policy, 34(2), 250–267. https://doi.org/10.1111/coep.12105