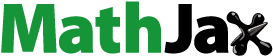
Abstract
This study aims to investigate the existence and nature of herding behavior in the banking sector in Bangladesh. It uses daily closing prices of 30 listed commercial banks from January 2007 to December 2018 to detect herding. This study confirms the presence of herding in the banking industry for the whole study period. Further, it finds herding in the banking sector during the domestic financial crisis and no herding in the Global financial crisis. By separating the study period into a rising market and a falling market condition, this paper finds asymmetry in herding behavior in the banking sector. This phenomenon is further confirmed when the extreme market condition of the bull and bear phase is used. Investors in the banking industry flock together ignoring any private information to reap the market return at the bull period while they avoid the crowd during the bear phase.
1. Introduction
In asset pricing literature in finance, herding refers to an investor’s behavior to pursue the activity of other investors (Javaira & Hassan, Citation2015; Spyrou, Citation2013) which causes a regular departure from the true value and price of an asset. The magnitude and consistency of herding behavior direct the market towards unexpected upward movements and asset bubbles and abrupt falls. When conventional theories couldn’t answer the irregularities of asset pricing, the herding behavior provides the possible explanation for the asset market volatility (Tan et al., Citation2008). Recall Keynes (Citation1936) in understanding the investors’ decision-making based on psychological factors mentioned as “beauty contest” and “animal spirit”. At times of fear and uncertainty, people tend to make impulsive and sentimental decisions and in most cases, these are irrational (Keynes, Citation1936). The greater the information asymmetry the larger the uncertainty and people take decision-based on short-run asset valuation and disregards fundamentals (Sewell, Citation2011) that intensify asset bubble and eventually bubble burst (Olsen, Citation2011). Thus, herd behavior becomes a major force of market volatility (Bekiros et al., Citation2017).
Earlier studies have conflict in explaining herd behavior theoretically and empirically. Demirer et al. (Citation2010) explain three theories, i.e. Psychological herding, information-related herding, and agency herding, for herd behavior from prior studies. Psychological herding takes place due to the substantial number of non-sophisticated traders and their optimism where pessimistic investors become optimistic and feel secure by following the crowd (Devenow & Welch, Citation1996; Lux, Citation1995). Information related herding works in two ways where the informed investors take their actions based on the information that is not available to others (Avery & Zemsky, Citation1998; Shleifer & Summers, Citation1990), and the behavior of informed investors signals market movements to the individual investors (Banerjee, Citation1992; Shiller, Citation2002) which eventually saves cost and time to make an investment decision. Another explanation arises from agency theory where agents tend to follow others disregarding private information so that their market reputation sustains (Scharfstein & Stein, Citation1990). Thus, there exist both rational and irrational theories of herd behavior.
Javaira and Hassan (Citation2015) and Sharma (Citation2018) summarize existing literature on herding from two different viewpoints, one is individual stock herding and the other is market-wise herding. Particular stock-wise herding implies investor behavior focusing on individual securities without thinking of other stocks with the same characteristics. This type of herding arises because of the informational surge, reputational case, institutional investment, compensational difficulty, psychological and communication setbacks of the investors (Banerjee, Citation1992;Bikhchandani et al., Citation1992; Brennan, Citation1993; Conlisk, Citation1980; Lakonishok et al., Citation1992; Lux, Citation1995; Nofsinger & Sias, Citation1999; Pingle, Citation1995; Roll, Citation1992; Scharfstein & Stein, Citation1990; Shiller & Pound, Citation1986; Trueman, Citation1994; Wermers, Citation1999; Zwiebel, Citation1995). Unlike herding in a particular stock, market-wise herding means investors’ tendency to follow the market movements disregarding fundamentals (Chang et al., Citation2000; Christie & Huang, Citation1995). Most of the empirical researches are based on market-wise herding and finds the mixed result of positive responses (Chang et al., Citation2000; Chiang & Zheng, Citation2010; Lindhe, Citation2012; Prosad et al., Citation2012), negative responses (Demirer et al., Citation2010; Garg & Jindal, Citation2014; Lakonishok et al., Citation1992) of herding behavior.
Prior studies (Cakan & Balagyozyan, Citation2014; Chang et al., Citation2000; Javaira & Hassan, Citation2015) already reasoned the cause and implications of the higher level of herding in the emerging market compared to developed market. Emerging markets have considerable speculative investors, high information asymmetry, and recurrent regulatory intervention which weaken the market efficiency and endorse herding. Even though herding is more prevalent in the emerging market, often composite market index in an emerging market can not reveal the herding behavior because the absence of herding in one industry may offset the presence of herding in another industry. Thus industry-specific herding analysis is more implicative for investors, academicians, and regulatory bodies.
The robust and dynamic financial sector is crucial for the growth and expansion of the economy in Bangladesh (Barua & Barua, Citation2021; Moudud-Ul-Huq et al., Citation2020; Yesmine et al., Citation2022). Even the economy is heavily dependent on the money market; the performance of the banking system is nothing but the growth and prosperity of the economy (Mujeri & Rahman, Citation2009). Each year government permits new banks and bank branches towards economic development (Banerjee et al., Citation2020). The market capitalization of banking sectors is about 14 percent of the total market capitalization in Dhaka stock exchange and remains at the top three industries in the economy (DSE, Citation2021). Again, banks are the financial institutions that survive on the public image, how its stakeholders perceive banks are crucial to its operation and success. Thereby examining the price discovery process in the banking industry can play a substantial role in the decision-making process for the stakeholders. As this study attempts to detect any herding evidence that demonstrates the investors’ behavior to flock together disregarding private or public information, it becomes an empirical confirmation of whether asset prices in the banking sector represent fundamentals or just follow the impulse of the investors. Only Ahsan and Sarkar (Citation2013) studied herding behavior in the Dhaka stock exchange for the period January 2005 to December 2011 and used techniques of Christie and Huang (Citation1995) and Chang et al. (Citation2000). They were unable to detect the presence of herding in the stock market in Bangladesh and reported the investors’ rational decision-making power using their own or otherwise public information. Since earlier studies claimed the inefficiency in this market (Ahmed, Citation2002; Mobarek et al., Citation2008; Arefin & Rahman, Citation2011; Shiblu and Ahmed, Citation2015;) and reported most investors as less informed (Nguyen et al., Citation2011; Rahman et al., Citation2017). Thus, the findings of Ahsan and Sarkar (Citation2013) is quite misleading. Considering the varying herding evidence at the different industries in the same market and its ability to lead absence of herding if anyone uses a composite market index, only industry-wise investigation can lead to a better understanding of the herding behavior. As a result, this study particularly focuses on the herding behavior in banking sector for its diverse implication to the economy.
The remainder of the paper is organized as follows. Section 2 describes the data and methodology of the study and section 3 presents the results and discussion and finally, section 4 concludes the paper.
2. Data and methodology
2.1. Data
This study uses daily data of thirty listed commercial banks from January 2007 to December 2018. The sample includes 3126 trading days and the daily closing stock prices of 30 banks. DSE library is the source of the data used in this study. Stock return is measured as . The market return of this industry is calculated by averaging the daily return of all banks at the time.
2.2. Methodology
The study uses the widely accepted methodology of cross-sectional standard deviation (CSSD) and cross-sectional absolute deviation (CSAD) proposed by Christie and Huang (Citation1995) and Chang et al. (Citation2000), respectively to find the herding pattern in banks listed in Dhaka Stock Exchange, Bangladesh. The recommended CSSD method follows;
Where is the individual stock return and
is the market return calculated by averaging return of N securities at t. N and t represent no. of securities in the banking sector and time, respectively. Now the CSSD is regressed on a constant and two dummy variables representing extreme market behavior.
Where,,
are two dummy variables for distinguishing the extreme period, one for a lower extreme period and another is for the upper extreme period. Both periods of extreme are defined at 99%, 95%, and 66% levels in the upper or lower tail of the market return distribution.
= 1, when market return falls in the lower tail in the distribution otherwise 0 and
=1, when market return falls in the upper tail in the distribution otherwise 0. At the time of extreme period, CSSD becomes smaller. Thus when
and
are negative and significant, then herding exists in the market.
Due to the use of the squared term, the cross-sectional standard deviation of return is highly affected by outliers. A better measure, cross-sectional absolute deviation (CSAD), is proposed by Chang et al. (Citation2000) which not only captures the herding but also assesses the non-linearity with the change in market conditions. Where the calculation of remains the same as the CSSD model The measure of CSAD is as follows;
Now according to Chang et al. (Citation2000) the equation for detecting the non-linear pattern of herding is as follows:
The model is improved following Cakan and Balagyozyan (Citation2014) by incorporating dummy variables reflecting the period of the world financial crisis in 2008 and the domestic financial crisis in 2010. In following equations, and
represent the global financial crisis and domestic share market crash, respectively. Where the period of global financial distress constitutes from 15 September 2008 to 15 July 2009 and the domestic financial crisis covers from 6 December 2010 to 5 August 2012.
The study then approaches to identify the pattern of herding during rising (upward) and falling (downward) market conditions. As a result, the following models of CASD are used:
Here lower than 0 market return indicates downmarket or upmarket otherwise. Negative and significant will indicate herding behavior.
Now the question may arise on what happens at the time of extreme up (bull) and extreme down (bear) market condition, to answer it following models are used:
Where takes the value of 1 when market return falls in the upper tail of the distributions of returns or otherwise zero. Similarly,
takes the value of 1 when market return falls in the lower tail of the distributions of returns or otherwise zero. Both the tails are defined at .99, .95, and .66 levels of confidence.
3. Empirical results
The descriptive statistics of cross-sectional standard deviation (CSSD) and cross-sectional absolute deviation (CSAD) and the market return (MR) are presented in Table . It displays that mean of CSSD, CSAD and MR are 3.44 percent, 1.14 percent, and 1.16 percent, respectively. The standard deviations of CSSD, CSAD, and MR are 3.91 percent, 0.75, and 49.59 percent respectively. All the series are positively skewed and kurtosis is greater than 3. The study employs OLS regression in CSSD and CSAD models assuming the normality tendency of a large sample size. Only the model which can detect herding will be further checked for robustness through the GARCH (1,1) method assuming nonlinearity since the null hypothesis of normality is rejected due to significant Jarque-Bera statistics in Table . The ADF test statistics of—28.82,—23.76, and −48.38 for CSSD, CSAD, and MR, respectively, confirm the stationary at a 1 percent level.
Table 1. Descriptive Statistics
Firstly the study approaches with a comparative investigation of cross-sectional standard deviation (CSSD) and cross-sectional absolute deviation (CSAD) model to detect herding in the banking sector in Bangladesh. Table presents the results of the OLS regression of the CSSD and CSAD model at .99, .95, and .66 confidence levels of upper and lower tails of the market. Even though the CSSD and CSAD models have significant and
, they cannot detect the herding due to positive coefficients. Since positive values of
and
imply stock return dispersion becomes larger with the rise in market return, and vice-versa; and their relation is linear, it reflects the CAPM assumptions of rational pricing theory. This result is consistent with Ahsan and Sarkar (Citation2013), Sharma (Citation2018), Huang et al. (Citation2015).
Table 2. Regression results for CSSD and CSAD model
Now the study approaches towards the basic model of CSAD of Chang et al. (Citation2000), which assumes stock return dispersion to nonlinearly increase or decrease with the change in market return and is regarded as more appropriate than the method of Christie and Huang (Citation1995). Again this study incorporates dummies to represent global financial crisis (GFC) and domestic financial crisis (DFC). The models with GFC and DFC run just after the basic model of CSAD. In table , the study presents the results of three models of CSAD, one is the basic model of Chang et al. (20,000), other models have GFC and DFC dummy respectively. All these model studies the whole sample period and report the significant negative value of , which indicates investors follow the market, flock together and herding exists. The insignificant dummy for GFC expresses the insusceptibility of investors’ behavior in the banking sector in Bangladesh to the international market. On the other hand, a dummy for DFC reports the existence of herding during a domestic financial crisis.
Table 3. Regression results for CSAD
The existence of herding for the whole period is further examined through the GARCH (1, 1) model and the estimated results are presented in Table . It is noticeable that is significant and negative. Thus, herding persists in the GARCH (1, 1) model which confirms the robustness of the findings in the OLS regression.
Table 4. GARCH (1, 1) results
After detecting the herding for the entire study period, this study divides the study period in terms of upward market and downward market. This division is initiated to detect any asymmetry in the herding behavior that persists if the market condition changes. Table presents the summary statistics of the upward and downward markets. This table shows division of market return into two parts based on its sign that is positive market return means upward market movement and the negative market return is downward market movement. Out of 3126 observations, 1632 represent upmarket, and the rest 1494 indicate the downmarket. The mean of CSAD at the upward market and downward market are 1.22 percent and 1.07 percent, respectively. Both CSAD series is positively skewed and kurtosis is greater than 3. The mean market return of the upmarket is 1.2 percent whereas the mean return of the downmarket is −1.02 percent. However, their standard deviations lie between 1.10 percent to 1.37 percent. The Jarque-Bera statistics and ADF test statistics infers the same conclusion of non-normality and stationary time series, respectively.
Table 5. Descriptive statistics of upmarket and downmarket condition
Table presents the herding behavior at the upward market and downward market conditions. The upmarket confirms herding behavior through the significant negative value of . While the downmarket is unable to pronounce any herding behavior. These findings are in line with the papers like Cakan and Balagyozyan (Citation2014), Huang et al. (Citation2015), Medhioub and Chaffai (Citation2019).
Table 6. Regression results for Upmarket and Downmarket
As herding behavior is mostly observed in the rising market in the banking sector in Bangladesh, this paper further investigates herding behavior incorporating both models of Christie and Huang (Citation1995) and Chang et al. (Citation2000) so that herding behavior can be evaluated in the context of extreme upmarket (bull market) and extreme downmarket (bear market). Table reports the results for an extreme upmarket and an extreme downmarket. In different criteria, the bull market persistently reports an inverse connection between return deviation and market return by significant negative values of . On the other hand, the bear market presents a significant positive value of
that implies return deviation moves with the changes of the market return. This positive relation indicates reverse herding or no herding in the bear market. Investors tend to escape from their flock as it appears to be a falling market. As result, this study confirms the asymmetry in herding behavior which is consistent with the findings of earlier literature like Chaffai and Medhioub (Citation2018), Shrotryia and Kalra (Citation2019).
Table 7. Regression results for extreme up and extreme down market
4. Conclusion
Primarily the study focuses on the detection of herding in the banking sector in Bangladesh; it extends its analysis to explain herd behavior in market asymmetries. By utilizing the daily data from January 2007 to December 2018, the study reveals the existence of herding in the banking sector and confirms the herding nature of investors during the domestic financial crisis but an absence of herding during the Global financial crisis. By dividing the study period into upmarket and downmarket contexts, this paper finds herding in the banking sector is common in a rising market but blurred in a falling market. This phenomenon is further confirmed when the extreme market condition of the bull and bear phase is used. At the bear phase investors expose anti-herd behavior to avoid the market catastrophe whereas they tend to mimic others overlooking any private information to gain from the market momentum.
This study affirms the nonlinearity in herding behavior as a quadratic term in the model of Chang et al. (Citation2000) is negative and significant. This indicates the incremental changes in the level of herding are less than proportional to the average market return. Eventhough herding persists in the banking sector of Bangladesh and there is connection between level of herding and average market return, but market return is inconclusive to define the exact level of herding because the same amount of market return may yield varying levels of herding. This study may turn out a significant insight to the regulatory agency in explaining investors’ behavior during domestic and international stock market crises. The asymmetry and nonlinearity in herding behavior in the banking sector have both social and financial implications that investors and regulatory bodies should perform with caution as the stocks are mispriced and market participants have varying behavior thereby banking sector would pronounce surprise now and then.
The study could have been further improved considering macroeconomic variables and fundamentals, volatility, and sentiments along with more industries and expanded periods in the domestic and foreign stock market.
Acknowledgements
The author is very thankful for the comments of the Editor and the anonymous reviewers which significantly improved this work.
Disclosure statement
No potential conflict of interest was reported by the author(s).
Additional information
Funding
References
- Ahmed, M. F. (2002). Market efficiency in emerging stock markets: The case of Dhaka stock exchange (DSE). Savings and Development, 26(1), 49–11. http://www.jstor.org/stable/25830783
- Ahsan, A. F. M., & Sarkar, A. H. (2013). Herding in Dhaka stock exchange. Journal of Applied Business and Economics, 14(2), 11–19. http://www.na-businesspress.com/JABE/AhsanAFMM_Web14_2_.pdf
- Arefin, J., & Rahman, R. M. (2011). Testing different forms of efficiency for Dhaka stock exchange. International Journal of Financial Services Management, 5(1), 1–20. https://doi.org/10.1504/IJFSM.2011.038325
- Avery, C., & Zemsky, P. (1998). Multidimensional uncertainty and herd behavior in financial markets. American Economic Review, 88, 724–748. https://www.jstor.org/stable/117003
- Banerjee, A. V. (1992). A simple model of herd behavior. Quarterly Journal of Economics, 107(3), 797–817. https://doi.org/10.2307/2118364
- Banerjee, P. K., Kayum, M. A., & Uddin, H. (2020). Financial sector development and its contribution to economic development of Bangladesh. In M. Barai. (Ed.), Bangladesh’s Economic and Social Progress. Palgrave Macmillan (pp. 147–178). https://doi.org/10.1007/978-981-15-1683-2_5
- Barua, B., & Barua, S. (2021). COVID-19 implications for banks: Evidence from an emerging economy. SN Bus Econ, 1(19), 1–28. https://doi.org/10.1007/s43546-020-00013-w
- Bekiros, S., Jlassi, M., Lucey, B., Naoui, K., & Uddin, G. S. (2017). Herding behavior, market sentiment and volatility: Will the bubble resume? North American Journal of Economics and Finance, 42, 107–131. https://doi.org/10.1016/j.najef.2017.07.005
- Bikhchandani, S., Hirshleifer, D., & Welch, I. (1992). A theory of fads, fashion, custom, and cultural change as informational cascades. Journal of Political Economy, 100(5), 992–1026. https://doi.org/10.1086/261849
- Brennan, M. (1993). Agency and asset prices. Finance Working Paper No. 6-93, UCLA.
- Cakan, E., & Balagyozyan, A. (2014). Herd behaviour in the Turkish banking sector. Applied Economics Letters, 21(2), 75–79. https://doi.org/10.1080/13504851.2013.842629
- Chaffai, M., & Medhioub, I. (2018). Herding behaviour in Islamic GCC stock market: A daily analysis. International Journal of Islamic and Middle Eastern Finance and Management, 11(2), 182–193. https://doi.org/10.1108/IMEFM-08-2017-0220
- Chang, E. C., Cheng, J. W., & Khorana, A. (2000). An examination of herd behavior in equity markets: An international perspective. Journal of Banking and Finance, 24(10), 1651–1679. https://doi.org/10.1016/S0378-4266(99)00096-5
- Chiang, T. C., & Zheng, D. (2010). An empirical analysis of herd behavior in global stock markets. Journal of Banking and Finance, 34(8), 1911–1921. https://doi.org/10.1016/j.jbankfin.2009.12.014
- Christie, W., & Huang, R. D. (1995). Following the pied piper: Do individual returns herd around the market? Financial Analysts Journal, 51(4), 31–37. https://doi.org/10.2469/faj.v51.n4.1918
- Conlisk, J. (1980). Costly optimizers versus cheap imitators. Journal of Economic Behavior and Organization, 1(3), 275–293. https://doi.org/10.1016/0167-2681(80)90004-9
- Demirer, R., Kutan, A. M., & Chen, C. (2010). Do investors herd in emerging stock markets? Evidence from the Taiwanese market. Journal of Economic Behaviorand Organization, 76(2), 283–295. https://doi.org/10.1016/j.jebo.2010.06.013
- Devenow, A., & Welch, I. (1996). Rational herding in financial economics. European Economic Review, 40(3–5), 603–615. https://doi.org/10.1016/0014-2921(95)00073-9
- DSE., (2021). Monthly review of Dhaka stock exchange. https://www.dsebd.org/assets/pdf/Mreview_July_21.pdf
- Garg, A., & Jindal, K. (2014). Herding behavior in an emerging stock market: Empirical evidence from India. Journal of Applied Finance, 20(1), 28–40. https://econpapers.repec.org/article/icficfjaf/v_3a20_3ay_3a2014_3ai_3a2_3ap_3a18-36.htm
- Huang, TC., Lin, BH. & Yang, TH. (2015). Herd behavior and idiosyncratic volatility. Journal of Business Research, 68(4), 763–770. https://doi.org/10.1016/j.jbusres.2014.11.025
- Javaira, Z., & Hassan, A. (2015). An examination of herding behavior in Pakistani stock market. International Journal of Emerging Markets, 10(3), 474–490. https://doi.org/10.1108/IJoEM-07-2011-0064
- Keynes, J. M. (1936). The general theory of employment, interest and money. Macmillan.
- Lakonishok, J., Shleifer, A., & Vishny, R. W. (1992). The impact of institutional trading on stock prices. Journal of Financial Economics, 32(1), 23–43. https://doi.org/10.1016/0304-405X(92)90023-Q
- Lindhe, E. (2012). Herd behavior in stock markets: A Nordic study. Master thesis, Department of Economics, Lunds University.
- Lux, T. (1995). Herd behavior, bubbles and crashes. Economic Journal, 105(431), 881–896. https://doi.org/10.2307/2235156
- Medhioub, I., & Chaffai, M. (2019). Islamic finance and herding behavior theory: A sectoral analysis for Gulf Islamic stock market. International Journal of Financial Studies, 7(65), 1–11. https://doi.org/10.3390/ijfs7040065
- Mobarek, A., Mollah, S., & Bhuyan, R. (2008). Market efficiency in emerging stock market: Evidence from Bangladesh. Journal of Emerging Market Finance, 7(17), 17–41. https://doi.org/10.1177/097265270700700102
- Moudud-Ul-Huq, S., Zheng, C., Gupta, A. D., Hossain, S. K. A., & Biswas, T. (2020). Risk and performance in emerging economies: Do bank diversification and financial crisis matter? Global Business Review, 097215092091530. https://doi.org/10.1177/0972150920915301
- Mujeri, M. K., & Rahman, M. H. (2009). Financing long term investments in Bangladesh: Capital market development issues. eSocialSciences. Accessed 6 February 2022.
- Nguyen, C. V., Islam, A. M., & Ali, M. M. (2011). The current state of the financial sector of Bangladesh: An analysis. AIUB Bus Econ Working Paper Series. American International University Bangladesh.
- Nofsinger, J., & Sias, R. (1999). Herding and feedback trading by institutional and individual investors. The Journal of Finance, 54(6), 2263–2295. https://doi.org/10.1111/0022-1082.00188
- Olsen, R. A. (2011). Financial risk perceptions: A behavioral perspective. In R. Yazdipour (Ed.), Advances in entrepreneurial finance with applications from behavioral finance and economics (pp. 45–68). Springer.
- Pingle, M. (1995). Imitation versus rationality: An experimental perspective on decision making. The Journal of Socioeconomics, 24(2), 281–315.
- Prosad, J. M., Kapoor, S., & Sengupta, J. (2012). An examination of herd behavior: An empirical evidence from Indian equity market. International Journal of Trade, Economics and Finance, 3(2), 154–157. http://www.ijtef.org/papers/190-Q00021.pdf
- Rahman, M. T., Hossain, S. Z., & Habibullah, M. (2017). Stock Market Crash in Bangladesh: The Moneymaking Psychology of Domestic Investors. American Journal of Theoretical and Applied Business, 3(3), 43–53. https://doi.org/10.11648/j.ajtab.20170303.12
- Roll, R. (1992). A mean/variance analysis of tracking error. The Journal of Portfolio Management, 18(4), 13–22. https://doi.org/10.3905/jpm.1992.701922
- Scharfstein, D. S., & Stein, J. C. (1990). Herd behavior and investment. The American Economic Review, 80(3), 465–479. https://scholar.harvard.edu/files/stein/files/aer1990_0.pdf
- Sewell, M., (2011). History of the Efficient Market Hypothesis. Working paper, University of Cambridge, UK
- Sharma, K. (2018). Herding in the banking sector of Indian stock market: An empirical study of BANKNIFTY. Journal of Commerce and Accounting Research, 7(2), 34–43. http://www.publishingindia.com/jcar/47/herding-in-the-banking-sector-of-indian-stock-market-an-empirical-study-of-banknifty/676/4738/
- Shiblu, K. A. & Nowrin, A. (2015). Determining the Efficiency of Dhaka Stock Exchange (DSE): A Study based on Weak Form Efficient Market Hypothesis. The Comilla University Journal of Business Studies, 2(1), 67–86.
- Shiller, R. J., (2002). From efficient market theory to behavioral finance. Cowles Foundation Discussion Papers 1385, Cowles Foundation, Yale University.
- Shiller, R. J., & Pound, J. (1986). Survey evidence on diffusion of investment among institutional investors. NBER Working Papers 1851, National Bureau of Economic Research, Inc.
- Shleifer, A., & Summers, L. H. (1990). The noise trader approach to finance. Journal of Economic Perspectives, 4(2), 19–33. https://doi.org/10.1257/jep.4.2.19
- Shrotryia, V.K. & Kalra, H. (2019). An empirical investigation of herding in the Indian stock market. e-Journal of Social and Behavioural Research in Business, 10(1), 40–53.
- Spyrou, S. (2013). Herding in financial markets: A review of literature. Review of Behavioural Finance, 5(2), 175–194. https://doi.org/10.1108/RBF-02-2013-0009
- Tan, L., Chiang, T. C., Mason, J. R., & Nelling, E. (2008). Herding behavior in Chinese stock markets: An examination of A and B shares. Pacific-Basin Finance Journal, 16(1), 61–77. https://doi.org/10.1016/j.pacfin.2007.04.004
- Trueman, B. (1994). Analyst forecasts and herding behavior. Review of Financial Studies, 7(1), 97–124. https://doi.org/10.1093/rfs/7.1.97
- Wermers, R. (1999). Mutual fund herding and the impact on stock prices. Journal of Finance, 54(2), 581–622. https://doi.org/10.1111/0022-1082.00118
- Yesmine, T., Hossain, M. E., Khan, M. A., Mitra, S., Saha, S. M., & Amin, M. R. (2022). Benchmarking the banking sector of Bangladesh: A comprehensive analysis of performance and efficiency, Asian Journal of Economics and Banking, ahead-of-print No. ahead-of-print. https://doi.org/10.1108/AJEB-08-2021-0094
- Zwiebel, J. (1995). Corporate conservatism and relative compensation. Journal of Political Economy, 103(1), 1–25. https://doi.org/10.1086/261973