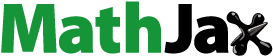
Abstract
The corporate debt reversibility analysis can be carried out not only from the owner/manager’s active intervention perspective but also from the perspective of a mechanical reversion, independent of owner/managers’ deliberations. Our study aims to discover how and which theoretical perspective underlying reversibility has the most significant impact on the capital structure of Portuguese industrial small and medium-sized enterprises (SMEs). The present paper proposes a new approach linking the measures commonly used to determine the target leverage level to the specific assumptions of theories addressing debt dynamics. Our results show that the perspective with the stronger impact on capital structure materialises in the dynamic trade-off theory assumptions. However, owners/managers also strongly consider the industry references to which firms belong. The perspective of mechanical debt reversion also contributes, at its level, to the firms’ debt permanent reversibility in the sense of possible long-term stationarity.
1. Introduction
The debt reversibility can be analysed from the perspective of the intervention of managers/owners or under a perspective independent of their deliberations.
The trade-off model assumes that there is an active owners/manager’s intervention in maintaining the optimal leverage level to maximise the firm value by the balance that tax savings and firm debt costs provide (e.g., Myers, Citation1984; Myers & Majluf, Citation1984). Therefore, these assumptions are directly tied to the firms’ characteristics once we consider that decisions regarding the debt dynamics are taken in the tax savings and the financial default context. The behavioural theory also argues the existence of a manager’s active intervention in the debt adjustments or its reversibility with an impact on the firm’s capital structure (e.g., Sen & Oruc, Citation2009). Underlying assumptions are completely different from those assumed by the trade-off theory (Brendea & Pop, Citation2019). Decisions under uncertainty are taken from the perspective of imitation behaviour (herd behaviour) since they are directly tied to the industry characteristics the firms belong to (e.g., Leary & Roberts, Citation2014). On the other hand, the mechanical mean reversion theory raised by Chen and Zhao (Citation2007) argues that the debt ratio reverts to a firm’s average leverage without economic meaning, independent of the owner/managers’ deliberations, which can lead to long-lasting debt stationarity (Lemmon et al., Citation2008).
Several optimal (or target) leverage ratio proxies have been used to explain firms’ capital structure dynamics. However, different measures (or proxies) have shown to be inconsistent in explaining a single theoretical perspective. According to D’Mello and Farhat (Citation2008), the choice of a proxy is crucial in empirical tests because inferences drawn depend critically on the measure (proxy) used. Furthermore, limited empirical support for the hypothesis that firms adjust their leverage ratio towards the proxied optimal ratio, as in Shyam-Sunder and Myers (Citation1999), leads to conclude that the trade-off theory does not explain firms’ capital structure choices. D’Mello and Farhat (Citation2008) tested three different proxies to analyse which measure best explains the dynamic trade-off perspective and obtained divergent results depending on the proxies used. Accordingly, these divergent results may be due to the measures (proxies) with different specific characteristics representing (identifying with) theoretical perspectives with specific characteristics.
In this scope, our paper aims to analyse how and what the theoretical perspective underlying debt reversibility has the most significant impact on the firm’s capital structure. The behaviour of owners/managers regarding the debt reversibility decisions can be made based on (i) firm characteristics, (ii) imitation of debt level of industry tendency, which may serve as a benchmark for creditors, or (iii) taking into account an “unknown factor” that also partially causes mechanical debt ratio reversion around its mean. This way, our paper allows us to immediately move away from the absolute or hegemonic perspective of a dynamic trade-off as regards the issues about the decisions of the adjustments or debt reversion and its tendency to exhibit possible long-lasting stationarity around its mean (e.g., Lemmon et al., Citation2008), mitigating the reducer aspect of the specific conclusions each analysis.
Based on a sample of 4 990 small and medium-sized enterprises (SMEs) from the Portuguese industrial sector for 2010–2019, we link each of the three leverage target ratios according to each theoretical approach. Our findings show that the perspective with the stronger impact on capital structure materialises in the dynamic trade-off theory assumptions. Notwithstanding, owners/managers also strongly consider the industry references to which firms belong. We also find that the perspective of mechanical debt reversion also contributes, at its level, to the firms’ debt permanent reversibility in the sense of possible long-term stationarity.
The rest of this paper is organised as follows. In section 2, we carry out the literature review to expose the most significant aspects of our study and the hypotheses to be tested. In section 3, we present the methodology and the econometric models. In section 4, we perform the interpretation and discussion of results, and section 5 concludes.
2. Literature review and hypotheses development
2.1. Measures (proxies) of target debt
Measures (proxies) used to represent the firm’s optimal debt have distinct characteristics and provide different results even within the scope of the trade-off theory (D’Mello & Farhat, Citation2008). These authors analysed which measure best explained debt adjustments since “not much attention has been paid to the validity of these leverage ratios as proxies for the optimum capital structure” (226). D’Mello and Farhat (Citation2008) stated that the choice of proxy is crucial in empirical tests because the inferences drawn depend critically on the measure used. According to these authors, the optimal debt ratio is unknown, and therefore, previous studies that test trade-off theory commonly use one of the following measures (proxies): (i) the average debt of a sample time series, (ii) the moving average based on historical debt ratios, (iii) the median debt of the industry to which firms belong, and (iv) the predicted debt ratio based on the estimates of a cross-sectional regression (the prediction models).
The debt time-series average assumes each firm has its optimal debt objective, which remains constant over time. Jalilvand and Harris (Citation1984), Shyam-Sunder and Myers (Citation1999), and Byoun and Rhim (Citation2005), among others, used this measure as a representation of optimal debt. The moving average of the historical debt ratio requires the firm to have at least one year of past data and eliminates the most severe drawback of the firm debt average. This includes future information not available to managers, as the number of historical information increases with each subsequent year added and, therefore, varies over time. This measure was also used by Jalilvand and Harris (Citation1984) and Shyam-Sunder and Myers (Citation1999). The median of the industry leverage to which firms belong assumes that each sector has an optimal debt, which is the firm’s debt objective. This level (ratio) also changes over time according to the variation of the industry characteristics to which firms belong. For example, Graham and Harvey (Citation2001), Leary and Roberts (Citation2014), among others, found that industry leverage ratios influence firms’ financing decisions.
Finally, the optimal debt represented in a predictive way (i.e., based on cross-sectional regression models) considers each firm’s specific characteristics. It uses the explanatory variables that constitute the direct determinants of the firms’ capital structure. Several authors used this measure (proxy) in the analysis of the dynamic trade-off as it varies according to the firm specifics or characteristics and over time (e.g., Aybar-Arias et al., Citation2012; Drobetz et al., Citation2006; Fitzgerald & Ryan, Citation2019; Flannery & Rangan, Citation2006; Frank & Goyal, Citation2008; Hovakimian et al., Citation2001; Memon et al., Citation2021; Rubio & Sogorb, Citation2011; Sardo et al., Citation2020). For the reasons mentioned, it is probably the most used measure (proxy) in the analysis of the dynamic trade-off.
2.2. The scope of dynamic trade-off
Given its essential assumptions, the dynamic trade-off theory is based on the conjectures directly related to firms’ specific characteristics (the capital structure determinants). The balance between tax savings level and leverage costs, the bankruptcy probability in the ambit of transaction costs, and the financial default contribute to the maximization of its value (e.g., Myers, Citation1984; Myers & Majluf, Citation1984). Within the scope of this theory, we can also add the agency issues in general (e.g., Jensen & Meckling, Citation1976; Jensen, Citation1986), and the agency costs that arise as a function of debt levels (e.g., Nishihara et al., Citation2019).
As mentioned in the previous section, the various studies concerning adjustments in the context of the dynamic trade-off, lead to different results according to the measure (proxy) used to determine the optimal debt level. Each of those measures has different characteristics, which provide different results within the scope of the trade-off theory analysis (D’Mello & Farhat, Citation2008). These authors claim (214) that “the choice of the proxy is crucial in empirical tests because the inferences drawn depend critically on the measure used”, and therefore, “the results and conclusions of studies that test the tradeoff theory are sensitive to the proxy used”. Even within the scope of the consistency with this theory, the results leading to infer different adjustment speeds can also lead to different conclusions. Within the specific analysis of the trade-off theory, researchers sometimes find contradictory results (e.g., Strebulaev, Citation2007), especially when it is confronted with competing theories (e.g., Xu, Citation2007). The analysis of Strebulaev (Citation2007), which uses a dynamic trade-off model, leads to the rejection of the model; in the sense that according to the former, the target debt is constant for any firm. This author states that (1772), “the observed positive relation between leverage and profitability at the refinancing point is purely a cross-sectional effect”. Also, “the negative relation is at the individual firm level since higher profitability lowers the current leverage of an individual firm unless it refinances in that period”. According to Xu (Citation2007), the fact that the authors analyse whether firms adjust toward the target leverage level has become an essential question in evaluating the credibility of competing capital structure theories.
The static and dynamic trade-off theories predict that firms will quickly revert to their optimal debt ratio whenever there are deviations in their capital structures. In contrast, the pecking order, market timing, and inertia theories all argue that firms do not have a market-value leverage target. Therefore, they will not adjust quickly toward the optimum. According to this author, a fast adjustment speed is therefore interpreted as supporting the trade-off theory. In contrast, a slow adjustment is interpreted as inconsistent with the target capital structure theory. For example, Flannery and Rangan (Citation2006) find that the speed of adjustment is 34.1% per year and argue that this speed is consistent with the dynamic trade-off theory. In Fama and French (Citation2002) firms adjust at a low pace (according to these authors, at a “snail” pace) which supports the pecking order theory. Huang and Ritter (Citation2009) interpret the slow speed of adjustment (11.3%) to be consistent with the market timing theory. For example, Gungoraydinoglu and Öztekin (Citation2021), when testing the debt objective relative to the different institutional factors, use three measures (proxies) of target debt. These authors report different percentage results for the same sample, although relatively close, in the sense of proving that firms quickly adjust their debt ratios. These values differ (Table , 12) according to the measures used to determine the optimal debt (e.g., for Portugal, 41.38%; 32.74%, and 36.05, respectively). When interpreting the evidence found in their analysis, Fitzgerald and Ryan (Citation2019) state that their findings vary with the categorization criterion and the specification of the target leverage. Byoun (Citation2008) uses a mixed prediction model (cross-sectional regressions) that includes the firms and industry characteristics, concluding that adverse selection costs and other costs and benefits must be part of a unified capital structure theory.
Table 1. Subsectors (Industry)
Table 2. Definition of the variables
Table 3. Statistics of variables *
Each study justifies the measure (proxy) used for the optimal debt according to its perspective in the specific context of its analysis. Several authors use proxies based on predictive models (cross-section) under the argument that certain specific variables constitute the determinants of the firm’s optimal debt (e.g., Bauer, Citation2004; Bradley et al., Citation1984; Kester, Citation1986). Other authors use industry characteristics (e.g., means/medians of industry variables) under the argument that firms of same industry tend to have a similar capital structure, and therefore, the same optimal debt level (Bradley et al., Citation1984; Fischer et al., Citation1989; Hull, Citation1999). On the other hand, Lemmon et al. (Citation2008), show that this dynamic rebalancing is directed towards a debt target that is largely invariant in time, in which firms’ financial policies are carried out to keep debt ratios relatively close to their long-term average values (stationarity).
Additionally, other issues can be considered regarding debt stationarity around its average. Several studies (e.g., Bontempi & Golinelli, Citation2001; Ahsan et al., Citation2016; Lin, Citation2020, among others) consider that a debt stationary series supports the trade-off theory. Non-stationary ones support the pecking order theory, in which the unit root tests can provide empirical support, depending on the fact whether the debt series is stationary or not. According to Drobetz et al. (Citation2006), the target debt determined through the historical mean debt constitutes exogenous to the firm (e.g., in Fama & French, Citation2002; Shyam-Sunder & Myers, Citation1999). This means that average leverage can also be considered, at least partly, independent of the characteristics that determine the optimal debt from the perspective of the dynamic trade-off. This way, we can also consider other perspectives regarding the debt reversion or convergence to a target value, within the scope of behavioural theory (e.g., Camara, Citation2017; Filbeck et al., Citation1996; Sen & Oruc, Citation2009) or even from the perspective of managers’ active intervention absence (e.g., Chen & Zhao, Citation2007).
Notwithstanding the assumptions or factors underlying the owners/managers’ intervention within the scope of the main financial theories on capital structure, we can still consider “the point of view” of the zero-leverage phenomenon analysed by several authors, asserting that despite the considerable advances made during the last decade, it is still not clear which are the theoretical approaches that best explain the zero-leverage phenomenon (e.g., Saona et al., Citation2020). The trade-off, pecking-order, and agency theories cannot explain such conservatism levels of debt (e.g., Morais et al., Citation2021). Conclusively, we can assert that the studies of debt adjustments, and, therefore, the debt reversibility in the trade-off theory analysis context, can constitute reductive to the perspective of the existence of only a target debt under the assumptions of tax shield and the financial default probability.
2.3. Corporate herd behaviour
Until recently, most works on the dynamics of firms’ leverage explained debt adjustments or their reversibility through the trade-off theory assumptions. One of the current assumptions underlying owners/managers’ decisions that lead to the firms’ debt reversibility towards a considered ideal value (or target value) can additionally result from a owners/managers’ imitation behaviour, revealed through the herd behaviour theory of corporate behavioural finance. This perspective consequently falls within the scope of owners/managers’ cognitive biases (e.g., Filbeck et al., Citation1996; Kahneman & Tversky, Citation1979; Zeckhauser et al., Citation1991). This dynamics of the debt of firms can therefore be explained in an alternative or complementary way through new concepts of behavioural theory, which lead in practical terms to “similar” functional results. Therefore, these also result from the owners/managers’ active intervention in the context of adjustment decisions or from the tendency for managers to revert debt to the leverage levels they consider ideal. This way, there is also an adjustment behaviour, but with completely different underlying motives (assumptions) from those presumed by the dynamic trade-off theory (e.g., Sen & Oruc, Citation2009). According to Brendea and Pop (Citation2019), a firm that acts according to herd behaviour moves away from its optimal capital structure, and thus loses the maximization of its value. These authors conclude, therefore, that it is in the managers’ interest to avoid herd behaviour, as they should try to act rationally when deciding on the firms’ financing sources.
In this context, the manager’s intervention in the firm will consider the characteristics of similar firms belonging to the same activity sector and, therefore, exogenous to its firm. Banerjee (Citation1992) defines herding behaviour as “everyone doing what everyone else is doing, even when their private information suggests doing something quite different”. According to Camara (Citation2017), this generalised form of herding can be applied to various situations, including corporate financing decisions. Managers when decide to make adjustments toward debt mean/median levels of the industry to which they belong (e.g., Brendea & Pop, Citation2019; Zeckhauser et al., Citation1991), or according to the leading firms’ debt levels in the sector (e.g., Filbeck et al., Citation1996), they will therefore have to relegate to a smaller plan the characteristics of their firms (i.e., the relevant information that is crucial to pursuit the most important activities of their firm, and that is dictated by own firm’s results). For example, Zeckhauser et al. (Citation1991) propose the “herd migration theory”, which suggests that managers can demonstrate a herding behaviour on capital structure policies according to the sector to which firms belong. Thus, there is also a debt objective to be reached, but not that trade-off theory specifically advocates. In behavioural theory, the leverage level that owners/managers consider collectively ideal is, therefore, determined through the owners/managers’ cognitive biases in the decision process on the debt values to revert. Let’s recall that corporate herd behaviour appears subsequently to the Kahneman and Tversky (Citation1979) prospect theory through the cognitive biases that are part of its original version, namely, loss aversion and regret aversion.
According to Zeckhauser et al. (Citation1991), there are at least three reasons that lead firms managers to adopt a herd behaviour: (i) The existence of free-riding in the information acquisition (manager believes that similar firms are better informed because they are more successful); (ii) the feeling of protection for being part of a certain group (identification with a certain group); and (iii) the essential level of preferences regarding the basic norms or principles of the group to which constituents belong (decisions made outside the basic norms or principles of the group can exacerbate the self-censorship costs when facing a bad outcome). According to these authors (19), agency theory reveals that managers, “acting by the interests of shareholders”, are better informed, and in these circumstances (in the presence of this information asymmetry) it may be efficient to make payoffs to agents that are based on their relative performances. In such a scenario, agents may find it advantageous to herd, particularly if they are risk-averse.
From a broader perspective, this makes herd behaviour additionally advantageous for managers. According to prospect theory, the error of having made a bad decision is more bearable when lived collectively, existing a feeling or a tendency leading decision-makers to “follow” others in decision-making. In the firms’ scope, these considerations are part of the analysis context of the activity sectors, which can thus constitute the reference groups that behavioural theory (more specifically, prospect theory) subscribes. Sen and Oruc (Citation2009, 34) state that “tendency of ‘avoiding from regret’ which is shown frequently by decision-makers may be expressed by the finding that regret pain is stronger than the pleasure caused by feeling of pride. This detection of behavioural finance may cause the directors who seek optimal capital structure to show a behaviour that we may called as ‘herd behaviour’ in capital structure decisions. Thus, since they will act with their group, digesting a possible failure or wrong decision will be easier in comparison with digesting a failure in case of avoiding the herd”. From the perspective of these authors, herd behaviour regarding capital structure decisions is consolidated in that decision-makers follow the average debt ratios of the sector to which they belong. As well, concerning the business risk perception, banks would therefore have reservations in granting credit to firms with debt levels that exceed the average leverage ratios of the respective activity sector.
Several authors have shown that the industry’s average/median debt ratios are important determinants of the firms’ capital structure (e.g., Welch, Citation2004; MacKay & Phillips, Citation2005; Frank & Goyal, Citation2008, among others). More recent authors also share these arguments. In the formulation of their analysis, Leary and Roberts (Citation2014) state that managers in a context of uncertainty determining their optimal capital structure will consider financing decisions and the characteristics of peer firms since the true model (optimal) is unknown. These authors show that firms do not make financing decisions in isolation. They conclude that funding decisions (for reasons of learning and reputation), and the characteristics of peer firms, are important capital structure determinants because peer firms’ behaviour does indeed have a remarkably robust and large impact on firms’ capital structure.
Anwar et al. (Citation2019) state that the results of their studies confirm that the peer firms’ variables (characteristics) coefficients are greater than the specific variables (characteristics) coefficients of their firms, revealing that while setting the firm’s leverage policy more attention is given to the averages of the peer firm characteristics (belonging to the same activity sector). These authors cite MacKay and Phillips (Citation2005) in that they confirmed that the characteristics of peer firms are more relevant for a firm to define its financial policies.
According to Anwar et al. (Citation2019), there are, therefore, three effects in this process. The endogenous effects are when firms, following the actions of their peers, decide to act in the same way. Exogenous effects occur when a firm adjusts its financial policy accordingly to changes in the characteristics of the peer firms’ financial policies. And finally, the correlated effects that occur when a change in the external environment makes it mandatory for the firm and the industry to align their financial policies, respectively.
Consistent with these results, the analysis by Brendea and Pop (Citation2019) indicates that Romanian listed firms adopt a herd behaviour and try to reach the average/median debt level of the sector they belong. According to these authors, their model estimates suggest that firms’ capital structure depends not only on the characteristics of their firms but also on the average characteristics of the firms in the industry to which they belong. In particular, these authors also find that firms do not follow the ideal capital structure but the capital structure (average/median) of the industry they belong, thus moving away from maximizing their value—the trade-off theory claims that firm maximises value when its debt is at the optimal level (e.g., Graham, Citation2006; Miller, Citation1977; Myers & Majluf, Citation1984). Damodaran (Citation2010) also suggests that firms tend to follow the leading firms in their industry, hoping to imitate their success.
These findings, therefore, lead us to conclude that they are constituted as a result of an analysis that does not reduce the leverage reversibility to the debt objective within the scope of the dynamic trade-off theory assumptions. By considering the owners/managers cognitive biases, those findings insert the reversibility in the behavioural perspective. Thus, as mentioned above, the present analysis also includes the adjustments or the debt reversibility in the subject of the owners/managers’ active intervention. However, we can assert that it is more effectively in line with the point of view of the corporate herd behaviour theory. Conclusively, we can, in this way, also place the leverage reversibility in another (or third) perspective—within the scope of a reversion trend of the leverage ratio that can be merely mechanical (e.g., Chen & Zhao, Citation2007) and that, therefore, it also does not inhibit the owners/managers’ active intervention.
2.4. Mechanical mean reversion
Lemmon et al. (Citation2008, 1577) refer to the existence of an “unidentifiable component” of debt that makes it stable or stationary over time. According to these authors, that component is divided into a transitory part, which translates into the convergence of debt ratios to more moderate leverage levels (which are due, at least “in part”, to the managers’ decisions); and the other, which leads to the stability of the leverage average ratios, since firms tend to maintain a stable debt level for more than 20 years periods. Lemmon et al. (Citation2008, 1577) suggest that the active leverage ratio management is at least “partially” responsible for the debt mean reversion. Therefore, if it is “partially” constituted, we can argue that this reversion may also be admissible in a debt dynamic independent of the owners/managers’ deliberation, which mechanically remains towards the average debt ratio (Chen & Zhao, Citation2007). Therefore, it happens without the owners/managers’ active intervention. These authors raised this issue arguing that debt can perform its reversion mathematically. In Chen and Zhao (Citation2007, 227), the reasoning underlying the analysis of deviations in Flannery and Rangan (Citation2006) from the perspective of the debt objective, was centered on leverage ratios that are related to the mechanics of the reversion trend around debt average, and not by the fact it was constituted in an arbitrary decision of adjustment to its optimal level.
From the Chen and Zhao (Citation2007) perspective, it is not clear whether the mean reversion materialises through the mechanics inherent in debt, as a ratio (in their mathematical perspective), or if it is due to the fact managers deliberately adjust. Other authors also share this argument, pointing out the presence of a merely mechanical debt reversion to the average leverage. Shyam-Sunder and Myers (Citation1999, 240) state that there can be a reversion to the mean, but that this does not mean that firms’ issue or redeem debt in order to move toward a target debt. These authors use a moving average as a measure of optimal leverage. Chang and Dasgupta (Citation2009) use simulations similar to Shyam-Sunder and Myers (Citation1999), showing the existence of a reversion that may only be mechanical, around the mean, without being deliberately conducted toward a debt target. These authors state that it is not enough to look at debt ratios, but it is necessary to consider the firm’s financing behaviour (or policy).
Kang and Jang (Citation2016) also show the existence of a mechanical debt reversion, obtaining similar significant values for the parameter of the reversion to mean, in the sense that they can also be obtained through simulated samples when using financing without a debt target or objective. These findings are also shared by Iliev and Welch (Citation2010), who use a new “placebo” process for the leverage ratios incorporating it with specific alternative hypotheses. Conclusively, studies that analyse debt reversion from the mathematical perspective of a ratio (e.g., Shyam-Sunder & Myers, Citation1999; Chen & Zhao, Citation2007; Chang & Dasgupta, Citation2009; Iliev & Welch, Citation2010; Kang & Jang, Citation2016) are consistent with the existence of a merely mechanical debt reversion towards to its average, with no economic meaning. This perspective does not reduce the debt reversibility of the owners/managers’ active intervention results.
2.5. Hypotheses
Our analysis is therefore consolidated in a study that includes the three most important theoretical considerations underlying debt dynamics or its reversibility, specified according to the characteristics of the measures used to represent the optimal debt. The permanent reversibility of firms’ debt can be due, therefore, not only to the owners/managers’ active intervention, in the sense of classical motives of dynamic trade-off classical theory, but also to those that may be underlying the perspective of the owners/managers’ cognitive biases. Notwithstanding, it can also materialise in the form of persistent reversibility (Lemmon et al., Citation2008) or merely mechanical (or mathematical), according to the Chen and Zhao (Citation2007) argument, without the managers’ active intervention. Therefore, this enables us to have a more specific analysis of what underlies the “praxis” of the owners/managers’ decision or the debt reversibility determinants in general. Thus, it allows us to set up the following hypotheses:
H1—Factors underlying debt reversion are due to dynamic trade-off assumptions;
H2—Factors underlying debt reversion are due to the owners/managers’ imitation behaviour, within the scope of the herd behaviour theory assumptions;
H3—Factors underlying debt reversion are independent of owners/managers’ deliberations—they can lead to long-term stationarity;
3. Methodology
3.1. Data and variables
The data consists of a panel sample constituted of 4 990 small and medium-sized enterprises (SMEs) from the Portuguese industrial sector, according to the code of NACE Rev. 2—Primary, for the period 2010–2019. This sample was obtained from the SABI Database supported by Van Dijk Bureau. Table shows the codes, the description and percentages in terms of each sector’s representativeness in the sample. The sample is not fully balanced as there are some missing values (around 2.13%), in some years for some firms in the sample period.
The raw data include the items on the balance sheets and firms’ income statements, which are transformed into variables in the form of ratios, constituting the capital structure determinants to be analysed. The variables’ definition is represented in Table .
The present study aims to establish a direct relationship between the theoretical arguments related to debt dynamics and the practical measures commonly used in the representation of considered optimal debt level or to which leverage tends to revert (target debt). In practical terms, the study essentially analyses the debt response (or the debt changes) to the gap between the current leverage level and debt to which reverts (target debt). This reversion, in general terms, takes place essentially within the scope of the owners/managers’ intervention or through a possible mathematical reversion around the average leverage.
3.2. Estimation methods
To analyze the different theoretical and practical arguments underlying debt reversibility, we use three similar mathematical models, each elaborated according to one of the three perspectives under analysis. The models differ only on the variable representing the distance (the lag) between current debt and target debt (the leverage level to which tends to revert). This level is determined according to the measure (or representation—proxy) that each perspective defines to establish the target debt and, therefore, its distance to the yearly observed debt. For estimation purposes, this variable is determined through the (mathematical) modulus of the distance between the two debt levels.
The generic model that generates the specific models of the perspectives presented is adapted similarly to the adjustment models used by various authors (e.g., Ozkan, Citation2001; Flannery & Rangan, Citation2006; Wanzenried, Citation2006; Byoun, Citation2008; Faulkender et al., Citation2012; Rihab & Lotfi, Citation2016; Devos et al., Citation2017; Aybar-Arias et al., Citation2012; Sardo et al., Citation2020, Citation2021), based on the model by D’Mello and Farhat (Citation2008) in the determination of the best proxy for the optimal capital structure. Unlike D’Mello and Farhat (Citation2008), who use each measure (proxy) of the target debt to determine which one provides greater firm value under the trade-off theory assumptions; we use each measure according to each perspective to analyze what is the most important factor of debt reversibility in general terms. Contrary to those authors, in our analysis we do not intend to reduce leverage reversibility to the classical trade-off theory basic foundations.
For this reason, we additionally use control variables related to the firms’ specific characteristics and the industry they belong (e.g., Brendea & Pop, Citation2019; Chang & Dasgupta, Citation2009; Chen & Zhao, Citation2007; Leary & Roberts, Citation2014). For the estimates, we apply the STATA software, which enables greater and better data “handling” compared to other software in the constitution, variables of interest instrumentalization and application to the regressions. In the estimating regression models, we use the (ordinary least squares—OLS) with the white’s heteroskedasticity-robust standard errors test to obtain more robust results. This test makes it possible to detect the presence of heteroscedasticity and subsequently remove it. After correcting the possible correlation levels between the residuals and explanatory variables, and the residuals variance level, this estimator proved to be the most adequate in determining the correlations, so we used these estimates to interpret the results.
Firms revert their debt levels so that their current (annual) leverage ratio is close to the considered target leverage ratio. This leads to an adjustment (or reversal) mechanism given by the following model:
Where: is the current debt ratio;
is the target debt ratio of firm i, of sector j, and in period t, determined for the proposed models according to each raised perspective (in an endogenous and exogenous way), respectively: (i) through the determinants of each firm capital structure in a cross-sectional predictive model; (ii) median of the industry to which firms belong, and (iii) through the average leverage of the considered sample period.
can be interpreted as the distance from the target debt to current debt, while only a part of the distance to the target leverage level is reached, which is equal to (
. This difference constitutes the response of the debt variation to the lag between the target debt and the current (annual) debt. Where:
represents the firm debt i, of sector j, in year t; (
represents current debt in the previous period;
is the parameter that indicates the level of response of the debt variation (
to the distance between the target debt level and the observed leverage in the previous period
. Rearranging the terms of equation (1), current debt is determined in the form of the following model:
If firms do not adjust (i.e., if there is no reversion) to the debt target level, the value of will be equal to or very close to zero, and thereby, the current debt level is equal to the debt level in the previous period (
). If there is a total adjustment (or a total reversion to the debt target level), the parameter
will take a value equal to or very close to “1”, meaning that current debt is subsequently equal to the target debt (
=
The coefficient
will, therefore, have a value between 0 and 1, representing the response level of the firm’s debt variation to the distance between its target debt and the observed debt for each year. The reversion level or adjustment is partial (due to transaction costs and other factors inherent to the reversion process, in general terms), which results from the owners/managers’ active intervention or when the reversion takes place independently of the owners/managers’ deliberations.
Extending the equation (1), our generic mathematical model is constituted as follows:
Where represents the dependent variable (ΔTotDebt), i.e., change in the total debt ratio of firm i, of sector j, and in period t;
represents, in general terms, the variable that is the distance from the target debt to the observed debt, determined in a different way for each model, and, therefore, constituting the variable differentiating the models according to each perspective raised: (i)
, (ii)(ii)
a nd (iii)
, as shown below;
represents the control variables vector (firm characteristics): Tang, SIZE, NDTS, ROA, EfTax, and Intang;
represents the second vector of the control variables (mean of industry characteristics to which the firms belong): TangMI, SIZEMI, NDTSMI, ROAMI, EfTaxMI, and IntangMI;
is the error term.
The first variable representing the distance from the target (optimal) debt to the observed debt for each firm (i.e., the target debt is determined through a cross-sectional predictive regression model, considering the firms specific characteristics and therefore, more suitable for the analysis within the scope of the dynamic trade-off theory assumptions. The model includes the firm’s capital structure-specific determinants (e.g., in Aybar-Arias et al., Citation2012; Byoun, Citation2008; Devos et al., Citation2017; Sardo et al., Citation2020), as described in the following mathematical model:
Where: represents the optimal debt (replaces the variable
in the generic model (3)) which refers to the assumptions of trade-off theory (TO), according to the firm characteristics (e.g., in Leary & Roberts, Citation2014), and therefore is determined in an endogenous way;
represents the vector of the most significant determinants of firm i capital structure, of sector j, and in period t, (Tang; SIZE; NDTS; ROA; EfTax; Intang); and
is the error term (the residuals). The analysis model in the trade-off perspective is represented as follows:
In which the variable represents the distance from the target debt to the observed debt in the current period (year), relative to the dynamic trade-off theory.
The second variable, which represents the distance from the target debt to the current debt for each firm, the target debt is determined through the median leverage of the industry to which firms belong (according to the herd behaviour theory perspective), in the form of the following model:
Where: , represents the target debt (replaces the variable
in the generic model (3) of firm i, sector j, and the period t (according to Anwar et al. (Citation2019), this optimal debt is exogenously determined because it refers to the characteristics of the industry to which firm belongs);
represents the median debt of peer firms belonging to the activity sector to which firm belongs (e.g., in Brendea & Pop, Citation2019; Frank & Goyal, Citation2008; Graham & Harvey, Citation2001; Leary & Roberts, Citation2014; Sen & Oruc, Citation2009). The analysis model in the herd behaviour perspective is therefore represented as follows:
Where the variable represents the distance from the target debt to the observed debt in the current period (year).
When analyzing herd behaviour, several authors use both the mean and the median of industry debt to which firms belong as a measure of target debt to assess whether they adopt herd behaviour (e.g., Brendea & Pop, Citation2019; Camara, Citation2017; Fama & French, Citation1997; Frank & Goyal, Citation2008; Leary & Roberts, Citation2014). For example, according to Brendea and Pop (Citation2019), determining target debt through the debt average or median produces similar results. After carrying out a more accurate (statistical) analysis of the measure to be used (average or median of the industry) for these estimates, we decided to use the debt median because it produces more robust results.
(iii) In the third variable, which represents the distance from the target debt to the current debt of the period (yearly), the target debt is determined through the average leverage of the sample period through the following model:
Where: represents the target debt (replaces the variable
in the generic model (3)), (according to Drobetz and Wanzenried, Citation2006, the target debt determined through the sample average it constitutes an exogenous factor; and from the point of view of; Chen & Zhao, Citation2007, it constitutes independent of the managers’ deliberations);
represents the average debt in the sample period (e.g., in Jalilvand & Harris, Citation1984; Shyam-Sunder & Myers, Citation1999; Byoun & Rhim, Citation2005; among others). Regarding the practical aspect of our analysis, the use of average or the moving average is indifferent, as our sample is cross-sectional, comprising an equal sample period for all firms. Therefore, we decided to use the simple average of the sample, as the moving average results are very similar in terms of correlations between the variables. The analysis model, from the debt reversion perspective without the owners/managers’ active intervention, is represented as follows:
Where the variable represents the distance from the target debt to the observed debt in the current period (yearly).
(iv) The remaining variables (control variables), aforementioned are the endogenous and exogenous capital structures determinants, respectively: the firm characteristics and the industry characteristics they belong to (e.g., Brendea & Pop, Citation2019; Camara, Citation2017; Frank & Goyal, Citation2008; Leary & Roberts, Citation2014). In order to carry out a complimentary analysis within the scope of the Covid-19 pandemic impact on the firms’ capital structure, we additionally use the Covid variable (dummy variable), which enables us to analyse the pandemic impact on the Portuguese SMEs capital structure in our sample.
4. Results
4.1. Descriptive statistics
Table presents the descriptive statistics of the variables.
The ΔTotDebt variable shows that the values series of the sample’s total debt (yearly) change presents, on average, a large variance, around 8.4%, which is above the average debt variations value, for a total of35669observations. This immediately suggests a high variability level in corporate debt, whether negatively or positively. The extreme values corroborate this argument because this variable also shows, on average, a minimum (negative) ratio of around—61.2%, and a (positive) maximum ratio of 61%, suggesting that the negative and positive variations may be high. We can observe that variations are, on average, slightly more negative than positive, although very close in value, as there is symmetry between these two values. This may suggest the existence of an effective debt response to possible changes in the values of the variables that represent the distance from the target debt to the current debt and, therefore, the existence of a reversion trend.
The distance from the target debt determined through the cross-sectional predictive model from the trade-off theory perspective (DistTO) shows, on average, a greater ratio than that of the other two similar variables, which represent, respectively, the distance from the target debt to the current debt from the herd behaviour perspective (DistIN) and the perspective of mechanical mean reversion (DistMA) of Chen and Zhao (Citation2007). The respective standard deviations also show a proportional values series variance of the ratios of these variables, indicating that, on average terms, the variations in the values series of the variable (DistTO) in the trade-off theory perspective also are greater than the variations in values of the similar variables. However, the volatility in individual terms for these variables is low, as they have variances below respective averages. This suggests that the respective series values remained stable (reduced variance) throughout the sample period. This may also indicate, in the face of the greater variations in total debt (ΔTotDebt), that these variations can strongly respond to small changes in the distances from target debt to observed debt for each period (yearly). It may also indicate a debt reversibility trend, which constitutes most likely a result, both within the scope of the owners/managers’ intervention, and within the scope of the existence of a reversion without economic meaning (e.g., Chen & Zhao, Citation2007). These variables also show, on average, very different extremes, with very small minimums (very close to zero) and maximums with ratios ranging from 0.462 to 0.598.
The DistIN variable suggests that, on average, in a collective way (industry), the distance from the target debt to the observed yearly debt, is very small or null on minimum, showing, however, a maximum with a value of 0.598. This may suggest that there are industries where this distance is very small or null in a given set of firms. In these terms, there will not necessarily exist reversibility in the debt levels of these firms (or it may be punctual in certain periods in time). Concerning the analysis of the maximums by counterpart, the values suggest that there will be sectors where this distance could be quite high and, therefore, a necessarily high level of debt reversibility, in the sense that this series (DistIN) presents relative stability, as indicated by its standard deviation. This result suggests that the strong (or fast) debt variation may contribute to this stability, as happens for the series of the other similar variables of the distance (DistTO and DistMA).
In general terms, the statistics suggest the existence of great heterogeneity in the analysed firms’ capital structure characteristics. Table shows the correlation levels between variables.
Table 4. Correlation matrix
Table presents the absence of multicollinearity problems. The variables are correlated far below 50%, showing that the collinearity level between variables is not relevant (Gujarati & Porter, Citation2010).
4.2. Debt reversibility determinants—results
Test statistics in Table show the test for the differences between the variables’ estimated coefficients that represent the distance between the target debt and observed debt, according to the perspectives (i), (ii) and (iii) presented. Differences are presented in terms of comparison pairs. This test makes it possible to immediately compare and assess the level of impact (or effect) on the capital structure resulting from the theoretical and practical perspectives underlying debt reversibility.
Table 5. Pairs test of the differences among coefficients of the variables of the distances to target debt
The results show that there exist significant differences between the estimated coefficients for the variables representing the distance (lag) between the target debt levels and the observed debt, except for the last pair of differences (iii) which compares the estimated parameters from the perspective of manager intervention in the context of herd behaviour and from the perspective of the mechanical mean reversion of Chen and Zhao (Citation2007). However, these results do not mean that the mechanical reversion of Chen and Zhao (Citation2007) is not, at its level, an additional contributory factor in the joint determination of corporate leverage reversibility. Estimates of the respective models also enable us to obtain additional relevant information.
Table shows the results of the estimates for the models according to the respective perspectives presented.
Table 6. Estimates results
The results in Table show that the estimated coefficients of the variables representing the distance from the target debt to the observed debt are significant for all estimation models. This suggests that all underlying theoretical and practical perspectives contribute, in part (each one own scale), to corporate debt reversibility in general terms. Therefore, we cannot reject the formulated hypotheses H1, H2 and H3.
The DistTO variable representing the distance between the target debt and the observed debt (from the trade-off model perspective) has a higher estimated coefficient than the coefficients for the other similar variables of the competing models. This suggests that the perspective of the trade-off theory underlying owners/managers’ decisions has a greater impact than the other two perspectives. A unit variation of the lag (distance) from optimal debt to observed debt corresponds to a variation of 14% in firms’ debt, in contrast to a variation of 13% and 12.7%, relative to the perspectives of herd behaviour and mechanical mean reversion, respectively. These results are reinforced by Table , as we can verify through the tests carried out on the differences among coefficients of the variables representing the distances to target debt relative to perspectives of the trade-off, herd behaviour, and mechanical mean reversion theories.
Regarding the estimated parameters for the control variables from model 1, the variables Tang, SIZE, and NDTS are significant and in line with the trade-off theory (e.g., DeAngelo & Masulis, Citation1980; Rajan & Zingales, Citation1995; Booth et al., Citation2001; Rihab & Lotfi, Citation2016; among others). This gives robustness to own model, given the assumptions of this theory. The estimated results for these variables, relative to models 2 and 3, also do not contradict the respective underlying theoretical perspectives assumptions. However, they also do not add robustness to the respective assumptions. For model 2, the results also suggest that, although owners/managers adopt a herd behaviour underlying the firms’ capital structure decisions (e.g., Brendea & Pop, Citation2019; Leary & Roberts, Citation2014) do not exclude the fact they may also partly consider the assumptions of the tax savings and the financial default possibility. This way, owners/managers consider both the firm-specific and their industry characteristics (e.g., Brendea & Pop, Citation2019; Leary & Roberts, Citation2014). Regarding model 3, despite the underlying perspective (e.g., Chang & Dasgupta, Citation2009; Chen & Zhao, Citation2007; Kang & Jang, Citation2016), the results for the control variables (Tang, SIZE and NDTS) suggest that, although debt reversibility can be constituted independently of the owners/managers’ decisions, they also do not exclude the possibility that owners/managers take into account the assumptions of tax savings and the financial default probability.
Regarding the ROA variable, we found estimates with significantly negative parameters for this variable regarding models 1, 2 and 3, suggesting that, despite the assumptions underlying the perspective of each model, Portuguese firms primarily prefer funding through internal resources (e.g., Nunes & Serrasqueiro, Citation2017; Pacheco, Citation2016; Vergas et al., Citation2015). These results are also consistent with authors finding trade-off and pecking order theories are not mutually exclusive (e.g., Lisboa, Citation2017; Pacheco & Tavares, Citation2017; Proença et al., Citation2014; Serrasqueiro & Caetano, Citation2015) since our results also suggest that Portuguese SMEs effectively behave in line with the trade-off theory assumptions. The estimated parameters for the EfTax and Intang variables are not significant for the three models, and, therefore, the result neither favours nor contradicts the perspectives raised. This result shows that the tax rate paid has no repercussions on debt, as it is insignificant, and the estimated parameters’ value is extremely low. The estimated results for the Intang variable suggest that Portuguese SMEs’ growth opportunities, proxied by intangible assets in the current study, do not impact the debt level.
Regarding the exogenous control variables (those that refer to the characteristics of the industry to which the firms belong), the results for the variables TangMI, SIZEMI, NDTSMI, and ROAMI, present coefficients with significant signs, suggesting that owners/managers, when making decisions about capital structure consider the industry characteristics to which they belong. The coefficients of the TangMI, SIZEMI and NDTSMI variables present higher estimated values than those of variables that represent the firm’s specific characteristics, reinforcing the argument that firms make decisions according to not only to firm endogenous characteristics. This is also consistent with Leary and Roberts (Citation2014). Despite being significant, the ROAMI variable presents estimated parameters with lower values than its homologous endogenous ROA variable for the three models, varying proportionally according to the values that this variable presents concerning the firms’ specific characteristics.
In sum, the estimated results for the parameters of the TangMI, SIZEMI, NDTSMI and ROAMI variables mainly reinforce the perspective inherent to model 2 since they show that the industry characteristics also have a strong impact on owners/managers’ decisions in determining the firms’ capital structure. However, they also do not subvert the results relating to the perspectives underlying the other models. The reversibility within the scope of the trade-off and mechanical mean reversion perspectives do not imply that owners/managers cannot also consider the industry references to which firms belong. Therefore, these are the reasons pointed out by behavioural literature (e.g., Brendea & Pop, Citation2019; Filbeck et al., Citation1996; Kahneman & Tversky, Citation1979; Leary & Roberts, Citation2014; Sen & Oruc, Citation2009; Zeckhauser et al., Citation1991) which complements the understanding concerning the firms’ debt reversibility. The same argument can be applied concerning what the estimated results suggest for the variable IntangMI (representing, on average, the industry growth opportunities to which firms belong), as it is significant for models 2 and 3. This result reinforces the argument that Portuguese firms do not just follow the reference points of their firms when making debt decisions. Banks would therefore be reluctant to finance firms with leverage levels above the industry average to which they belong (e.g., Sen & Oruc, Citation2009), which is another reason justifying the fact that firms must also have to consider the industry references to which they belong. This additionally reinforces model 3 of the herding behaviour perspective; however, it also does not subvert the results for model 3, as previously mentioned. The fact that the results for this model point to a possible mechanical reversibility trend around the debt average does not imply that there can be no owner/managers’ intervention contributing to the debt reversibility in the analysed firms (factors that may work jointly).
The results for the Covid variable are significant for the three models, suggesting that this pandemic did indeed have a notable impact on the Portuguese firms’ activity. The significantly negative sign for the estimated parameter shows a retraction in firms’ debt levels. Debt is the main funding source for SMEs, as the national financial system is institutionally geared towards banking (e.g., Morais et al., Citation2021). Therefore, these results suggest that the economic and financial activity of Portuguese SMEs had an atypical behaviour in this period, meaning that firms resorted to bank funding to a lesser extent. Therefore, this indicator is consistent with the three perspectives analysed.
Finally, we can assert that the global results of the estimates validate all the hypotheses raised, as we found that all theoretical and practical factors (perspectives) contribute to the reversibility of the firms’ debt.
5. Conclusions
Our study’s aim consisted of analysing the theoretical and practical perspective underlying debt reversibility which has the greatest impact on the firms’ capital structure. Our methodological research proposes adapting the measures used to determine target debt to the specific assumptions of theories addressing debt dynamics. Given the theoretical and practical body on the subject, it allows us to raise several perspectives underlying debt reversibility in a more detailed analysis. The literature has analysed these perspectives separately, drawing conclusions that may become, in conclusive terms, reductive only to one of the analysed perspectives.
Our results show that the perspective underlying debt reversibility with the greatest impact on the capital structure of Portuguese industrial SMEs is materialised in the trade-off theory assumptions. However, the assumptions underlying the perspectives of the herd behaviour theory and from the mathematical point of view of mean mechanical reversion also strongly impact the determination of firms’ debt reversibility. Within the scope of owner/managers’ intervention, our results suggest that decision-makers consider the tax savings and financial default assumptions and the industry characteristics to which firms belong. From the perspective of mechanical debt reversion, the results also suggest the possibility of a trend without economic meaning for firms’ debt to revert towards its average. Our results also favour Lemmon and Zender (Citation2010) perspective in terms of stationarity that may be long-lasting.
Our results show that the Portuguese industry SMEs follow a behaviour according to the trade-off theory assumptions and, in the process, follow a pecking order of financing preferences. Regarding the industry characteristics to which firms belong also showed that owners/managers follow an imitation behaviour when making capital structure decisions. That indicates that the herding behaviour cognitive bias is present in the Portuguese SME’s debt decisions, suggesting that firms may sometimes deviate from the optimal capital structure, pushing firms away from their maximum value. This factor may also contribute to the need for permanent debt reversibility to considered ideal values.
Therefore, we can conclude that each perspective must be analysed according to the characteristics of each proxy used to determine the considered target debt. We also found that the Covid-19 pandemic diminished the firm debt level of Portuguese SMEs. Besides, although this result is not directly associated with the main focus of the present work, we believe it deserves further investigation in the future, even though there are already several recent studies that confirm the effect of this pandemic on the governance and performance of small and medium-sized firms (e, g., Dovbischuk, Citation2022; Shafeeq Nimr Al-Maliki et al., Citation2022).
The results of our study allow us to argue that factors complying with the three raised perspectives contribute, albeit on different scales, to the debt reversion trend. No other study has raised the theoretical perspectives within the scope of the debt dynamics analysis. Its reversibility has always been studied according to only one of those perspectives. This opens the way for a new way of looking at the topic. Studies addressing debt reversibility or adjustments only from a theoretical perspective (either within the scope of the owner/managers’ intervention or independent of their deliberations) are, therefore, reductive to the assumptions of a single perspective.
Contrary to what materialises in most studies, which depart from a single theoretical and practical point of view, we can state that theoretical perspectives addressing the firms’ debt dynamics are not exclusive. When a study concludes that certain firms follow herd behaviour, the understanding at the conclusion level may be situated only in the perspective that owners/managers, when deciding in a context of uncertainty, only are limited to imitating the industry behaviour to which they belong. However, our results show that each perspective can constitute a particular case of the firms’ debt reversion trend since firms’ leverage reversibility cannot be reduced to a single perspective. Thus, we can say that the Portuguese SMEs’ debt reversibility and resultant capital structure constitution materialise due to the “partial” sums of these theoretical and practical factors.
Our analysis has some limitations. It only uses book debt, as most firms in our sample are not listed on the Stock Exchange, thus not enabling the inclusion of market debt. Another limitation is that our sample has a little data missing, although in a very small percentage, which becomes less relevant given the number of observations our sample provides.
Our paper provides evidence that permanent debt reversibility is not due only to a specific factor in that the various theoretical and practical perspectives are not exclusive but complementary.
Disclosure statement
No potential conflict of interest was reported by the author(s).
Additional information
Funding
References
- Ahsan, T., Man, W., & Qureshi, M. A. (2016). Mean reverting financial leverage: Theory and evidence from Pakistan. Applied Economics, 48(5), 379–23. https://doi.org/10.1080/00036846.2015.1080802
- Anwar, M., Hassan, A., & Hameed, F. (2019). Peer effect in firms’ financial decision making: Evidence from corporate capital structure. Journal of Managerial Sciences, 13(3), 33–47.
- Aybar-Arias, C., Casino-Martinez, A., & Lopez-Gracia, J. (2012). On the adjustment speed of SMEs to their optimal capital structure. Small Business Economics, 39(4), 977–996. https://doi.org/10.1007/s11187-011-9327-6
- Banerjee, A. V. (1992). A simple model of herd behavior. The Quarterly Journal of Economics, 107(3), 797–817. https://doi.org/10.2307/2118364
- Bauer, P. (2004). Determinants of capital structure, empirical evidence from the Czech Republic. Czech Journal of Economics and Finance, 54(1), 2–21.
- Bontempi, M. E., & Golinelli, R. 2001. “Is financial leverage mean-reverting? Unit root tests and corporate financing models”. Working Papers 422. Dipartimento Scienze Economiche, Universita’ di Bologna.
- Booth, L., Aivazian, V., Demirguc-Kunt, A., & Maksimovic, V. (2001). Capital Structure in Developing Countries. The Journal of Finance, 56(1), 87–130. https://doi.org/10.1111/0022-1082.00320
- Bradley, M., Jarrell, G. A., & Kim, E. H. (1984). On the existence of an optimal capital structure: Theory and evidence. The Journal of Finance, 39(3), 857–877. https://doi.org/10.2307/2327950
- Brendea, G., & Pop, F. (2019). Herding behavior and financing decisions in Romania. Managerial Finance, 45(6), 716–725.https://doi.org/10.1108/MF-02-2018-0093
- Byoun, S. (2008). How and When Firms Adjust Their Capital Structure toward Targets? The Journal of Finance, 63(6), 3069–3096. https://doi.org/10.1111/j.1540-6261.2008
- Byoun, S., & Rhim, J. (2005). Tests of the pecking order theory and the trade-off theory of optimal capital structure. The Global Business and Finance Review, 10, 1–20.
- Camara, O. (2017). Industry herd behaviour in financing decision making. Journal of Economics and Business, 94, 32–42. https://doi.org/10.1016/j.jeconbus.2017.08.001
- Chang, X., & Dasgupta, S. (2009). Target behavior and financing: How conclusive is the evidence. The Journal of Finance, 64(4), 1767–1796. https://doi.org/10.1111/j.1540-6261.2009.01479.x
- Chen, L., & Zhao, X. (2007). Mechanical mean reversion of leverage ratios. Economics Letters, 95(2), 223–229. https://doi.org/10.1016/j.econlet.2006.10.008
- Damodaran, A. (2010). Applied corporate finance. In 3rd edition, John Wiley & Sons.
- DeAngelo, H., & Masulis, R. (1980). Optimal capital structure under corporate and personal taxation. Journal of Financial Economics, 8(1), 3–29. https://doi.org/10.1016/0304-405X(80)90019-7
- Devos, E., Rahman, S., & Tsang, D. (2017). Debt covenants and the speed of capital structure adjustment. Journal of Corporate Finance, 45, 1–18. https://doi.org/10.1016/j.jcorpfin.2017.04.008
- D’Mello, R., & Farhat, J. (2008). A comparative analysis of proxies for an optimal leverage ratio. Review of Financial Economics, 17(3), 213–227. https://doi.org/10.1016/j.rfe.2007.06.001
- Dovbischuk, I. (2022). Innovation-oriented dynamic capabilities of logistics service providers, dynamic resilience and firm performance during the COVID-19 pandemic. The International Journal of Logistics Management, 33(2), 499–519. https://doi.org/10.1108/IJLM-01-2021-0059
- Drobetz, W., Pensa, P., & Wanzenried, G. 2006. “Firm characteristics and dynamic capital structure adjustment”. SSRN: https://ssrn.com/abstract=952268 or
- Fama, E. F., & French, K. R. (1997). Industry costs of equity. Journal of Financial Economics, 43(2), 153–193. https://doi.org/10.1016/S0304-405X(96)00896-3
- Fama, E. F., & French, K. R. (2002). Testing trade-off and pecking order predictions about dividends and debt. Review of Financial Studies, 15(1), 1–33. https://doi.org/10.1093/rfs/15.1.1
- Faulkender, M., Flannery, M. J., Hankins, K. W., & Smith, J. M. (2012). Cash flows and leverage adjustments. Journal of Financial Economics, 103(3), 632–646. https://doi.org/10.1016/j.jfineco.2011.10.013
- Filbeck, G., Gorman, R. F., & Preece, D. C. (1996). Behavioral aspects of the intra- industry capital structure decision. Journal of Financial and Strategic Decisions, 9(2), 55–67.
- Fischer, E., Heinkel, R., & Zechner, J. (1989). Dynamic capital structure choice: Theory and tests. The Journal of Finance, 64(1), 19–40. https://doi.org/10.1111/j.1540-6261.1989.tb02402.x
- Fitzgerald, F., & Ryan, J. (2019). The impact of firm characteristics on speed of adjustment to target leverage: A UK study. Applied Economics, 51(3), 315–327. https://doi.org/10.1080/00036846.2018.1495822
- Flannery, J. M., & Rangan, K. P. (2006). “Partial adjustment toward target capital structures. Journal of Financial Economics, 79(3), 469–506. https://doi.org/10.1016/j.jfineco.2005.03.004
- Frank, M., & Goyal, V. 2008. “Trade-off and pecking order theories of debt”. Research - Working Paper, Center for Corporate Governance, Tuck School of Business at Dartmouth. https://doi.org/10.2139/ssrn.670543.
- Graham, G. R. (2006). A review of taxes and corporate finance. Foundations and Trends in Finance, 1(7), 573–691. https://doi.org/10.1561/0500000010
- Graham, J. R., & Harvey, C. R. (2001). The theory and practice of corporate finance: Evidence from the field. Journal of Financial Economics, 60(2–3), 187–243. https://doi.org/10.1016/S0304-405X(01)00044-7
- Gujarati, D., & Porter, D. 2010. “Essentials of econometrics”. 4th edition McGraw – Hill International.
- Gungoraydinoglu, A., & Öztekin, O. (2021). Financial leverage and debt maturity targeting: International evidence. Journal of Risk and Financial Management, 14(9), 1–36. https://doi.org/10.3390/jrfm14090437
- Hovakimian, A., Opler, T., & Titman, S. (2001). The debt-equity choice. The Journal of Financial and Quantitative Analysis, 36(1), 1–24. https://doi.org/10.2307/2676195
- Huang, R., & Ritter, J. (2009). Testing theories of capital structure and estimating the speed of adjustment. The Journal of Financial and Quantitative Analysis, 44(2), 237–271. https://doi.org/10.1017/S0022109009090152
- Hull, R. (1999). Leverage ratios, industry norms, and stock Price reaction: An empirical investigation of stock-for-debt transactions. The Journal of the Financial Management Association, 28(2), 32–45.
- Iliev, P., & Welch, I. 2010. “Reconciling estimates of the speed of adjustment of leverage ratios”. Researche - Working Paper. Department of Economics, Pennsylvania State University, Brown University.
- Jalilvand, A., & Harris, R. S. (1984). Corporate behavior in adjusting to capital structure and dividend targets: An econometric study. The Journal of Finance, 39, 127–145. https://doi.org/10.1111/j.1540-6261.1984.tb03864.x.1
- Jensen, M. C. (1986). Agency costs of free cash flow, corporate finance, and takeovers. American Economic Review, 76(2), 323–329.
- Jensen, M. C., & Meckling, W. H. (1976). Theory of the firm: Managerial behavior, agency costs and ownership structure. Journal of Financial Economics, 3(4), 305–360. https://doi.org/10.1016/0304-405X(76)90026-X
- Kahneman, D., & Tversky, A. (1979). Prospect theory: An analysis of decision making under risk. Econometrica, 47(2), 263–291. https://doi.org/10.2307/1914185
- Kang, Y. J., & Jang, W. W. (2016). Mechanical mean reversion of leverage ratios: analysis of south Korean firms. The Journal of Eurasian Studies, 13(3), 103–125. https://doi.org/10.31203/aepa.2016.13.3.005
- Kester, C. W. (1986). Capital and ownership structure: A comparison of United States and Japanese manufacturing corporations. Financial Management, 15(1), 5–16. https://doi.org/10.2307/3665273
- Leary, M. T., & Roberts, M. R. (2014). Do peer firms affect corporate financial policy? The Journal of Finance, 69(1), 139–178. https://doi.org/10.1111/jofi.12094
- Lemmon, M. L., Roberts, M. R., & Zender, J. F. (2008). Back to the beginning: Persistence and the cross-section of corporate capital structure. The Journal of Finance, 63(4), 1575–1608. https://doi.org/10.1111/j.1540-6261.2008.01369
- Lemmon, M., & Zender, J. (2010). Debt capacity and tests of capital structure theories. The Journal of Financial and Quantitative Analysis, 45(5), 1161–1187. https://doi.org/10.1017/S0022109010000499
- Lin, F.-L. (2020). “Do DJIA firms reflect stationary debt ratios? Economies, 8(4), 76. https://doi.org/10.3390/economies8040076
- Lisboa, I. (2017). Capital structure of exporter SMEs during the financial crisis: Evidence from Portugal. The European Journal of Management Studies, 22(1), 25–49.
- MacKay, P., & Phillips, G. M. (2005). How does industry affect firm financial structure? Review of Financial Studies, 18(4), 1433–1466. https://doi.org/10.1093/rfs/hhi032
- Memon, P. A., Md-Rusb, R., & Ghazalib, Z. B. (2021). Adjustment speed towards target capital structure and its determinants. Economic Research-Ekonomska Istraživanja, 34(1), 1966–1984. https://doi.org/10.1080/1331677X.2020.1860792
- Miller, M. H. (1977). Debt and Taxes. The Journal of Finance, 32(2), 261–275. https://doi.org/10.2307/2326758
- Morais, F., Serrasqueiro, Z., & Ramalho, J. (2021). The zero-leverage phenomenon in European listed firms: A financing decision or an imposition of the financial market? Business Research Quarterly, 1(23), 1–23. https://doi.org/10.1177/23409444211024653
- Myers, S. C. (1984). The capital structure puzzle. The Journal of Finance, 39(3), 575–592. https://doi.org/10.2307/2327916
- Myers, S. C., & Majluf, N. S. (1984). Corporate financing and investment decisions when firms have information that investors do not have. Journal of Financial Economics, 13(2), 187–221. https://doi.org/10.1016/0304-405X(84)90023-0
- Nishihara, M., Sarkar, S., & Zhang, C. (2019). Agency cost of debt overhang with optimal investment timing and size. Journal of Business Finance & Accounting, 46(5–6), 784–809. https://doi.org/10.1111/jbfa.12379
- Nunes, P. M., & Serrasqueiro, Z. (2017). Short-term debt and long-term debt determinants in small and medium-sized hospitality firms. Tourism Economics, 23(3), 543–560. https://doi.org/10.5367/te.2015.0529
- Ozkan, A. (2001). Determinants of capital structure and adjustment to long run target: Evidence from UK company panel data. Journal of Business Finance & Accounting, 28(1&2), 175–198. https://doi.org/10.1111/1468-5957.00370
- Pacheco, L. (2016). Capital structure and internationalization: The case of Portuguese industrial SMEs. Research in International Business and Finance, 38, 531–545. https://doi.org/10.1016/j.ribaf.2016.07.014
- Pacheco, L., & Tavares, F. (2017). Capital structure determinants of hospitality sector SMEs. Tourism Economics, 23(1), 113–132. https://doi.org/10.5367/te.2015.0501
- Proença, P., Laureano, R. M. S., & Laureano, L. M. S. (2014). Determinants of capital structure and the 2008 financial crisis: Evidence from Portuguese SMEs. Procedia - Social and Behavioral Sciences, 150, 182–191. https://doi.org/10.1016/j.sbspro.2014.09.027
- Rajan, R. G., & Zingales, L. (1995). What do we know about capital structure? Some evidence from international data. The Journal of Finance, 50(5), 1421–1460. https://doi.org/10.3386/w4875
- Rihab, B. A., & Lotfi, B. J. (2016). Managerial overconfidence and debt decisions. Journal of Modern Accounting and Auditing, 12(4), 225–241. https://doi.org/10.17265/1548-6583/2016.04.004
- Rubio, G., & Sogorb, F. (2011). The adjustment to target leverage of Spanish public firms: Macroeconomic conditions and distance from target. Revista de Economía Aplicada, 19(57), 1–29.
- Saona, P., Vallelado, E., & Martín, P. (2020). Debt, or not debt, that is the question: A Shakespearean question to a corporate decision. Journal of Business Research, 115, 378–392. https://doi.org/10.1016/j.jbusres.2019.09.061
- Sardo, F., Serrasqueiro, Z., & Félix, E. G. S. (2020). Does Venture Capital affect capital structure rebalancing? The case of small knowledge-intensive service firms. Structural Change and Economic Dynamics, 53, 170–179. https://doi.org/10.1016/j.strueco.2020.02.003
- Sardo, F., Vieira, S. E., & Serrasqueiro, Z. (2021). The role of gender and succession on the debt adjustments of family firm capital structure. Eurasian Business Review, 12(2), 349–372. https://doi.org/10.1007/s40821-021-00186-w
- Sen, M., & Oruc, E. (2009). Behavioral dimension of cross-sectional capital structure decisions: ISE (Istanbul Stock Exchange) application. International Research Journal of Financial and Economics, 28(1), 33–41.
- Serrasqueiro, Z., & Caetano, A. (2015). Trade-off theory versus Pecking order theory: Capital structure decisions in a peripheral region of Portugal. Journal of Business Economics and Management, 16(2), 445–466. https://doi.org/10.3846/16111699.2012.744344
- Shafeeq Nimr Al-Maliki, H., Salehi, M., & Kardan, B. (2022). “The effect of COVID 19. on risk-taking of small and medium-sized, family and non-family firms”. Journal of Facilities Management 1. ahead-of-print https://doi.org/10.1108/JFM-09-2021-0105
- Shyam-Sunder, L., & Myers, S. C. (1999). Testing static trade-off against pecking order models of capital structure. Journal of Financial Economics, 51(2), 219–244. https://doi.org/10.1016/S0304-405X(98)00051-8
- Strebulaev, I. (2007). Do tests of capital structure theory mean what they say? The Journal of Finance, 62(4), 1747–1787. https://doi.org/10.1111/j.1540-6261.2007.01256.x
- Vergas, N., Cerqueira, A., & Brandão, E. 2015. “The determinants of the capital structure of listed on stock market non-financial firms: Evidence for Portugal”. Working paper 0870-8541, FEP-UP, School of Economics and Management, University of Porto.
- Wanzenried, G. (2006). Capital Structure Dynamics in the UK and Continental Europe. The European Journal of Finance, 12(8), 693–716. https://doi.org/10.1080/13518470500460178
- Welch, I. (2004). Capital structure and stock returns. Journal of Political Economy, 112(1), 106–131. https://doi.org/10.1086/379933
- Xu, Z. 2007. “Do firms adjust toward a target leverage level?”. Working Paper/Document de travail 2007-50. Bank of Canada, 1-44.
- Zeckhauser, R., Patel, J., & Hendricks, D. (1991). Nonrational actors and financial market behavior. Theory and Decision, 31(2), 257–287. https://doi.org/10.1007/BF00132995