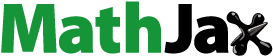
Abstract
We explore the taxonomy of cryptocurrencies and integrate our analysis with traditional ways of understanding financial assets. We thus classify cryptocurrencies using the time series and distributional properties of returns. Cryptocurrencies appear inherently speculative in nature. The result is even more clear cut when time series measures of distance are used. Results tally with wider concerns raised regarding excessive volatility of stablecoins.
1. Introduction
Cryptocurrencies are a new type of financial instrument that have received much popular (Buterin, Citation2022) and acade mic (Kayal & Rohilla, Citation2021) attention. The first major cryptocurrency Bitocin was created by Satoshi Nahomoto in 2008 as a peer-to-peer electronic currency without needing financial institutions to act as intermediaries. Though originally conceived as an alternative to national currencies (Dowd, Citation2014), cryptocurrency markets are famed for their dramatic boom-bust patterns and the potential for a complete collapse in market prices (J. Fry, Citation2018). Relatedly, early work suggests Bitcoin and cryptocurrencies are more of a speculative asset than a genuine currency (Baur et al., Citation2018; Corbet, Meegan, et al., Citation2018).
The empirical classification of cryptocurrencies remains an interesting problem in its own right. Alongside an interesting technical discussion (see Section 2) the statistical properties of cryptocurrency returns are known to change over time (Jiang et al., Citation2018; Urquhart, Citation2016). There is also a vast literature on cryptocurrencies covering areas as diverse as speculative bubbles (Corbet, Lucey, et al., Citation2018), market efficiency (López-Martin et al., Citation2021), price discovery (Doan et al., Citation2022) and the market response to the pandemic (Corbet et al., Citation2020).
We thus seek to empirically determine the extent to which Bitcoin and cryptocurrencies are a purely speculative asset or a genuine currency. Here, a comparison is made with both major currency pairs and major tech stocks. There is long-standing interest in the stylized empirical facts of both cryptocurrency markets (Al-Yahyaee et al., Citation2018) and financial markets more generally (Cont, Citation2001). Moreover, it is natural to approach this question from both distributional and time series perspectives.
Firstly, from a distributional perspective, it is well known that crypto assets exhibit boom-bust patterns sometimes culminating in complete collapse. Speculative bubbles are also not a necessary requirement for these effects to occur (Fry, Citation2018). Moreover, this marked volatility has previously raised questions about how well Bitcoin and cryptocurrencies can serve as a unit of account (Fry & Cheah, Citation2016). Secondly, the time series properties of crypto assets are interesting and important in their own right (Gkillas et al., Citation2022; Katsiampa, Citation2017). The comparison with the time series data from other asset classes is also a natural one to make (Al-Yahyaee et al., Citation2018).
In this paper we develop four new methods to classify crypto assets based on either their distributional or time series properties. Cryptocurencies appear to be inherently speculative in nature. The result can be seen most clearly from a time series perspective though a distributional approach also leads to broadly the same conclusions. Results are consistent with previous concerns raised over the excessive volatility of stablecoins (Ante et al., Citation2023; Briola et al., Citation2023; Hoang & Baur, Citation2021).
The layout of this paper is as follows. Section 2 lays out the methodology used. Empirical results are given in Section 3. Robustness checks are conducted in Section 4. Section 5 concludes.
2. Methodology
In this section we propose four classification methods for asset price returns:
A method based on the Kolmogorov-Smirnov distance.
A method based on the Cramér-von Mises distance.
A GARCH-based method based on the supremum norm.
A GARCH-based method based on the Euclidean norm.
Methods 1–2 thus derive from a distributional comparison. Methods 3–4 derive from a time series comparison.
2.1. Measuring distributional distance
A measure of the difference in distribution between two variables and
can be constructed as follows. Define the empirical CDF
as
Given two samples and
the Kolmogorov-Smirnov distance between the two distributions can be calculated as
As a robustness check an alternative measure of distributional distance can be obtained from the Cramér-von Mises distance. This involves more observations in the sample and is often thought to be more powerful than the Kolmogorov-Smirnov test in empirical applications. If denotes the pooled sample the Cramér-von Mises distance can be calculated in terms of the Euclidean distance between the two empirical CDFs as
2.2. Measuring time series distance
Given the apparent ubiquity of the GARCH(1, 1) in financial econometrics (Hansen & Lunde, Citation2005) we can define a notion of distance between two financial time series as follows.Footnote1. Suppose that using e.g. forward selection (Fry & Burke, Citation2022) two sets of ARCH and GARCH parameters are estimated:
By analogy with the Kolmogorov-Smirnov distance defined in (1) a notion of time series distance can be constructed via the supremum norm. Under the conventions that
define
A time series distance can then be constructed as
By analogy with the Cramér-von Mises distance in EquationEquation (2)(2)
(2) a second time series distance can also be constructed based around the Euclidean norm using
3. Empirical results
In this paper we compare the top-ten most highly capitalised cryptocurrencies: (Cardano, Binance Token, Bitcoin, Binance USD, Doge, Ethereum, Solana, USD Coin, USD Tether, and Ripple) with top major dollar-denominated national currency prices (Australian Dollar, Canadian Dollar, Swiss Franc, Chinese Yuan, Euro, GB Sterling, Hong Kong Dollar, Japanese Yen, South Korean Won, and New Zealand Dollar) and with ten leading tech stocks (Apple, Adobe, AMD, Amazon, Cisco, Dell, Google, Meta, Microsoft, Netflix). Daily price data was collected from Jan 1st 2020 to Sept 28th 2022. Cryptocurrency data is collected from coinmarketcap.com. This is broadly in line with recommendations in Vidal-Tomás (Citation2022) following concerns raised in Alexander and Dakos (Citation2020). Stock price data is collected from Yahoo finance. All prices are denominated in US dollars. We then used the methods outlined in Section 2 to compare cryptocurrencies with tech stocks and national currencies. Summary statistics for this data are shown in Table .
Table 1. Summary statistics of collected data
3.1. Distributional results
Results based on distributional measures of distance are shown in Tables . Both sets of results confirm previous suggestions of cryptocurrencies being an inherently speculative asset (Baeck & Elbeck, Citation2015; Selgin, Citation2015). Results suggest that unless a cryptocurrency is explicitly pegged to the US Dollar they are inherently speculative in nature. Only the stable coins Binance USD, USD Coin and USD Tether more closely resemble a conventional currency. In contrast, a better comparison for crypto assets would appear to be tech stocks. Summary statistics shown in Table also suggests a similar conclusion.
Table 2. Suggested categorisation of cryptocurrencies based on Kolmogorov-Smirnov distance
Table 3. Suggested categorisation of cryptocurrencies based on Cramér-von Mises distance
The above notwithstanding concerns have been raised about the excessive volatility of stablecoins (Ante et al., Citation2023). A concern is that stablecoins may, unintentionally, increase speculative trading in Bitcoin (Hoang & Baur, Citation2021). Stablecoins may also share the same vulnerabilities as other crypto assets. These include a propensity to crash, concerns over their practical usage as a medium of exchange and their ultimate vulnerability to self-fulfilling perceptions of intrinsic worth (Briola et al., Citation2023).
3.2. Time series results
The time series measures of distance examined in Section 3.2 require the order of the GARCH model to be estimated via forward selection (see e.g. Fry & Burke, Citation2022). Results are summarised in Table . Results for the time series measures of distance constructed are shown in Tables . When viewed from a time series perspective volatility fluctuations suggest only Bitcoin can be viewed as a genuine currency. Results thus reinforce wider concerns raised regarding the excessive volatility of stablecoins (Ante et al., Citation2023; Briola et al., Citation2023; Hoang & Baur, Citation2021).
Table 4. Order of the fitted GARCH models chosen using forward selection
Table 5. Suggested categorisation of cryptocurrencies based on the GARCH distance measure shown in EquationEquation (3)(3)
(3)
Table 6. Suggested categorisation of cryptocurrencies based on the GARCH distance measure shown in EquationEquation (4)(4)
(4)
4. Robustness checks
As a robustness check we repeat the same analysis but replaced the ten Fin Tech stocks with ten major stock indices. In particular the stock indices we analyse are the S&P 500, DJIA, Nasdaq, DAX, CAC 40, Nikkei, Hang Seng, SSE Composite Index, Shenzhen Index and Bel 20. Results for the distributional measures of distance are shown in Tables . Results for the time series measures of distance are shown in Tables . Results lead to broadly the same conclusions as Section 4 if the tech stocks are replaced by stock indices. Results again classify cryptocurrencies as primarily speculative assets with only Bitcoin and Solana seen as genuine currencies if time series measures of distance are used.
Table 7. Suggested categorisation of cryptocurrencies based on Kolmogorov-Smirnov distance
Table 8. Suggested categorisation of cryptocurrencies based on Cramér-von Mises distance
Table 9. Suggested categorisation of cryptocurrencies based on the GARCH distance measure shown in EquationEquation (3)(3)
(3)
Table 10. Suggested categorisation of cryptocurrencies based on the GARCH distance measure shown in EquationEquation (4)(4)
(4)
5. Conclusions
This paper undertakes a taxonomic analysis of leading cryptocurrencies based on traditional ways of analysing financial asset returns. We develop four new methods to classify cryptocurrencies based on either their distributional or their time series properties. From a distributional perspective unless pegged to the US Dollar cryptocurrencies more closely resemble a tech stock. However, time series measures more clearly classify cryptos as primarily speculative assets. The only exceptions are Bitcoin and possibly Solana. Results reinforce wider concerns raised in the literature regarding the excessive volatility of stablecoins (Ante et al., Citation2023; Briola et al., Citation2023; Hoang & Baur, Citation2021).
The implications of this study are that despite economic motivations to the contrary (Dowd, Citation2014) cryptocurrencies continue to be more of a speculative asset than a genuine currency (Baeck & Elbeck, Citation2015; Selgin, Citation2015). Stablecoins seem to share many of the vulnerabilities associated with other crypto assets (Briola et al., Citation2023). Results suggest that although Bitcoin seems to have achieved a degree of traction crypto investors should tread carefully.
Acknowledgments
The authors would like to acknowledge helpful and supportive comments from the editor and from two anonymous referees. Advice and suggestions from Matt Burke are also gratefully acknowledged. The usual disclaimer applies.
Disclosure statement
No potential conflict of interest was reported by the authors.
Notes
1. Evidence of the sufficiency of low-order GARCH models for the data collected for this study is presented below in Table .
References
- Al-Yahyaee, K. H., Mensi, W., & Yoon, S. M. (2018). Efficiency, multifractality, and the long-memory property of the Bitcoin market: A comparative analysis with stock, currency, and gold markets. Finance Research Letters, 27, 228–9. https://doi.org/10.1016/j.frl.2018.03.017
- Alexander, C., & Dakos, M. (2020). A critical investigation of cryptocurrency data and analysis. Quantitative Finance, 20(2), 173–188. https://doi.org/10.1080/14697688.2019.1641347
- Ante, L., Fiedler, I., Willruth, J. M., & Steinmetz, F. (2023). A systematic literature review of empirical research on stablecoins. FinTech, 2(1), 34–47. https://doi.org/10.3390/fintech2010003
- Baeck, C., & Elbeck, M. (2015). Bitcoins as an investment or speculative vehicle? A first look. Applied Economics Letters, 22(1), 30–34. https://doi.org/10.1080/13504851.2014.916379
- Baur, D. G., Hong, K., & Lee, A. D. (2018). Bitcoin: Medium of exchange or speculative assets? Journal of International Financial Markets, Institutions and Money, 54, 177–189. https://doi.org/10.1016/j.intfin.2017.12.004
- Briola, A., Vidal-Tomás, D., Wang, Y., & Aste, T. (2023). Anatomy of a stablecoin’s failure: The Terra-Luna case. Finance Research Letters, 51, 103358. https://doi.org/10.1016/j.frl.2022.103358
- Buterin, V. (2022). Proof of stake. The making of Ethereum and the philosophy of blockchains. Harper Collins.
- Cont, R. (2001). Empirical properties of asset returns: Stylized facts and statistical issues. Quantitative Finance, 1(2), 223–236. https://doi.org/10.1080/713665670
- Corbet, S., Hou, Y. G., Hu, Y., Larkin, C., & Oxley, L. (2020). Any port in a storm: Cryptocurrency safe-havens during the covid-19 pandemic. Economics Letters, 194, 109377. https://doi.org/10.1016/j.econlet.2020.109377
- Corbet, S., Lucey, B., & Yarovaya, L. (2018). Datestamping the bitcoin and ethereum bubbles. Finance Research Letters, 26, 81–88. https://doi.org/10.1016/j.frl.2017.12.006
- Corbet, S., Meegan, A., Larkin, C., Lucey, B., & Yarovaya, L. (2018). Exploring the dynamic relationships between cryptocurrencies and other financial assets. Economics Letters, 165, 28–34. https://doi.org/10.1016/j.econlet.2018.01.004
- Doan, B., Pham, H., & Nguyen Thanh, B. (2022). Price discovery in the cryptocurrency market: Evidence from institutional activity. Journal of Industrial and Business Economics, 49(1), 111–131. https://doi.org/10.1007/s40812-021-00202-0
- Dowd, K. (2014). New private monies. A bit-part player?. Institute of Economic Affairs.
- Fry, J. (2018). Booms, busts and heavy-tails: The story of Bitcoin and cryptocurrency markets? Economics Letters, 171, 225–229. https://doi.org/10.1016/j.econlet.2018.08.008
- Fry, J., & Cheah, E. T. (2016). Negative bubbles and shocks in cryptocurrency markets. International Review of Financial Analysis, 47, 343–352. https://doi.org/10.1016/j.irfa.2016.02.008
- Fry, J. M., & Burke, M. (2022). Quantitative methods in finance using R. Open University Press.
- Gkillas, K., Katsiampa, P., Konstantatos, C., & Tsagkanos, A. (2022). Discontinuous movements and asymmetries in cryptocurrency markets. European Journal of Finance, (forthcoming), 1–25. https://doi.org/10.1080/1351847X.2021.2015416
- Hansen, P. R., & Lunde, A. (2005). A forecast comparison of volatility models: Does anything beat a GARCH(1, 1)? Journal of Applied Econometrics, 20(7), 873–889. https://doi.org/10.1002/jae.800
- Hoang, L. T., & Baur, D. G. (2021). How stable are stablecoins? European Journal of Finance, (forthcoming), 1–17. https://doi.org/10.1080/1351847X.2021.1949369
- Jiang, Y., Nie, H., & Ruan, W. (2018). Time-varying long-term memory in Bitcoin market. Finance Research Letters, 25, 280–284. https://doi.org/10.1016/j.frl.2017.12.009
- Katsiampa, P. (2017). Volatility estimation for Bitcoin: A comparison of GARCH models. Economics Letters, 158, 3–6. https://doi.org/10.1016/j.econlet.2017.06.023
- Kayal, P., & Rohilla, P. (2021). Bitcoin in the economics and finance literature: A survey. SN Business & Economics, 1(7), 88. https://doi.org/10.1007/s43546-021-00090-5
- López-Martin, C., Benito Muela, S., & Arguedas, R. (2021). Efficiency in cryptocurrency markets: New evidence. Eurasian Economic Review, 11(3), 403–431. https://doi.org/10.1007/s40822-021-00182-5
- Selgin, G. (2015). Synthetic commodity money. Journal of Financial Stability, 17, 92–99. https://doi.org/10.1016/j.jfs.2014.07.002
- Urquhart, A. (2016). The inefficiency of Bitcoin. Economics Letters, 148, 80–82. https://doi.org/10.1016/j.econlet.2016.09.019
- Vidal-Tomás, D. (2022). Which cryptocurrency data sources should scholars use? International Review of Financial Analysis, 81, 102061. https://doi.org/10.1016/j.irfa.2022.102061