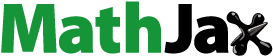
Abstract
Recently worldwide Islamic finance has gained considerable attention. However, Islamic financial institutions face multiple risks to sustaining and growing further. Against this backdrop, the paper examines the impact of both liquidity and credit risk on the efficiency of Islamic banks (IBs) operating in Bangladesh. This paper uses IB’s data from 2007 to 2018 and offers a two-stage assessment. In the first stage, it uses data envelopment analysis (DEA), and in the second stage regression models to assess the impact of both liquidity and credit risk on the efficiency of the IBs. Efficiency scores confirm that IBs are operating with an 86% efficiency level through a 68% share in the constant returns to scale (CRS). Our results also confirm that both liquidity risk (LR) and credit risk (CR) have a significant impact on the efficiency of the IBs in Bangladesh. A higher score for efficiency is shown by a higher liquidity risk, whereas mixed results are confirmed by credit risk indicators. Moreover, the Z-score (a bank stability measurement) and number of branches (a measurement of the bank’s network coverage), have a positive impact on efficiency. On the other side, the size of the bank and the financial crisis period show a negative relationship with the bank’s efficiency. The findings of our paper significantly contribute to the Islamic banking sector, especially for the policymakers and academic researchers.
Introduction
Banking is one of the fundamental elements of financial systems and is crucial to the growth of the economy (Batten & Vo, Citation2019). Banking directly and widely impacts the overall monetary system and economic development. However, the banking systems are not well established in underdeveloped and developing countries, like developed ones. The majority of borrowers in developing nations lack access to the capital market; hence, banking is also described as the “blood vessels” of these economies for obtaining finance. Moreover, the recent downturn in the financial system is a strong reminder that the banking industry needs to keep an eye on its health to find out what’s wrong and what could be done to resolve it. Therefore, particular attention has been paid to finding out the drivers of financial uncertainty linked to the economy and banking system as well (Agnello & Sousa, Citation2012). Accordingly, researchers are giving efforts on identifying the influencing factors of the bank’s performance so that they can suggest to the policymakers regarding the improvement of the financial system (Agnello & Sousa, Citation2012).
Banks are facing multiple financial risks such as liquidity risk (LR), credit risk (CR), operational risk, and interest rate risk (Ferreira et al., Citation2019). Among these risks, credit risk and liquidity risk are key players and are closely related to banking operations (Cecchetti et al., Citation2006; Saleh et al., Citation2020). As such, recent studies on the banks in the Middle East and North Africa (MENA) region show that both LR and CR can separately and jointly influence bank instability (Abdelaziz et al., Citation2022; Cecchetti et al., Citation2006; Ghenimi et al., Citation2017; Rashid et al., Citation2020). Moreover, banks that have high levels of credit risk or liquidity risk may experience increased loan losses or difficulty in meeting financial obligations, which can weaken their financial position, damage their reputation, decrease customer confidence, and ultimately impact their profitability and viability.
IB’s liquidity risk sources are different from those faced by conventional banks (CBs). Such as, first, investment venues of IBs are limited. However, they have to pay profits on their collected deposit, which produces liquidity risk. Second, the money market of IBs is restricted; it is difficult for IBs to generate funds during a short-term liquidity crisis. Third, as per regulatory requirements, all banks have to maintain reserves with the central bank, and under Islamic banking law, IBs are not allowed to receive interest from the central bank. Therefore, IBs need to keep more liquid assets in cash (Hassan et al., Citation2019). For the purpose of our study to address those issues, we use different liquidity risk indicators.
Conversely, credit risk usually is the most considerable risk impacting the bank’s performance (Boffey & Robson, Citation1995). The rise in the default loan trend appears to be a significant predictor of credit risk, and this has gained significant attention, given the linkage between CR and financial instability (Porath, Citation2006). IBs credit risk is linked with the asset side of the balance sheet as CBs. However, in the PLS (profit-loss sharing) mode (Musharakah and Mudarabas), CR arises due to a lack of collateral supported by asymmetric information and moral hazard involved with such loans (Investments) (Ashraf et al., Citation2016).
We consider the impact of LR and CR on the efficiency of IBs in Bangladesh. As per the modern banking theory of intermediation, banks generate the majority of the liquidity through deposits and then use that liquid fund to generate income (Abdelaziz et al., Citation2022; Partovi & Matousek, Citation2019). Bank efficiency plays a crucial role in the smooth movement of funds from the depositors to the borrowers through the banking channel. This transformation is connected with both liquidity and credit risk. So, it is necessary to identify whether and how the credit and liquidity risks are influencing the efficiency of the bank to ensure its stability of the bank.
We select the Islamic banking sector of Bangladesh, as it represents a significant part of the Bangladeshi banking industry. According to the articulation of the central bank (Bangladesh Bank), the IBs have continued to show strong growth since their inception, as reflected by the increasing market share of Islamic banking in terms of assets, financing, and deposits of the total banking system. In addition, IBs in Bangladesh are also performing a key role in reducing poverty compared to conventional banking through different initiatives (Parvej et al., Citation2020). Accordingly, a particular concentration is required to identify the trade-off between these major financial risks and the efficiency of this vital sector. Figure shows the consistent contribution of IBs in the banking industry of Bangladesh over the selected time period. The average share in deposits was 22.65% over the period, with a minimum of 21.08% in the 2nd quarter of 2015. On the other side, the average share of credit/loan (investment) over time was 22.87%. In the case of liquidity, the share of IBs decreased over time with some ups and downs. The average share was 8.7%.
Figure 1. Share of the Islamic banking industry in the overall banking industry.
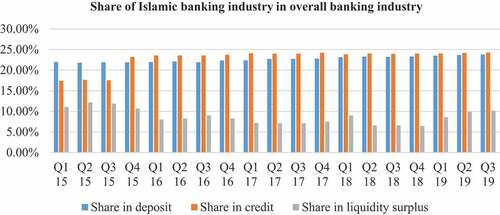
In this paper, we intend to obtain a comprehensive assessment of the liquidity and credit risk of Bangladeshi IBs, as well as their effect on efficiency over a period of 12 years. To do this, at first, we employ the DEA method to get the efficiency of the IBs. Every IB’s efficiency has been calculated using the non-parametric DEA model. Then, we examine the influence of both liquidity and credit risk on IBs efficiency over the same period via OLS, robust regression, fixed effect, and random effect models. Later to check the robustness, we extended our model with some additional control variables. We also employ two stages of least squares (2SLS) and Panel corrected Standard Errors to remove the endogeneity problem. We exclude listed commercial banks with few Islamic banking branches or windows, which may create selection bias. To minimize the possible selection bias in our study, we employ two stages of the Heckman selection model, also used in another recent study in this area (T. Q. Le et al., Citation2020).
Findings confirm that both liquidity and credit risk have a significant impact on the efficiency of the IBs in Bangladesh. The efficiency scores indicate that IBs operate at an 86% efficiency level, indicating that there is still a 14% space for improvement. The efficiency scores also increased from past years to recent years, such as the highest efficiency of the IBs in 2018 and the lowest in 2007. Moreover, 68% are operating at the constant returns to scale (CRS). We also find that efficient IBs tend to be riskier with liquidity to remain efficient over time, supported by the positive impact of both liquidity risk indicators on the efficiency of IBs. However, the impact of the credit risk on IBs efficiency varies with the nature of the indicators. Inefficient banks are facing a higher amount of non-performing loans (investments), and as they lose control over their fund, they can maintain lower loan (investment) loss provisions. Moreover, Z-score (bank stability indicator) shows a positive impact on IBs efficiency, which indicates that inefficient banks have poor financial stability with a higher risk of default. Two-stage Heckman model indicates that the selection bias does not influence our results.
Our work fills the gaps in the current literature on the comprehensive assessment of the efficiency of the IBs over a longer period and also the impact of liquidity and credit risk on the changing efficiency of the IBs operating in Bangladesh. Where other researchers observe the impact of these two risks on bank performance by considering profitability or other indicators proxying to bank performance. Our results are consistent with multiple previous studies, where they find that well-performing banks want to reduce their credit risk and take higher liquidity risk such as Nitoi et al.(Citation2016); Tesfay (Citation2016); Tan and Floros (Citation2018); Abdelaziz et al. (Citation2022). However, there are some contradictions with some existing research (for example, Sufian and Kamarudin (Citation2015); Zeineb and Mensi (Citation2018)), where they mentioned that a higher risk-taking tendency is the nature of the efficient and stable banks. Our findings are robust for the banking sector of Bangladesh and have policy implications for the regulators, top management, and shareholders to understand the risk-return trade-off of the IBs with the change in the efficiency level and take precautionary actions against the prospective credit loss. For robustness and removing endogeneity, we use several consecutive tests and offer inclusive evidence of evaluation.
The remainder of this paper is organized as follows: Section 2 looks at the literature and develops research hypotheses. Section 3 explains the data and methodology of the study. Section 4 describes the results. Finally, section 5 concludes the paper.
Literature review and hypotheses development
The literature review part has been subdivided into four parts. In the first part, the theoretical background of liquidity and credit risk has been discussed. In the second part, research on the effect of LR and CR on the performance of banks, as measured by profitability ratios, is shown. In the third portion of the literature review, we provide a synopsis of the research pertaining to the efficiency of banks. In addition, the literature gap and the development of the hypothesis are displayed in the last section.
The theoretical literature of liquidity risk and credit risk
Both LR and CR have an unavoidable impact on bank performance. The impact of both risks on banking is based on the three major theories of banking. In the first theory, banks are considered the institution that serves loans (investments) by collecting from customers, known as the “Financial intermediation theory of banking” (Bryant, Citation1980; Yahaya et al., Citation2022). Whereas, in the second theory, it has been outlined that money can only create by multiple deposit expansion, and the bank as an intermediary has no control over this creation process, called as “Fractional reserve theory of banking” (Ravn, Citation2022; Werner, Citation2016). Finally, the third theory, the “Credit creation theory of banking”, rejected the previous one and argued that the bank could create money by extending credit (Werner, Citation2016). The first theory is dominant in recent times and this theory points out the role of the bank as an intermediary where the bank collects money from the depositors to maintain liquidity and then use those funds for loan (investment) or credit to generate profit. This theory develops a link between liquidity and credit risk. The second theory depicted that the lower the fraction of reserve the higher the capacity of the banks to create new credit and which will again create new deposits. This theory is again linked with our study as we use the deposit and loan (investment) creation process in measuring the efficiency (input and output variables) of the banks. Where the first two theory supports the bank as an intermediary institution, the third theory is at odds with the previous two theories and said a bank need not depend on a deposit to create a loan (investment) or credit, however, by providing a loan (investment) it again creates deposits. So, the third theory also helped us to identify the role of managing the quality of credit to have a sufficient amount of liquidity in terms of deposits.
In Commercial Bank, liquidity refers to the Bank’s capacity to meet all its financial commitments when required. Such obligations comprise providing loans (investments) (investments), deposit and matured liability withdrawals, and investments during the bank’s normal operations (Amengor, Citation2010). LR arises when banks lack adequate liquid funds (İ̇ncekara & Çetinkaya, Citation2019). On the other hand, a bank’s biggest source of earnings is a loan (investment) or credit (Abdelaziz et al., Citation2022; Kargi, Citation2011). However, credit exposes the banks to loan (investment) default or CR. The Basel committee indicated CR as to the probability of borrowers not reimbursing the loan (investment) in full or in part. LR considers the “profit lowering cost” where a problem loan (investment) or loan (investment) default enhances this LR due to the reduced and blocked cash inflow and the allowances it requires against the increasing amount of credit risk (Dermine, Citation1986). Many studies support that CR enhances LR, which leads to bank defaults such as Imbierowicz and Rauch (Citation2014), mentioned that nearly half of 254 commercial banks failed because the CR pushes the LR between 2007 and 2010 in the USA.
There are three hypotheses found which show the connection between the bank’s risk and the bank’s performance. One of these hypotheses is the moral hazard hypothesis mentioned by Jeitschko and Jeung (Citation2005), which indicates that, due to the information gap and agency problems, managers of less efficient or inefficient banks try to take higher risks. Another hypothesis is the “bad management or bad luck” hypothesis (Berger & DeYoung, Citation1997), which suggests that credit risk increases due to poor monitoring by inefficient banks. However, another hypothesis, the “efficient market” hypothesis (Fama, Citation1965), suggests that an increase in the LR or CR subsequently reduces the market share price, further enhancing the bank’s default probability.
The influence of liquidity and credit risk on bank performance
While searching for the impact of LR and CR on the efficiency of the bank, we find that most of the researchers focus on the impact on bank performance measured by other indicators, like ROA, ROE, and profitability ratios. Table shows a summary of different literature on LR and CR with the performance of both IBs and Conventional banks (CBs). IBs use almost the same functional mechanism as CBs to meet the stakeholders’ demand; however, there is a difference in the contractual structures of loans (investments) and deposits (Van Greuning & Iqbal, Citation2008).
Table 1. Summary of the literature showing the relation of the liquidity and credit risk with bank performance
Earlier most of the researchers used other profitability indicators like ROA, ROE, and net interest margin (NIM) instead of bank efficiency to measure the performance of the bank (Ghenimi et al., Citation2017; Golubeva et al., Citation2019; Hakimi & Zaghdoudi, Citation2017). They find a significant impact of both types of risks on bank performance. Results are summarized in Table . One group of researchers find that there is a positive relationship between these risks and bank performance. They reveal that higher CR-taking banks are targeting to get more profit by charging higher interest on risky credit customers. They also suggest that banks take liquidity risk by using most of their liquid funds to distribute credit and earn more profit, as liquid funds or assets are not profit-generating investments. Another group finds a negative relationship between these two risks and bank performance. Their justification is that banks with lower credit risk can also generate increasing income by improving loan (investment) quality. They also point out that banks facing liquidity crises need to manage liquid funds with a higher cost, which reduces their earnings. Considering these research works, we can also assume that CR and LR can influence bank efficiency as like other indicators of bank performance.
Literature on efficiencies of banks around the world
Efficiency is a good measurement of performance compared to other profitability ratios. As efficiency scores under DEA technique employ multiple inputs and outputs, the efficiency of the banking sector is necessary to ensure a sustainable and sound banking system both in developing and developed countries, as it improves financial stability (Blejer, Citation2006). Banking efficiencies literature concentrating on developing countries is small in number. In recent decades, there has been rising literature on bank efficiencies in developing countries (Banna et al., Citation2017; Batten & Vo, Citation2019; T. D. Le et al., Citation2022).
As IBs become more relevant each year, as such, a large volume of research studies is focusing on the comparison of IBs and CBs such as Akram and Ur Rahman (Citation2018), Ferhi (Citation2018), Musa et al. (Citation2020). Besides the comparison, some studies separately examined determinants of bank efficiency. Among these, most of the studies are done on CBs. For example, T. D. Le et al. (Citation2022), Adeabah et al. (Citation2018), Rekik and Kalai (Citation2018). However, recently researchers are now also focusing on the determinants (including CR and LR) of the efficiency of IBs (Endri et al., Citation2022; Mezzi, Citation2018; Rehman et al., Citation2022). Table shows a summary of the recent literature on efficiency and its determinants around the world, where a two-stage methodology like this research has been employed. Here, the researchers also find both positive and negative relationships between CR, LR, and bank efficiency, as with bank performance measured by profitability ratios. All this research considers CR and LR as control variables or explanatory variables not the main variables of the model. Moreover, only two papers use the advanced technique to remove endogeneity (T. D. Le et al., Citation2022; Tan & Floros, Citation2018); however, these are based on conventional banking data.
Table 2. Efficiency and its determinants around the world
In the case of Bangladesh, research works on bank efficiency are very few. Moreover, most of the studies are conducted on measuring the comparative efficiency of the CBs and IBs (Haque & Sohel, Citation2019; Islam & Kassim, Citation2015; Jahan, Citation2019; Nabi et al., Citation2019). They only compare the efficiency level of IBs with CBs and do not go for the second-stage analysis to check the influencing factor of efficiency. However, some of the studies try to find out the determinants of the efficiency of commercial banks, which consider only CBs or combine both CBs and IBs (Chaity & Islam, Citation2021; Miah & Sharmeen, Citation2015; Rashid et al., Citation2020; Zheng et al., Citation2022). They try to identify the impact of size, corporate social responsibility, corporate governance, and earnings on the efficiency of the banks. Moreover, they do not check the impact of those factors separately on IBs. Recently, Samad (Citation2019) uses the data of IBs only, from 2008 to 2012 and find that capital adequacy and the number of branches have a positive impact on efficiency. This indicates that a higher amount of capital and branches can enhance the earning capacity of the IBs. They find a negative impact of poor loan (investment) quality, higher liquidity claims and bank size. This suggests that a higher amount of poor loans (investments) and liquidity crisis can reduce the efficiency of the IBs complying with the “efficient market hypothesis” and “bad management hypothesis”. Limitations of that study are that it uses only five years of data, not considers other indicators of LR or CR and not also removed the endogeneity problems. From here, we can summarize that still there is a significant gap in the literature of the efficiency of IBs in connection with CR and LR.
Literature gap and hypotheses to be tested
Ikra et al. (Citation2021) in their recent review paper that works on the bibliometric analysis of the literature on the efficiency of IBs from 2000 to 2020, also find that there is a huge gap in the existing literature related to the efficiency of IBs. In the previous two sections, existing research has been divided into two parts to link with the objective of our study. In summary of the above two sections, we have identified some gaps in the existing works. Firstly, much research has been conducted on determinants of a bank’s performance, measured by profitability ratios instead of efficiency scores. Secondly, only a little research has been conducted on the factors affecting the efficiency of banks, and this research focuses mostly on CBs or comparisons between CBs and IBs. In this paper, we focus on only IBs. Then, we examined the impact of LR and CR on the efficiency of IBs, which was not done in previous studies related to Bangladesh. Additionally, previous studies on Bangladesh are based on the shorter dataset and did not remove the endogeneity problem also. To fill the gap in the existing literature regarding the impact of CR and LR on the bank’s efficiency of IBs in Bangladesh, we have identified the following hypotheses:
Hypothesis 1:
An increase in liquidity risk impacts bank efficiency negatively.
The “efficient market” hypothesis by Fama (Citation1965) is closely related to our first hypothesis. As the forward-looking information is incorporated into the stock prices, both will find a way to impact the price of the shares for increasing the default probability of the bank in the event of high liquidity and credit crisis. Such a rise in the bank default probability will transfer to the inefficiency of the bank. Moreover, Allen and Gale (Citation2000) identified that liquidity risk arises from the bank’s liability side, such as demand or current deposit, and also the reason behind the bank run.
Hypothesis 2:
An increase in credit risk impacts the bank’s efficiency negatively.
This hypothesis is also closely connected with the “bad luck” or “bad management” hypothesis. It has been mentioned that increased bank risk enhances the bank default probability, and further impact is working less efficiently by the employees (Berger & DeYoung, Citation1997). This is because, in such a risky situation, bank managers need to divert their attention towards precautionary activities for reducing the credit risk instead of doing their regular operational activities. Such actions by the managers will further deteriorate their financial and working capacity. Moreover, in extreme situation, the bank has to bear the dear cost of managing their soundness and safety to the supervisory authority. In both situations, one would expect higher costs to increase bank inefficiency, triggered by an increase in bank default risks (Saeed & Izzeldin, Citation2016).
Research method and data description
Methods
In this study, we employ a two-stage Data Envelopment (DEA) approach to examine the impact of CR and LR on the efficiency of the IBs in Bangladesh. In the first stage, we measure the efficiency of IBs and, in the second stage, we examine the impact of CR and LR on the efficiency scores derived in the first stage. This two-stage approach has also been used by other researchers (Nitoi et al., Citation2016; T. D. Le et al., Citation2022).
First stage analysis: measurement of efficiencies
Evaluating the efficiency of commercial banks to monitor their financial condition has become a popular topic for researchers since the initial works by Benston (Citation1965) and Greenbaum (Citation1967). DEA has been commonly used to evaluate the relative effectiveness of a collection of bank branches with common operational goals with unequal inputs and outputs (Boubaker et al., Citation2022; Saranga & Moser, Citation2010; T. D. Le et al., Citation2022).
Accordingly, to test the DMU (decision-making units) and selected banks, we use the non-parametric approach DEA. In the initial DEA model, the CCR (Charnes, Cooper and Rhodes) assumes that efficiency has no significant relation with the scale of operations (Charnes et al., Citation1978). In the extended DEA model, BCC (Banker, Charnes and Coope) introduces variable returns to scale (VRS) by reducing constant returns to scale’s (CRS’s) assumption (Banker et al., Citation1984). Under this model, there are three efficiency scores: technical efficiency (TE), pure technical efficiency (PTE), and scale efficiency (SE). PTE is measured as the measurement of TE in the absence of SE. If there seems to be a disparity between a particular DMU’s TE and PTE ratings, it represents the presence of the scale’s inefficiency. As the CRS completely ignores the size of operations, it would likely lead to very unrealistic targets we use VRS in our study (Munksgaard et al., Citation2005).
DEA analysis has two main types of orientation: input-oriented and output-oriented (Dinçer et al., Citation2019). The input orientation considers the outputs as fixed, and the inputs are reduced, whereas output orientation considers the input fixed and increases the outputs (Cooper et al., Citation2007). It has been well established that in the competitive market, DMUs are output-oriented (Barros et al., Citation2015). Therefore in our study, we use output orientation. The DEA’s output-oriented BCC model can be formulated in the following Equationequation (1)(1)
(1) following the work by Emrouznejad and Tavana (Citation2013).
max h
here h indicates the relative efficiency of the output of the kth DMU. ykj indicates ith outputs of the kth DMUs. xkj indicates the jth inputs of the kth DMU. λl used to indicate the virtual multiplier of the lth DMU. J represents the outputs, and I represent the inputs, and K indicates the DMUs.
Second stage analysis: model specification
We regress efficiency scores (TE, PTE, and SE) against LR and CR indicators in the second stage. Here at first, we use only one control variable, z-score, to control the effect of the bank’s insolvency risk or financial stability suggested in the study by Tan and Floros (Citation2018). Under this model, we employ OLS then we employ White’s Robust-standard error, random effect model, and fixed effect model for getting additional outputs. Later, for checking the robustness, we incorporate some additional control variables age of the banks, size of the banks, and crisis period. Here, we also use OLS, FE, and RE. Later on, to avoid the endogeneity that may arise due to the correlation between CR and LR indicators with the error term, we employ two-stage least squares (2SLS) and Panel-Corrected Standard Errors (PCSE).
The models used in our study are developed on the basis of the works by Mia and Soltane (Citation2016); T. D. Le et al. (Citation2022), and so on.
where i represents IBs, t represents time, LR represents the liquidity risk, Effic represents the efficiency score of the banks, CR represents the credit risks, Z score represents the insolvency risk or financial stability of the bank, is for the age of the bank,
representing the number of branches and
is for crisis period (2007–2009) dummy. In equation 4,
is for control variables, which include Z score and Crisis. In equation 6, IVs are instrumental variables, which include Age, Size, ROA, and ROE.
-
are the coefficients of right-hand side variables and
represents the error term. The description and characteristics of all the variables used in this research are given in the next section.
Data description
In this study, we use the recent panel data of seven IBs operating in Bangladesh from 2007 to 2018. All the data are on a yearly basis and obtained from the individual annual report of the respective banks. Currently, 10 IBs are working in Bangladesh, and in our study, we include only seven IBs, which are listed under SEC (Bangladesh Securities and Exchange Commission). The other three banks are not included in our observations as two of them enlisted under the SEC in 2022 and another one converted to an Islamic bank in 2021. In our selected variables, there are no missing data and no negative values. One of the key data criteria used for DEA is that it must not be negative (Sarkis and Weinrach, Citation2001). IBs operated under shariah (Islamic laws) which allows only profit-based investment as an alternative to the interest-based loan for CBs. For simplicity, we use the term “Loan (investment)” in this study which includes the sum of all the types of Islamic modes of investment available in the selected banks.
Specification of input and output variables for efficiency measurement (first stage)
We consider employee expense, deposits, and fixed assets as the input variables. On the other hand, total loans (investments) and other earning assets are selected as the output variables. These variables are incorporated based on the intermediation approach and also used by other scholars (Yilmaz & Güneş, Citation2015). The description of these selected variables for this stage is shown in Table .
Table 3. Inputs and outputs considered in this paper
Variables used in the second stage
We employ two major risks of the banks, liquidity risk, and credit risk, as the main independent variables to show their influence on the banks’ efficiency. We use two measures, loan (investment)-to-deposit ratio and financing gap, as the indicators of liquidity risk. In comparison, we consider three indicators, the amount of non-performing loans (investments), NPL ratio, and loan (investment) loss provision, as the proxy for credit risk. Moreover, we also control insolvency risk or financial stability measured by z-score, age of the bank, bank size (measured by the number of branches), and financial crisis period (2007–2009) proxied by crisis dummy. Later on, we also consider ROA (return on asset), ROE (return on equity), age of the bank, and bank size as instrumental variables in 2SLS. The definitions of all the selected independent variables for the second-stage models are shown in Table .
Table 4. Description of independent variables
Results and discussion
The efficiency of the Islamic banks in Bangladesh
In this section, the efficiency outputs of IBs in Bangladesh are discussed. The efficiency of each year has been examined. It is more flexible to build a unique annual efficiency frontier for every year, and thus it is more reasonable than developing a single multiannual frontier for the selected banks (Isik & Hassan, Citation2002). Therefore, we separate annual frontiers for each year using panel data. One major advantage of panel data is that each bank can be assessed multiple times over the selected period. However, one of the major challenges is that efficient technology in one period can be inefficient in another year due to the continuously changing business environment (Sufian, Citation2009).
Figure depicts the trends of the efficiency scores of all the Bangladeshi IBs over the selected period. Overall, TE was at its peak in 2018 and was at its lowest in 2007. During the selected period, the overall TE was 86.00%. It indicates a mean waste of 14.00% of the inputs used for all the IBs in Bangladesh. Moreover, scale efficiencies dominate the technical efficiencies, as in all the years, PTE is higher than SE. The findings show that while IBs operated on a relatively optimal operating scale during the study time, they were managerially inefficient in making full use of their resources.
Composition of the efficiency frontier
In this segment, we address the scale of IBs source inefficiencies in Bangladesh. A bank can do business under CRS or VRS. After that, a bank operating under VRS can be at DRS (decreasing return to scale) or IRS (increasing returns to scale). The result regarding the frontier is consistent with previous studies (such as Noulas et al. (Citation1990); McAllister and McManus (Citation1993)). Table shows that most of the banks are operating at CRS, and the share is around 68% on average, over the selected period. It indicates that they are operating on the right scale. The implication is that these banks increase their inputs to get a similar amount of output. However, the share of DRS reached a maximum of 43% in 2018 with an average share of 18% over the period, and it implies that these banks may face lower outputs by increasing the inputs. The lowest portion of the RTS (returns to scale) is covered by IRS, which is only 14% on average, over the selected time. This indicates that these banks can get a higher amount of output considering a smaller increase in the inputs.
Table 5. Bangladeshi Islamic banks’ RTS for 2007 = 2018 (percentage share)
Heteroscedasticity test
One of the crucial assumptions for getting the best results is that the modeling errors are uniform and uncorrelated (Goldberger, Citation1970). Table shows the output of the Breusch—Pagan test on three models. Findings suggest that all the selected models have heteroscedasticity, and findings are robust for all models.
Table 6. Breusch-Pagan/Cook–Weisberg test for heteroscedasticity
Factors affecting the efficiency of Islamic banks in Bangladesh
The regression outputs of TE, PTE, and SE are shown in Tables . All the approaches used in each model (Model 1 for TE, Model 2 for PTE, and Model 3 for SE) show the fitness as the p-value of the models is less than 0.05, except for Model 2 (used for PTE). Moreover, a, b, c, and d of each model represents the output from OLS, Robust regression, fixed effect, and random effect, respectively. Considering this, we discuss the outcomes found in Model 1 (used for TE) and Model 3 (used for SE).
Table 7. Model 1 shows the Multivariate regression analysis models considering TE as the dependent variable
Table 8. Model 2 shows the Multivariate regression analysis models considering PTE as the dependent variable
Table 9. Model 3 shows the Multivariate regression analysis models considering SE as the dependent variable
The proxy of bank stability, Z-score, reveals a positive impact (significant at a 5% level) on TE and SE under a fixed effect approach (Model 1 (c) and Model 3 (c)). A higher Z score reflects a lower risk of failure. The result matches our expected sign and is consistent with the study of Nitoi et al.,(Citation2016). The output implies that IBs try to ensure their stability to be efficient. However, it contradicts our expectations and the study done by Zeineb and Mensi (Citation2018), where it has been mentioned that efficient banks try to take more risks to improve their situation.
The loan (investment)-to-deposit ratio and financing gap is used as the proxy of liquidity risk. A higher loan (investment)-to-deposit ratio (LTD) and financing gap (Fgap) indicates higher liquidity risk (Williams & Nguyen, Citation2005). In maximum models, our findings confirm that there is a positive and significant (at 5% and 10% levels) relation between LTD and F_gap with bank efficiency, measured by TE and SE. Our findings are consistent with the study by Řepková (2015) and Tesfay (Citation2016). Findings suggest that IBs are taking higher liquidity risks to generate a higher amount of output and are reluctant to reduce the liquidity risk. However, this result contradicts Tan and Floros (Citation2018), and our initial expected sign.
The natural logarithm of the amount of non-performing loans (investments) (LNNPL), non-performing loan (investment) to total loan (investment) ratio (NPL ratio), and the natural logarithm of loan (investment) loss provision (LNLLP) are considered as a proxy for the credit risk. Most of the outputs regarding the credit risk indicators from the models show a negative and significant impact on the banks’ efficiency, measured by TE and SE. LNNPL shows a negative and significant impact on bank efficiency in most of the models. This result is consistent with the moral hazard and “bad management hypothesis” and Tan and Floros (Citation2018). This suggests that due to a lack of supervision, information gaps, and agency problems, less efficient or inefficient bank managers attempt to take higher risks. The second indicator, NPL ratio (NPL to total loan (investment)), shows a positive impact on efficiency under one model (Model 2 (b)) only; however, that model is not fit to consider. The third indicator LNLLP shows a positive impact on efficiency. The output is in line with the findings of Rosman et al. (Citation2014). They mentioned that this positive impact might be due to the bank’s more prudent activities by increasing their provision amount when they are becoming more efficient.
Robustness and endogeneity
We check the robustness by adding some additional control variables, age, size of the bank, and financial crisis period. As we are assuming that the age of the bank can influence its efficiency of the bank. A long existence (age) of the bank in the market can give a competitive advantage over the new banks (Ramadhan et al., Citation2022). On the other hand, Gupta et al. (Citation2020) find a negative relationship between age and bank performance and mention that younger banks have more sophisticated technology, which enables them to perform better than older banks. We are also assuming the number of branches also has an influence on bank performance or efficiency. Larger banks can control a larger market through their wide range of branches and can distribute their fixed expenses over a large number of customers (Hauner, Citation2005; T. D. Le et al., Citation2022). Then we employ OLS, FE, and RE. New results also support the previous results; however, it reduces the standard error of the coefficients. The second, third, and fourth columns of Tables show the results of OLS, FE, and RE, respectively.
Table 10. Impact of liquidity risk and credit risk on TE (checking robustness and removing endogeneity)
Table 11. Impact of liquidity risk and credit risk on PTE (checking robustness and removing endogeneity)
Table 12. Impact of liquidity risk and credit risk on SE (checking robustness and removing endogeneity)
To avoid endogeneity, we employ the 2SLS and PCSE techniques on the new model. Endogeneity may arise due to the correlation between independent variables with other variables that are not included in the model. For 2SLS, we need to find instruments with whom independent variables may have a significant relationship. Here, we are assuming that ROA and ROE can influence credit risk and liquidity risk which may change the impact of CR and LR on bank efficiency. In this stage, we are also assuming that age and bank size may not affect bank efficiency directly, rather the effect may transfer through credit risk and liquidity risk. For this indirect effect of age and size, we also consider age and size as instruments. Results of both 2SLS and PCSE also support the main model. Moreover, standard errors of coefficients under the PCSE technique were reduced dramatically. The fifth and sixth columns of Tables are representing the results of 2SLS and PCSE.
Heckman two-stage selection model
In Bangladesh, few CBs have Islamic banking branches or windows; those branches are not included in our observations. So, there is possible selection bias from the missing information. Therefore, we employ the Heckman (Citation1976) selection model to solve this problem. The Heckman selection model outcomes have been depicted in column 7 of Tables , and 9. The coefficient for the inverse Mill’s ratio lambda of the second-stage Heckman selection regression is positive in Table for TE, positive in Table for PTE, and again negative in Table for SE. However, it is not statistically significant in all cases, indicating that the selection bias does not influence the findings.
Hausman test for fixed versus random-effects model
The Husman test output suggests that the random effect approach dominates the fixed effect, as the p-value is more than .05. This result suggest that there is correlation between the error term of one specific time with another time of the same bank, so random effect is superior in this case. Table shows the outcomes of the Husman tests between Model 1 (c) and (d), Model 2 (c) and (d), and Model 3 (c) and (d).
Table 13. Hausman (Citation1978) specification test
Conclusions
Our paper aims to determine the efficiency of IBs and investigate the impact of credit risks and liquidity risks on IBs bank efficiency in Bangladesh between 2007 and 2018, employing a two-stage DEA method. In the first stage, we measure the efficiency scores, TE, PTE, and SE using output-oriented DEA under the BCC model. The impact of credit risk and liquidity risk is examined in the second stage. Findings show that credit risk and liquidity risk can influence the efficiency of IBs in Bangladesh. This thus rejects the “efficiency hypothesis” and confirms that IBs having less liquidity can be more efficient by utilizing the funds in profitable sectors. On the other side, credit risk confirms the “bad management hypothesis” that low credit risk-taking banks can be more efficient by making conservative and selective investments.
Findings also reveal that IBs of Bangladesh are overall 86% efficient, indicating that there is still 14% space for improvement. The efficiency of the IBs highest in 2018 and lowest in 2007. While decomposing the efficiency frontier, it has been found that most of the IBs are operating at CRS (68%), then at DRS (18%), and the lowest portion is covered by IRS (14%). Moreover, we also observe a significant impact of the financial stability measured by the Z-score on individual banks’ efficiency. It suggests that financially stable bank can utilize their resources in a more efficient way to improve their overall efficiency scores.
Furthermore, robustness and endogeneity are major issues related to this type of analysis. However, robustness checks and endogeneity controlling findings also confirm the similar relationship between credit risks, liquidity risks, and bank efficiency of IBs in Bangladesh. Where the age of the bank shows a negative relationship with bank efficiency. This implies that comparatively younger IBs can assure efficient performance, this can be due to their quick adaptability to new technologies. The increasing number of branches has a positive relation with efficiency. This highlights the importance of using a larger network through branches to utilize the resources in an efficient way. The crisis dummy confirms that during the financial crisis period banks are performing inefficiently.
Our study contributes to the existing limited literature in the area of IBs by measuring their efficiency and examining the impact of credit risks and liquidity risks on efficiency. Findings might have importance to the regulators of financial institutions, government policymakers, and bank management. Based on our findings, we have two major suggestions. First, the government and regulators should incorporate regulations to improve the level of liquidity held by IBs, as efficient IBs are taking higher liquidity risks to remain efficient. Second, the risk management unit of the inefficient IBs should work to reduce the NPL amount and maintain the loan (investment) loss provision to remain efficient.
Conversely, our study has a few limitations as well. First, we have considered only SEC-listed seven IBs; many banks are doing Islamic banking alongside their conventional banking. Our selected banks may cover most of the Islamic banking segment; however, they may not cover Bangladesh’s entire Islamic banking segment. Second, as Bangladesh is a developing country, the findings from this study may not be appropriate for developed countries. Third, while credit risk and liquidity risk are important risks in Islamic banking, it should be noted that other types of risks, such as market risk, operational risk, Shariah risk, legal and regulatory risk, strategic risk, and concentration risk, have not been explored in this article. Further research is needed to fully understand the risks involved in Islamic banking. Finally, although most of the liquidity and credit risk indicators are considered, some other indicators of liquidity risk, such as LCR (liquidity coverage ratio), net stable fund ratio, and liquid assets to total loans (investments), are not considered. Future studies can be extended in multiple ways by considering these limitations. Researchers may conduct their research by comparing the influence of liquidity and credit risk on efficiency between IBs and CBs. Additionally, a cross-country analysis can also be performed to compare developed and developing countries’ outputs.
Disclosure statement
No potential conflict of interest was reported by the authors.
References
- Abdelaziz, H., Rim, B., & Helmi, H. (2022). The interactional relationships between credit risk, liquidity risk and bank profitability in MENA region. Global Business Review, 23(3), 561–23. https://doi.org/10.1177/0972150919879304
- Adeabah, D., Gyeke Dako, A., & Andoh, C. (2018). Board gender diversity, corporate governance and bank efficiency in Ghana: A two-stage data envelope analysis (DEA) Approach. SSRN Electronic Journal. https://doi.org/10.2139/ssrn.3312103
- Adusei, M., McMillan, D., McMillan, D., & McMillan, D. (2016). Determinants of bank technical efficiency: Evidence from rural and community banks in Ghana. Cogent Business & Management, 3(1), 0. https://doi.org/10.1080/23311975.2016.1199519
- Agnello, L., & Sousa, R. M. (2012). How do banking crises impact on income inequality? Applied Economics Letters, 19(15), 1425–1429. https://doi.org/10.1080/13504851.2011.631885
- Ajibike, J. O., & Aremu, O. S. (2015). The impact of liquidity on Nigerian bank performance: A dynamic panel approach. Journal of African Macroeconomic Review, 5(2), 23–41.
- Akram, H., & Ur Rahman, K. (2018). Credit risk management: A comparative study of Islamic banks and conventional banks in Pakistan. ISRA International Journal of Islamic Finance, 10(2), 185–205. https://doi.org/10.1108/IJIF-09-2017-0030
- Allen, F., & Gale, D. (2000). Financial contagion. Journal of Political Economy, 108(1), 1–33. https://doi.org/10.1086/262109
- Alshatti, A. S. (2015). The effect of the liquidity management on profitability in the Jordanian commercial banks. International Journal of Business & Management, 10(1), 62. https://doi.org/10.5539/ijbm.v10n1p62
- Amengor, E. C. (2010). Importance of liquidity and capital adequacy to commercial banks. A Paper Presented at Induction Ceremony of ACCE, UCC Campus,
- Ashraf, D., Rizwan, M. S., & L’Huillier, B. (2016). A net stable funding ratio for Islamic banks and its impact on financial stability: An international investigation. Journal of Financial Stability, 25, 47–57. https://doi.org/10.1016/j.jfs.2016.06.010
- Azad, M., Kalam, A., Munisamy, S., Masum, A. K. M., Saona, P., & Wanke, P. (2017). Bank efficiency in Malaysia: A use of malmquist meta-frontier analysis. Eurasian Business Review, 7(2), 287–311. https://doi.org/10.1007/s40821-016-0054-4
- Banker, R. D., Charnes, A., & Cooper, W. W. (1984). Some models for estimating technical and scale inefficiencies in data envelopment analysis. Management Science, 30(9), 1078–1092. https://doi.org/10.1287/mnsc.30.9.1078
- Banna, H., Ahmad, R., & Koh, E. H. (2017). Determinants of commercial banks’ efficiency in Bangladesh: Does crisis matter? The Journal of Asian Finance, Economics & Business, 4(3), 19–26. https://doi.org/10.13106/jafeb.2017.vol4.no3.19
- Banya, R., & Biekpe, N. (2018). Banking efficiency and its determinants in selected frontier African Markets. Economic Change and Restructuring, 51(1), 69–95. https://doi.org/10.1007/s10644-016-9200-3
- Barros, C. P., & Athanassiou, M. (2015). Efficiency in European seaports with DEA: Evidence from Greece and Portugal. In Port management (pp. 293–313). Springer.
- Batir, T. E., Volkman, D. A., & Gungor, B. (2017). Determinants of bank efficiency in Turkey: Participation banks versus conventional banks. Borsa Istanbul Review, 17(2), 86–96. https://doi.org/10.1016/j.bir.2017.02.003
- Batten, J., & Vo, X. V. (2019). Determinants of bank profitability—Evidence from Vietnam. Emerging Markets Finance & Trade, 55(6), 1417–1428. https://doi.org/10.1080/1540496X.2018.1524326
- Bayuny, A. F. R., & Haron, R. (2017). Determinants of efficiency of Islamic Banks: Indonesian evidence. Journal of Islamic Finance, 6(1), 1–15. https://doi.org/10.12816/0042729
- Benston, G. J. (1965). Branch banking and economies of scale. The Journal of Finance, 20(2), 312–331. https://doi.org/10.1111/j.1540-6261.1965.tb00212.x
- Berger, A. N., & DeYoung, R. (1997). Problem loans and cost efficiency in commercial banks. Journal of Banking & Finance, 21(6), 849–870. https://doi.org/10.1016/S0378-4266(97)00003-4
- Blejer, M. I. (2006). Economic growth and the stability and efficiency of the financial sector. Journal of Banking & Finance, 30(12), 3429–3432. https://doi.org/10.1016/j.jbankfin.2006.06.001
- Boffey, R., & Robson, G. N. (1995). Bank credit risk management. Managerial Finance, 21(1), 66–78. https://doi.org/10.1108/eb018497
- Boubaker, S., Le, T. D., & Ngo, T. (2022). Managing bank performance under COVID‐19: A novel inverse DEA efficiency approach. International Transactions in Operational Research, 30(5), 2436–2452. https://doi.org/10.1111/itor.13132
- Bryant, J. (1980). A model of reserves, bank runs, and deposit insurance. Journal of Banking & Finance, 4(4), 335–344. https://doi.org/10.1016/0378-4266(80)90012-6
- Cecchetti, S. G., Schoenholtz, K. L., & Fackler, J. (2006). Money, banking, and financial markets. McGraw-Hill/Irwin.
- Chaity, N. S., & Islam, K. Z. (2021). Bank efficiency and practice of earnings management: A study on listed commercial banks of Bangladesh. Asian Journal of Accounting Research, 7(2), 114–128. https://doi.org/10.1108/AJAR-09-2020-0080
- Charnes, A., Cooper, W. W., & Rhodes, E. (1978). Measuring the efficiency of decision making units. European Journal of Operational Research, 2(6), 429–444. https://doi.org/10.1016/0377-2217(78)90138-8
- Cooper, W. W., Seiford, L. M., & Tone, K. (2007). Data envelopment analysis: A comprehensive text with models, applications, references and DEA-solver software. Springer.
- Daly, S., & Frikha, M. (2017). Determinants of bank performance: Comparative study between conventional and Islamic banking in Bahrain. Journal of the Knowledge Economy, 8(2), 471–488. https://doi.org/10.1007/s13132-015-0261-8
- Dermine, J. (1986). Deposit rates, credit rates and bank capital: The Klein-Monti model revisited. Journal of Banking & Finance, 10(1), 99–114. https://doi.org/10.1016/0378-4266(86)90022-1
- Dinçer, H., Hacioglu, U., Tatoglu, E., & Delen, D. (2019). Developing a hybrid analytics approach to measure the efficiency of deposit banks. Journal of Business Research, 104, 131–145. https://doi.org/10.1016/j.jbusres.2019.06.035
- Djan, G. O., Stephen, F., Bawuah, J., Halidu, O. B., & Kuutol, P. K. (2016). Credit risk management and its impact on financial performance of listed banks in Ghana. International Journal of Financial Markets, 2(2), 24–32.
- Ekinci, R., & Poyraz, G. (2019). The effect of credit risk on financial performance of deposit banks in Turkey. Procedia computer science, 158, 979–987. https://doi.org/10.1016/j.procs.2019.09.139
- Emrouznejad, A., & Tavana, M. (2013). Performance measurement with fuzzy data envelopment analysis. Springer.
- Endri, E., Fatmawatie, N., Sugianto, S., Humairoh, H., Annas, M., & Wiwaha, A. (2022). Determinants of efficiency of Indonesian Islamic rural banks. Decision Science Letters, 11(4), 391–398. https://doi.org/10.5267/j.dsl.2022.8.002
- Fama, E. F. (1965). The behavior of stock-market prices. The Journal of Business, 38(1), 34–105. https://doi.org/10.1086/294743
- Ferhi, A. (2018). Credit risk and banking stability: A comparative study between Islamic and conventional banks. International Journal of Law and Management, 60(4), 1009–1019. https://doi.org/10.1108/IJLMA-05-2017-0112
- Ferreira, S., Dickason-Koekemoer, Z., & Papavassiliou, V. (2019). A conceptual model of operational risk events in the banking sector. Cogent Economics & Finance, 7(1), 1706394. https://doi.org/10.1080/23322039.2019.1706394
- Ghenimi, A., Chaibi, H., & Omri, M. A. B. (2017). The effects of liquidity risk and credit risk on bank stability: Evidence from the MENA region. Borsa Istanbul Review, 17(4), 238–248. https://doi.org/10.1016/j.bir.2017.05.002
- Goldberger, A. S. (1970). Econometric Theory, New York, 1964. GoldbergerEconometric Theory1964.
- Golubeva, O., Duljic, M., & Keminen, R. (2019). The impact of liquidity risk on bank profitability: Some empirical evidence from the European banks following the introduction of Basel III regulations. Journal of Accounting and Management Information Systems, 18(4), 455–485. https://doi.org/10.24818/jamis.2019.04001
- Greenbaum, S. I. (1967). Competition and efficiency in the banking system–empirical research and its policy implications. Journal of Political Economy, 75(4, Part 2), 461–479. https://doi.org/10.1086/259318
- Gupta, N., Mahakud, J., & McMillan, D. (2020). Ownership, bank size, capitalization and bank performance: Evidence from India. Cogent Economics & Finance, 8(1), 1808282. https://doi.org/10.1080/23322039.2020.1808282
- Hakimi, A., & Zaghdoudi, K. (2017). Liquidity risk and bank performance: An empirical test for Tunisian banks. Business and Economic Research, 7(1), 46–57. https://doi.org/10.5296/ber.v7i1.10524
- Haque, M. R., & Sohel, M. N. I. (2019). Are Islamic banks less efficient than other banks? Evidence from Bangladesh. Asian Economic and Financial Review, 9(10), 1136–1146. https://doi.org/10.18488/journal.aefr.2019.910.1136.1146
- Hassan, M. K., Khan, A., & Paltrinieri, A. (2019). Liquidity risk, credit risk and stability in Islamic and conventional banks. Research in International Business and Finance, 48, 17–31. https://doi.org/10.1016/j.ribaf.2018.10.006
- Hauner, D. (2005). Explaining efficiency differences among large German and Austrian banks. Applied Economics, 37(9), 969–980. https://doi.org/10.1080/00036840500081820
- Hausman, J. A. (1978). Specification tests in econometrics. Econometrica: Journal of the Econometric Society, 46(6), 1251–1271. https://doi.org/10.2307/1913827
- Heckman, J. J. (1976). The common structure of statistical models of truncation, sample selection and limited dependent variables and a simple estimator for such models. Annals of Economic and Social Measurement. 5(4) 475–492: NBER.
- Ikra, S. S., Rahman, M. A., Wanke, P., & Azad, M. A. K. (2021). Islamic banking efficiency literature (2000–2020): A bibliometric analysis and research front mapping. International Journal of Islamic & Middle Eastern Finance & Management, 14(5), 1043–1060. https://doi.org/10.1108/IMEFM-05-2020-0226
- Imbierowicz, B., & Rauch, C. (2014). The relationship between liquidity risk and credit risk in banks. Journal of Banking & Finance, 40, 242–256. https://doi.org/10.1016/j.jbankfin.2013.11.030
- İ̇ncekara, A., & Çetinkaya, H. (2019). Liquidity risk management: A comparative analysis of panel data between Islamic and conventional banking in Turkey. Procedia computer science, 158, 955–963. https://doi.org/10.1016/j.procs.2019.09.136
- Isik, I., & Hassan, M. K. (2002). Technical, scale and allocative efficiencies of Turkish banking industry. Journal of Banking & Finance, 26(4), 719–766. https://doi.org/10.1016/S0378-4266(01)00167-4
- Islam, S., & Kassim, S. (2015). Efficiency of islamic and conventional banks in Bangladesh: Comparative study using DEA approach. Journal of Islamic Economics, Banking and Finance, 11(3), 83–110. https://doi.org/10.12816/0024442
- Jahan, N. (2019). Productivity analysis of commercial banks of Bangladesh: A malmquist productivity index approach. International Journal of Economics and Financial Issues, 9(1), 108.
- Jeitschko, T. D., & Jeung, S. D. (2005). Incentives for risk-taking in banking–A unified approach. Journal of Banking & Finance, 29(3), 759–777. https://doi.org/10.1016/S0378-4266(04)00057-3
- Kargi, H. S. (2011). Credit risk and the performance of Nigerian banks. Ahmadu Bello University, Zaria.
- Kodithuwakku, S. (2015). Impact of credit risk management on the performance of commercial banks in Sri Lanka.
- Le, T. Q., Duong, H. N., & Nguyen, P. T. (2020). To list or not to list, that is the question: New evidence from the performance of Vietnamese commercial banks. Journal of Financial Regulation & Compliance, 29(2), 163–186. https://doi.org/10.1108/JFRC-03-2020-0026
- Le, T. D., Ho, T. N., Nguyen, D. T., & Ngo, T. (2022). Intellectual capital–bank efficiency nexus: Evidence from an emerging market. Cogent Economics & Finance, 10(1), 2127485. https://doi.org/10.1080/23322039.2022.2127485
- McAllister, P. H., & McManus, D. (1993). Resolving the scale efficiency puzzle in banking. Journal of Banking & Finance, 17(2–3), 389–405. https://doi.org/10.1016/0378-4266(93)90039-G
- Mezzi, N. (2018). Efficiency of Islamic banks and role of governance: Empirical evidence. Managerial Finance,
- Miah, M. D., & Sharmeen, K. (2015). Relationship between capital, risk and efficiency: A comparative study between Islamic and conventional banks of Bangladesh. International Journal of Islamic & Middle Eastern Finance & Management, 8(2), 203–221. https://doi.org/10.1108/IMEFM-03-2014-0027
- Mia, M. A., & Soltane, B. I. B. (2016). Productivity and its determinants in microfinance institutions (MFIs): Evidence from South Asian countries. Economic Analysis & Policy, 51, 32–45. https://doi.org/10.1016/j.eap.2016.05.003
- Munksgaard, J., Pade, L., & Fristrup, P. (2005). Efficiency gains in Danish district heating. Is there anything to learn from benchmarking? Energy Policy, 33(15), 1986–1997. https://doi.org/10.1016/j.enpol.2004.03.019
- Musa, H., Natorin, V., Musova, Z., & Durana, P. (2020). Comparison of the efficiency measurement of the conventional and Islamic banks. Oeconomia Copernicana, 11(1), 29–58. https://doi.org/10.24136/oc.2020.002
- Nabi, M. G., Islam, M. A., & Bakar, R. (2019). Do private commercial banks outperform state-owned commercial banks? Empirical evidence from Bangladesh. Journal of Applied Finance & Banking, 9(5), 167–186.
- Nitoi, M., & Spulbar, C. (2016). The relationship between bank efficiency and risk and productivity patterns in the Romanian Banking System. Espera, 19(1).
- Noman, A. H. M., Pervin, S., Chowdhury, M. M., & Banna, H. (2015). The effect of credit risk on the banking profitability: A case on Bangladesh. Global Journal of Management and Business Research, 15(C3), 41–48.
- Noulas, A. G., Ray, S. C., & Miller, S. M. (1990). Returns to scale and input substitution for large US banks. Journal of Money, Credit, and Banking, 22(1), 94–108. https://doi.org/10.2307/1992130
- Okuda, H., & Aiba, D. (2016). Determinants of operational efficiency and total factor productivity change of major Cambodian financial institutions: A data envelopment analysis during 2006–13. Emerging Markets Finance & Trade, 52(6), 1455–1471. https://doi.org/10.1080/1540496X.2015.1105630
- Partovi, E., & Matousek, R. (2019). Bank efficiency and non-performing loans: Evidence from Turkey. Research in International Business and Finance, 48, 287–309. https://doi.org/10.1016/j.ribaf.2018.12.011
- Parvej, M. M., Chowdhury, M. A. I., Azam, M. K. G., Hossain, M. M., & Mamun, A. M. A. (2020). Role of Islamic Microfinance in Alleviating Poverty in Bangladesh: A Study on RDS of IBBL. International Journal of Financial Research, 11(4), 111–129. https://doi.org/10.5430/ijfr.v11n4p111
- Porath, D. (2006). Estimating probabilities of default for German savings banks and credit cooperatives. Schmalenbach Business Review, 58, 214–233. https://doi.org/10.1007/BF03396732
- Rahman, M. L., & Banna, S. H. (2015). Liquidity risk management: A comparative study between conventional and Islamic banks in Bangladesh. Journal of Business and Technology (Dhaka), 10(2), 18–35. https://doi.org/10.3329/jbt.v10i2.29465
- Ramadhan, E. M. R., Wijaya, M. B. L., & Ruslan, B. (2022). Corporate governance and principal-agent theory: A critical review. EKOMBIS REVIEW: Jurnal Ilmiah Ekonomi Dan Bisnis, 10(2), 1391–1404. https://doi.org/10.37676/ekombis.v10i2.2108
- Rashid, M., Ur, H., Zobair, S. A. M., Chowdhury, M., Iqbal, A., & Islam, A. (2020). Corporate governance and banks’ productivity: Evidence from the banking industry in Bangladesh. Business Research, 13(2), 615–637. https://doi.org/10.1007/s40685-020-00109-x
- Ravn, I. (2022). Werner’s typology of banking theories. Paper Presented at the Forum for Social Economics, 51(3), 301–318. https://doi.org/10.1080/07360932.2019.1668286
- Rehman, A. U., Aslam, E., & Iqbal, A. (2022). Intellectual capital efficiency and bank performance: Evidence from islamic banks. Borsa Istanbul Review, 22(1), 113–121. https://doi.org/10.1016/j.bir.2021.02.004
- Rekik, M., & Kalai, M. (2018). Determinants of banks’ profitability and efficiency: Empirical evidence from a sample of banking systems. Journal of Banking and Financial Economics, 1(9), 5–23. https://doi.org/10.7172/2353-6845.jbfe.2018.1.1
- Rosman, R., Abd Wahab, N., & Zainol, Z. (2014). Efficiency of Islamic banks during the financial crisis: An analysis of Middle Eastern and Asian countries. Pacific-Basin Finance Journal, 28, 76–90. https://doi.org/10.1016/j.pacfin.2013.11.001
- Saeed, M., & Izzeldin, M. (2016). Examining the relationship between default risk and efficiency in Islamic and conventional banks. Journal of Economic Behavior & Organization, 132, 127–154. https://doi.org/10.1016/j.jebo.2014.02.014
- Saleh, I., Abu Afifa, M., & Murray, L. (2020). The effect of credit risk, liquidity risk and bank capital on bank profitability: Evidence from an emerging market. Cogent Economics & Finance, 8(1), 1814509. https://doi.org/10.1080/23322039.2020.1814509
- Samad, A. (2019). Determinants of efficiency of the islamic banks of Bangladesh during 2008-2012. Journal of Islamic Banking and Finance, 7(1), 1–13. https://doi.org/10.15640/jibf.v7n1a1
- Saranga, H., & Moser, R. (2010). Performance evaluation of purchasing and supply management using value chain DEA approach. European Journal of Operational Research, 207(1), 197–205. https://doi.org/10.1016/j.ejor.2010.04.023
- Sarkis, J., & Weinrach, J. (2001). Using data envelopment analysis to evaluate environmentally conscious waste treatment technology. Journal of Cleaner Production, 9(5), 417–427. https://doi.org/10.1016/S0959-6526(00)00084-6
- Sufian, F. (2009). Determinants of bank efficiency during unstable macroeconomic environment: Empirical evidence from Malaysia. Research in International Business and Finance, 23(1), 54–77. https://doi.org/10.1016/j.ribaf.2008.07.002
- Sufian, F., & Kamarudin, F. (2015). Determinants of revenue efficiency of Islamic banks. International Journal of Islamic & Middle Eastern Finance & Management, 8(1), 36–63. https://doi.org/10.1108/IMEFM-12-2012-0114
- Tan, Y., & Floros, C. (2018). Risk, competition and efficiency in banking: Evidence from China. Global Finance Journal, 35, 223–236. https://doi.org/10.1016/j.gfj.2017.12.001
- Tesfay, T. (2016). Determinants of commercial banks efficiency: Evidence from selected commercial banks of Ethiopia. International Journal of Scientific & Research Publications, 6(5), 551–556.
- Van Greuning, H., & Iqbal, Z. (2008). Risk analysis for Islamic banks. World Bank Publications.
- Werner, R. A. (2016). A lost century in economics: Three theories of banking and the conclusive evidence. International Review of Financial Analysis, 46, 361–379. https://doi.org/10.1016/j.irfa.2015.08.014
- Williams, J., & Nguyen, N. (2005). Financial liberalisation, crisis, and restructuring: A comparative study of bank performance and bank governance in South East Asia. Journal of Banking & Finance, 29(8–9), 2119–2154. https://doi.org/10.1016/j.jbankfin.2005.03.011
- Yahaya, A., Mahat, F., Yahya, M. H., & Matemilola, B. T. (2022). Liquidity risk and bank financial performance: An application of system GMM approach. Journal of Financial Regulation & Compliance, 30(3), 312–334. https://doi.org/10.1108/JFRC-03-2021-0019
- Yilmaz, A., & Güneş, N. (2015). Efficiency comparison of participation and conventional banking sectors in Turkey between 2007-2013. Procedia-Social & Behavioral Sciences, 195, 383–392. https://doi.org/10.1016/j.sbspro.2015.06.338
- Zeineb, G. B., & Mensi, S. (2018). Corporate governance, risk and efficiency: Evidence from GCC Islamic banks. Managerial Finance,
- Zheng, Y., Rashid, M. H. U., Siddik, A. B., Wei, W., & Hossain, S. Z. (2022). Corporate social responsibility disclosure and firm’s productivity: Evidence from the banking industry in Bangladesh. Sustainability, 14(10), 6237. https://doi.org/10.3390/su14106237