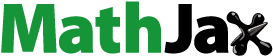
Abstract
Financial distress is a vexing managerial challenge for businesses worldwide, especially during a turbulent period like the COVID-19 pandemic. Motivated by an increasing number of closed businesses in Vietnam during the recent COVID-19 pandemic, this study is conducted to provide a comprehensive analysis of financial distress for Vietnamese listed firms. Machine learning approaches are employed using the annual data of 492 listed firms from 2012 to 2021. Specifically, we aim to identify the appropriate distress predictors for the Vietnamese listed firms using LASSO, a technique known to be superior compared to other variable selection techniques. Empirical results reveal that there are four key financial distress predictors for the Vietnamese listed firms, namely the ratios of (i) working capital and total assets, (ii) retained earnings and total assets, (iii) earnings before interest and taxes and total assets and (iv) net income and total assets. We also conducted an industry-level analysis and found that the Energy sector experienced the highest number of financially distressed firms during Covid-19. In contrast, Communication Services, Health Care, and Utilities had the lowest number of distressed firms. Policy implications have emerged based on these important findings from our analysis.
1. Introduction
The appearance of the COVID-19 pandemic has adversely influenced the global economy. The pandemic has accentuated the economic and financial vulnerabilities in many nations (Reinhart, Citation2021). The October 2020 World Economic Report presents that 183 out of 197 countries covered in their analysis had a contraction in per capita real GDP during the pandemic. Nevertheless, the COVID-19 pandemic, as a severe health crisis, does not travel alone. Previous evidence shows that a crisis can affect the form and rapidity of economic recovery. It arrives with sovereign debt, the exchange rate crashes, and becomes a severe corporate crisis. Thus, a health crisis can turn into a financial crisis. Indeed, the pandemic has resulted in waves of business failures and financially distressed firms, leading to widespread economic distress and a deterioration in the global economy.
Vietnam is also a part of the COVID-19 sphere of influence as the pandemic brings unprecedented consequences to the country. The country underwent four waves of the pandemic with an alarming fatal rate, forcing the government to adopt prolonged social distancing measures. As a result, the domestic and international demand in contact-intensive sectors and low-value-added manufacturing plummeted, resulting in significant liquidity shortages (Kroeger et al., Citation2020). Non-financial sectors also witnessed a sharp rise in total debt and a marked revenue fall (Tan & Tran, Citation2020). Moreover, there was an escalating number of businesses closed in light of the pandemic (Nguyen et al., Citation2022). A report from Vietnam’s General Statistics Office (GSO) indicates that 119,800 businesses were closed permanently in 2021 (18 per cent higher than 2020) in light of stringent lockdown and social distancing measures. Also, the total number of newly established businesses in the market declined by 11 per cent during the same year.
Against this background, providing immediate support for distressed firms after the shock would attenuate firms’ liquidity constraints and labour market stress. In this regard, understanding the nature of risks and the complete picture of corporate distress in Vietnam, especially during a turbulent period like the COVID-19 pandemic, is vital for policy reassessment, formulation and implementation.
The term “financial distress” is commonly referred to as a situation in which a firm cannot generate sufficient income to cover its financial obligations (Pindado et al., Citation2008; Tinoco & Wilson, Citation2013; Vassalou et al., Citation2004). Financial distress can be a herald of bankruptcy. Specifically, bankruptcy will be the only option if the financially distressed condition of a firm gets out of control. As such, much research has been devoted to modelling corporate financial distress and/or default and/or bankruptcy for the past few decades. There are two main strands of research. The first research strand concentrates on the accounting-based models (Altman, Citation1968; Ohlson, Citation1980; Zmijewski, Citation1984). In particular, accounting-based variables are adopted as predictors, and static models are often utilized for analysis purposes. Another strand of research focuses on market-based models, suggesting that the inclusion of market-based variables can enhance the model’s predictive performance (Bharath & Shumway, Citation2008; Merton, Citation1974; Vassalou et al., Citation2004). Meanwhile, recent studies also consider other factors affecting corporate distress, such as corporate social responsibility, industry-relative information, audit report information, capital structure, board directors’ characteristics, and others (Boubaker et al., Citation2018, Citation2020; García & Herrero, Citation2021; Lizares & Bautista, Citation2021; Muñoz-Izquierdo et al., Citation2020).
Nevertheless, more attention should be paid to modelling corporate distress for the Vietnamese market up to now. A few attempts exist, but these studies do not account for the COVID-19 pandemic’s effect (D. Vo et al., Citation2019; Pham et al., Citation2018). Therefore, this study is designed to provide an updated and comprehensive analysis of corporate financial distress in the Vietnamese market. In particular, this study identifies the key distress predictors for 492 non-financial Vietnamese listed firms from 2012 to 2021. In addition, an analysis at the industry level during the COVID-19 pandemic is conducted to provide more useful insights and implications.
Empirical findings reveal that there are four key distress predictors in the context of Vietnam, namely the ratios of (i) working capital total assets, (ii) retained earnings total assets, (iii) earnings before interest and taxes total assets, and (iv) net income to total assets. Our findings on the key accounting-based predictors align with previous studies in the field (Altman et al., Citation2017; Li et al., Citation2021; Ohlson, Citation1980; Pham et al., Citation2018; Zmijewski, Citation1984). Specifically, these selected ratios are negatively correlated with the probability of distress, indicating that the higher these ratios are, the lower the probability of financial distress. Moreover, our results are robust across different estimation techniques and model evaluation approaches. Besides, the analysis at the industry level shows that Energy was the most genitively impacted industry during the COVID-19 pandemic since it experienced the largest number of financially distressed firms. Meanwhile, the most resilient industries include Communication Services, Health Care, and Utilities.
The contributions of our study can be summarized as follows. First, our study extends the existing literature on financial distress prediction models for Vietnamese listed firms by identifying the well-fitted distress predictors. As a result, our findings can be beneficial to practitioners, policymakers, and scholars. In particular, our improved financial distress prediction model is straightforward and can be used in the financial markets, bankruptcy prediction, or distress classification. Second, our industry-level analysis offers practitioners and policymakers a complete perspective on corporate financial distress in Vietnam, from which they can evaluate the financial distress risk and take immediate responses. Third, our study provides broader implications for other emerging markets as emerging markets may share common characteristics.
Following this introduction, the remainder of this paper is structured as follows. Section 2 reviews the literature on corporate financial distress. Section 3 briefly discusses the methodology. Empirical findings are provided and discussed in section 4. Section 5 conducts further analysis at the industry level, followed by the concluding remarks and policy implications in section 6.
2. Literature review
This section briefly reviews the early and recent prominent empirical studies on modelling corporate distress and/or bankruptcy and/or default. There is a growing strand of research shedding on this topic. The research varies in terms of sample coverage and variables, and methodology selection.
The initial strand of research focuses on accounting-based variables as predictors. Early studies on this strand examine corporate default through ratio analysis (Beaver, Citation1966; Jackendoff, Citation1962). However, a seminal concept in statistical reduced-form financial distress modelling was introduced, forming the foundation of accounting-based models (Altman, Citation1968). In particular, using multivariate discriminant analysis (MDA), Altman (Citation1968) introduced the Z-score model predicting corporate distress using five accounting ratios, namely the ratios of (i) working capital total assets, (ii) retained earnings total assets, (iii) earnings before interest and taxes total assets, (iv) sales to total assets, and (v) market value of equity to book value of total liabilities. However, later studies criticized the restrictive assumptions of MDA, so other reduced-form models were introduced, for instance, the conditional logit (Ohlson, Citation1980) or the probit (Zmijewski, Citation1984) models, which allow different variances in the observations. Early reduced-form models use static models with accounting-based variables as predictors (Deakin, Citation1972; Dichev, Citation1998; Griffin & Lemmon, Citation2002; Lennox, Citation1999; Taffler, Citation1983). However, static models can only consider one set of predictors for each firm. As a result, the hazard model, which has superior predictive performance, was introduced. In particular, the model can consider the time-domain perspective (changing characteristics over time) and predict bankruptcy at different time horizons (Bauer & Agarwal, Citation2014). Additionally, adopting the hazard model in distress prediction enables straightforward estimation and interpretation (Shumway, Citation2001). Several later studies, thus, adopted the hazard model (Campbell et al., Citation2008; Cathcart et al., Citation2020; Charalambakis & Garrett, Citation2019).
The second strand of research suggests the inclusion of market-based variables as predictors, as the static nature of accounting-based variables can reduce the distress/bankruptcy/default measurement accuracy (Katz et al., Citation1985). In the early stage, an option-pricing theory, a seminal concept that laid a foundation in the literature on market-based models, was first utilized as a market-based default probability measure (Black & Scholes, Citation1973; Merton, Citation1974). Later, empirical evidence revealed that including market-based variables could improve the model prediction accuracy (Shumway, Citation2001; Tinoco & Wilson, Citation2013). Thus, numerous studies were also conducted using the market-based models (Bharath & Shumway, Citation2008; Dinh et al., Citation2021; Friewald et al., Citation2014; Vassalou et al., Citation2004; Wu et al., Citation2010). For instance, Merton’s (Citation1974) market-based model was adopted to analyze the relationship between corporate default probability and equity returns (Friewald et al., Citation2014; Vassalou et al., Citation2004). However, the predictive power of the Merton’s (Citation1974) model was later revisited. The results indicated that this model is insufficient for determining default probability (Bharath & Shumway, Citation2008).
Besides the abovementioned research, recent research considers predictors other than the traditional accounting-based or market-based variables. For instance, leverages, including both financial leverage and operating leverage, are shown to play crucial roles in determining the probability of distress and/or default (Cathcart et al., Citation2020; Tao et al., Citation2022). Likewise, real earnings management is also an early warning indicator for distressed firms (Li et al., Citation2021). Besides, capital structure, audit report information, industry-relative information, the composition of the board of directors, and the engagement level in corporate social responsibility activities can also influence the probability of distress and/or default (Boubaker et al., Citation2018, Citation2020; García & Herrero, Citation2021; Lizares & Bautista, Citation2021; Muñoz-Izquierdo et al., Citation2020).
The literature review reveals a vast amount of empirical research on the modelling of corporate financial distress with distinctive choices of variables and methodologies. Additionally, although accounting-based models have several limitations, they still have been widely adopted by practitioners and researchers worldwide due to their straightforward estimation and interpretation. Nonetheless, little is known about corporate distress prediction in Vietnam, especially when the Vietnamese market has just suffered from the severe consequences of the COVID-19 pandemic. There are few attempts to model corporate distress in Vietnam. However, the empirical evidence on this topic has not been updated despite the changing market structures (D. Vo et al., Citation2019; Pham et al., Citation2018; V. X. Vo, Citation2015). As such, this study aims to examine corporate financial distress using accounting-based variables as predictors for Vietnamese listed firms throughout 2012–2021.
3. Methodology
In this section, we introduce our dataset and then the measurement of corporate financial distress. We then discuss all the potential predictors utilized in the study. Finally, we briefly discuss the study’s empirical strategy.
3.1. Sample and data
The financial data utilized in this study are sourced from Refinitiv Eikon—one of the world’s most comprehensive financial time series databases. Refinitiv Eikon provides vast global economic, company and financial data widely used in academia and industry. Definitions of utilized variables are summarized in Appendices—Appendix 1.
Our dataset covers non-financial Vietnamese firms listed on the Ho Chi Minh Stock Exchange (HOSE) and Hanoi Stock Exchange (HNX) from 2012 to 2021. The concentration on this period is driven by the appearance of the COVID-19 pandemic, which has led to the recent weakened financial performance of the Vietnamese listed firms. The initial dataset includes 860 Vietnamese listed firms. However, firms in the financial sector are excluded from our dataset due to their distinctive characteristics. Additionally, firms with missing values on the key variables are also excluded. Then, the dataset is winsorized at the 1st and 99th percentile to deal with the issue of potential outliers. The final dataset, thus, consists of 4,920 firm-year observations corresponding with 251 firms listed on HOSE and 241 firms listed on HNX.
3.2. Measuring corporate financial distress
Our dependent variable is – a dummy variable denoting whether a firm is financially distressed in a specific year.
We follow prior studies to construct the dependent variable measuring corporate financial distress (Asquith et al., Citation1994; Dinh et al., Citation2021; Pham et al., Citation2018; Pindado et al., Citation2008; Tinoco & Wilson, Citation2013). These studies define financial distress as when a firm cannot generate sufficient revenue to cover its financial obligations. The probability of distress, therefore, can be identified as the ratio between a firm’s earnings and its interest expenses. Specifically, a firm will be classified as having financial distress in a particular year if it has an interest coverage ratio of less than one (or its earnings are less than its interest expenses). Table below presents the frequency of the dependent variable used in this study.
Table 1. Frequency counts of the dependent variable
3.3. Potential financial distress predictors
Our potential predictors include 12 financial ratios (accounting-based determinants) chosen from the literature on corporate financial distress (Altman, Citation1968, Citation1983; Shumway, Citation2001; Taffler, Citation1983; Zmijewski, Citation1984). These predictors are divided into three groups: (i) Liquidity, (ii) Profitability, and (iii) Leverage. Table presents the descriptive statistics of all these potential variables used in the paper.
Table 2. Descriptive statistics
3.4. The empirical analysis strategy
3.4.1. A logit model
The static model is appropriate for predicting corporate financial distress (Adnan Aziz & Dar, Citation2006; Altman, Citation1968; Li et al., Citation2021; Ohlson, Citation1980; Zmijewski, Citation1984). Thus, we adopt the logit model—one of the most widely-used models for classification purposes. The specific form of the logit model can be presented as follows.
P represents the probability of financial distress. Where Y denotes the binary dependent variable, taking a value of 1 if a firm is financially distressed and 0 otherwise. Xi represents the independent variables, and βi are the corresponding coefficients.
Our empirical model is constructed after selecting well-fitted distress predictors (which will be discussed later). Because the empirical model is constructed after the LASSO variable selection approach, we call it the LASSO-based model. The empirical model is as follows.
Where i = 1, 2, 3, … and t = 1, 2, 3, … represent firm i and year t, respectively. is the binary dependent variable classifying distressed firms.
is the ratio of working capital to total assets.
is the ratio of retained earnings to total assets.
is the ratio of earnings before interest and taxes to total assets.
is the ratio of net income to total assets. We also consider the industry and year-fixed effects to improve the predictive performance of our model and control for the potential endogeneity.
3.4.2. The selection of variables
In this study, we utilize LASSO regression, a penalized shrinkage approach, to select the key predictors from a set of 12 potential predictors drawn from the existing literature for our logit model. In addition, we also utilize the backward and forward Stepwise regression models to ensure the robustness of our results.
The reason for adopting LASSO as a main variable selection technique is that LASSO is computationally feasible and still retains the stability of Ridge regression (Efron et al., Citation2004; Tian et al., Citation2015; Tibshirani, Citation1996). LASSO has been frequently used for distress modelling in recent years due to its powerful predictive ability. The basic idea of LASSO regression is to estimate the by minimizing the following function:
The first term, , is the prediction error, which is the same value that least squares minimize. The second term,
, is a penalty that increases in value the more complex the model, causing LASSO to omitted variables. For using LASSO, the sparsity assumption—the unknown true model contains few variables relative to N observations—must be held.
Although less powerful than LASSO regression, stepwise regression is a widely used conventional variable selection approach. Stepwise regression is conducted automatically to fit the regression models from the potential predictors. Each step of the procedure examines the relevance of each predictor to determine its inclusion or removal based on a pre-specified criterion (Draper & Smith, Citation1998). The backward stepwise regression begins with a full model and then removes unsuitable predictors from the model. Meanwhile, the forward stepwise regression begins with an empty model and then adds suitable predictors into the model.
3.4.3. A model evaluation approach
We evaluate the model validity by (i) computing the area under the receiver operating characteristic curve (AUC), (ii) comparing our model with the Altman’s Z”-score model, and (iii) splitting the entire sample into the training set and the testing set.
The AUC denotes the area under the receiver operating characteristic (ROC) curve, measuring the model’s predictive ability (Hosmer et al., Citation2013). The ROC curve summarizes the trade-off between the true positive rate (correctly classifies non-distressed firms as non-distressed) and the false positive rate (incorrectly classifies non-distressed firms as distressed) for a classification model (Fawcett, Citation2006). Accordingly, a model with a greater AUC is considered superior in predicting corporate financial distress.
Altman developed several well-known Z-score models for distinct types of firms (private, public, manufacturing, non-manufacturing) in separate groups of countries. However, the Altman Z“-score model is appropriate in the international context, especially the emerging market (Altman et al., Citation2017). Thus, this model is selected to compare the predictive performance with our constructed model. The Altman’s Z”-score model is as follows:
The Z”-score components include the working capital over total assets (WCTA), the ratio between retained earnings to total assets (RETA), the ratio between earnings before interest and taxes over the total asset (EBITTA), and the book value of equity to the total liability (TETL). Firms with a Z”- score greater than 5.85 are classified as non-distress, whereas firms with a Z”-score less than 4.15 are classified as distressed.
Researchers frequently adopt sample splitting in distress and bankruptcy prediction (Altman et al., Citation2021; Li et al., Citation2021; Serrano-Cinca et al., Citation2019). The initial dataset will be split into two sets: (i) a training set (in-sample) and (ii) a testing set (out-of-sample) to evaluate the model performance. In particular, 80 per cent of our sample will be the training set, while the other 20 per cent will be the testing set. We use the classification accuracy rate and the AUC to compare and evaluate the in-sample and the out-of-sample predictive performance.
4. Empirical results
4.1. The selection of variables
We utilize LASSO as a main technique to recognize a set of key predictors for explaining corporate financial distress in Vietnam. In addition, the backward and forward stepwise models are also used to ensure the robustness of our findings. Table presents the results of variable selection. Detailed results of the variable selection are provided in Appendices—Appendix 2. As presented in Table below, the results are consistent across the three techniques.
Table 3. The selection of variables
The results suggest that four predictors are consistently selected from 12 potential predictors from the existing literature. These four selected predictors include the working capital over total assets (WCTA), the ratio between retained earnings to total assets (RETA), the ratio between earnings before interest and taxes over the total asset (EBITTA), and the ratio of the net income to total assets (NITA). These selected predictors are identical to the Altman Z”-score model, except for the NITA ratio. The book value of equity to the total liability (TETL) ratio used in the Altman Z”-score model is no longer appropriate for our analysis of the Vietnamese market.
4.2. The empirical results using the logistic regression
After selecting the four key predictors, we examine whether the four selected predictors can predict the probability of distress for Vietnamese listed firms using logistic regression. We also consider the effects of industry and year dummies to improve the model performance and control for the potential endogeneity. Finally, the AUC value is computed to assess the model’s predictive performance. Table presents the logistic regression results for our model. The estimated coefficients of the four predictors are negative and significant. The negative signs of the estimated coefficients indicate that the probability of distress declines with increased values of these four predictors. These findings are consistent with previous studies regarding corporate financial distress (Altman et al., Citation2017; Pham et al., Citation2018).
Table 4. Logistic regression results for the LASSO-based model
Furthermore, the model performance is improved when we control for industry and year dummies. In particular, the AUC value for model (4), which controls for industry and year dummies, is higher than that of model (1). We also split our sample into training and testing sets to evaluate our model’s predictive performance and ensure our findings’ robustness. Detailed results of sample splitting are provided in Appendices—Appendix 3.
4.3. The comparison of the performance of our various models
In this section, we compare our model—the LASSO-based model with the Altman Z”-score model. Table compares the logistic regression results. The four predictors’ coefficients in our model are all highly significant. Meanwhile, the term TETL in the Z”-score model is insignificant, implying that TETL may not be suitable for financial distress prediction in the Vietnamese market. These results indicate that our model performs slightly better than the Altman Z”-score model for Vietnamese listed firms.
Table 5. The comparison between our model and Altman Z”-score model – Logistic regression
We now examine other evaluation metrics to gain a deeper and more comprehensive insight into the model’s predictive performance. In this study, we use (i) the classification accuracy rate (AR) and (ii) the area under the receiver operating characteristic curve (AUC). For comparison purposes, we provide illustrative figures. Detailed comparisons between our model and the Z”-score model are provided in Appendix 4. The overall results indicate that our model performs slightly better than the Z”-score model for the Vietnamese context for listed firms.
In particular, Figure compares the proportion of correct and incorrect distress classification of the two models. The two models correctly classify non-distress firms as non-distress with 97.1 per cent, implying that the two models work well for non-distressed firms. However, when it comes to distressed firms, our model correctly classifies 71.1 per cent, slightly higher than the Z“-score model, which correctly classifies 70.3 per cent. Furthermore, Figure compares the AUC between the two models. The AUC metrics also confirm that the AUC value of our model, the LASSO-based model, is higher than that of the Altman Z”-score model. Overall, our model (with AR = 92.28% and AUC = 0.9517) performs slightly better than the Z”-score model (with AR = 92.14% and AUC = 0.9510).
Figure 1. Normalized confusion matrix (%).
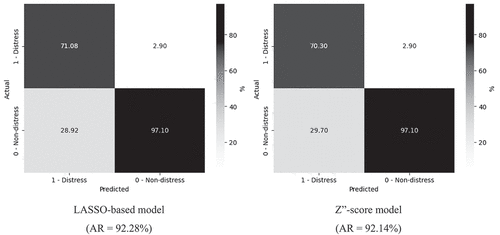
5. The empirical results of the financial distress at the industry level in Vietnam
An analysis of financial distress for a single country would be incomplete without analysis at the industry level, especially during a turbulent period such as the COVID-19 pandemic, resulting in an increasing number of distressed firms worldwide. Therefore, this section presents and discusses results from the analysis at the industry level for Vietnamese listed firms during the COVID-19 pandemic.
The analysis is conducted for 2020 and 2021, in which businesses suffered the most from the COVID-19 pandemic. The Vietnamese listed firms are classified into each industry based on the Global Industry Classification Standard (GICS). The Altman Z”-score model is adopted for the industry-level analysis. The reason for this choice is that the Z”-score model performs better than our model during the pandemic period (Appendices—Appendix 5) and has a specific classification threshold (mentioned in section 3).
Table presents the empirical results from the industry-level analysis. These results provide the proportion of financially distressed firms in each industry for 2020 and 2021. The results show that the number of distressed firms decreased after one year of the pandemic. Overall, Energy is the most negatively impacted industry during the pandemic, followed by Industrials and Information Technology. Meanwhile, the most resilient industries include Communication Services, Health Care, and Utilities.
Table 6. Industry-level analysis during the Covid-19 pandemic
6. The concluding remarks and policy implications
The COVID-19 pandemic has negatively affected the business system on a global scale. Vietnam is no exception, as the country has experienced increased closed businesses during the pandemic. Corporate financial distress, thus, has become one of the most vexing challenges for Vietnamese businesses, especially during and after the COVID-19 pandemic. Nonetheless, little is known about corporate financial distress in the Vietnamese market. Accordingly, our study is conducted to comprehensively analyze corporate financial distress in the Vietnamese market. The dataset underpinning this study spans 2012–2021 on 492 Vietnamese listed firms. Machine learning techniques are adopted for analysis purposes.
The key findings can be summarized as follows. First, we proposed a revised prediction model for financial distress in the context of Vietnam—the LASSO-based prediction model. Empirical findings indicate that four key financial ratios are found to predict financial distress in the context of the Vietnamese listed firms, including the working capital over total assets (WCTA), the ratio between retained earnings to total assets (RETA), the ratio between earnings before interest and taxes over the total asset (EBITTA), and the ratio of the net income to total assets (NITA). Second, we analyzed the industry-level during the COVID-19 pandemic and found that the effect of the pandemic on each industry varies. Empirical findings reveal that during the COVID-19 pandemic, Energy suffered the most, with the largest number of distressed firms, followed by Industrials and Information Technology. Meanwhile, the least impacted industries include Communication Services, Health Care, and Utilities.
Our study contributes primarily to distress prediction models for Vietnamese listed firms. We find the well-fitted distress predictors for Vietnamese listed firms using LASSO regression. In the Vietnamese context, profitability ratios seem to have the most significant prediction power compared to other financial ratios, such as liquidity or leverage ratios. Additionally, the relationship between the likelihood of distress and profitability is negative, suggesting that firms with high profitability tend to experience a low likelihood of being financially distressed. Although the Z”-score model has a good predictive ability, our model performs better than the Z”-score model in identifying many distressed firms in the Vietnamese context. Therefore, our revised distress prediction model may benefit Vietnam’s practitioners, policymakers, scholars and potentially other emerging markets. In particular, the revised model is an accounting-based model, which is straightforward and economical for both managerial and analysis purposes. Also, the revised model can be adopted in numerous areas, including distress classification, bankruptcy prediction, and financial markets.
Another contribution of our study is that we provide an analysis at the industry level during the COVID-19 pandemic. This extended analysis offers a comprehensive perspective on corporate financial distress in Vietnam. The industry-level analysis helps practitioners and policymakers fully understand the Vietnamese corporate distress during a turbulent period so that they can evaluate the risk of each industry and provide immediate support to prevent the contagion effect on the whole system. Findings from our study can also provide implications for other emerging markets since emerging markets may share similar characteristics, such as poor credit ratings or weak regulatory systems (Li et al., Citation2021). Hence, adopting our model could help countries with similar characteristics to Vietnam enhance the accuracy and reliability of corporate performance evaluation.
However, our study exhibits limitations that can be addressed and improved. Specifically, the study focuses on a single-country analysis spanning ten years, which could not account for the effect of the Global Financial Crisis simultaneously with the COVID-19 pandemic. In addition, there is still a need for a cross-country analysis covering a longer period to provide implications for other emerging markets. Furthermore, further research can examine the nature of risks using more advanced and novel machine learning methods and evaluation metrics to provide different insights into the literature on corporate financial distress. Additionally, further research can approach and examine the effect of the global crises on corporate financial distress, for instance, using the event study methodology. Besides, the way to measure corporate financial distress in the context of Vietnam is limited and needs to be more comprehensive due to data availability. Thus, further studies can be conducted to improve or create the measure of corporate financial distress, especially for emerging economies.
Correction
This article has been corrected with minor changes. These changes do not impact the academic content of the article.
Disclosure statement
No potential conflict of interest was reported by the authors.
Additional information
Funding
References
- Adnan Aziz, M., & Dar, H. A. (2006). Predicting corporate bankruptcy: Where we stand? Corporate Governance the International Journal of Business in Society, 6(1), 18–17. https://doi.org/10.1108/14720700610649436
- Altman, E. I. (1968). Financial Ratios, Discriminant Analysis and the Prediction of Corporate Bankruptcy. The Journal of Finance, 23(4), 589. https://doi.org/10.1111/j.1540-6261.1968.tb00843.x
- Altman, E. I. (1983). Corporate financial distress: A complete guide to predicting, avoiding, and dealing with bankruptcy. The Journal of Business Strategy, 5(1). Wiley Interscience, John Wiley and Sons. http://www.loc.gov/catdir/enhancements/fy0607/82016103-b.html%5Cnhttp://www.loc.gov/catdir/enhancements/fy0607/82016103-d.html%5Cnhttp://www.loc.gov/catdir/enhancements/fy0607/82016103-t.html
- Altman, E. I., Hu, X., & Yu, J. (2021). Understanding Credit Risk of Chinese Companies using Machine Learning: A Default-Based Approach. https://ssrn.com/abstract=3734053
- Altman, E. I., Iwanicz-Drozdowska, M., Laitinen, E. K., & Suvas, A. (2017). Financial Distress Prediction in an International Context: A Review and Empirical Analysis of Altman’s Z-score Model. Journal of International Financial Management & Accounting, 28(2), 131–171. https://doi.org/10.1111/jifm.12053
- Asquith, P., Gertner, R., & Scharfstein, D. (1994). Anatomy of Financial Distress: An Examination of Junk-Bond Issuers. The Quarterly Journal of Economics, 109(3), 625–658. https://doi.org/10.2307/2118416
- Bauer, J., & Agarwal, V. (2014). Are hazard models superior to traditional bankruptcy prediction approaches? A comprehensive test. Journal of Banking & Finance, 40(1), 432–442. https://doi.org/10.1016/J.JBANKFIN.2013.12.013
- Beaver, W. H. (1966). Financial Ratios as Predictors of Failure. Journal of Accounting Research, 4, 71. https://doi.org/10.2307/2490171
- Bharath, S. T., & Shumway, T. (2008). Forecasting Default with the Merton Distance to Default Model. The Review of Financial Studies, 21(3), 1339–1369. https://doi.org/10.1093/RFS/HHN044
- Black, F., & Scholes, M. (1973). The Pricing of Options and Corporate Liabilities. The Journal of Political Economy, 81(3), 637–654. https://doi.org/10.1086/260062
- Boubaker, S., Cellier, A., Manita, R., & Saeed, A. (2020). Does corporate social responsibility reduce financial distress risk? Economic Modelling, 91, 835–851. https://doi.org/10.1016/J.ECONMOD.2020.05.012
- Boubaker, S., Hamza, T., & Vidal-García, J. (2018). Financial distress and equity returns: A leverage-augmented three-factor model. Research in International Business and Finance, 46, 1–15. https://doi.org/10.1016/J.RIBAF.2016.09.003
- Campbell, J. Y., Hilscher, J., & Szilagyi, J. (2008). In Search of Distress Risk. The Journal of Finance, 63(6), 2899–2939. https://doi.org/10.1111/j.1540-6261.2008.01416.x
- Cathcart, L., Dufour, A., Rossi, L., & Varotto, S. (2020). The differential impact of leverage on the default risk of small and large firms. Journal of Corporate Finance, 60, 101541. https://doi.org/10.1016/j.jcorpfin.2019.101541
- Charalambakis, E. C., & Garrett, I. (2019). On corporate financial distress prediction: What can we learn from private firms in a developing economy? Evidence from Greece. Review of Quantitative Finance and Accounting, 52(2), 467–491. https://doi.org/10.1007/s11156-018-0716-7
- Deakin, E. B. (1972). A Discriminant Analysis of Predictors of Business Failure. Journal of Accounting Research, 10(1), 167. https://doi.org/10.2307/2490225
- Dichev, I. D. (1998). Is the Risk of Bankruptcy a Systematic Risk? The Journal of Finance, 53(3), 1131–1147. https://doi.org/10.1111/0022-1082.00046
- Dinh, D. V., Powell, R. J., & Vo, D. H. (2021). Forecasting corporate financial distress in the Southeast Asian countries: A market-based approach. Journal of Asian Economics, 74, 101293. https://doi.org/10.1016/J.ASIECO.2021.101293
- Draper, N. R., & Smith, H. (1998). Applied regression analysis (3rd ed.). Wiley. https://www.wiley.com/en-us/Applied+Regression+Analysis%2C+3rd+Edition-p-9781118625682
- Efron, B., Hastie, T., Johnstone, I., & Tibshirani, R. (2004). Least angle regression. Annals of Statistics, 32(2), 32(2. https://doi.org/10.1214/009053604000000067
- Fawcett, T. (2006). An introduction to ROC analysis. Pattern Recognition Letters, 27(8), 861–874. https://doi.org/10.1016/j.patrec.2005.10.010
- Friewald, N., Wagner, C., & Zechner, J. (2014). The Cross-Section of Credit Risk Premia and Equity Returns. The Journal of Finance, 69(6), 2419–2469. https://doi.org/10.1111/JOFI.12143
- García, C. J., & Herrero, B. (2021). Female directors, capital structure, and financial distress. Journal of Business Research, 136, 592–601. https://doi.org/10.1016/J.JBUSRES.2021.07.061
- Griffin, J. M., & Lemmon, M. L. (2002). Book-to-Market Equity, Distress Risk, and Stock Returns. The Journal of Finance, 57(5), 2317–2336. https://doi.org/10.1111/1540-6261.00497
- Hosmer, D. W., Lemeshow, S., & Sturdivant, R. X. (2013). Applied Logistic Regression. Wiley. https://doi.org/10.1002/9781118548387
- Jackendoff, N. (1962). A Study of Published Industry Financial and Operating Ratios (Vol. 52). Bureau of Economic and Business Research - Temple University. https://books.google.com.vn/books?hl=en&lr=&id=c88DAAAAMAAJ&oi=fnd&pg=PA11&dq=jackendoff+1962&ots=KEAhccenok&sig=cLjRPurzTIFnTTuOTZHX3VAf934&redir_esc=y#v=onepage&q=jackendoff%201962&f=false
- Katz, S., Lilien, S., & Nelson, B. (1985). Stock Market Behavior Around Bankruptcy Model Distress and Recovery Predictions. Financial Analysts Journal, 41(1), 70–74. https://doi.org/10.2469/faj.v41.n1.70
- Kroeger, T., Nguyen, T. N. A., Zhang, S., Pham, D. T., Nguyen, H. M., & Duong, D. T. (2020). Corporate Vulnerabilities in Vietnam and Implications of COVID-19. IMF Working Papers, 20(260). https://doi.org/10.5089/9781513561820.001
- Lennox, C. (1999). Identifying failing companies: A re-evaluation of the logit, probit and DA approaches. Journal of Economics and Business, 51(4), 347–364. https://doi.org/10.1016/S0148-6195(99)00009-0
- Li, C., Lou, C., Luo, D., & Xing, K. (2021). Chinese corporate distress prediction using LASSO: The role of earnings management. International Review of Financial Analysis, 76, 101776. https://doi.org/10.1016/j.irfa.2021.101776
- Lizares, R. M., & Bautista, C. C. (2021). Corporate financial distress: The case of publicly listed firms in an emerging market economy. Journal of International Financial Management & Accounting, 32(1), 5–20. https://doi.org/10.1111/JIFM.12122
- Merton, R. C. (1974). On the Pricing of Corporate Debt: The Risk Structure of Interest Rates. The Journal of Finance, 29(2), 449. https://doi.org/10.2307/2978814
- Muñoz-Izquierdo, N., Laitinen, E. K., Camacho-Miñano, M. D. M., & Pascual-Ezama, D. (2020). Does audit report information improve financial distress prediction over Altman’s traditional Z-Score model? Journal of International Financial Management & Accounting, 31(1), 65–97. https://doi.org/10.1111/JIFM.12110
- Nguyen, N. H., Vu, N. T., Tran, Q., Tran, T., & Vo, D. H. (2022). Market performance and volatility during the Covid-19 pandemic in Vietnam: A sector-based analysis. Cogent Business & Management, 9(1). https://doi.org/10.1080/23311975.2022.2119681
- Ohlson, J. A. (1980). Financial Ratios and the Probabilistic Prediction of Bankruptcy. Journal of Accounting Research, 18(1), 109. https://doi.org/10.2307/2490395
- Pham, B., Do, T., & Vo, D. (2018). Financial distress and bankruptcy prediction: An appropriate model for listed firms in Vietnam. Economic Systems, 42(4), 616–624. https://doi.org/10.1016/J.ECOSYS.2018.05.002
- Pindado, J., Rodrigues, L., & de la Torre, C. (2008). Estimating financial distress likelihood. Journal of Business Research, 61(9), 995–1003. https://doi.org/10.1016/J.JBUSRES.2007.10.006
- Reinhart, C. M. (2021). From Health Crisis to Financial Distress. http://www.worldbank.org/prwp.
- Serrano-Cinca, C., Gutiérrez-Nieto, B., & Bernate-Valbuena, M. (2019). The use of accounting anomalies indicators to predict business failure. European Management Journal, 37(3), 353–375. https://doi.org/10.1016/j.emj.2018.10.006
- Shumway, T. (2001). Forecasting bankruptcy more accurately: A simple hazard model. The Journal of Business, 74(1), 101–124. https://doi.org/10.1086/209665
- Taffler, R. J. (1983). The Assessment of Company Solvency and Performance Using a Statistical Model. Accounting and Business Research, 13(52), 295–308. https://doi.org/10.1080/00014788.1983.9729767
- Tan, S., & Tran, T. (2020). Impacts of Covid-19 on Firms in Vietnam. https://openknowledge.worldbank.org/bitstream/handle/10986/34580/Results-from-the-First-Round-of-COVID-19-Business-Plus-Survey.pdf?sequence=1&isAllowed=y
- Tao, Q., Zahid, Z., Mughal, A., & Shahzad, F. (2022). Does operating leverage increase firm’s profitability and bankruptcy risk? Evidence from China’s entry into WTO. International Journal of Finance & Economics, 27(4), 4705–4721. https://doi.org/10.1002/IJFE.2395
- Tian, S., Yu, Y., & Guo, H. (2015). Variable selection and corporate bankruptcy forecasts. Journal of Banking & Finance, 52, 89–100. https://doi.org/10.1016/j.jbankfin.2014.12.003
- Tibshirani, R. (1996). Regression Shrinkage and Selection via the Lasso. Journal of the Royal Statistical Society Series B (Methodological), 58(1), 267–288. https://doi.org/10.1111/j.2517-6161.1996.tb02080.x
- Tinoco, M. H., & Wilson, N. (2013). Financial distress and bankruptcy prediction among listed companies using accounting, market and macroeconomic variables. International Review of Financial Analysis, 30, 394–419. https://doi.org/10.1016/J.IRFA.2013.02.013
- Vassalou, M., Xing, Y., Campbell, J., Cochrane, J., Chen, L., French, K., Hirshleifer, D., Jagannathan, R., Lando, D., Nielsen, L. T., Ritter, J., Shanken, J., & Stein, J. (2004). Default Risk in Equity Returns. The Journal of Finance, 59(2), 831–868. https://doi.org/10.1111/J.1540-6261.2004.00650.X
- Vo, D., Pham, B., Ho, C., & McAleer, M. (2019). Corporate Financial Distress of Industry Level Listings in Vietnam. Journal of Risk and Financial Management, 12(4), 155. https://doi.org/10.3390/JRFM12040155
- Vo, V. X. (2015). Using Accounting Ratios in Predicting Financial Distress: An Empirical Investigation in the Vietnam Stock Market. Journal of Economics and Development, 17(1), 42. https://doi.org/10.33301/2015.17.01.03
- Wu, Y., Gaunt, C., & Gray, S. (2010). A comparison of alternative bankruptcy prediction models. Journal of Contemporary Accounting & Economics, 6(1), 34–45. https://doi.org/10.1016/J.JCAE.2010.04.002
- Zmijewski, M. E. (1984). Methodological Issues Related to the Estimation of Financial Distress Prediction Models. Journal of Accounting Research, 22, 59. https://doi.org/10.2307/2490859
Appendices
Appendix
Appendix
Appendix 3.
The sample splitting – Evaluating the performance of the LASSO-based model
Appendix 4.
Comparison between our model and Z”-score model – Model performance metrics
Appendix 5.
Comparison between the pre-Covid- 5. and the Covid- 5.period – The AUC value