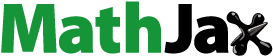
Abstract
Efficiency and productivity measurement in the insurance industry has attracted great interest from academicians, practitioners, financial market analysts, insurance regulators, and researchers; however, the near absence of empirical work on the productivity changes of the life insurance industry in Ghana poses management challenges. The main objective of this paper is to measure the efficiency and productivity changes in the life insurance industry in Ghana. The Ghana National Insurance Commission’s annual report data was used in this study. We employ the non-parametric Malmquist productivity change indices and the bootstrap technique to measure the productivity changes of a sample of 19 out of 20 Ghanaian life insurance firms for the period 2015–2020. The empirical results show that the level of output-oriented technical inefficiency of the life insurance industry in Ghana is approximately 17% over the period 2015–2020. This result provides us with a general guide to identify the periods in which the industry is most and least technically efficient. Also, our findings show that the estimate of total productivity changes has retrogressed about 13% over the period in the Ghanaian life insurance industry. We further identified that the deterioration in the productivity is due to efficiency changes. The decomposition of the technological changes also reveals that the life insurance industry did not benefit from pure scale efficiency. This study contributes by encouraging the policy makers and managers of life insurance firm to use scarce resources efficiently and productively in order to stay in business.
1. Introduction
Efficiency and productivity measurement of insurance industry has attracted great interest from academicians, practitioners, investors, financial market analysts, insurance regulators, and researchers (Kaffash et al., Citation2020; Mends-Brew & Ashiagbor, Citation2016). The insurance industry worldwide and in Ghana has developed quite over the past decade, especially in terms of its expansion to semi-urban and rural areas.
In Ghana, insurance business is dominated by major non-life insurers nevertheless the works of the Ghana national insurance commission (NIC) and other industry players positions the life insurers to play a leading role in the Ghanaian insurance industry. This is probably because the life insurance sector is generally meant for long term protection (10 years, 15 years or for life) while the general sector is aimed at short-term protection such as annual renewal (Lim et al., Citation2021).
It is increasingly becoming more difficult to effectively manage the meagre resources at the firms’ disposal especially when the managers are unable to identify the rightful amount of inputs to be used to achieve the desire outputs overtime and more importantly when the source of these inefficiencies are neither precisely measured nor known.
As reported by the NIC in its 2020 annual report, the gap between the gross premium of the life and non-Life insurance sectors has been narrowing over the years. The total assets of the Ghana insurance industry grew by 17%, that is, from GHS7.65 billion in 2019 to GHS8.9 billion in 2020. The assets of the life insurance sector grew from GHS3.9 billion in 2019 to GHS4.6 billion in 2020 representing 20% increase.
In narrow sense, life insurance is any form of insurance whose payment is contingent upon whether the insured is dead (or alive). According to the NIC (2020), the contribution of total insurance premiums to Gross Domestic Product (GDP) is less than 2% in Ghana which highlights that the insurance penetration in Ghana is relatively low. The absence of empirical evidence of the level of productivity change in the life insurance industry in Ghana over the years clearly inhibits effective decision making by managers and regulator. The assets of Ghana’s insurance sector covering the period of current study are presented in Table .
Table 1. The assets of Ghana’s life insurance sector (2014–2020)
The growing numbers of studies based on frontier efficiency techniques are tilted toward more advanced economies (Kaffash et al., Citation2020; Naushad et al., Citation2020). Also, the bootstrap technique and Malmquist productivity change index (MPI) concept are less applied in the insurance industry. For instance, Ferrier and Hirschberg (Citation1997) applied both bootstrapping and MPI to the Italian banking data, Tortosa-Ausina et al. (Citation2008) also used bootstrapping technique combined with the estimated MPI to examine the Spanish banking sector. To the best of knowledge, there is near absence of studies that empirically measure the statistical precision of efficiency and productivity changes of the Ghanaian life insurance industry. There is therefore a yawning gap, and the current study seeks to fill such a gap.
The rest of the paper is organized as follows. In the section 2, we present related literature of the study. Section 3 touches on the methodology used to obtain the measure of efficiency and productivity changes as well as components of productivity change. Section 4 describes the main results concerning efficiency levels and productivity changes and presents the explanatory analysis. Conclusions are given in a section 5.
2. Literature review
The theoretical framework that underpins this study is the production economics (Abdurakhmanov, Citation2020; Couix, Citation2020). This part of microeconomic theory deals with the production of output from a given set of inputs usually expressed in terms of a production function. Efficiency is a major issue in insurance because it is a component of total productivity (Barros et al., Citation2005; Kaffash et al., Citation2020). Productivity change is a widely used to as a performance metric for firms and establishment (Alrikabi, Citation2022; Kaydos, Citation2020).
There are different measures of productivity and the choice between them depends either on the purpose of the productivity measurement and/or data availability. The foremost approach to measure productivity change in the insurance literature is the Malmquist productivity index (MPI). Since this concept of this index was pioneered by Malmquist (Citation1953), and it has been further developed in the sphere of non-parametric framework by several authors, for example, Caves et al. (Citation1982b, Citation1982a), Färe et al. (Citation1994), Thrall (Citation2000), etc.
Malmquist productivity index is a theoretical index, expressed in terms of distance functions defined on the true, but unknown, technology (Obi & Visser, Citation2020; Pathak, Citation2019). It is essentially a nonparametric index that can compute productivity changes of a decision-making unity (DMU), that is, life insurance companies over time, in that it indicates progress or regress in efficiency along the frontier technology under multiple inputs and output framework (Eling et al., Citation2020; Gregoriou & Zhu, Citation2005).
Even though the MPI have a number of advantages, such as modeling multidimensional inputs and outputs even in absence of price information, the concept has one major drawback. The estimates from the conventional data envelopment analysis (DEA) based Malmquist analysis offers relatively no information on random noise, hence one cannot clearly determine whether differences between two or more estimates are statistically significant because it lacks statistical inference (Mends-Brew & Ashiagbor, Citation2016; Odeck & Schøyen, Citation2020; Odeck, Citation2009). Many studies have adopted various methods in attempt to correct this bias in DEA. Berger and Humphrey (Citation1997) indicate that attempts should be made to estimate confidence interval for efficiency and productivity measures obtained non-parametric approach, so that statistical inference exercises can be conducted.
Some studies on insurance efficiency focused on partial aspects of productivity, such as ratio analysis (Alexakis et al., Citation2019; Masud et al., Citation2019; Oppong et al., Citation2019) or used the Tornquist price index (Azzam et al., Citation2021; Chessa et al., Citation2017), or with combination of other analytical tools such as probit, logit and multiple regression (Masara & Dube, L2017), a technique not currently preferred by current researchers.
The non-parametric approach, which generally allows the data to speak for itself, also enable to analyse the productivity changes under the assumption of variable returns to scale, and to decompose the technical changes into frontier shift (pure technology) and changes in shapes of the frontier (scale of technology). Bootstrapping technique thus helps to correct the influence of environmental factors and all random interferences to improve upon accuracy of efficiency and productivity measurement (H. Li & Dong, Citation2015; Kweh et al., Citation2020; Simar & Wilson, Citation2012).
Malmquist productivity change attracts many attention and have been applied in many sectors. For instance it is applied in agriculture (Kataoka, Citation2020; RL & Mishra, Citation2022), banking (Christopoulos et al., Citation2020; Dar et al., Citation2021), climate conditions (Long et al., Citation2020; Zhu et al., Citation2020). Other areas in which the concept of MPI is employed include energy (Tachega et al., Citation2021; Zheng, Citation2021), tourism sector (Kim et al., Citation2021; Tzeremes, Citation2020), education sector (Agasisti et al., Citation2020; Arjomandi et al., Citation2015).
One of early study in Ghana using the concept of frontier analysis was carried out by Owusu-Ansah et al. (Citation2010) to determine the level of managerial efficient of the general insurers. Their findings revealed that the general insurers operated at an average overall efficiency of 68%, technical efficiency of 87% and scale efficiency of 78%. They also observed that Ghanaian general insurers with higher dimension and market shares tend to have higher efficiencies; implying that general insurers could increase their efficiencies by trying to increase among other things their dimension and market shares.
Again, Ansah‐adu et al. (Citation2012) set to assess the cost efficiency of insurance companies in Ghana using a two-stage procedure to ascertain whether insurance companies are cost efficient and also to examine the efficiency determinants of insurance companies. The results revealed that larger firms tend to be more efficient than smaller firms and also showed that the ratio of equity to total invested assets have an inverse relationship with cost efficiency. Ansah‐adu et al. (Citation2012) also reveal that market share is the key determinant of efficiency among insurance companies in Ghana. For policy implication, they suggest that insurance companies in Ghana should adopt a benchmark management procedures in order to evaluate their relative position and to adopt appropriate managerial procedures for catching up with the frontier of “best practices”.
Another empirical study in the Ghana insurance industry was conducted by Alhassan et al. (Citation2015) to examine the impact of the regulatory-driven market structure on firm pricing behaviour. This was done by testing the structure-conduct-performance hypothesis for both life and non-life insurance markets in Ghana. It was revealing that there is an increasing level of competition in both life and non-life insurance industry in Ghana though they still remain concentrated with the life insurance sector having high levels of efficiency compared to the non-life sector.
The dynamic cost productivity growth of the Ghanaian insurance industry from 2005 to 2014 was examined by Ohene-Asare et al. (Citation2019). They observed that the introduction of the Ghana insurance Act of 2006 saw some large cost productivity growths and the cost improving policies in the Act that encouraged cost efficiency must be revisited by regulators. It is also revealed by Ohene-Asare et al. (Citation2019) that not many insurers in Ghanaian market have been operating at the optimal production scale over the period.
Recently, Tuffour et al. (Citation2021) examines the determinants of cost and profitability efficiency of life insurance companies in Ghana, utilising data from 12 life insurance companies for the period of 2013–2017. They used the fixed effect panel regression which results show that the significant determinants of both cost and profit functions are: price of labour, commission, gross premium and net investment income.
Tuffour et al. (Citation2021) also revealed that, on the average, the life insurance companies were about 71.2% cost efficient and 41.7% profit efficient. Further analysis reveals that both profit and cost efficiency changes have statistically significant positive effect on firms’ return on asset.
From the aforementioned studies, it could be observed that the concepts of bootstrapping, efficiency and efficiency change which provide statistical precision and are very important in managerial decision making are not fully explored in the life insurance industry in Ghana; this thus motivates the current study.
3. Methods and materials
3.1. Data source, sample size and analysis
A balanced panel secondary data for the period 2015–2020 are computed from the published annual reports of the NIC. This period is chosen to reflect to reflect any possible change in the industry. As indicated earlier, literature on measuring life insurance industry is very minimal. As at 31st December 2020, the regulated life entities in the Ghanaian insurance industry is 20, out of which we selected 19 firms taking into consideration the consistency of availability of data within the stated period. Also, this is to ensure that the decision making units (DMUs) are homogeneous and the number of the DMUs is three times more than the number of input and output. Each insurance company was regarded and treated as a DMU.
For our analysis, an application of bootstrapping which is a data-based simulation method in making statistical inference precision was used. The bootstrapping technique combined with MPI was used to measure the total factor productivity change with statistical precision. The results are decomposed into the technical efficiency change (EFFCH) and technological change (TECH). The TECH was further decomposed into pure technological change (PTEC) and scale efficiency change (SEC). This affords us the opportunity to analyse changes in the efficiency and productivity with the scores of bootstrapped MPI and its components. The PTEC estimates how close an insurer is to the production frontier under variable returns-to-scale (VRS). The production frontier refers to the use of minimum resources to produce a targeted amount of product by a highly efficient insurer. The SEC estimates the decrease in production as a result of variation in constant returns-to-scale (CRS).
3.2. Input and output data selection
In the use of Malmquist technique, the selection of right inputs and outputs are crucial. These input and output set of data are used to construct a piecewise frontier over the data point for the period under consideration; productivity changes are then measured relative to these frontiers that represent. The selection of inputs and outputs variable in this paper is largely influenced by insurers’ provision of three key services, namely risk pooling relating to insured losses, and financial intermediation services. The data for input and output variables are contained in annual financial report of the national insurance commission (NIC), the regulator of the insurance industry.
Following the works of Masud et al. (Citation2019), K. Li (Citation2005), Rai (Citation1996), as well as Gardner and Grace (Citation1993), we used the gross premium and investment income as the output variables. For the selection of inputs, we followed the recent literature on efficiency (Huang & Eling, Citation2013; Cummins & Rubio-Misas, 20006Masud et al., Citation2019) and thus selected labour, claim payment and equity capital as input variables.
3.3. Malmquist productivity index
The output-oriented concept of MPI measures productivity changes of DMUs in transforming inputs into outputs from time period to time period
is as follows:
Also, MPI at time period t + 1 is given by
where
is the distance function measuring the efficiency of inputs
to input
during the period
, this represents the distance from the period
to observation to period technology (own-period efficiency)
this also represents own-period efficiency, it computes the distance from the period
to observation to period
technology
is cross-period efficiency that represents the distance from period
observation to the period
technology.
is also cross-period efficiency that represents the distance from period
observation to the period
technology.
To avoid an arbitrariness in the choice of benchmark technology, a geometric mean of (1) and (2) are taken and this yield the MPI as
EquationEquation (3(3)
(3) ) can be decomposed into efficiency change (EFFCH) and technological change (TECH) as follows:
The MPI gives us the opportunity to decompose total factor productivity changes into technical and technological changes, and further be decomposed into pure technical efficiency change (PTEC) and scale efficiency changes (SEC).
Clearly, (4) can be written as
EquationEquation 7(7)
(7) ) can further be decomposed into pure technical efficiency change (PTEC) and scale efficiency changes (SEC). A value of MPI, EFFCH, TECH, PTEC or SEC greater than one (1.0000) indicates an improvement and less than one indicates deterioration.
3.4. Algorithm for bootstrapping MPI and its components
The idea of bootstrap was introduced by Efron (Citation1979) and later extended by Simar and Wilson (Citation2012, 2010; 1998). It is a data-based simulation method that helps in making statistical inference in measuring confidence intervals (Efron & Tibshirani, Citation1994; H. Li & Dong, Citation2015; Simar & Wilson, Citation2000). The bootstrap approach helps to identify whether the growth indicated by the MPI is significant. It will further enable us to assess whether changes in MPI, efficiency, technology, pure and scale efficiency are also significant (Simar & Wilson, Citation2012). The current study performed bootstrap DEA and Malmquist with the R and FEAR software packages. The confidence interval of 0.05 was used.
The technique for bootstrapping the MPI and its components is based on the fact that the Malmquist is a function of distance estimators as defined in (1) through to (7). The following algorithm was deployed to find the MPI, EFFCH, TECH, PTC, and SEC scores of the insurance companies;
Step 1. Compute the for each insurer, i = 1,2,3, … … … … n by solving (1)
Step 2. Compute the pseudo data set to obtain the reference bootstrap technology by using the bivariate kernel density where the bandwidth was selected by following the normal reference rule.
Step 3. Compute the bootstrap estimate of the MPI for insurer through the pseudo sample obtained in step 2.
Step 4. Repeat steps 2 and 3 for a large B times (we use B = 5000, that is, the number of bootstrap replications) in order to obtained the bootstrap sample for each insurer.
Step 5. From, the bootstrap sample, compute the bias-corrected estimates and confidence intervals for the MPI by selecting the appropriate percentiles
The basic idea designed for the construction of the confidence intervals of the MPI is that the distribution of is unknown and can be approximated by the distribution of
, where
is the true unknown idex,
is the estimate of the MPI, and
is the bootstrap estimate of the index. Hence
and
defining the
confidence interval;
EquationEquation (8(8)
(8) ) can be approximated by estimating the values of
and
given by:
Thus, an estimated (1-a) percent confidence interval for the i-th MPI is given by
An MPI for the ith insurer is said to be significantly different from unity (which would indicate no productivity change), at the 5% level, if the interval in EquationEquation (10(10)
(10) ) does not include unity.
It should be mentioned that using the calculated bootstrap value in step 4, we can also correct for any finite-sample bias in the original estimators of the MPI.
We only need to apply a simple procedure outline by Simar and Wilson (Citation1999) as follows:
The bootstrap bias estimate for the original estimator is:
Thus, a bias-corrected estimate of can be computed as:
However, as explained by Simar and Wilson (Citation1999), this bias-corrected estimator may have a higher mean-square error than the original estimator, and hence it will be less reliable. Overall, the bias-corrected estimator should only be considered if the sample variance of bootstrap value
is less than
4. Results and discussions
4.1. Descriptive statistics
Descriptive statistics for all input-output variables are found in Table . From this schedule, it can be observed that the standard deviations are relatively higher for all variables under consideration which demonstrates that large inequalities exist among the life insurance firms. This is an indication of how imperative it is for the life insurance companies to manage their inputs-outputs variables.
Table 2. Descriptive statistics of input and output variables used
4.2. An isotonicity test of input and output variable used
An isotonicity test was conducted with the input and output variables to ensure that the DEA isotonicity criteria are met. The concept of isonicity in DEA is to ensure that the relationship between inputs and outputs is not erratic (Maity, Citation2020; Wang et al., Citation2020; Y. Li, Citation2020).
Increasing the value of any input while keeping other factors constant should not decrease any output but should instead lead to an increase in the value of at least one output. In other words, the relationship between the input and output variables used should be positive and have high correlation coefficient. That is the level of output is at least the same, and dord not fall when inputs increases (Tsai et al., Citation2016).
Table indicates the correlation coefficient among the input-output variables of which relatively high correlation among the input and output variables indicates that an acceptable isonicity is fulfilled with regard to inputs and output variable used in this study.
Table 3. Correlation coefficients of input and output variables
4.3. Technical efficiency scores
We estimate the output-oriented technical efficiency for each of the 19 life insurance companies for the entire period as indicates in Table . A value of less than one implies inefficiency on the part of the firm under consideration. Column two lists the mean efficiency estimates, and column 3 through 6 lists the bias-corrected estimates, the bootstrap bias estimate and the lower and upper boundaries of the efficiency scores. It could be observed from Table that the level of efficiency of the industry is inconsistent. It could be noted that in all cases the average of estimated efficiency lies to the right of the estimated confidence intervals; this result reflects the theory behind the construction of the confidence intervals presented by Simar and Wilson (Citation1998).
Table 4. Bootstrap estimates for the period 2015 –2020
Also, it could be observed that the technical efficiency estimates differ from the bias-corrected estimates. In some periods this difference (the bias) is quite small. For instance, the difference was less than 0.04 in the following periods; 2015, 2017 and 2020, while in other periods, such as 2016 and 2018 the difference was about 0.08. The means of the minor biases of variable return to scale estimates (0.058), and the relatively smaller confidence intervals in these years imply that the results are relatively stable.
We take into cognizance the relative comparisons of the performance based on the estimated efficiency scores with caution.
This result provides us with a general guide to identify the period within which the industry is most and least technically efficient. For the entire period under consideration, the industry had exhibited less efficiency. That is, the biased-corrected estimate of the life insurance industry has experienced approximately 16.8% technical inefficiency.
4.4. Productivity changes
Focusing only on the efficiency estimates can provide an incomplete overview of the performance of the industry over the period under consideration (Arjomandi et al., Citation2011). As stated earlier, the main focus of the current study is to compute statistical precision of the efficiency and productivity changes and the various sources of these changes of the life industry over the period. These estimates for the period 2015–2020 are reported in Table . We observed that only one of the life insurance company (L14) has consistently experienced improvement over the period. This might be possibly due to using appropriate amount of inputs to achieve the desire output as reflected in their indices of the period. The productivity of the industry as a whole has consistently underperformed over the years, resulting in the approximately 12.6% deterioration for the entire period.
Table 5. Bias-corrected productivity changes for each insurer for the period 2015–2020
4.5. Sources of productivity changes
As indicated earlier, concentrating only on efficiency estimates can provide an incomplete view of the performance of life insurance firm over time (Arjomandi et al., Citation2011). Changes in distance-function values over time could result from either the movement of the firm within the input-output space (efficiency changes) or progress/regress of the boundary of the production set over time (technological changes).
The decomposition of the Malmquist index, as explained in EquationEquation 3(3)
(3) through to EquationEquation 7
(7)
(7) , makes it possible to distinguish changes in productivity, efficiency and technology. Table highlights some stylized fact about the change in productivity and the various sources of these changes.
Table 6. Source of productivity change for life insurance industry for the entire period
From Table it could be observed that the MPI for the industry is 12.6% deterioration in the productivity over the period and this was due to a fall in the EFFECH of average of about 17.4%. This was further highlighted by the scale efficiency changes as indicated in Table . All the indices at global level are statistically significant at 95 per cent. The average pure technical efficiency which is related to the capability of managers to use firms’ given resources was estimated to be higher than the average scale efficiency, is related with exploiting scale. Thus, the result implies that the technical inefficiency was mostly a result of scale inefficiency instead of pure technical efficiency. The major source of scale inefficiency was found to be decreasing returns to scale.
5. Conclusion and policy implications
This study relied on the non-parametric bootstrapped Malmquist indices and efficiency scores to measure and analyse the statistical precision level of the technical efficiency and productivity changes of the life insurance industry in Ghana over the period 2015–2020. This has led to further estimation of the changes in technological efficiency, changes in efficiency, pure changes in technology and changes in scale of technology. The bootstrap approach shows that the majority of our estimates are statistically significant.
Our findings reveal that the level of output-oriented technical inefficiency of the life insurance industry in Ghana is approximately 17% over the period 2015–2020. Furthermore, our findings show that the estimate of total productivity changes has retrogressed about 13% over the period in the Ghanaian life insurance industry.
We further identified that the deterioration in the productivity are mainly due to efficiency changes. The decomposition of the technological changes also reveals that the life insurance industry did not benefit from the pure scale efficiency.
To conclude, this study has measured the statistical precision of efficiencies and productivity indices of life insurance industry in Ghana, the study further identified the exact sources of productivity change in of life insurance industry in Ghana. It is hoped that managers of life insurance firms and the regulators of the industry would adequately act on specific needs by orientation of policies in the right direction that would lead to improvement in efficiency and productivity of life insurance industry in Ghana and lesson learnt can be applied elsewhere. Thus, this study contributes by encouraging the policy makers and managers of life insurance firm to use scarce resources efficiently and productively in order to stay in business.
Disclosure statement
No potential conflict of interest
References
- Abdurakhmanov, K. (2020). Labour Economics. Theory and practice. Naukowe Wydawnictwo IVG.
- Agasisti, T., Shibanova, E., Platonova, D., & Lisyutkin, M. (2020). The Russian Excellence Initiative for higher education: A nonparametric evaluation of short‐term results. International Transactions in Operational Research, 27(4), 1911–14. - Search (bing.com. https://doi.org/10.1111/itor.12742
- Alexakis, C., Izzeldin, M., Johnes, J., & Pappas, V. (2019). Performance and productivity in Islamic and conventional banks: Evidence from the global financial crisis. Economic Modelling, 79, 1–14. https://doi.org/10.1016/j.econmod.2018.09.030
- Alhassan, A. L., Addisson, G. K., & Asamoah, M. E. (2015). Market structure, efficiency and profitability of insurance companies in Ghana. International Journal of Emerging Markets, 10(4), 648–669. https://doi.org/10.1108/IJoEM-06-2014-0173
- Alrikabi, A. A. M. (2022). Measuring and analyzing productivity indicators in the Iraqi general insurance company for the period (2011-2020). American Journal of Economics and Business Management, 5(11), 265–284.
- Ansah‐adu, K., Andoh, C., & Abor, J. (2012). Evaluating the cost efficiency of insurance companies in Ghana. The Journal of Risk Finance, 13(1), 61–76. https://doi.org/10.1108/15265941211191949
- Arjomandi, A., Salleh, M. I., & Mohammadzadeh, A. (2015). Measuring productivity change in higher education: An application of Hicks–Moorsteen total factor productivity index to Malaysian public universities. Journal of the Asia Pacific Economy, 20(4), 630–643. https://doi.org/10.1080/13547860.2015.1045323
- Arjomandi, A., Valadkhani, A., & Harvie, C. (2011). Analysing productivity changes using the bootstrapped Malmquist approach: The case of the Iranian banking industry. Australasian Accounting Business & Finance Journal, 5(3), 35–56. https://ro.uow.edu.au/aabfj/vol5/iss3/4/
- Azzam, A., Walters, C., & Kaus, T. (2021). Does subsidized crop insurance affect farm industry structure? Lessons from the US. Journal of Policy Modeling, 43(6), 1167–1180. https://doi.org/10.1016/j.jpolmod.2021.06.003
- Barros, C. P., Barroso, N., & Borges, M. R. (2005). Evaluating the efficiency and productivity of insurance companies with a Malmquist index: A case study for Portugal. The Geneva Papers on Risk and Insurance-Issues and Practice, 30(2), 244–267. https://doi.org/10.1057/palgrave.gpp.2510029
- Berger, A. N., & Humphrey, B. C. (1997). Efficiency in financial institutions: International survey and directions for future research. European Journal of Operational Research, 98(2), 175–212. https://doi.org/10.1016/S0377-2217(96)00342-6
- Caves, D. W., Christensen, L. R., & Diewert, W. E. (1982a). Multilateral comparisons of output, input, and productivity using superlative index numbers. https://doi.org/10.2307/2232257
- Caves, D. W., Christensen, L. R., & Diewert, W. E. (1982b). The economic theory of Index numbers and the measurement of input, output, and productivity. Econometrica: Journal of the Econometric Society, 50(6), 1393–1414. https://doi.org/10.2307/1913388
- Chessa, A. G., Verburg, J., & Willenborg, L. (2017, May). A comparison of price index methods for scanner data. Proceedings of the 15th Meeting of the Ottawa Group on Price Indices, Eltville am Rhein (pp. 10–12).
- Christopoulos, A., Dokas, I., Katsimardou, S., & Spyromitros, E. (2020). The Malmquist Productivity measure for UK-listed firms in the aftermath of the global financial crisis. Operational Research, 22(2), 1–18. https://doi.org/10.1007/s12351-020-00595-1
- Couix, Q. (2020). Georgescu-Roegen’s flow-fund theory of production in retrospect. Ecological Economics, 176, 106749. https://doi.org/10.1016/j.ecolecon.2020.106749
- Dar, A. H., Mathur, S. K., & Mishra, S. (2021). The efficiency of Indian banks: A DEA, Malmquist and SFA analysis with bad output. Journal of Quantitative Economics, 19(4), 653–701. https://doi.org/10.1007/s40953-021-00247-x
- Efron, B. (1979). Bootstrap methods: Another look at the jackknife. Annals of Statistics, 7(1), 1–26. https://doi.org/10.1007/978-1-4612-4380-9_41
- Efron, B., & Tibshirani, R. J. (1994). An introduction to the bootstrap (Vol. 57). CRC press. https://doi.org/10.1201/9780429246593
- Eling, M., Hoyt, R. E., & Schaper, P. (2020). The impact of capacity on price and productivity change in insurance markets. Journal of Insurance Issues, 43(1), 22–58. https://www.ivw.unisg.ch/wp-content/uploads/2018/04/WP200.pdf
- Färe, R., Grosskopf, S., Norris, M., & Zhang, Z. (1994). Productivity growth, technical progress, and efficiency change in industrialized countries. The American Economic Review, 84(1), 66–83. http://www.jstor.org/stable/2117971
- Ferrier, G. D., & Hirschberg, J. G. (1997). Bootstrapping confidence intervals for linear programming efficiency scores: With an illustration using Italian banking data. Journal of Productivity Analysis, 8(1), 19–33. https://doi.org/10.1023/A:1007768229846
- Gardner, L. A., & Grace, M. F. (1993). X-efficiency in the US life insurance industry. Journal of Banking & Finance, 17(2), 497–510. https://doi.org/10.1016/0378-42669390048-I
- Gregoriou, G. N., & Zhu, J. (2005). Evaluating hedge fund and CTA performance: Data envelopment analysis approach ISBN: 978-0-471-73004-0. John Wiley & Sons.
- Huang, W., & Eling, M. (2013). An efficiency comparison of the non-life insurance industry in the BRIC countries. European Journal of Operational Research, 226(3), 577–591. https://doi.org/10.1016/j.ejor.2012.11.008
- Kaffash, S., Azizi, R., Huang, Y., & Zhu, J. (2020). A survey of data envelopment analysis applications in the insurance industry 1993–2018. European Journal of Operational Research, 284(3), 801–813. https://doi.org/10.1016/j.ejor.2019.07.034
- Kataoka, M. (2020). Total factor productivity change in Indonesia’s provincial economies for 1990–2015: Malmquist productivity index approach. Letters in Spatial and Resource Sciences, 13(3), 233–243. https://doi.org/10.1007/s12076-020-00256-z
- Kaydos, W. (2020). Operational performance measurement: Increasing total productivity. CRC press. https://doi.org/10.4324/9780367802103
- Kim, C., Kang, H. J., Chung, K., & Choi, K. (2021). COVID-19 and hotel productivity changes: An empirical analysis using Malmquist productivity index. Service Science, 13(4), 243–257. https://doi.org/10.1287/serv.2021.0283
- Kweh, Q. L., Downing, K., Lu, W. M., & Ting, I. W. K. (2020). Productivity changes of insurers: Metafrontier Malmquist productivity index and a bootstrapping approach. The Singapore Economic Review. https://doi.org/10.1142/S0217590820500344
- Li, K. (2005). The empirical analysis of Chinese life insurance companies. Insurance Research (China), 2, 13–17.
- Li, Y. (2020). Analyzing efficiencies of city commercial banks in China: An application of the bootstrapped DEA approach. Pacific-Basin Finance Journal, 62, 101372. https://doi.org/10.1016/j.pacfin.2020.101372
- Li, H., & Dong, S. (2015). Measuring benchmarking technical efficiency of public hospital in Tianjin, China; a bootstrap-data envelopment analysis approach. INQUIRY: The Journal of Health Care Organization, Provision, and Financing, 52, 004695801560548. provision and financing https://doi.org/10.1177/0046958015605487 https://doi.org/10.1177/0046958015605487
- Lim, Q. M., Lee, H. S., & Har, W. M. (2021). Efficiency, productivity and competitiveness of the Malaysian insurance sector: An analysis of risk-based capital regulation. The Geneva Papers on Risk and Insurance-Issues and Practice, 46(1), 146–172. https://doi.org/10.1057/s41288-020-00173-8
- Long, R., Ouyang, H., & Guo, H. (2020). Super-slack-based measuring data envelopment analysis on the spatial–temporal patterns of logistics ecological efficiency using global Malmquist Index model. Environmental Technology & Innovation, 18, 100770. https://doi.org/10.1016/j.eti.2020.100770
- Maity, S. (2020). Are private sector banks really more efficient than public sector banks?—A comparative analysis using DEA. NMIMS Management Review, 38(2), 82–92.
- Malmquist, S. (1953). Index numbers and indifference surfaces. Trabajos de Estadisticay de InvestigacionOperativa, 4(2), 209–242. https://doi.org/10.1007/BF03006863
- Masud, M. M., Rana, M. S., Mia, M. A., & Saifullah, M. K. (2019). How productive are life insurance institutions in Malaysia? A Malmquist approach. The Journal of Asian Finance, Economics and Business, 6(1), 241–248. https://doi.org/10.13106/jafeb.2019.vol6.no1.241
- Mends-Brew, E., & Ashiagbor, A. A. (2016). Computing statistical precision of efficiency and productivity change indices: A bootstrap application to Ghanaian polytechnics. European Scientific Journal, ESJ, 12(9), 273. https://doi.org/10.19044/esj.2016.v12n9p273
- Naushad, M., Faridi, M. R., & Faisal, S. (2020). Measuring the managerial efficiency of insurance companies in Saudi Arabia: A data envelopment analysis approach. The Journal of Asian Finance, Economics and Business, 7(6), 297–304. https://doi.org/10.13106/jafeb.2020.vol7.no6.297
- Obi, O. F., & Visser, R. (2020). Productivity measurement of New Zealand forest harvesting sector using the DEA-Malmquist index. International Journal of Forest Engineering, 31(3), 224–232. https://doi.org/10.1080/14942119.2020.1770566
- Odeck, J. (2009). Statistical precision of DEA and Malmquist indices: A bootstrap application to Norwegian grain producers. Omega, 37(5), 1007–1017. https://doi.org/10.1016/j.omega.2008.11.003
- Odeck, J., & Schøyen, H. (2020). Productivity and convergence in Norwegian container seaports: An SFA-based Malmquist productivity index approach. Transportation Research Part A: Policy and Practice, 137, 222–239. https://doi.org/10.1016/j.tra.2020.05.001
- Ohene-Asare, K., Asare, J. K. A., & Turkson, C. (2019). Dynamic cost productivity and economies of scale of Ghanaian insurers. The Geneva Papers on Risk and Insurance-Issues and Practice, 44(1), 148–177. https://doi.org/10.1057/S41288-018-0111-6
- Oppong, G. K., Pattanayak, J. K., & Irfan, M. (2019). Impact of intellectual capital on productivity of insurance companies in Ghana: A panel data analysis with system GMM estimation. Journal of Intellectual Capital, 20(6), 763–783. https://doi.org/10.1108/JIC-12-2018-0220
- Owusu-Ansah, E., Dontwi, I. K., Seidu, B., Abudulai, G., & Sebil, C. (2010). Technical efficiencies of Ghanaian general insurers. American Journal of Social and Management Sciences, 1(1), 75–87. https://doi.org/10.5251/AJSMS.2010.1.1.75.87
- Pathak, H. P. (2019). Malmquist productivity index approach in assessing performance of Commercial Banks: Evidence from Nepal. NRB Economic Review, 31(2), 25–55. https://doi.org/10.3126/nrber.v31i2.35303
- Rai, A. (1996). Cost efficiency of international insurance firms. Journal of Financial Services Research, 10(3), 213–233. https://doi.org/10.1007/BF00114085
- RL, M., & Mishra, A. K. (2022). Agricultural production efficiency of Indian states: Evidence from data envelopment analysis. International Journal of Finance & Economics, 27(4), 4244–4255. https://doi.org/10.1002/ijfe.2369
- Simar, L., & Wilson, P. (2012). Estimation and inference in two-stage, semi-parametric models of production processes. Journal of Econometrics1, 136(1), 31–64. https://doi.org/10.1016/j.jeconom.2005.07.009
- Simar, L., & Wilson, P. W. (1998). Sensitivity analysis of efficiency scores: How to bootstrap in non-parametric frontier models. Management Science, 44(1), 49–61. https://doi.org/10.1287/mnsc.44.1.49
- Simar, L., & Wilson, P. W. (1999). Estimating and bootstrapping Malmquist indices. European Journal of Operational Research, 115(3), 459–471. https://doi.org/10.1016/S0377-2217(97)00450-5
- Simar, L., & Wilson, P. W. (2000). Statistical inference in non-parametric frontier models: The state of the art. Journal of Productivity Analysis, 13(1), 49–78. https://doi.org/10.1023/A:1007864806704
- Tachega, M. A., Yao, X., Liu, Y., Ahmed, D., Li, H., & Mintah, C. (2021). Energy efficiency evaluation of oil producing economies in Africa: DEA, Malmquist and multiple regression approaches. Cleaner Environmental Systems, 2, 100025. https://doi.org/10.1016/j.cesys.2021.100025
- Thrall, R. M. (2000). Measures in DEA with an application to the Malmquist index. Journal of Productivity Analysis, 13(2), 125–137. https://doi.org/10.1023/A:1007800830737
- Tortosa-Ausina, E., Grifell-Tatje, E., Armero, C., & Conesa, D. (2008). Sensitivity analysis of efficiency and Malmquist productivity indices: An application to Spanish savings banks. European Journal of Operational Research, 184(3), 1062–1084. https://doi.org/10.1016/j.ejor.2006.11.035
- Tsai, W., Lee, H., Yang, C., & Huang, C. (2016). Input-output for sustainability by using DEA method: A comparison study between European and Asian countries. Journal of Sustainability, 8(12), 1230. https://doi.org/10.3390/su8121230
- Tuffour, J. K., Ofori-Boateng, K., Ohemeng, W., & Akuaku, J. K. (2021). Life insurance companies: Determinants of cost efficiency and profitability. Journal of Accounting, Business and Management (JABM), 28(2), 1–19. https://doi.org/10.31966/jabminternational.v28i2.501
- Tzeremes, N. G. (2020). Robust Malmquist productivity measurement: Evidence from Spanish hotel industry during the Great Recession. International Journal of Productivity and Performance Management, 70(2), 408–426. https://doi.org/10.1108/IJPPM-01-2019-0037
- Wang, C. N., Tibo, H., & Nguyen, H. A. (2020). Malmquist productivity analysis of top global automobile manufacturers. Mathematics, 8(4), 580. https://doi.org/10.3390/math8040580
- Zheng, Z. (2021). Energy efficiency evaluation model based on DEA-SBM-Malmquist index. Energy Reports, 7, 397–409. https://doi.org/10.1016/j.egyr.2021.10.020
- Zhu, W., Zhu, Y., & Yu, Y. (2020). China’s regional environmental efficiency evaluation: A dynamic analysis with biennial Malmquist productivity index based on common weights. Environmental Science and Pollution Research, 27(32), 39726–39741. https://doi.org/10.1007/s11356-019-06966-2