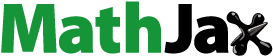
Abstract
In developing countries, economic inequality is attracting considerable attention. Many factors including financial exclusion are key in explaining income gap in developing countries. This paper examines the effect of access to financial services through digital technologies on income inequality. Using data from the World Development Indicator (WDI), the Central Bank of West African States (BCEAO) and the Standardized World Income Inequality Database (SWIID), we estimated a pooled means group estimation (PMGE) and a dynamic fixed effect (DFE) as a robustness test. The results indicate that digital financial inclusion leads to a decrease in income inequality. In the long run, there is a negative and significant effect of digital financial inclusion on inequality. The short run results evidenced more of the heterogeneity effect of digital financial inclusion in WAEMU countries due to the diversity, inconclusiveness, and counterintuitive results of the effect of DFI on inequality.
PUBLIC INTEREST STATEMENT
In the countries of West African Economics and monetary Union (WAEMU) like in other developing countries, financial exclusion is cited as a key factor that explains income disparities. In this paper, we evidenced the extent to which digital financial inclusion can affect income inequality across the eight countries of WAEMU. We used data on the eight WAEMU’s countries over the period 2007 to 2020 and find that in the long run, there is a negative and significant effect of digital financial inclusion on inequality. However, in the short run, the effects vary across WAEMU countries. They suggest that policies should be centered primarily on eliminating obstacles and developing innovative digital products so that to get more people banked. By expanding financial inclusion and Fintech, financial institutions can gain sufficient funds to tackle the credit rationing problem. Second, there is also a need to provide good infrastructure and appropriate digital finance regulations.
1. Introduction
Digital technology like mobile phone, computers, and Internet provides great opportunities for the well-being of people in developing countries (Senou et al., Citation2019; Yao et al., Citation2022). It has played a positive role in making finance more inclusive (Ji et al., Citation2021; Khera et al., Citation2022). The use of mobile phone and Internet can accelerate the expansion of financial services and reduce the income gap between and within countries. In fact, as a combination of digital technology and financial inclusion, digital financial inclusion (DFI) refers to all actions that promote financial inclusion through digital financial services (Senou et al., Citation2019; Shen et al., Citation2022). A number of scholars have argued that the inclusive nature of digital finance is an effective means to reduce poverty and increase income, and a lack of finance may lead to a widening income gap owing to financial constraints (Yao et al., Citation2022; Yu & Wang, Citation2021). The rise of financial technology allows more access to financial services for a wider group of people. With digital technology, barriers in conventional financial systems have decreased and thus, there is an increase in financial inclusion, a stable economic growth, and a decrease in inequality (Dabla-Norris et al., Citation2015; GPFI, Citation2016; Senou et al., Citation2019; Senou et al., Citation2019). Digital financial inclusion contributes to less discrimination in lending, thereby allowing more people to have access to loans (Bartlett et al., Citation2019; Cicchiello et al., Citation2021; Girón et al., Citation2022; Kazemikhasragh et al., Citation2022; Philippon, Citation2019).
Current existing literature that has systematically argued the relationship between digital finance and income distribution of residents is relatively limited, and there is still room for further exploration in terms of theoretical and empirical studies. For instance, some theoretical works have mostly focused on entrepreneurship as the causal mechanism linking access to financial services and inequality (Aghion and Bolton, Citation1997, Citation2005; Banerjee & Newman, Citation1993; Dabla-Norris et al., Citation2021). There are only a few findings on how financial inclusion directly impacts inequality at the country level (Demirguc-Kunt et al., Citation2017; Klapper et al., Citation2016; Senou & Soro, Citation2023; Shen et al., Citation2022). Thus, this paper will add to the growing literature of digital financial inclusion and inequality dynamics. Specifically, we aim to shed light on the heterogeneity effect of digital financial inclusion on income inequality in the West African Economic and Monetary Union (WAEMU) countries. WAEMU is a sub-regional organization that brings together eight countries, namely Benin, Burkina Faso, Côte d’Ivoire, Guinea-Bissau, Mali, Niger, Senegal, and Togo. With rising inequality in many countries, income distribution has attracted considerable attention in recent years in WAEMU. It is obvious that high levels of inequality impede the expansion of skills, limit economic and social mobility and human development, and as a result, inhibit economic growth (Ali et al., Citation2019). The evolution of per capita income in WAEMU displays some ambiguities despite the economic integration process. While income disparities between some countries seem to be narrowing, those between other countries seem to be widening at the same time.
The primary contribution of this study to the literature is that, to the best of our knowledge, this study is among the first to consider the heterogeneous jointly with the causal effect of DFI on income inequality using country-level panel data. In addition, the literature is not unanimous on the effect of digital financial inclusion on income inequality. Some studies have concluded that digital financial inclusion reduces income inequality (Omar & Inaba, Citation2020; Yao et al., Citation2022), while others have found that it widens or exacerbates the inequality (Ashenafi & Dong, Citation2022; Das et al., Citation2021). This study employs dynamic panel data estimators including PMGE and DFE. In general, previous empirical studies that have explored the effect of financial inclusion on income inequality have mostly used statics panel technics, pooled OLS or 2sls estimators (Agyemang-Badu et al., Citation2018; Ashenafi & Dong, Citation2022; Kling et al., Citation2022; Neaime & Gaysset, Citation2018; Omar & Inaba, Citation2020). The findings of this study suggest that digital financial inclusion leads to a decrease in income inequality. In the long run, there is a negative and significant effect of digital financial inclusion on inequality for all of the WAEMU countries. The short-run results evidenced more the heterogeneity effect of digital financial inclusion on inequality in the WAEMU countries.
The rest of the paper is organized as follows: Section 3 reviews the relevant literature. Section 4 presents the methodology and data. Section 5 presents and discusses the estimation results, while Section 6 concludes the article.
2. Literature review
There is an important and significant theoretical and empirical tradeoff between digital technology, financial inclusion, and inequality. However, the literature that incorporates heterogeneity aspects into the analysis of digital financial inclusion and inequality in the countries of WAEMU is scanty.
Economic theory suggests that financial exclusion can lead to persistent inequality. In the context of imperfect financial market, individuals’ endowment drives their ability to invest in physical or human capital, which prevents social mobility of the poor and perpetuates inequality (Aghion & Bolton, Citation1997; Banerjee & Newman, Citation1993; Galor & Zeira, Citation1993). These seminal authors model a binary choice to be or not to be an entrepreneur or a skilled worker, which requires investment. They suggest that financial inclusion can alleviate income inequality by increasing opportunities for education and entrepreneurship among the poor. In other words, high access to financial services could reduce income inequality among low-income households via saving, investment and borrowing activities (Galor & Moav, Citation2004; Greenwood & Jovanovic, Citation1990; Mookherjee & Ray, Citation2003).
Several studies have broadly evaluated the effect of financial inclusion on inequality (Fouejieu et al., Citation2020; Kling et al., Citation2022; Lacalle-Calderon et al., Citation2019; Li et al., Citation2022; Omar & Inaba, Citation2020, Citation2020; Ouechtati, Citation2020; Turégano & Herrero, Citation2018). Kling et al. (Citation2022) develop a theory linking financial inclusion to income inequality and evidenced the positive effect of financial inclusion on the reduction of income inequality. Omar and Inaba (Citation2020) investigate the impact of financial inclusion on reducing poverty and income inequality, as well as their driving factors and conditional effects in 116 developing countries. The results provide robust evidence that financial inclusion significantly reduces poverty rates and income inequality in developing countries. Fouejieu et al. (Citation2020) in their side examine the relationships between access to and use of financial services and economic inequality. Building on a new dataset on the availability and use of financial services, the analysis provides empirical estimates of the impact of financial inclusion on inequality, and explores potential nonlinearities in this relationship by highlighting the role of the prevailing macroeconomic and financial conditions. Using a dynamic panel data estimator, Ouechtati (Citation2020) examines the effect of financial inclusion on poverty and income inequality on a sample of 53 developing countries over the period 2004 to 2017. They find that a high bank penetration rate and credit facilitate access to financial services for the poor and reduce income inequality. The inequality reducing effect of the participation in microfinance programs has also been evidenced by Lacalle-Calderon et al. (Citation2019). They show that the level of income inequality is lower in developing countries where the level of participation in microfinance programs is higher. Turégano and Herrero (Citation2018) provide further evidence that countries with a more inclusive financial system tend to have a less unequal income distribution. Aslan et al. (Citation2017) show that increasing the intensity of use of financial services leads to a reduction in income inequality.
However, most of the current studies go beyond the single tradeoff between financial inclusion and inequality and evidence the positive effect of digital financial inclusion on inequality reduction (Demir et al., Citation2022; Meniago & Asongu, Citation2018; Polloni‑Silva et al., Citation2021; Ratnawati, Citation2020; Tchamyou et al., Citation2019; Yao et al., Citation2022). Yao et al. (Citation2022) used the statistical data of 280 prefectural-level cities in China over 2011 to 2020, to empirically test the relationship between digital finance and residents’ income. The study shows a Kuznets effect of digital finance development on the income distribution of Chinese residents. They find a positive effect of digital finance on income disparity that initially increase with the increase in regional economic level. Polloni‑Silva et al. (Citation2021) test if financial inclusion and technology adoption decrease the poverty headcount ratio and the Gini index of 13 Latin America countries. They used unbalanced panel dataset to estimate a Feasible Generalized Least Squares (FGLS) and a Limited Information Maximum Likelihood (LIML). Their results suggest that financial inclusion is a powerful tool to tackle poverty and inequality.
Demir et al. (Citation2022)’s study on 140 countries find that Fintech reduces the overall income inequality measured by Gini coefficients. Similarly, Ratnawati (Citation2020), examining the impact of financial inclusion in terms of banking penetration, access to banking services, and use of banking services, on economic growth, poverty, income inequality, and financial stability in Asia, shows that all dimensions of financial stability simultaneously have significant influence on economic growth, poverty, and income inequality.
Tchamyou et al. (Citation2019) using the Generalized Method of Moments investigated the role of information and communication technology (ICT) on income inequality, through financial development dynamics in 48 African countries over the period 1996 to 2014. They found that both financial depth and size are established to reduce inequality. Similarly, Meniago and Asongu (Citation2018) explore the relationship without policy variables in the light of the Kuznets hypothesis to conclude that financial access and intermediation efficiency reduce inequality. Moreover, Asongu and Nwachukwu (Citation2018) investigate the linkage between inequality, poverty, and mobile banking in 93 developing countries for the year 2011. They find a positive correlation between mobile banking and inclusive development when a certain threshold of the Human Development Index is reached. A closely related study by Asongu and Odhiambo (Citation2018) shows that mobile banking can contribute to reducing income inequality in countries where it is lowest or highest.
However, there may be a negative or an insignificant effect of financial inclusion or digital financial inclusion on inequality (Aginta et al., Citation2018; Agyemang-Badu et al., Citation2018; Ali et al., Citation2021; Ashenafi and Dong, Citation2022; Asongu & Odhiambo, Citation2018; Haan & Sturm, Citation2017; Jauch & Watzka, Citation2016). Ashenafi and Dong (Citation2022) investigates the impact of financial inclusion and Fintech on income inequality using the 2011, 2014, and 2017 waves of across 39 African countries. Employing pooled ordinary least square and two-stage least square (2sls) estimation methods they find that financial inclusion and Fintech exacerbate income inequality. Das & Chatterjee (Citation2021) explore both direct impact of digital technologies dissemination and their indirect impact through the channel of digital finance on poverty eradication and income inequality reduction at the sub-national level in India. They find that ICT innovation has no direct impact, though financial inclusion reduces inequality in both rural and urban areas. Interestingly, ICT diffusion in the banking sector dampens the positive role of financial inclusion on urban inequality reduction, whereas it has no impact on rural inequality. Ali et al. (Citation2021) examine the impact of financial inclusion on income inequality in a panel of 18 Asian countries over the period 1997–2017. They indicate that micro-level financial inclusion has a weak negative and statistically significant impact on income inequality. Macro-level index and all individual indicators of financial inclusion do not affect income inequality in the selected sample of economies. Asongu and Odhiambo (Citation2019) find a negative relationship between mobile, Internet, and broadband penetration and inequality in a panel of 48 African countries. Aginta et al. (Citation2018) constructs financial inclusion indicator and analyzes the link between financial inclusion and income inequality for 33 provinces in Indonesia. By using Fixed Effect Panel Model, they find that financial inclusion appears to have an insignificant effect on inequality at the national level.
Moreover, Agyemang-Badu et al. (Citation2018) did not find a significant relationship between income inequality and financial inclusion in Africa. Although there have been many reforms targeting the financial sector to trigger economic growth in Africa and reduce the income gap between rich and poor, they have significantly failed, and Africa remains the poorest and one of the most unequal regions in the world. For some authors, the positive redistributive effect of financial inclusion on income inequality could become negative due to its failure to reach the poor segments of the population (Haan & Sturm, Citation2017; Jauch & Watzka, Citation2016).
In sum, looking at the above‐mentioned empirical literature, we hypothesize that the wider use of digital technology can reduce inequality albeit the effect is heterogenous through WAEMU countries.
3. Methodology and data
3.1. Estimation strategy
The estimation strategy starts from the fact that most macroeconomic relations are dynamic in nature; this requires dynamic panel modelling (Baltagi, Citation2008). One of the advantages of estimating dynamic models is that the dynamics of adjustments are well captured. This study applies the pooled means group estimation (PMGE) (Cicchiello et al., Citation2021; Pesaran et al., Citation1999). The Panel analysis on the unrestricted specification of the autoregressive distributed lag model for time periods t = 1, 2, …., T and groups i = 1, 2, …, N is presented as follows:
where is a scalar-dependent variable,
is the k-1 vectors of explanatory variables for group i,
denotes fixed effects,
are scalar coefficients of the lagged dependent variables,
are vectors of k-1 coefficients.
The transformed form of EquationEquation (1)(1)
(1) can be formulated as follows:
It is assumed that the disturbance terms are distributed independently between i and t, with null averages and variances
. It is further assumed that
for all i. Thus, there is a long-term relationship between
and
which is defined by
where is the vector k × 1 of the long-term coefficients and
are stationary with possibly non-null averages (including fixed effects). Therefore, EquationEquation (3)
(3)
(3) can be written as follows:
where, is the error correction term given by EquationEquation (4)
(4)
(4) and therefore
is the coefficient of the error correction term measuring the adjustment speed to long-term equilibrium. This parameter should be significantly negative, which implies that the variables return to a long-term equilibrium.
The PMGE estimation method allows short-term coefficients, intercepts, and error variances to vary between countries but forces long-term coefficients to be equal. This implies that for all i. In order to estimate short-term coefficients and long-term common coefficients, Pesaran et al. (Citation1999) adopted the maximum likelihood estimation (MLE) approach assuming
disturbances are normally distributed. Estimators are denoted by:
;
;
;
and
To test the robustness of our results, we re-estimate the PMG on country groups by the level of development. So, we build two clusters, the first consists of Côte d’Ivoire, Senegal, Benin, and Burkina Faso, which are considered lower-middle-income countries. The second cluster includes Guinea Bissau, Mali, Niger, and Togo, which are considered low-income countries. In addition, we estimate a Dynamic Fixed Effect Panel model to compare the results of the PMG.
3.2. Data and construction of variables
To highlight the heterogeneous role of digital financial inclusion in reducing inequality, the study exploited data from many sources including the Central Bank of West African States (BCEAO), World Bank World Development Indicators (WDI), World Bank World Governance Indicators (WGI), and UNU‐WIDER World Income Inequality Database (WIID). For inequality measure, the study drawn the Gini index before tax and transfer (GINI) from the Standardized World Income Inequality Database [SWIID] proxy the income inequality. Despite some technical drawbacks (Jenkins, Citation2015), the literature generally acknowledges that the SWIID is one of the best available indices to proxy income inequality in a country (Gimpelson & Treisman, Citation2018). The institutions who produce the data ensure high levels of data quality by implementing high standards and methodologies, and also collect data from sources and definitions that are globally accepted. The data relate to the eight (08) WAEMU countries over the period 2007 to 2020. These countries belong to the same monetary zone.
The dependent variable used in this paper is the Gini coefficient, which is widely used to measure the Inequality of Outcome. Gini coefficient measures the extent to which the income distribution contrasts with the perfect equal distribution. Its value lies between 0 and 1, 0 indicates perfect equality, while 1 reflects perfect inequality in income distribution. The coefficient shows the comparison of income distribution among populations despite its sizes. A higher Gini coefficient presents a more unequal distribution. Gini coefficient has its limitation which is not easily separated into components. Also, a very distinct income distribution can provide the same coefficient. Despite its limitation, the Gini coefficient is still the most efficient proxy to use as an income inequality measure. Furthermore, the independent variables used in this paper are based on the issue paper of Digital Financial Inclusion and the Implications for Customers, Regulators, Supervisors, and Standard‐Setting Bodies from GPFI, a body which was established by the G20 (GPFI, Citation2014).
A number of control variables are also used. To capture the degree of macroeconomic instability, we use the inflation rate measured as percent change in consumer price index. Education captures the heterogeneity in human capital. This is in line with Abrigo et al. (Citation2018) who find that human capital investment has a stronger positive effect on labor productivity for poorer households and is being therefore beneficial for income equity. In addition, most studies found education to be an important factor that creates a wider income gap between the poor and the rich (Chongvilaivan & Kim, Citation2016; De Silva & Sumarto, Citation2013; dos Santos & da Cruz Vieira, Citation2013). However, some human capital models also acknowledge that the average years of schooling may have either positive or negative impact on income inequality due to differences in the rates of return on education (Lee & Lee, Citation2018). Moreover, the socio-political environment and the institutional diversity play a significant role and these aspects have to be taken into consideration when the income inequality is studied (Demir, Citation2016; Valentinov et al., Citation2015). Indeed, we use the rule of law and control of corruption (Fouejieu et al., Citation2020; Matekenya et al., Citation2021). According to Nguyen and Ha (Citation2021), institutional quality plays an important role in financial inclusion, which can lead to a reduction in economic inequality.
To control for differences in the degree of economic development, we also include GDP per capita levels (Fouejieu et al., Citation2020). STATA statistical software is used to analyze the data. After converting the data into a STATA‐compatible dataset, we then ordered it in panel form, where STATA indicated that it is a strongly balanced panel data. Tables present the descriptive results.
4. Results and discussion
4.1. Empirical results
The results provide statistical evidence about the effect of digital financial inclusion on inequality, using pool mean group estimator (PMGE). This approach allows us to evidence the heterogeneity in the relationship between digital financial inclusion and inequality among the countries of West African Economics and monetary Union (WAEMU). This heterogeneity effect, to the best of our knowledge, has not been fully addressed in the existing literature.
Table presents the results of the regression by the PMG estimator. We test the homogeneity of the long-run coefficients with the Hausman (Citation1978) test. The probabilities associated with the Hausman test are all greater than 5%; this does not reject the hypothesis of restriction of long-run homogeneity. All coefficients have the expected signs and are significantly different from zero at the 5% level. In both long and short term, digital finance is strongly correlated with inequality.
Table 1. Estimation results using PMG estimator
The advent of digital technology has globally a favorable impact on financial system, livelihood, poverty, as well as inequality. This is confirmed by the results of the PMG estimates which suggest that digital financial inclusion significantly reduces inequality within WAEMU’s countries. Indeed, in the long run, it appears that inequality could fall by almost 1.8%. Indeed, a one percent increase in the digital financial inclusion decreases the Gini coefficient by 1.8% for the whole WAEMU countries. However, the results suggest that in the short run, countries such as Benin and Niger are the most respondent to the effect of digital finance as far as inequality reduction is concerned, while Burkina and Mali are the most resilient. The results are inconclusive for the other WAEMU countries including Burkina Faso, the Ivory Coast, and Guinea and count intuitively positive for Mali. This diversity of the effect confirms the heterogeneity of the digital financial services on inequality measures in developing countries and particularly in WAEMU. This inconclusive result is in line with the results of Demir et al. (Citation2022) that find that financial inclusion reduces inequality in high- and upper-middle-income countries but not in lower-middle and low-income countries. However, the counterintuitive results would be to attribute to socio-political situation that widen income disparity in those countries. These heterogenous results highlight the important role played by digital technologies including Internet and mobile phone diffusion in the reduction of income inequalities in our modern societies. Furthermore, the long-run coefficients for the use of Internet and mobile are much larger in comparison to the short-run effects emphasizing the growing importance of these technological aspects in the future policies aiming at reducing income inequalities.
Our findings are consistent with the results obtained by other recent cross-country studies which, unlike ours, are based on supply-side data on financial inclusion (Asongu and Nwachukwu, Citation2018; Demir et al., Citation2022; Kim, Citation2016; Park & Mercado, Citation2018; Turégano & Herrero, Citation2018) or microfinance (Lacalle-Calderon et al., Citation2019). Our results are also in line with those of single-country studies that have found a negative relationship between household financial inclusion and (income or consumption) inequality in developing countries, such as Bangladesh (Khandker, Citation2005; Mahjabeen, Citation2008) and China (Zhang & Posso, Citation2019). The findings also complement those of Altunbas¸ & Thornton (Citation2019), according to which an increase in financial development is associated with an increase in income inequality, which suggest that mobile banking tends to reduce income inequality in relatively higher-income countries. Furthermore, the results show negative and statistically significant coefficients associated with the financial inclusion variable (TGUSF), suggesting a negative correlation between financial inclusion and income inequality. These results support those of Agyemang-Badu et al. (Citation2018) who found that financial inclusion is inversely related to both poverty and income inequality in Africa and thus recommended implementing policies and programs that strengthen formal financial inclusion of the poor.
As far as the control variables are concerned, they behave largely as expected. Like previous studies, we find that, together with financial inclusion, some variables significantly affect income inequality in WAEMU. The coefficients associated with GDP per capita are negative and statistically significant in most cases in the short run but positive and significant in the long run. This suggests that lower-income countries tend to face higher inequality compared to higher-income countries in the long run. However, building human capital via education can help alleviate inequality by improving access to employment and increasing wage. Digital finance such as the usage of mobile banking, Internet banking, or credit/debit card, however, is still confined among a handful of educated customers leading then to an increase in income inequality. However, good institutional quality contributes to ensure fairer distribution of wealth, and therefore can help reduce inequality. Indeed, in line with Le et al. (Citation2019) we find that rule of law and control of corruption are key in reducing inequality. In sum, our results indicate that digital financial inclusion is key in reducing inequality.
4.2. Robustness analysis
To check the robustness of our results, we use two approaches. The first is to estimate re-estimates of the PMG on the clusters of the WAEMU countries. The first cluster includes middle-income countries, namely Benin, Côte d’Ivoire, and Senegal (Table ). The second cluster includes low-income countries such as Burkina Faso, Guinea-Bissau, Mali, Niger, and Togo (Table ). The results of the PMG estimates for the two clusters support the overall negative long-run effect of the pandemic on the supply of bank credit in the WAEMU. However, this effect is negative for middle-income countries and positive for most low-income countries except Togo. Moreover, the magnitude of the effect is higher for middle-income countries. The second approach consists in estimating a Dynamic Fixed Effect (DFE) to see the consistency of the results (Table ). Indeed, the DFE is also appropriate for dynamic panels with a temporal dimension higher than the individual dimension, as in our case. Thus, the DFE estimates confirm the long-run effects of the digital financial inclusion on inequality in the WAEMU (Table )
5. Concluding remarks
A number of studies suggest that financial market imperfections, such as information asymmetries and transaction costs, prevent poor people from lifting from poverty, by limiting their access to formal financial services. But, with recent developments, digital finance is seen as a key enabler of financial inclusion and poverty and inequality alleviation. This article aims at evidencing the heterogeneity effect of digital financial inclusion on inequality in WAEMU. Using data from WDI, BCEAO, and SWIID, we estimated a pooled means group estimation (PMGE) and a dynamic fixed effect as a robustness test. The results indicate that, overall, digital financial inclusion leads to a decrease in income inequality. In the long run, there is a negative and significant effect of digital financial inclusion on inequality. The short run results evidenced more the heterogeneity effect of digital financial inclusion in WAEMU countries due to the diversity, inconclusiveness, and counterintuitive results of the effect of DFI on inequality.
Our findings provide two important policy implications. First, a tradeoff exists between financial inclusion, digital technology, and income inequality. Financial institutions serve as a good instrument to narrow income inequality. Policies should be centered primarily on eliminating obstacles and developing innovative digital products so that to get more people banked. By expanding financial inclusion and Fintech, financial institutions can gain sufficient funds to tackle the credit rationing problem. This measure will lessen capital constraints for small businesses and in fine reduce inequality. Second, there is also a need to provide good infrastructure, appropriate digital finance regulations. In fact, in most parts of Africa, it is still difficult and sometimes even impossible to transfer money and pay bills. A workable solution to benefit from Fintech is adopting a telecom-led regulatory model. Last but not least, good institutions conducive to control of corruption and rule of law should be promoted.
However, the limited recent data available for income inequality and digital financial inclusion may constitute a limitation of our study. The findings constitute an initial point of analysis of a topic that has been widely studied in the literature, especially in Sub-Saharan Africa. Future research should use longer-term data with more depth and coverage on inequality and digital financial inclusion indicators.
Disclosure statement
No potential conflict of interest was reported by the author(s).
Additional information
Notes on contributors
Kolotioloman Soro
Kolotioloman Soro (PhD) is an assistant Professor in the university Peleforo GON COULIBALY (Ivory Coast). His research interests revolve around economic development issues including gender, poverty and inequality.
Melain Modeste Senou
Melain Modeste Senou (PhD) is a post-doctoral research fellow at the African Centre of Excellence for Inequality Research in the University of Ghana. Dr SENOU is an alumnus of the African Economic Research Consortium (AERC), Nairobi. His research interests revolve around economic development issues including mainly inclusive finance, poverty and inequality.
References
- Abrigo, M. R., Lee, S. H., & Park, D. (2018). Human capital spending, inequality, and growth in middle-income Asia. Emerging Markets Finance and Trade, 54(6), 1285–17. https://doi.org/10.1080/1540496X.2017.1422721
- Aghion, P., & Bolton, P. (1997). A theory of trickle-down growth and development. The Review of Economic Studies, 64(2), 151–172. https://doi.org/10.2307/2971707
- Aghion, P., & Bolton, P. (2005). A theory on trickle-down growth and development. The Review of Economic Studies, 64(2), 151–172. https://doi.org/10.2307/2971707
- Aginta, H., Soraya, D. A., & Santoso, W. B. (2018). Financial development and income inequality in Indonesia: A sub-national level analysis. Economics and Finance in Indonesia, 64(2), 111–130.
- Agyemang-Badu, A. A., Agyei, K., & Kwaku Duah, E. (2018). Financial inclusion, poverty and income inequality: Evidence from Africa. Spiritan International Journal of Poverty Studies, 2(2), 1–19.
- Ali, M. A., Alam, K., Taylor, B., & Rafiq, S. (2019). Do income distribution and socio-economic inequality affect ICT affordability? Evidence from Australian household panel data. Economic Analysis & Policy, 64(2019), 317–328. https://doi.org/10.1016/j.eap.2019.10.003
- Ali, J., Khan, M. A., Wadood, M., & Khan, U. S. (2021). Revisiting financial inclusion and income inequality Nexus: Evidences from selected economies in Asia. The Journal of Asian Finance, Economics & Business, 8(12), 19–29.
- Altunbas¸, Y., & Thornton, J. (2019). The impact of financial development on income inequality: A quantile regression approach. Economics Letters, 175, 51–56. https://doi.org/10.1016/j.econlet.2018.12.030
- Ashenafi, B. B., & Dong, Y. (2022). Financial inclusion, Fintech, and income inequality in Africa. FinTech, 1(4), 376–387. https://doi.org/10.3390/fintech1040028
- Aslan, G., Deléchat, C., Newiak, M. M., & Yang, M. F. (2017). Inequality in financial inclusion and income inequality. International Monetary Fund Working Paper Series, 17(17/236), 1. https://doi.org/10.5089/9781484324905.001
- Asongu, S. A., & Nwachukwu, J. C. (2018). Comparative human development thresholds for absolute and relative pro-poor mobile banking in developing countries. Information Technology & People, 31(1), 63–83. https://doi.org/10.1108/ITP-12-2015-0295
- Asongu, S. A., & Odhiambo, N. M. (2018). Human development thresholds for inclusive mobile banking in developing countries. African Journal of Science, Technology, Innovation & Development, 10(6), 735–744. https://doi.org/10.1080/20421338.2018.1509526
- Asongu, S. A., & Odhiambo, N. M. (2019). How enhancing information and communication technology has affected inequality in Africa for sustainable development: An empirical investigation. Sustainable Development, 27(4), 647–656. https://doi.org/10.1002/sd.1929
- Baltagi, B. (2008). Econometric analysis of panel data (Vol.1). John Wiley & Sons.
- Banerjee, A. V., & Newman, A. F. (1993). Occupational choice and the process of development. Journal of Political Economy, 101(2), 274–298. https://doi.org/10.1086/261876
- Bartlett, R., Morse, A., Stanton, R., & Wallace, N. (2019). Consumer‐lending discrimination in the FinTech era. 1–51. https://www.nber.org/papers/w25943.pdf
- Chongvilaivan, A., & Kim, J. (2016). Individual income inequality and its drivers in Indonesia: A Theil decomposition reassessment. Social Indicators Research, 126(1), 79–98. https://doi.org/10.1007/s11205-015-0890-0
- Cicchiello, A. F., Kazemikhasragh, A., Monferrá, S., & Girón, A. (2021). Financial inclusion and development in the least developed countries in Asia and Africa. Journal of Innovation and Entrepreneurship, 10(1), 1–13. https://doi.org/10.1186/s13731-021-00190-4
- Dabla-Norris, E., Ji, Y., Townsend, R. M., & Unsal, D. F. (2015). Identifying constraints to financial inclusion and their impact on GDP and inequality: A structural framework for policy. International Monetary Fund.
- Dabla-Norris, E., Ji, Y., Townsend, R. M., & Unsal, D. F. (2021). Distinguishing constraints on financial inclusion and their impact on GDP, TFP, and the distribution of income. Journal of Monetary Economics, 117, 1–18. https://doi.org/10.1016/j.jmoneco.2020.01.003
- Das, S., Chatterjee, A., Banerjee, R. B., & Bhattacharya, T. (2021). Role of ICT dissemination and digital finance in poverty eradication and income inequality reduction: A sub-national level study from India. Madras School of Economics.
- Demir, F. (2016). Effects of FDI flows on institutional development: Does it matter where the investors are from? World Development, 78, 341–359. https://doi.org/10.1016/j.worlddev.2015.10.001
- Demirguc-Kunt, A., Klapper, L., & Singer, D. (2017). Financial inclusion and inclusive growth a review of recent empirical evidence. Development research group finance and private sector development team. Policy Research Working Paper No. 8040. World Bank. http://documents.worldbank.org/curated/en/403611493134249446/Financial-inclusionand-inclusive-growth-a-review-of-recent-empirical-evidence.
- Demir, A., Pesqué-Cela, V., Altunbas, Y., & Murinde, V. (2022). Fintech, financial inclusion and income inequality: A quantile regression approach. European Journal of Finance, 28(1), 86–107. https://doi.org/10.1080/1351847X.2020.1772335
- De Silva, I., & Sumarto, S. (2013). Poverty-growth-inequality triangle: The case of Indonesia. TNP2K Working Paper 04 - 2013. Tim Nasional Percepatan Penanggulangan Kemiskinan. http://www.tnp2k.go.id/images/uploads/downloads/WP4-PovertyTriangle(1).pdf.
- dos Santos, V. F., & da Cruz Vieira, W. (2013). Effects of growth and reduction of income inequality on poverty in Northeastern Brazil, 2003-2008. Economia Aplicada, 17(4), 647–666. https://doi.org/10.1590/S1413-80502013000400006
- Fouejieu, A., Sahay, R., Cihak, M., & Chen, S. (2020). Financial inclusion and inequality: A cross-country analysis. The Journal of International Trade & Economic Development, 29(8), 1018–1048. https://doi.org/10.1080/09638199.2020.1785532
- Galor, O., & Moav, O. (2004). From physical to human capital accumulation: Inequality and the process of development. Review of Economic Studies, 71(4), 1001–1026. https://doi.org/10.1111/0034-6527.00312
- Galor, O., & Zeira, J. (1993). Income distribution and macroeconomics. Revista Economica, 60(1), 35–52. https://doi.org/10.2307/2297811
- Gimpelson, V., & Treisman, D. (2018). Misperceiving inequality. Economics & Politics, 30(1), 27–54. https://doi.org/10.1111/ecpo.12103
- Girón, A., Kazemikhasragh, A., Cicchiello, A. F., & Panetti, E. (2022). Financial inclusion measurement in the least developed countries in Asia and Africa. Journal of the Knowledge Economy, 13(2), 1198–1211. https://doi.org/10.1007/s13132-021-00773-2
- GPFI. (2014, October). Issues paper digital financial inclusion and the implications for customers.
- GPFI. (2016). G20 high-level principles for digital financial inclusion. https://www.gpfi.org/sites/gpfi/files/G20%20High%20Level%20Principles%20for%20Digital%20Financial%20Inclusion.pdf
- Greenwood, J., & Jovanovic, B. (1990). Financial development, growth, and the distribution of income. Journal of Political Economy, 98(5, Part 1), 1076–1107. https://doi.org/10.1086/261720
- Haan, J., & Sturm, J. (2017). Finance and income inequality: A review and new evidence. European Journal of Political Economy, 50, 171–195. https://doi.org/10.1016/j.ejpoleco.2017.04.007
- Hausman, J. A. (1978). Specification tests in econometrics. Econometrica, 46(6), 1251. https://doi.org/10.2307/1913827
- Jauch, S., & Watzka, S. (2016). Financial development and income inequality: A panel data approach. Empirical Economics, 51(1), 291–314. https://doi.org/10.1007/s00181-015-1008-x
- Jenkins, S. P. (2015). World income inequality databases: An assessment of WIID and SWIID. Journal of Economic Inequality, 13(4), 629–671. https://doi.org/10.1007/s10888-015-9305-3
- Ji, X., Wang, K., Xu, H., & Li, M. (2021). Has digital financial inclusion narrowed the urban-rural income gap: The role of entrepreneurship in China. Sustainability, 13(15), 8292. https://doi.org/10.3390/su13158292
- Kazemikhasragh, A., Cicchiello, A. F., Monferrá, S., & Girón, A. (2022). Gender inequality in financial inclusion: An exploratory analysis of the Middle East and North Africa. Journal of Economic Issues, 56(3), 770–781. https://doi.org/10.1080/00213624.2022.2079936
- Khandker, S. R. (2005). Microfinance and poverty: Evidence using panel data from Bangladesh. The World Bank Economic Review, 19(2), 263–286. https://doi.org/10.1093/wber/lhi008
- Khera, P., Ng, S., Ogawa, S., & Sahay, R. (2022). Measuring digital financial inclusion in emerging market and developing economies: A new index. Asian Economic Policy Review, 17(2), 213–230. https://doi.org/10.1111/aepr.12377
- Kim, J. H. (2016). A study on the effect of financial inclusion on the relationship between income inequality and economic growth. Emerging Markets Finance and Trade, 52(2), 498–512. https://doi.org/10.1080/1540496X.2016.1110467
- Kling, G., Pesqué-Cela, V., Tian, L., & Luo, D. (2022). A theory of financial inclusion and income inequality. European Journal of Finance, 28(1), 137–157. https://doi.org/10.1080/1351847X.2020.1792960
- Lacalle-Calderon, M., Larrú, J. M., Garrido, S. R., & Perez-Trujillo, M. (2019). Microfinance and income inequality: New macro level evidence. Review of Development Economics, 23(2), 860–876. https://doi.org/10.1111/rode.12573
- Lee, J. W., & Lee, H. (2018). Human capital and income inequality. Journal of the Asia Pacific Economy, 23(4), 554–583. https://doi.org/10.1080/13547860.2018.1515002
- Le, H. Q., Ho, H. L., & Mai, N. C. (2019). The impact of financial inclusion on income inequality in transition economies. Management Sciences Letters, 9, 661–672. https://doi.org/10.5267/j.msl.2019.2.005
- Li, M. C., Feng, S. X., & Xie, X. (2022). Spatial effect of digital financial inclusion on the urban–rural income gap in China—analysis based on path dependence. Economic Research-Ekonomska Istraživanja, 36(2), 1–22. https://doi.org/10.1080/1331677X.2022.2106279
- Mahjabeen, R. (2008). Microfinancing in Bangladesh: Impact on households, consumption and welfare. Journal of Policy Modeling, 30(6), 1083–1092. https://doi.org/10.1016/j.jpolmod.2007.12.007
- Matekenya, W., Moyo, C., & Jeke, L. (2021). Financial inclusion and human development: Evidence from Sub-Saharan Africa. Development Southern Africa, 38(5), 683–700. https://doi.org/10.1080/0376835X.2020.1799760
- Meniago, C., & Asongu, S. A. (2018). Revisiting the finance-inequality nexus in a panel of African countries. Research in International Business and Finance, 46, 399–419. https://doi.org/10.1016/j.ribaf.2018.04.012
- Mookherjee, D., & Ray, D. (2003). Persistent inequality. Review of Economic Studies, 70(2), 369–393. https://doi.org/10.1111/1467-937X.00248
- Neaime, S., & Gaysset, I. (2018). Financial inclusion and stability in MENA: Evidence from poverty and inequality. Finance Research Letters, 24, 230–237. https://doi.org/10.1016/j.frl.2017.09.007
- Nguyen, Y. H. D., & Ha, D. T. T. (2021). The effect of institutional quality on financial inclusion in ASEAN countries. The Journal of Asian Finance, Economics & Business, 8(8), 421–431.
- Omar, M. A., & Inaba, K. (2020). Does financial inclusion reduce poverty and income inequality in developing countries? A panel data analysis. Journal of Economic Structures, 9(1), 37. https://doi.org/10.1186/s40008-020-00214-4
- Ouechtati, I. (2020). The contribution of financial inclusion in reducing poverty and income inequality in developing countries. Asian Economic and Financial Review, 10(9), 1051–1061. https://doi.org/10.18488/journal.aefr.2020.109.1051.1061
- Park, C.-Y., & Mercado, R. (2018). Financial inclusion, poverty, and income inequality. The Singapore Economic Review, 63(1), 185–206. https://doi.org/10.1142/S0217590818410059
- Pesaran, M. H., Shin, Y., & Smith, R. P. (1999). Pooled mean group estimation of dynamic heterogeneous panels. Journal of the American Statistical Association, 94(446), 621–634. https://doi.org/10.1080/01621459.1999.10474156
- Philippon, T. (2019). On FinTech and financial inclusion. National Bureau of Economic Research. https://doi.org/10.4337/9781788114226.00024
- Polloni‑Silva, E., da Costa, N., Moralles, H. F., & Neto, M. S. (2021). Does financial inclusion diminish poverty and inequality? A panel data analysis for Latin American countries. Social Indicators Research, 158(3), 889–925. https://doi.org/10.1007/s11205-021-02730-7
- Ratnawati, K. (2020). The impact of financial inclusion on economic growth, poverty, income inequality, and financial stability in Asia. The Journal of Asian Finance, Economics & Business, 7(10), 73–85. https://doi.org/10.13106/jafeb.2020.vol7.no10.073
- Senou, M. M., Ouattara, W., & Acclassato Houensou, D. Is there a bottleneck for mobile money adoption in WAEMU?. (2019). Transnational Corporations Review, 11(2), 143–156. https://doi.org/10.1080/19186444.2019.1641393
- Senou, M. M., Ouattara, W., Acclassato Houensou, D., & Rossi, S. Financial inclusion dynamics in WAEMU: Was digital technology the missing piece?. (2019). Cogent Economics & Finance, 7(1), 1665432. https://doi.org/10.1080/23322039.2019.1665432
- Senou, M. M., & Soro, K. (2023). Reexaming the income inequality and digital financial inclusion nexus. Empirical evidence in WAEMU. The Empirical Economics Letters, 12(5), 27–32.
- Shen, L., Zhang, X., & Liu, H. (2022). Digital technology adoption, digital dynamic capability, and digital transformation performance of textile industry: Moderating role of digital innovation orientation. Managerial and Decision Economics, 43(6), 2038–2054. https://doi.org/10.1002/mde.3507
- Tchamyou, V. S., Erreyger, G., & Cassimon, D. (2019). Inequality, ICT and financial access in Africa. Technological Forecasting and Social Change, 139, 169–184. https://doi.org/10.1016/j.techfore.2018.11.004
- Turégano, D. M., & Herrero, A. G. (2018). Financial inclusion, rather than size, is the key to tackling income inequality. The Singapore Economic Review, 63(1), 167–184. https://doi.org/10.1142/S0217590818410047
- Valentinov, V., Hielscher, S., & Pies, I. (2015). Nonprofit organizations, institutional economics, and systems thinking economic systems. Economic Systems, 39(3), 491–501. https://doi.org/10.1016/j.ecosys.2014.12.002
- Yao, L., Ma, X., & Ughetto, E. (2022). Has digital finance widened the income gap? PLoS ONE, 17(2), e0263915. https://doi.org/10.1371/journal.pone.0263915
- Yu, N., & Wang, Y. (2021). Can digital inclusive finance narrow the Chinese urban–rural income gap? The perspective of the regional urban–rural income structure. Sustainability, 13(11), 6427. https://doi.org/10.3390/su13116427
- Zhang, Q., & Posso, A. (2019). Thinking inside the box: A Closer look at financial inclusion and household income. The Journal of Development Studies, 55(7), 1616–1631. https://doi.org/10.1080/00220388.2017.1380798
Appendices
Table A1. Descriptive statistics
Table A2. Mean difference test
Table A3. PMG for middle-income countries (Benin, Côte d’Ivoire et Sénégal)
Table A4. PMG for low-income countries (Burkina Faso, Guinea, Mali, Niger, and Togo)
Table A5. Dynamics fixed effect