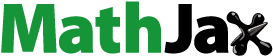
Abstract
The purpose of this study is to estimate the relationship and influence between regional inequality variables, human capital, the open unemployment rate, and economic growth in Indonesia using panel data. The panel data consists of a combination of time series data from the 2010–2020 period, based on information from 32 provinces in Indonesia. The estimation model employed in this study is a panel regression model utilizing three methods: the common effect model (CEM), fixed effect model (FEM), and random effect model (REM). The findings of the study reveal a positive and significant effect of the ETI factor on the LOG (GRDP) factor. Additionally, the HDI variable exhibits a positive and significant impact on the LOG (GRDP) variable, while the UNR variable also shows a positive and significant effect on the LOG (GRDP) variable. Furthermore, the ETI variable is found to have a positive and significant influence on the UNR variable, while the HDI variable has a negative and significant impact on the UNR variable. Finally, the LOG (GRDP) variable demonstrates a positive and significant effect on the UNR variable.
Public Interest Statement
Over the last 20 years, researchers have been actively investigating the connection between regional inequality and economic growth, making it a crucial topic of study. They have used various measures like disparities, mismatch, inequality, and imbalance to understand how different human activities vary across regions. Depending on the context, such as convergence, polarization, concentration, dispersion, and hierarchy, these indicators are analyzed to gain deeper insights. The research findings suggest that reducing economic inequality requires sustained efforts, while short-term strategies can also be explored to address regional disparities. Although there is a considerable amount of literature exploring the relationship between economic growth and regional inequality, there is still a lack of research incorporating essential variables like human capital and unemployment. This study stands out as it addresses this gap and brings together all these critical macroeconomic variables for a comprehensive analysis.
1. Introduction
The correlation between economic growth and inequality has received extensive attention in research and remains a subject of ongoing investigation (Fan & Sun, Citation2008; Panizza, Citation2002; Partridge, Citation2005; Adrián & Sánchez, Citation2012). According to Chen (Citation2003), the long-term relationship between income distribution and economic growth follows an inverted U-shaped pattern (Panzera & Postiglione, Citation2020, Citation2022). This suggests that higher inequality occurs at the beginning of the period, with growth deteriorating, as pointed out by Alesina and Rodrik (Citation1994), Perotti (Citation1994, Citation1996), and Torsten and Tabellini (Citation1994), implying a negative correlation between inequality and growth. However, this consensus is gradually weakening, as negative relationships are primarily observed in developing countries, while no significant relationship is found in affluent countries. On the contrary, a contrasting and diverse consensus seems to exist, where some studies suggest that inequality can actually stimulate economic growth (Arjona et al., Citation2003; Forbes, Citation2000). Regional inequality has become a significant focus of academic research and a matter of concern for governments, as it poses a threat to national unity and social stability (Heidenreich & Wunder, Citation2008; Wei, Citation1999). In the context of Indonesia, regional inequality has emerged as a primary concern for policymakers. The broader concept of spatial inequality, which refers to disparities between different regions within a country, has become a major issue for policymakers globally (Fan & Sun, Citation2008; Fan et al., Citation2009).
Development economists and policymakers are particularly worried about the widening regional inequality during the early stages of economic growth (Enflo & Rosés, Citation2015). The relationship between income inequality, asset distribution, and long-term growth has been extensively studied by researchers like Alesina and Rodrik (Citation1994), Torsten and Tabellini (Citation1994), and Castelló and Doménech (Citation2002). However, income inequality alone is not sufficient to measure overall welfare inequality, as other variables, such as human capital, play a crucial role in determining wealth and growth. Therefore, in various analytical models examining the relationship between inequality and economic growth, the role of human resources, especially their contribution to income distribution through human capital, is considered essential. Human capital plays a crucial role in driving economic growth, but directly measuring it is challenging, often necessitating the use of proxy indicators based on education data (Galor, Citation2005, Citation2012; Hippe & Baten, Citation2012; Husnah & Adam, Citation2022). Disparities between regions stem from multiple factors, including infrastructure and accessibility, competitiveness of local businesses, foreign investment volume, availability of skilled labor, utilization of local resources, proximity to key markets, labor migration patterns, and remittance inflows. Growing inequality is a common phenomenon in developing economies (Istrate & Horea-Serban, Citation2016).
In 2020, Indonesia’s Human Development Index (HDI) reached 71.94, growing by 0.03 percent compared to the previous year. The slowdown in HDI growth was primarily due to a decline in adjusted average per capita expenditure, dropping from Rp11.30 million in 2019 to Rp11.01 million in 2020. In the education sector, 7-year-old children had an expected education duration of 12.98 years, almost equivalent to a Level I diploma. Moreover, the average schooling duration for the population in 2020 was 8.48 years. In terms of health, the life expectancy for babies born in 2020 was 71.47 years. However, Indonesia’s economic performance contracted by 2.07 percent (c to c) in 2020 compared to 2019. The transportation and warehousing sector experienced the deepest growth contraction, at 15.04 percent. Additionally, almost all components on the expenditure side contracted, including the export of goods and services, which decreased by 7.70 percent. As of 2020, the spatial structure of the Indonesian economy was still dominated by the provincial group on the island of Java, contributing 58.75 percent, but experiencing a growth contraction of (2.51) percent (Official BPS News of Citation2021).
Initially, regional inequalities, both socially and economically, were triggered by societal transformation or economic growth, but in subsequent stages, there was a relative and/or absolute decrease in inequality (Sala-I-Martin, Citation1996; Zaman et al., Citation2013). Several theories propose that regional inequality is a natural consequence arising from variations in physical and geographical conditions between regions or countries (Sachs, Citation2006). Conversely, other perspectives support the idea of development emanating from the center and extending to the periphery through economies of scale, product differentiation, and transportation costs (Krugman, Citation1991). Consequently, Piketty (Citation2014) argues that it is becoming increasingly evident that the law of the economic market does not lead to a process of convergence and reduction of inequality; instead, developed regions tend to attract more capital and labor than less developed regions (Istrate & Horea-Serban, Citation2016). Regional development, particularly the issue of regional inequality, is a significant field of interest for both academic research and government policy. However, theorists have long held differing opinions regarding the root causes of regional inequality and have engaged in debates surrounding regional policies. Advocates of various theories have examined the impacts of institutional change, globalization, agglomeration, and technology on regional development (Krugman, Citation1991; Wei, Citation2002). Empirical studies have also shown variations in the effects of these variables.
Some countries have witnessed a decrease in regional inequality, while others continue to face persistent or even increasing disparities. The fast-paced global and domestic changes have amplified the discussions on inequality. Additionally, fierce debates have arisen concerning whether former socialist countries experienced a decline in regional inequality following economic reforms or if inequality has instead widened. These debates have far-reaching implications, sparking discussions about development policies among policymakers and even resulting in power struggles. Regional inequality becomes a focal point for reformists to justify their political agenda. The issue of inequality is closely linked to economic growth, social stability, ethnic relations, and political cohesion. The topic of regional inequalities is extensively discussed from various perspectives, including equity and efficiency, the role of countries and markets, global and local implications, and the disparities between the rich and the poor (Wei, Citation2002). Regional inequality analysis has gained significant attention in the last two decades, attracting considerable interest from researchers (Benedek & Veress, Citation2013; Kuttor, Citation2009). Inequality, often referred to as disparity, non-conformance, imbalance, or inequality, has been extensively utilized to identify, measure, and analyze the intensity of various human activities using specific indicators (Avram & Postoiu, Citation2016). Depending on the context being studied, different terms such as convergence (Rey & Janikas, Citation2005), polarization, concentration, dispersion, and hierarchy (Boldea et al., Citation2012) are used to describe inequality.
The literature extensively explores the role of space in measuring regional inequality (Benedek, Citation2015; Chirila & Chirila, Citation2014; Jencova et al., Citation2015). Researchers in this field recognize that reducing economic inequality is a long-term process, and a realistic perspective on inequality reduction can be gained by analyzing short-term trends. Moreover, the literature extensively explores the relationship between economic growth and unemployment (Kurz & Salvadori, Citation1997; Pambayun, Citation2021). Classical Economics mainly concentrates on short-term growth and pays little attention to immediate economic conditions. On the other hand, Neoclassical Economists share similar viewpoints with Classical thought but focus more on intertemporal analysis, particularly concerning physical investment and human capital. Grossman and Helpman (Citation1994) describe two primary characteristics of growth theory. Firstly, output expansion has surpassed population growth in the two centuries since the industrial revolution. Secondly, countries experiencing growth tend to maintain distinct growth trajectories for relatively long periods due to disparities in capital accumulation. However, Campos (Citation2001) and Staehr (Citation2003) find that traditional growth factors do not significantly influence the transition to economic growth.
Postel-Vinay (Citation1998) argues that technological advancements help reduce unemployment due to the capitalization effect. Rapid economic growth leads to increased company returns and profitability, leading to the creation of more job opportunities. However, rapid innovation may also result in unemployment as workers adapt to changing job demands. Growth and technological progress have played crucial roles in reducing unemployment, but these benefits remain concentrated in a few regions, leading to existing regional disparities. Various theories attempt to explain the problem of unemployment, and multiple aspects or factors influence this issue. Rational unemployment theory should consider mechanisms to eventually bring the economy back to its normal level of unemployment (Lindbeck, Citation1991). Additionally, Martin (Citation1997) focuses on regional unemployment, as regional inequality and unemployment tend to vary throughout the business cycle.
The research conducted by various scholars, such as Adrián and Sánchez (Citation2012), Panizza (Citation2002), Partridge (Citation2005), Fan and Sun (Citation2008), Panzera and Postiglione (Citation2020, 2021), Chen (Citation2003), Alesina and Rodrik (Citation1994), Perrotti (Citation1994, Citation1996), Torsten and Tabellini (Citation1994), has primarily focused on examining the relationship between economic growth and inequality. Their studies consistently found a negative correlation between inequality and growth. In contrast, the research carried out by Kurz and Salavdori (Citation1997) exclusively investigated the link between growth and unemployment. However, it is important to note that human capital is widely recognized as one of the most critical determinants of economic growth, as evidenced by the findings of Hippe and Baten (Citation2012) and Galor (Citation2005, Citation2012). Additionally, Postel-Vinay (Citation1998) discovered that technological progress contributes to reducing unemployment through capitalization effects. Rapid economic growth boosts company returns and stimulates the establishment of new businesses, generating various advantages. Nevertheless, fast-paced innovation may also lead to unemployment as the workforce adapts to changing job requirements. Overall, growth and technological advancements have played a crucial role in minimizing unemployment. Unemployment is a complex issue, and multiple theories attempt to explain its dynamics, with various factors influencing the problem. Rational unemployment theories should consider both fast and slow mechanisms to bring the economy back to its natural unemployment rate (Lindbeck & Snower, Citation1989). As a result, this research addresses a gap that previous studies have not explored, by incorporating variables overlooked in the context of the relationship between economic growth and inequality, namely, human capital and unemployment variables that were not tested by previous researchers.
The research findings significantly contribute to the existing knowledge by demonstrating the interconnections between regional disparities, human resources, unemployment, and economic growth in Indonesia. Policymakers could benefit from these outcomes for devising strategies to decrease regional gaps, enhance human capabilities, and lessen unemployment ratios, ultimately promoting Indonesia’s economic growth. Furthermore, these conclusions could expand our knowledge of economic development and regional variations in developing countries.
2. Literature review
Various economic theories propose different outcomes for convergence or divergence over time (Gardiner et al., Citation2012). Some theories are based on the assumption that the rate of economic growth is influenced by differences in endowment resources, while others focus on production factors such as capital accumulation or economic agglomeration activities (Rey & Janikas, Citation2005). In explaining the factors that influence the economy and the implications for convergence or divergence, at least three competing economic theories exist. Solow’s (Citation1956) growth model is one of them, based on the assumption that capital, labor, and technological progress exogenously drive economic growth. The production function proposed by Solow (Citation1956) follows a constant return to scale model, allowing the model to be expressed in terms of worker requirements. This model indicates that the capital stock per worker determines the economic output per worker. As capital is assumed to have diminishing returns to scale, regions with higher levels of capital stock receive lower returns from investment compared to regions with lower capital stock. Consequently, regions with the same level of technology and exogenous technological progress are expected to converge, as capital flows to poorer regions, leading to higher returns and faster economic growth. Eventually, this higher rate of economic growth helps these regions catch up to the wealthier ones over time. The application of the Solow model to regions within the European Union has yielded mixed evidence.
Sala-I-Martin (Citation1996) suggests that the Solow growth model can be applied to the European Union region, and the convergence technique supports the findings of convergence between regions. However, Heidenreich and Wunder (Citation2008) discovered a different pattern when observing regions within the European Union using the Gini coefficient, which is inconsistent with the results of the Solow model. Similarly, the findings from Puga (Citation2002) confirm that the Solow model is insufficient in explaining regional growth in the European Union, as it fails to account for the persistent level of regional income inequality. An alternative growth theory, the endogenous growth theory proposed by Romer (Citation1990), implies continuous differences between countries and regions. This theory suggests that economic growth stems from technological progress, which depends on investments in innovation, the diffusion of innovation, and the effectiveness of protecting innovation (Gardiner et al., Citation2012). Consequently, this growth model exhibits increasing returns to scale. As a result, differences in technology levels between countries or regions can lead to variations in economic development achieved. Moreover, with the process of technological progress, countries or regions that take advantage of past advancements are likely to maintain their lead, leading to persistent divergences between countries or regions, as explained by Heidenreich and Wunder (Citation2008) in relation to the divergence pattern of economic activity observed in the European Union.
The theory of new economic geography (NEC) posits that industrial production will concentrate in specific core regions (Fujita et al., Citation2001). This concentration in certain areas brings economic agglomeration benefits, leading to increasing returns to scale (Puga, Citation2002). As activities become concentrated in these core regions, they become more cost-effective, attracting other companies and workers due to the area’s market potential. This market-centric pattern fosters access to knowledge and skills, leading to self-reinforcement that further attracts economic activity to the core regions. However, centrifugal forces such as congestion and high land rents prevent all economic activity from concentrating in a single location, resulting in some core areas being surrounded by poorer suburbs or peripheries (Krugman, Citation1998). This NEC theory predicts that regional income will experience divergence, with industrial concentration in the core regions and relative backwardness in the periphery. Puga (Citation2002) suggests that this theory can explain the observed income and employment level divergences across EU regions over time (Doran & Jordan, Citation2013).
There is an extensive body of literature exploring the correlation between regional inequality and economic growth. Researchers have used various approaches to investigate the impact of inequality on growth performance. Alesina and Rodrik (Citation1994) and Torsten and Tabellini (Citation1994) employed a cross-sectional growth regression model with individual income data to examine the initial increase in inequality’s effect on the average growth rate of per capita income, along with other control variables. Chen (Citation2003) conducted a cross-sectional analysis, exploring the inverse U-shaped phenomenon in the relationship between initial income distribution and long-term economic growth. On the other hand, Li and Zou (Citation1998), Forbes (Citation2000), and Panizza (Citation2002) used panel data and the fixed-effect model with the general moment method (GMM) to estimate the relationship between inequality and economic growth. Partridge (Citation2005) introduced an estimation model that distinguishes the long-term and short-term effects of inequality and economic growth. However, different studies have yielded varied results regarding the impact of inequality on economic growth, largely influenced by the data and methodologies used. Some empirical studies across countries support the negative impact of inequality on growth (Alesina & Rodrik, Citation1994; Torsten & Tabellini, Citation1994), while other research points to a positive relationship (Forbes, Citation2000; Li & Zou, Citation1998). Additionally, Panizza (Citation2002) found a negative relationship between inequality and economic growth, noting that the relationship changed only due to minor alterations in the data or econometric model specifications. In the field of regional inequality and its relationship with economic growth and development rates, various research contributions have suggested different approaches (Atif, Citation2021; Lessmann, Citation2014; Lessmann & Seidel, Citation2017; Petrakos et al., Citation2005). Additionally, Henderson et al. (Citation2018) have conducted research on the inequality in economic distribution related to geographical characteristics and variables associated with the level of development.
Both developed and underdeveloped countries recognize the crucial significance of growth. Implementing sustainable growth strategies and job creation policies become essential measures to tackle the pressing issue of unemployment. Growth plays a vital role in advancing human progress and fostering prosperity, as it contributes to raising the overall standard of living. Developing countries aspire to achieve higher growth rates through accelerated efforts. However, these countries often face challenging economic conditions, marked by inadequate infrastructure, low literacy rates, minimal investment, and unstable governments, heavily relying on the Agricultural Sector. In the early growth theories, the problem of unemployment was not considered a significant and strategic concern (Hussain et al., Citation2010). Classical and neoclassical economics view unemployment as a rigid condition in the labor market, caused by external factors such as wage laws, taxes, and regulations that limit the hiring of workers. On the other hand, the Keynesian view focuses on unemployment arising from the ineffectiveness of demand for goods and services in the economy. According to Blanchard et al. (Citation1997), conventional growth theory suggests that unemployment is not influenced by the unemployment growth rate, and long-term growth only affects the balance of unemployment. Meanwhile, Romer (Citation1990) discovered that growth brings about inter-sectoral changes, leading to shifts in the economic sector’s structure and causing structural unemployment. Technological innovations alter production methods, leading to joblessness for workers whose skills become outdated. Structural changes can lead to job losses in certain firms, resulting in high job turnover due to new production technologies. As a consequence, faster economic growth can lead to unemployment through the displacement of skills by new machines. Unemployment remains a critical issue, and Lin (Citation2004) found that developing countries sometimes adopt inappropriate capital-intensive policies that prioritize certain sectors without the ability to compete in the market. This condition leads to policy distortions and failures in achieving growth.
3. Methods
3.1. Data source
The data utilized in this study is obtained from the provinces in Indonesia, as released by the Central Statistics Agency (BPS) for the period of 2010–2020. The research relies on panel data, which is a secondary data source from various provinces in Indonesia, including Nanggroe Aceh Darussalam, North Sumatra, South Sumatera, West Sumatra, Bengkulu, Riau, the Riau Islands, Jambi, Lampung, Bangka Belitung, West Kalimantan, East Kalimantan, South Kalimantan, Central Kalimantan, North Kalimantan, Banten, Jakarta, West Java, Central Java, Yogyakarta Istimewa Region, East Java, Bali, East Nusa Tenggara, West Nusa South, Gorontalo, West Sulawesi, Central Sulawesi, North Sulawesi, Southeast South Sulawesi, Maluku North, and West Papua. The panel data comprises a combination of cross-sectional data and time series. For the regional inequality data, the study employs the Entropy Theil Index formulation model to calculate the relevant measures.
3.2. Model specification
The study utilizes panel regression models to examine the correlation between regional disparities, human capital, unemployment, and Indonesia’s economic growth. Panel data offers advantages in addressing issues related to regional and temporal heterogeneity, as well as in examining the impact of unobserved variables on Indonesia’s economic growth. Moreover, it aids in tackling challenges such as multicollinearity and endogeneity that often arise in research on Indonesian economic growth. To ensure precise and reliable results, the study adopts appropriate estimation methods and conducts tests such as normality tests, heteroskedasticity tests, and autocorrelation tests.
Fischer (Citation2011) puts forward a model that investigates the correlation between regional inequality and economic growth, utilizing the Gini index as a measure of regional inequality, as introduced by Rey and Smith (Citation2013). However, the examination of the relationship between inequality and economic growth in this study draws on crucial aspects of the model developed by De Dominicis (Citation2014), which explores the same subject. De Dominicis builds upon the specifications of the model introduced by Ertur et al. (Citation2006), while the empirical study proposed here extends the framework of Fischer’s model (Fischer, Citation2011).
The model aims to estimate the correlation between regional inequality and economic growth by incorporating additional human capital variables explicitly. Moreover, the measure of regional inequality utilizes the Entropy Theil Index as the calculation model. The estimation model for examining the relationship between regional inequality, human capital, and economic growth adopts a panel data econometric approach, requiring a well-defined and accurate model specification.
In this research, the panel data regression model employs three models: the common effect model (CEM), fixed effect model (FEM), and random effect model (REM). The regression model equation takes the general form as depicted in Table .
Table 1. Panel regression econometric model
4. Result and discussions
The findings of panel regression model I showed that the three panel regression models yield similar outcomes, but the fixed effect model (FEM) proves to be the most suitable one due to its highest goodness of fit value (R-squared value and F-statistics) (Table ). The FEM model successfully estimates the impact of the independent variable on the dependent variable, indicating that the ETI variable has a positive and significant effect on the LOG (GRDP) variable. This suggests that higher regional inequality (measured by the Entropy Theil index) among provinces in Indonesia leads to increased economic growth. This outcome implies that the inequality between provinces is not a contributing factor to the deterioration of economic growth within Indonesia. Rather, regional inequalities between provinces have a positive influence on economic growth, aligning with the Solow (Citation1956) growth theory, although other theories may predict divergence.
Table 2. Model I. Panel regression results
Table presents the results of panel regression model II, indicating that the three panel regression models yield almost identical outcomes. All three models successfully estimate the relationship between the HDI variable and the LOG (GRDP) variable. However, the fixed effect model (FEM) stands out as the most suitable model due to its highest goodness of fit value (highest R-squared value and F-statistic). According to this FEM model, the HDI variable has a positive and significant effect on the LOG (GRDP) variable. This implies that higher HDI values positively impact GDP, aligning with Solow’s economic growth theory, which posits that regions with higher levels of capital supply will receive lower returns.
Table 3. Model II. Panel regression results
Table presents the results of panel regression model III, and the fixed effect model (FEM) is identified as the best panel regression model due to its highest goodness of fit value (highest R-squared value and F-statistic). The FEM model successfully estimates the relationship between the UNR variable and the LOG (GRDP) variable. It shows that the UNR variable has a positive and significant effect on the LOG (GRDP) variable, indicating that a higher unemployment rate leads to lower economic growth among provinces in Indonesia. This suggests that the current unemployment rate in Indonesia has not negatively affected economic growth. Unlike other economic theories that propose convergences, such as Solow’s theory, and divergences, such as endogenous growth theory and the New Economic Geography (NEC) theory, the findings in this study demonstrate a positive and statistically significant relationship between the unemployment rate and inter-provincial economic growth in Indonesia.
Table 4. Model III. Panel regression results
Table displays the results of panel regression model IV, and the three panel regression models yield almost identical outcomes in estimating the effect of the independent variable on the dependent variable. However, the fixed effect model (FEM) stands out as the best model due to its highest goodness of fit value (highest R-squared value and F-statistics). The FEM model provides better estimates of the influence of the independent variables on the dependent variable. The results indicate that the ETI variable has a positive and significant effect on the LOG (GRDP) variable. This suggests that higher levels of regional inequality between provinces in Indonesia are associated with higher levels of economic growth between provinces. Therefore, regional inequality does not worsen economic growth in Indonesia. Similarly, the HDI variable has a positive and significant effect on the LOG (GRDP) variable. This means that higher human capital among provinces in Indonesia is linked to higher growth rates between provinces. Thus, higher levels of human capital, encompassing productivity and competitiveness, contribute to increased growth rates. Lastly, the UNR variable has a positive and significant effect on the LOG (GRDP) variable. This implies that higher unemployment rates between provinces in Indonesia are associated with higher growth rates between provinces. However, this condition indicates that unemployment conditions among provinces in Indonesia have not had a negative impact or acted as an obstacle in encouraging higher growth among provinces.
Table 5. Model IV. Panel regression results
The panel regression model estimates indicate that all three independent variables have a positive and statistically significant impact on the dependent variable, which is economic growth. These results align with existing economic growth theories, but it’s worth noting that empirical findings can vary based on the data and methodology used in the analysis.
Based on the results of Table , all three panel regression models show similar outcomes, effectively estimating the relationship between regional inequality (ETI variable) and the open unemployment rate (UNR variable). However, the fixed effect model (FEM) stands out as the most suitable model due to its higher goodness of fit value (highest R-squared value and F-statistic). The analysis indicates that regional inequality (ETI variable) has a positive and significant impact on the open unemployment rate (UNR variable). In other words, higher levels of regional inequality between provinces in Indonesia are associated with a higher unemployment rate. This positive correlation between regional inequalities and the open unemployment rate supports both Solow’s reference theory and endogenous growth theories. Moreover, industrial concentrations in core areas offer economic agglomeration advantages.
Table 6. Model V. Panel regression results
Based on the findings in Table , two out of the three panel regression models, namely the fixed effect model (FEM) and the random effect model (REM), produce nearly identical results regarding the relationship between human capital (HDI variable) and the open unemployment rate (UNR variable). However, the fixed effect model (FEM) is deemed the most appropriate model due to its superior goodness of fit value (highest R-squared value and F-statistic). The analysis reveals that the human capital gap among provinces in Indonesia (HDI variable) has a negative and significant impact on the open unemployment rate (UNR variable). In simpler terms, when there is a greater disparity in human capital levels between provinces, the unemployment rate tends to be lower in those provinces.
Table 7. Model VI. Panel regression results
The panel regression results indicate a significant negative relationship between the disparity in human capital and the open unemployment rate in different provinces of Indonesia. According to Solow’s Growth Theory, capital and technology from external sources tend to converge and reach a state of equality. On the other hand, endogenous theories and the NEC suggest divergence may occur. Further research is required to better understand the impact of inequality on economic growth and its implications for regional economic development.
Table presents the results of panel regression model VII, where the common effect model (CEM) is identified as the best model, as it successfully estimates the relationship between the LOG (GRDP) variable and the UNR variable. The variable LOG (GRDP) has a positive and significant effect on the UNR variable. This implies that higher economic growth between provinces in Indonesia leads to higher unemployment rates, indicating that the growth has not generated sufficient job opportunities to accommodate the labor force entering the labor market in different provinces. The panel regression model’s findings suggest a positive association between economic growth and unemployment. According to Solow’s theory of economic growth, richer and poorer regions tend to converge over time, leading to lower unemployment rates. On the other hand, endogenous growth theories propose that differences in technology levels between regions or countries can result in disparities in economic growth. However, the relationship between inequality and economic growth remains complex and requires further investigation to better understand the true nature of this correlation.
Table 8. Model VII. Panel regression results
Based on the results of panel regression model VIII presented in Table , the three panel regression models yield nearly identical outcomes, successfully estimating the impact of the independent variable on the dependent variable. However, the panel regression model in the form of a fixed effect model (FEM) stands out as the most optimal model due to its high goodness of fit value (highest R-squared value, F-statistics). The FEM model demonstrates a more precise estimation of the relationship between the independent and dependent variables.
Table 9. Model VIII. Panel regression results
The ETI variable shows a positive and significant impact on the UNR variable, indicating that higher regional inequality between provinces in Indonesia leads to increased unemployment rates in the labor market. This suggests that inequality among provinces has the potential to raise unemployment levels. On the other hand, the HDI variable exhibits a negative and significant effect on the UNR variable, suggesting that higher human capital among the provinces in Indonesia results in lower unemployment rates. This indicates that a higher quality of human capital, characterized by increased productivity and competitiveness, leads to greater absorption of the labor force in the job market, as it creates new job opportunities.
The LOG (GRDP) variable demonstrates a positive and significant influence on the UNR variable, indicating that higher economic growth rates among the provinces in Indonesia correspond to higher unemployment rates. This suggests that economic growth has not been successful in fostering and expanding job opportunities in the provinces. Several economic theories attempt to explain the connection between inequality and economic growth, such as the Solow growth model, endogenous growth theory, and new economic geography theory. However, empirical studies on the relationship between inequality and economic growth have produced conflicting findings. The findings indicate that the panel regression model, specifically the FEM model, examined the relationship between regional inequality and unemployment in Indonesia. The FEM model revealed that the ETI variable had a positive and statistically significant impact on the unemployment variable, while the human capital variable had a negative and significant effect on the UNR variable. Additionally, the economic growth variable had a positive and statistically significant influence on the UNR variable.
5. Conclusion
Based on the estimation results using the panel regression model, several conclusions can be drawn. Firstly, the ETI variable has a positive and significant effect on the LOG (GRDP) variable, indicating that higher regional inequality between provinces in Indonesia leads to higher economic growth between provinces. Secondly, the HDI variable has a positive and significant effect on the LOG (GRDP) variable, meaning that higher human capital among provinces in Indonesia is associated with higher growth rates between provinces. Thirdly, the UNR variable has a positive and significant effect on the LOG (GRDP) variable, showing that higher unemployment rates between provinces in Indonesia are linked to higher growth rates between provinces. Lastly, the ETI variable has a positive and significant effect on the UNR variable, indicating that higher regional inequality between provinces in Indonesia is associated with higher unemployment rates between provinces.
The findings reveal that the HDI variable has a negative and statistically significant impact on the UNR variable. This implies that higher human capital among the provinces in Indonesia leads to lower unemployment rates among them. On the other hand, the variable LOG (GRDP) has a positive and significant effect on the UNR variable, indicating that higher economic growth rates among the provinces in Indonesia are associated with higher unemployment rates among them.
Based on the obtained results, several policy suggestions can be formulated. Firstly, efforts should be made to enhance human capital and reduce unemployment by facilitating access to resources, especially in the domains of education and skill development. Secondly, promoting economic growth and addressing regional inequalities between provinces can foster overall economic progress. Implementing skill training programs and creating new job opportunities would be essential to mitigate unemployment rates.
However, certain limitations should be acknowledged concerning this research. The utilization of data within a specific timeframe may restrict the generalization of findings to other periods. Additionally, other influential factors like infrastructure and government policies were not incorporated in the analysis, which could impact economic growth and unemployment rates. Furthermore, this model cannot determine causation, making it challenging to ascertain whether the examined variables are causing negative changes in each other. For future studies, it is recommended to explore additional factors that might influence economic growth and unemployment rates. Expanding the analysis timeframe could provide insights into the evolving patterns of economic growth and unemployment rates over time. Lastly, conducting more detailed sector-specific analyses might reveal which sectors have the most significant influence on economic growth and unemployment rates.
Disclosure statement
No potential conflict of interest was reported by the author(s).
Additional information
Notes on contributors
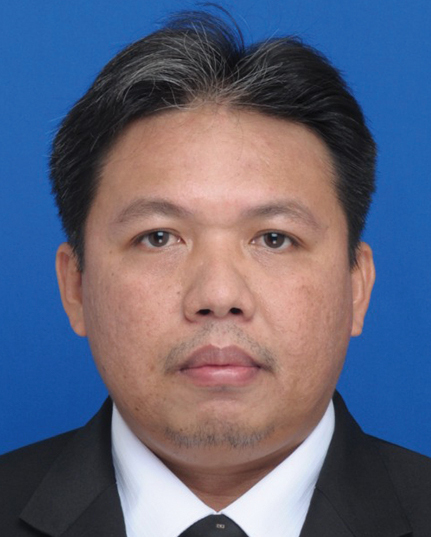
Suparman Suparman
Suparman Suparman is a senior lecturer at the Department of Economics, Universitas Tadulako, Palu, Indonesia. His research focuses on promoting industrialization and regional income inequality with a focus on agriculture transformation, studying tourism governance and supply chain management in the perspective of economic competitiveness, analyzing the social economic index of disaster recovery, and addressing sustainable development issues.
Muzakir Muzakir
Muzakir Muzakir is a senior lecturer at the Department of Economics, Universitas Tadulako, Palu, Indonesia. His research focuses on promoting tourism governance and supply chain management in the competitiveness of the tourism sector, analyzing the impact of creative management on enterprising performance, exploring the effects of strategic leadership and organizational innovation, strategic management and the role of IT capability, and conducting an analysis of forming dimensions of e-service quality for online travel services.
References
- Adrián Risso, W., & Sánchez Carrera, E. J. (2012). Inequality and economic growth in China. Journal of Chinese Economic and Foreign Trade Studies, 5(2), 80–14. https://doi.org/10.1108/17544401211233453
- Alesina, A., & Rodrik, D. (1994). Distributive politics and economic growth. The Quarterly Journal of Economics, 109(2), 465–490. https://doi.org/10.2307/2118470
- Arjona, R., Ladaique, M., & Pearson, M. (2003). Growth, inequality and social protection. Canadian Public Policy/Analyse de Politiques, 29, S119–S139. https://doi.org/10.2307/3552279
- Atif, M. (2021). The effects of energy consumption, economic growth, and financial development on CO2 emissions in Greece. Arthatama, 5(1), 13–26. https://arthatamajournal.co.id/index.php/home/article/view/40
- Avram, M., & Postoiu, C. (2016). Territorial patterns of development in the European Union. Theoretical and Applied Economics, 23(1), 77–88. http://store.ectap.ro/articole/1161.pdf
- Badan Pusat Statistik. (2021). Berita Resmi BPS Tahun 2021.
- Benedek, J. (2015). Spatial differentiation and core-periphery structures in Romania. Eastern Journal of European Studies, 6(1), 49–61. https://ejes.uaic.ro/articles/EJES2015_0601_BEN.pdf
- Benedek, J., & Veress, N. C. (2013). Economic disparities and changes in the convergence of the Romanian NUTS 2 and NUTS 3 regions. Romanian Review of Regional Studies, 9(1), 85–90. https://rrrs.reviste.ubbcluj.ro/arhive/Artpdf/v9n12013/RRRS901201309.pdf
- Blanchard, O. J., Nordhaus, W. D., & Phelps, E. S. (1997). The medium run. Brookings Papers on Economic Activity, 1997(2), 89–158. https://doi.org/10.2307/2534687
- Boldea, M., Parean, M., & Otil, M. (2012). Regional disparity analysis: The case of Romania. Journal of Eastern Europe Research in Business & Economics, 2012, 1. https://doi.org/10.5171/2012.599140
- Campos, N. F. (2001). Will the future be better tomorrow? The growth prospects of transition economies revisited. Journal of Comparative Economics, 29(4), 663–676. https://doi.org/10.1006/jcec.2001.1741
- Castelló, A., & Doménech, R. (2002). Human capital inequality and economic growth: Some new evidence. The Economic Journal, 112(478), C187–C200. https://doi.org/10.1111/1468-0297.00024
- Chen, B. L. (2003). An inverted-U relationship between inequality and long-run growth. Economics Letters, 78(2), 205–212. https://doi.org/10.1016/S0165-1765(02)00221-5
- Chirila, V., & Chirila, C. (2014). The impact of economic and financial crisis on the regional disparities in Romania and European Union. Procedia-Social & Behavioral Sciences, 109, 502–506. https://doi.org/10.1016/j.sbspro.2013.12.497
- De Dominicis, L. (2014). Inequality and growth in European regions: Towards a place-based approach. Spatial Economic Analysis, 9(2), 120–141. https://doi.org/10.1080/17421772.2014.891157
- Doran, J., & Jordan, D. (2013). Decomposing European NUTS2 regional inequality from 1980 to 2009: National and European policy implications. Journal of Economic Studies, 40(1), 22–38. https://doi.org/10.1108/01443581311283484
- Enflo, K., & Rosés, J. R. (2015). Coping with regional inequality in S weden: Structural change, migrations, and policy, 1860–2000. The Economic History Review, 68(1), 191–217. https://doi.org/10.1111/1468-0289.12049
- Ertur, C., Le Gallo, J., & Baumont, C. (2006). The European regional convergence process, 1980-1995: Do spatial regimes and spatial dependence matter? International Regional Science Review, 29(1), 3–34. https://doi.org/10.1177/0160017605279453
- Fan, S., Kanbur, R., & Zhang, X. (Eds.). (2009). Regional Inequality in China: Trends, Explanations and Policy Responses (1st ed.). Routledge. https://doi.org/10.4324/9780203881484
- Fan, C. C., & Sun, M. (2008). Regional inequality in China, 1978-2006. Eurasian Geography and Economics, 49(1), 1–18. https://doi.org/10.2747/1539-7216.49.1.1
- Fischer, M. M. (2011). A spatial mankiw–romer–weil model: Theory and evidence. The Annals of Regional Science, 47(2), 419–436. https://doi.org/10.1007/s00168-010-0384-6
- Forbes, K. J. (2000). A reassessment of the relationship between inequality and growth. American Economic Review, 90(4), 869–887. https://doi.org/10.1257/aer.90.4.869
- Fujita, M., Krugman, P. R., & Venables, A. (2001). The spatial economy: Cities, regions, and international trade. MIT press.
- Galor, O. (2005). From stagnation to growth: unified growth theory. In P. Aghion & S. N. Durlauf (Eds.), Handbook of economic growth (Vol. 1, pp. 171–293). North Holland. https://doi.org/10.1016/S1574-0684(05)01004-X
- Galor, O. (2012). The demographic transition: Causes and consequences. Cliometrica, 6(1), 1–28. https://doi.org/10.1007/s11698-011-0062-7
- Gardiner, B., Martin, R., & Tyler, P. (2012). Competitiveness, productivity and economic growth across the European regions. In R. Martin, M. Kitson, & P. Tyler (Eds.), Regional competitiveness (1st ed., pp. 61–84). Routledge.
- Grossman, G. M., & Helpman, E. (1994). Endogenous innovation in the theory of growth. Journal of Economic Perspectives, 8(1), 23–44. https://doi.org/10.1257/jep.8.1.23
- Heidenreich, M., & Wunder, C. (2008). Patterns of regional inequality in the enlarged Europe. European Sociological Review, 24(1), 19–36. https://doi.org/10.1093/esr/jcm031
- Henderson, J. V., Squires, T., Storeygard, A., & Weil, D. (2018). The global distribution of economic activity: Nature, history, and the role of trade. The Quarterly Journal of Economics, 133(1), 357–406. https://doi.org/10.1093/qje/qjx030
- Hippe, R., & Baten, J. (2012). Regional inequality in human capital formation in Europe, 1790–1880. Scandinavian Economic History Review, 60(3), 254–289. https://doi.org/10.1080/03585522.2012.727763
- Husnah, H., & Adam, R. P. (2022). Challenges and strategic issues in regional development: Analysis of regional performance Enhancement strategies. Economic and Business Horizon, 1(2), 13–22. Retrieved from. https://journal.lifescifi.com/index.php/ebh/article/view/113
- Hussain, T., Siddiqi, M., & Iqbal, A. (2010). A Coherent Relationship between Economic Growth and Unemployment: An Empirical Evidence from Pakistan. International Journal of Economics and Management Engineering, 4(3), 288–295. https://doi.org/10.5281/zenodo.1335018
- Istrate, M., & Horea-Serban, R. I. (2016). Economic growth and regional inequality in Romania. Analele Universitatii din Oradea, Seria Geografie, 26(2), 201–209. https://geografie-uoradea.ro/Reviste/Anale/Art/2016-2/10.AUOG_717_Istrate.pdf
- Jencova, S., Litavcova, E., Kotulic, R., Vavrek, R., Vozarova, I. K., & Litavec, T. (2015). Phenomenon of poverty and economic inequality in the Slovak republic. Procedia Economics and Finance, 26, 737–741. https://doi.org/10.1016/S2212-5671(15)00832-1
- Krugman, P. (1991). Increasing returns and economic geography. Journal of Political Economy, 99(3), 483–499. https://doi.org/10.1086/261763
- Krugman, P. (1998). What’s new about the new economic geography? Oxford Review of Economic Policy, 14(2), 7–17. https://doi.org/10.1093/oxrep/14.2.7
- Kurz, H. D., & Salvadori, N. (1997). Theory of production: A long-period analysis. Cambridge University Press.
- Kuttor, D. (2009). Territorial inequalities in Central Europe–spatial analysis of the Visegrad countries. Romanian Review of Regional Studies, 5(1), 25–36. https://rrrs.reviste.ubbcluj.ro/arhive/Artpdf/v5n12009/RRRS051200904.pdf
- Lessmann, C. (2014). Spatial inequality and development—Is there an inverted-U relationship? Journal of Development Economics, 106, 35–51. https://doi.org/10.1016/j.jdeveco.2013.08.011
- Lessmann, C., & Seidel, A. (2017). Regional inequality, convergence, and its determinants–A view from outer space. European Economic Review, 92, 110–132. https://doi.org/10.1016/j.euroecorev.2016.11.009
- Lin, J. Y. (2004). Development strategies for inclusive growth in developing Asia. Asian Development Review, 21(2), 1–27. https://doi.org/10.1142/S0116110504000053
- Lindbeck, A. (1991). Unemployment and labour market imperfections. In Issues in contemporary economics (pp. 75–101). Palgrave Macmillan. https://doi.org/10.1007/978-1-349-11576-1_4
- Lindbeck, A., & Snower, D. J. (1989). The insider-outsider theory of employment and unemployment. MIT Press Books.
- Li, H., & Zou, H. F. (1998). Income inequality is not harmful for growth: Theory and evidence. Review of Development Economics, 2(3), 318–334. https://doi.org/10.1111/1467-9361.00045
- Martin, R. (1997). Regional unemployment disparities and their dynamics. Regional Studies, 31(3), 237–252. https://doi.org/10.1080/00343409750134665
- Pambayun, D. A. (2021). Indicators of the association of unemployment in Indonesia with the level of employment opportunity, GDP, and SER. Research Horizon, 1(5), 189–206. https://doi.org/10.54518/rh.1.5.2021.189-206
- Panizza, U. (2002). Income inequality and economic growth: Evidence from American data. Journal of Economic Growth, 7(1), 25–41. https://doi.org/10.1023/A:1013414509803
- Panzera, D., & Postiglione, P. (2020). Measuring the spatial dimension of regional inequality: An approach based on the Gini correlation measure. Social Indicators Research, 148(2), 379–394. https://doi.org/10.1007/s11205-019-02208-7
- Panzera, D., & Postiglione, P. (2022). The impact of regional inequality on economic growth: A spatial econometric approach. Regional Studies, 56(5), 687–702. https://doi.org/10.1080/00343404.2021.1910228
- Partridge, M. D. (2005). Does income distribution affect US state economic growth? Journal of Regional Science, 45(2), 363–394. https://doi.org/10.1111/j.0022-4146.2005.00375.x
- Perotti, R. (1994). Income distribution and investment. European Economic Review, 38(3–4), 827–835. https://doi.org/10.1016/0014-2921(94)90119-8
- Perotti, R. (1996). Growth, income distribution and democracy: What the data say. Journal of Economic Growth, 1(2), 149–187. https://doi.org/10.1007/BF00138861
- Perrotti, R. (1994). Income distribution and growth: An empirical investigation. Columbia University.
- Petrakos, G., Rodríguez-Pose, A., & Rovolis, A. (2005). Growth, integration, and regional disparities in the European Union. Environment and Planning A, 37(10), 1837–1855. https://doi.org/10.1068/a37348
- Piketty, T. (2014). Capital in the twenty-first century. In Capital in the twenty-first century. Harvard University Press.
- Postel-Vinay, F. (1998). Transitional dynamics of the search model with endogenous growth. Journal of Economic Dynamics and Control, 22(7), 1091–1115. https://doi.org/10.1016/S0165-1889(97)00095-X
- Puga, D. (2002). European regional policy in Light of recent location theories. Journal of Economic Geography, 2(4), 373–406. https://doi.org/10.1093/jeg/2.4.373
- Rey, S. J., & Janikas, M. V. (2005). Regional convergence, inequality, and space. Journal of Economic Geography, 5(2), 155–176. https://doi.org/10.1093/jnlecg/lbh044
- Rey, S. J., & Smith, R. J. (2013). A spatial decomposition of the Gini coefficient. Letters in Spatial and Resource Sciences, 6(2), 55–70. https://doi.org/10.1007/s12076-012-0086-z
- Romer, P. M. (1990). Endogenous technological change. Journal of Political Economy, 98(5, Part 2), S71–S102. https://doi.org/10.1086/261725
- Sachs, J. D. (2006). The end of poverty: Economic possibilities for our time. Penguin.
- Sala-I-Martin, X. X. (1996). Regional cohesion: Evidence and theories of regional growth and convergence. European Economic Review, 40(6), 1325–1352. https://doi.org/10.1016/0014-2921(95)00029-1
- Solow, R. M. (1956). A contribution to the theory of economic growth. The Quarterly Journal of Economics, 70(1), 65–94. https://doi.org/10.2307/1884513
- Staehr, K. (2003, January 23). Reforms and economic growth in transition economies: Complementarity, sequencing and speed. Sequencing and Speed. https://doi.org/10.2139/ssrn.1015454
- Torsten, P., & Tabellini, G. (1994). Is inequality harmful for growth. American Economic Review, 84(3), 600–621. https://www.jstor.org/stable/2118070
- Wei, Y. D. (1999). Regional inequality in China. Progress in Human Geography, 23(1), 49–59. https://doi.org/10.1191/030913299676254572
- Wei, Y. D. (2002). Multiscale and multimechanisms of regional inequality in China: Implications for regional policy. Journal of Contemporary China, 11(30), 109–124. https://doi.org/10.1080/10670560120091165
- Zaman, G., Goschin, Z., & Vasile, V. (2013). Evoluţia dezechilibrelor teritoriale din România în contextul crizei economice. Revista Română de Economie, 2(46), 20–39. http://www.revecon.ro/articles/2013-2/2013-2-2.pdf