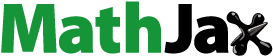
Abstract
The study extends the literature on the nexus between cryptocurrency and uncertainty. This study proxied the cryptocurrencies and global uncertainty, respectively, with the seven most significant and variationally susceptible cryptos and the comprehensive world uncertainty in measuring the crypto-uncertainty nexus over the period (2015–2022) and further employing the quantile regression approach. The OLS model results point to a blend of both significant and insignificant relationship between global uncertainty and cryptocurrencies. These relationships were further examined in quantiles and further accounted for the impact of investor sentiments (VIX) and volatility (OVX), and the results were largely corroborated with the results from the conventional OLS, except for the Bitcoin, Litecoin, and Ripple markets. It was also discovered that the nexus changes across quantiles. The results revealed a blend of strong and weak hedges and safe havens among the selected cryptos against global uncertainty during normal and extreme market conditions. In the face of global turmoil, it was revealed that the average crypto market could serve as a safe haven. Also, the cryptos with an insignificant nexus with global uncertainty were found to be significantly affected by investor sentiment. These findings were further confirmed by the quantile-on-quantile and causality-in-quantile estimations. Given the intense precariousness and lack of hedge and haven capacities within the majority of the cryptocurrencies, it is pertinent for investors to consider the market in general as a means of diversifying their portfolios and reserve the hedge and haven option to the few markets that possess such luxury.
1. Introduction
The world we live in today is full of unknowns. Given the very uncertain nature of humanity, it is hard to imagine a world without uncertainties. Recent occurrences such as the pandemic, political polarisation, international conflicts, and the financial meltdown have all sparked concerns about growing economic uncertainty (Ahir et al., Citation2022; Al-Shboul et al., Citation2022; Bashir & Kumar, Citation2023). Uncertainty has emerged as the most universal description of the world in the last decades with its impact felt across all markets (Adediran et al., Citation2021; Ahir et al., Citation2022; Al-Shboul et al., Citation2023; Papadamou et al., Citation2021). Accordingly, uncertainties in the global economy have a bearing on markets in a broad sense and explicitly on the financial markets, while most major global currencies are also driven by uncertainties (Adediran et al., Citation2021; Aharon et al., Citation2022; Ahir et al., Citation2022; Bashir & Kumar, Citation2023; Shaikh, Citation2020). In this regard, exchange instruments have changed and evolved in response to the market’s need to make the exchange as simple as possible (Das & Kannadhasan, Citation2018; Das et al., Citation2022).
The global financial markets have seen significant changes over time, especially from barter to commodities, coins, precious metals and alloys, contemporary economic structures, and the latest cryptocurrency advancements (Das & Kannadhasan, Citation2018). Cryptocurrencies (cryptos henceforth) have emerged among the greatest economic discoveries and inventions of the modern century, allowing counterparties to process and record transactions without the use of financial intermediaries (Chowdhury & Damianov, Citation2022; Papadamou et al., Citation2021). Unique characteristics such as constrained obscurity, objectivity from a central authority, and security against double-spending attacks distinguish the innovative currency (Chowdhury & Damianov, Citation2022; Das & Kannadhasan, Citation2018; Owusu Junior et al., Citation2020). Also, unlike conventional currencies, cryptos can be used to make payments anywhere in the globe and are not regulated by central bank regulations or inflation (Shaikh, Citation2020). The crypto market expanded as a result of these advancements and in anticipation of numerous applications of cryptography to payment systems and financial contracts (Chowdhury & Damianov, Citation2022). Furthermore, the financial services industry has seen seismic changes as a result of developments in distributed ledger technology and cryptography (Chowdhury & Damianov, Citation2022; Papadamou et al., Citation2021; Yousaf & Ali, Citation2020; Yousaf et al., Citation2022).
The popularity and advancement in cryptos incited the rollout of Bitcoin as a digital coin on a peer-to-peer mechanism for the first time in 2009 (Aharon et al., Citation2022; Chen et al., Citation2021; Paule-Vianez et al., Citation2020; Yousaf et al., Citation2022). The most valuable crypto per market capitalisation remains Bitcoin, which accounts for more than half of all worldwide crypto’s market value (Chowdhury & Damianov, Citation2022; Hazgui et al., Citation2021; Yousaf & Ali, Citation2020). Once touted as a substitute for conventional currency, bitcoin quickly established itself as a more profitable investment asset (Bouri et al., Citation2017; Papadamou et al., Citation2021). For instance, Demir et al. (Citation2018) and Bouri et al. (Citation2017) demonstrated how bitcoin is unconditioned by real assets and the global pecuniary system and remedies the volatility of the financial system’s economic framework. Also, Bitcoin’s augmented usage and value, especially during the financial crisis period, were attributed to its perceived safe haven capacity from turbulence around economic institutions. Demir et al. (Citation2018) further posited that Bitcoin experienced its highest monthly capitalisation turnover from November to December 2017, with a beginning and ending value of $111–278 billion. This sector can only be deemed to have experienced augmented expansion over a short period, with a total market value of over $300 billion as of 2018 (Owusu Junior et al., Citation2020). Yet Bitcoin is not the sole crypto; according to market capitalisation as of February 2022, the top five cryptos were Bitcoin, Ethereum, Ripple, Bitcoin Cash, and its SV alternative (Chez, Citation2022).
The value of cryptos is of essence to stakeholders, including investors, especially their haven and hedge abilities both in turbulence and in normal times. Instinctively, the investment and diversification options at the disposal of comprehensive market speculators have augmented given the inception of these asset classes (Chowdhury & Damianov, Citation2022; Owusu Junior et al., Citation2020; Papadamou et al., Citation2021), as have the factors that affect their market values and efficiency. For instance, considering the recent plunge that gripped the market in the last quarter of 2022 with a record-high loss of over $900 billion and an approximated daily loss of 13% in most of the significant cryptos, which has raised investor sentiment due to the perceived market perils (Aharon et al., Citation2022; Almeida et al., Citation2022), while also increasing the perceived volatility due to the extreme level of losses (Al-Shboul et al., Citation2023; Bashir & Kumar, Citation2023; Yousaf & Ali, Citation2020), one can argue on the market’s diversification capacity. Subject to the above contestation in the market, Spithoven (Citation2019) argued the need for regulation and oversight by trustworthy third parties despite the numerous advantages. This indicates that the crypto market is not without limitations and is affected by numerous factors. The aforementioned submissions demand that the relationship between cryptos and the global forces influencing them be investigated, as this remains pertinent, especially for investors who would rely on such information to make informed decisions.
Investors, according to the adaptive market hypothesis (AMH), are most sensitive and overreact during times of high market volatility and engage in satisficing rather than maximising conduct (Lo, Citation2004). Accordingly, when immense shifts or pecuniary tremors occur, the market’s phylogenetic changes, and initially, handy algorithms might become dismissive (Mushinada, Citation2020; Neely et al., Citation2009). Hence, the AMH is an efficient predictor of markets amid uncertainty (Aharon et al., Citation2022; Mushinada, Citation2020). This implies that the conventional assertions of market efficiency may prove inadequate in elucidating market dynamics amidst such a precarious regime, thereby underscoring the imperative for investors to exhibit adaptability (Lo, Citation2004). On the other hand, the modern portfolio theory (MPT) emphasises diversification, and given the prior justifications of cryptos as diversifying assets, one could argue the theory’s relevance in explaining the crypto-uncertainty nexus. For instance, in terms of uncertainty, cryptos, particularly Bitcoin, have been regarded as efficient (Kurihara & Fukushima, Citation2017; Noda, Citation2021; Tiwari et al., Citation2018) and as hedging and diversifying assets (Demir et al., Citation2018; Wang et al., Citation2019; Yen & Cheng, Citation2021). However, prior literature has established the efficiency (Kurihara & Fukushima, Citation2017; Tiwari et al., Citation2018; Vidal-Tomás & Ibañez, Citation2018) and inefficiency (Bariviera, Citation2017; Urquhart, Citation2016; Vidal-Tomás et al., Citation2019) of the crypto market with evidence of long memory and multifractality (Assaf et al., Citation2023). In addition, Vidal-Tomás et al. (Citation2019) appraised the market as weak-form inefficient with an extended level of inefficiency. These findings, when paired with the findings of other studies, substantiated the explanation for the market crash of 2018 and could further account for the chaotic market plunge in 2022. These assertions urge an examination of these effects in light of the aforementioned theories and, with it, a mark on their relevance in examining the crypto market’s performance amid uncertainty. This study seeks to reveal the safe haven and hedge-urge aspects of cryptos by examining the crypto-uncertainty nexus subject to the aforesaid suppositions.
Recent research has shown that economic uncertainty in general and policy uncertainty, in particular, have a significant impact on the financial markets. Studies have also considered the uncertainty nexus with cryptos, considering the market value and assets’ significance to market participants. Despite the significance of comprehending the overall crypto market, prior studies have concentrated on how uncertainty affects Bitcoin returns and volatility (Chen et al., Citation2021; Demir et al., Citation2018; Foglia & Dai, Citation2021; Yousaf & Yarovaya, Citation2022). These studies further proxied uncertainty with the economic policy uncertainty (EPU) index. On the other hand, studies such as Aharon et al. (Citation2022), Al-Shboul et al (Citation2022, Citation2023). Bashir and Kumar (Citation2023), and Lucey et al. (Citation2022) have all examined the crypto-uncertainty nexus, employing EPU, pandemic-induced, crypto-specific, and Twitter-based uncertainties as uncertainty metrics, with somewhat contradictory findings. None of the previous studies on the crypto-uncertainty nexus has considered the Ahir et al.-led world uncertainty index (WUI, henceforth), with the majority focusing largely on Baker et al. (Citation2016) limited coverage EPU as a measure of global uncertainty. This is due to its emphasis on advanced economies, which leaves developing economies largely unrepresented (Ahir et al., Citation2022). The aforementioned limitation is likely to affect the reliability of prior findings. Global market uncertainties are not limited to advanced economies, and the world has shrunk to the size of a village, necessitating the crafting of a WUI. The WUI stands as the first index that considers both advanced and developing economies and covers 143 countries across the globe. In addition to being superior in terms of coverage, the WUI considers relevant uncertainties and covers a wide range of these uncertainties compared to other indexes. For instance, the metrics take account of relevant macroeconomic events such as Brexit and the recent pandemic, as well as diverse political conflicts and polarisation. Given its expansive coverage in terms of major events and economies, the WUI is the most reliable index for measuring global uncertainties (Ahir et al., Citation2022). Furthermore, the current study also accommodates the relevance of investor sentiment and the overall impact of market volatility on global uncertainty (Al-Yahyaee et al., Citation2019; Das et al., Citation2022). Thus, to account for these factors, the study controls for the impact of the aforesaid measure of uncertainty to unearth the actual influence of WUI as established by extant literature.
Furthermore, the majority of the prior studies that adopted regressions as a means of examining the crypto-uncertainty nexus largely focused on the use of simple linear regression (Aharon et al., Citation2022; Colon et al., Citation2021; Demir et al., Citation2018; Kyriazis, Citation2021; Yen & Cheng, Citation2021). Due to spurious correlation, regressions as a technique for measuring correlation are unproductive (Das, Citation2022; Yen & Cheng, Citation2021) and susceptible to outliers (Aharon et al., Citation2022; Bashir & Kumar, Citation2023; Das et al., Citation2022; Demir et al., Citation2018). But subject to quantile regression’s (QR) ability to articulate the qualified quantile of a predicting variable as a rectilinear function of the explanatory variables rather than just the restrictive average of the explained variable, their approximations are more vigorous against extreme values in response measurement (Aharon et al., Citation2022; Bashir & Kumar, Citation2023; Sim & Zhou, Citation2015). Furthermore, QR depicts the predictive parameter’s influence on the response variable in greater detail.
This study partly follows Aharon et al. (Citation2022), Bashir and Kumar (Citation2023), and Colon et al. (Citation2021) to analyse the nexus between uncertainties and crypto returns by QR, but deviates from these studies by complementing the QR with embedded robust quantile-on-quantile regression (QQR, henceforth) instead of linear regressions and volatility-based models such as the generalised autoregressive conditional heteroscedasticity (GARCH, henceforth) and further adopting the WUI, which is more comprehensive than the usual EPU index adopted by most studies. Furthermore, the present investigation deviates from the aforementioned research endeavours by incorporating meticulous control measures to account for the influence of investor sentiment and overarching market volatility, which have been found to generally affect uncertainty measures in the financial markets (Al-Yahyaee et al., Citation2019; Das et al., Citation2022; Umar et al., Citation2023). Specifically, the study takes into consideration the volatility uncertainty index (VIX), which quantifies investor sentiment, and the crude volatility index (OVX), which measures general market volatility. Instead of the QQR, Yen and Cheng (Citation2021) rather employed the stochastic volatile model of Wang et al. (Citation2019) in examining the relationship between EPU and crypto volatility amid government regulation. Owusu Junior et al. (Citation2020) further employed both the QR and the QQR in examining the connectedness of cryptos and gold returns. The model’s urge lies in quantile-based projections and its ability to adapt to excesses in acceleration measurement, especially with relevant conditional quantile distributions.
The paper complements the prior literature in the area, with the immediate contribution being an extension of the nexus between the variables. Subject to reviewed literature, this study represents the first to employ both the QR and the QQR to analyse the above nexus while also utilising WUI in place of the limited EPU. A supplementary advantage of our empirical design is that the type of uncertainty we examine, global uncertainty, is largely exogenous to the activities of investors in the crypto markets and other relevant stakeholders, allowing us to draw inferences from the results (Ahir et al., Citation2022). Due to the paucity of literature on the nexus between crypto returns and uncertainty, combined with the lack of studies focusing on WUI, the conclusions of this study will benefit investors, and its significance cannot be overemphasised. The study’s findings offer information about how the world’s uncertainty affects cryptos, which is useful when choosing an investment strategy. For instance, if the precariousness and peril of the globe have an adverse stimulus on the chosen crypto market, investors relying on the study’s findings may decide to minimise their exposure to cryptos during times of high uncertainty. Alternatively, with either insignificant results or a significant positive influence from the increased level of global uncertainty, investors may choose to maintain or expand their exposure to cryptos. In addition, knowledge of an asset’s behaviour given normal, bearish, and bullish market conditions will contribute to choosing an asset as a hedge, haven, or diversifier. This is notwithstanding that the crypto market is intricate and influenced by numerous elements other than general uncertainty. This study aims to answer the following questions: “Are crypto returns correlated with GU?” “Do these links change over time and quantiles?” “Do cryptos serve as a hedge, diversifier, or safe haven during GU?” and “What is the impact of GU on crypto returns?”
Our analysis demonstrates a substantial connection between the GU and the selected cryptos. The findings from the OLS model point to a blend of both significant and positive (negative) impacts of GU on crypto returns, except for Bitcoin and Litecoin (Ripple). The results from the QR analysis with and without controlling for the respective influence of VIX and OVX were largely corroborated with those from the OLS, except for Bitcoin, Litecoin, and Ripple, which exhibited diverse directional nexuses, with the lower quantiles exhibiting significant but positive nexuses. It was also discovered that the crypto-uncertainty relationship changes across quantiles. The findings from the QR further revealed that, under routine (turbulent) circumstances, the selected cryptos could both function as a stout and flimsy hedge (safe haven) against GU except for Monero, which consistently exhibited the urge to function only as a diversifier against GU shocks across quantiles. The study confirms the studies by Aharon et al. (Citation2022), Almeida et al. (Citation2022), Al-Shboul et al. (Citation2023), Colon et al. (Citation2021), Paule-Vianez et al. (Citation2020), Wang et al. (Citation2019), and Wu et al. (Citation2019).
The remaining section is disseminated as follows: the next segment is a review of the literature, where prior studies are discussed and analysed. The third base segment captures the methodology that pronounces the measurement variables and sampled data, pre-estimation data analysis, and idealised model. The fourth segment provides results and discussions of the idealised models. The last part, christened conclusions, provides the ultimate annotations on the study.
2. Literature review
The augmented upsurge in crypto value has been complemented by a large body of empirical evidence concerning the potential hedging and safe-haven urges, likewise the market efficiency of these assets amid economic peril. Although a comprehensive review of the literature is beyond the scope of this research, we provide a summary of recent and pertinent studies on the relationship between cryptos and uncertainty. The review focused on the theories (AMH and MPT) underpinning the crypto and uncertainty nexus; the GU and their related index; the efficiency of the crypto market; and empirical studies that support the nexus between cryptos and uncertainties.
2.1. Adaptive market hypothesis and modern portfolio theory
According to the AMH, people are motivated by their self-interests, make blunders, adjust, and further study and acclimatise. For efficient forecasting, the AMH must be used to predict changes in crypto prices over time while taking into account time-varying market conditions (Khursheed et al., Citation2020). Investors, according to the AMH, engage in satisficing rather than maximising behaviour. This is consistent, especially when the evolutionary ecology of the market changes and major transformations or economic shocks take place and formerly beneficial heuristics become maladaptive. The most pertinent hypothesis for comprehending investor behaviour is the AMH since it demonstrates that the EMH may not be accurate in uncertain circumstances and that investors would choose to adapt to these markets.
Previous research into the interaction of both AMH and predictability has been limited in scope, making it difficult to determine specific AMH implications. Over a set period, the returns are predictable, and AMH is confirmed by the changing nature of predictable patterns throughout time (Mushinada, Citation2020). The varying market efficiency of cryptos in light of the AMH was examined by Khursheed et al. (Citation2020) and Noda (Citation2021), and it was respectively discovered that changing market times had a substantial impact on crypto price changes and that the magnitude of efficacy diverges across horizons and markets, with Bitcoin’s efficiency priming over Ethereum’Khuntia and Pattanayak (Citation2018) further examined the AMH’s performance and the efficiency of Bitcoin pricing and concluded that the proof of vibrant efficiency supports the AMH hypothesis. Furthermore, the AMH, gold, and cryptos were all subjected to a dynamic analysis of market efficiency by Ghazani and Jafari (Citation2021). The AMH was verified by the examination of observed results in all markets; however, the degree of adaptability between the data was variable. This suggests that AMH is the strongest candidate to explain the link between cryptos and economic uncertainty.
The MPT, on the other hand, heavily emphasises diversification, and investors, according to the theory, could achieve the best results by combining the two most viable assets based on their risk tolerance. The peril and yield characteristics of each investment ought to be measured in connection with the portfolio’s total risk and return characteristics rather than in isolation (Adediran et al., Citation2021). In light of the aforementioned suppositions, Qin et al. (Citation2021) examined Bitcoin’s function in risk diversification during periods of significant volatility. The study revealed that in the face of international trade tensions and a complex environment, entrepreneurs and authorities can leverage the Bitcoin market to optimise their investments. In other studies, a crypto portfolio was chosen based on its risk-bearing capacity, and Bitcoin, Ethereum, and XRP came out on top in terms of risk-adjusted returns. These studies, along with others, affirm the relevance of the MPT in explaining the ability of cryptos to construct a risk-adjusted investment portfolio given the established safe haven and hedge properties of cryptos amid uncertainties (Aharon et al., Citation2022; Mokni et al., Citation2022).
2.2. Global economic uncertainties
For the last few decades, the issue of uncertainties in general and policy uncertainty, in particular, has emerged (Ahir et al., Citation2022; Al-Shboul et al., Citation2022). Depending on the size and strength of the economy, a country’s uncertainty affects the markets of other countries (Ahir et al., Citation2022). The idea of uncertainty captures the turbulence and angst that individuals, corporate institutions, and authorities have about prospective future events, notwithstanding events like elections, wars, and climate change (Ahir et al., Citation2022). Uncertainty characterises unexpected changes in the economic environment as well as pecuniary policy variation, and this has been substantially touted by financial developments and academia as a major challenge (Aharon et al., Citation2022; Al-Shboul et al., Citation2022, Citation2023). Baker et al. (Citation2016) characterises uncertainties as the perils affiliated with a pecuniary setting bearing on unknown pending government policies and supervisory frameworks. Also, policy ambiguity in the future will only have a long-term influence. This suggests that a range of other factors, some short-term and others long-term, influence uncertainty, as established in earlier studies (Aharon et al., Citation2022; Al-Shboul et al., Citation2022; Almeida et al., Citation2022).
2.3. World Uncertainty Index
The WUI represents countrywide GDP-scaled average uncertainty estimates from emerging and developed economies across the globe. A total of 143 countries were covered in the comprehensive index, with coverage from developed economies like Australia, the majority of Europe, and North America, as well as some representatives from emerging and developing economies in Asia and Africa. The WUI captures a very wide element of uncertainty around the globe, with most recent events like the US-China trade tension, Britain’s exit from the European Union, the coronavirus pandemic, and not to forget the persisting Russia-Ukraine all factored into the analysis, making the dataset more comprehensive and accurate than other uncertainty indices. The regularity of the “uncertainty” phrase in reports from the economic intellectual units of 143 nations, both emerging and developed economies, is reflected in the WUI. The index is based on a single origin and incorporates essential issues pertaining to political and economic trends using a consistent methodology (Ahir et al., Citation2022). The comprehensive nature of data coverage makes the index enviable for global analysis and comparable to other related indices with limited coverage. Cross-country studies have found that there are substantial variations in the level of uncertainty between economies, with advanced economies generally offering lower levels of uncertainty than the rest of the globe (Ahir et al., Citation2022). In addition to being more prone to natural disasters like floods and native political tremors like rebellions and combats, emerging economies also tend to experience more of these incidents quite frequently. This renders their economies more precarious as they experience external shocks quite frequently, have less capacity to handle them, and also have enormous diversity (Ahir et al., Citation2022).
2.4. Cryptocurrency efficiency and economic uncertainties
The crypto market has been touted as informationally efficient but with exclusive characteristics of a semi-strong market (Kurihara & Fukushima, Citation2017; Vidal-Tomás & Ibañez, Citation2018). Kurihara and Fukushima (Citation2017) advanced the argument that the crypto market is not ineffective but that its dealings will advance over time, indicating that future returns will be random. In contrast to previous research that found the bitcoin market to be informationally inefficient, Tiwari et al. (Citation2018) further established the incidence of efficiency in the market, which complements the conclusions in Vidal-Tomás and Ibañez (Citation2018). In contrast, Bariviera (Citation2017) and Assaf et al. (Citation2023) further demonstrated the inefficiencies of the Bitcoin market together with its return patterns. According to their findings, daily yields present obstinate behaviour in the upper sampled segment of the study period, but their activity is more information-efficient in the early period. Furthermore, there was intense volatility in the return series during the period for daily peaks and troughs, and this demonstrates predictability. This indicates that the pricing and volatility are generated by a distinct underlying dynamic process. These findings were expanded to a broader perspective by Vidal-Tomás et al. (Citation2019). The researchers used a QR technique to investigate the determinants of efficient markets, and the bitcoin market was deemed inefficient due to indications of multifractality. These findings, when paired with the findings of other studies, substantiated the explanation for the market crash of 2018 and, similarly, those of 2022.
Following the crypto market’s strong price gains in 2017 and subsequent crashes in 2018, cryptos, especially Bitcoin, attracted the attention of a larger community, necessitating taking into account the influence of uncertainties, particularly those related to economic policy. Demir et al. (Citation2018) did an empirical study to see how the unpredictability of economic policy affects Bitcoin returns. EPU was characterised as having an adverse interrelation with bitcoin returns in a broader spectrum but with constructive and significant effects at both the optimal and trough of the bitcoin return distribution and the EPU. According to the findings, bitcoin functions as a hedging instrument against perils. Shaikh (Citation2020) extended this study by examining the EPU-Bitcoin nexus as well as the impact of the latter on the former in Asia (Japan, China, and Hong Kong), the United States (US), and the United Kingdom (UK). Bitcoin returns are more receptive to EPU in China, the US, and Japan, per the findings, and EPU adversely impacts the Japanese and US Bitcoin markets while the converse holds in China.
Kyriazis (Citation2021) explored the nexus between sophisticated digital assets and EPU. The focus of the research was to use empirical evidence to show a link between EPU and digital assets. Digital assets such as Bitcoin, Litecoin, and Ethereum were considered. Bitcoin’s performance is heavily linked to EPU, whereas Ethereum’s and Litecoin’s performances are only strongly linked to Bitcoin’s (Kyriazis, Citation2021). The study also discovered that the volatility uncertainty index had weak negative effects on both cryptos, with commodities like oil having weak positive effects on Ethereum. According to the findings, Ethereum and Litecoin might function as diversifiers in a portfolio comprising Bitcoin or hedges against the conventional classes during times of high stress, with the added benefit of not being influenced by the EPU. Hazgui et al. (Citation2021), on the other hand, studied the spectra and frequency-based connections between Bitcoin, EPU, gold, and oil. They assessed the co-movements between Bitcoin, the US-based EPU index, gold, and crude oil and further looked at the asymmetric frequency dependencies of the aforementioned variables. They discovered a constructive connection between the selected commodities (crude oil and gold) and bitcoin across the sample period at both medium and low frequencies, while the bitcoin-EPU nexus rather proved to be negative across the majority of the frequencies (low and medium). The findings suggest that strategic commodities and the EPU index can forecast BTC price returns across the above-listed frequencies.
In light of the findings from Colon et al. (Citation2021), political polarisation and instability pertaining to pecuniary circumstances adversely affect the bitcoin market. The bitcoin market’s ability to significantly function as a hedge (weak hedge and safe haven) against geopolitical perils (EPU) was revealed in the study. It was further proposed that perils and ambiguity stand as pertinent predictors of Bitcoin returns. Chen et al. (Citation2021) examined the bearing of the Chinese-induced EPU measure on the daily yields of Bitcoin and found a favourable bearing of the former on the latter. However, only the higher quantiles of the current Chinese EPU show a statistically significant positive impact (Chen et al., Citation2021). Furthermore, Cheema et al. (Citation2020) examined the nexus between crypto returns and EPU in multiple countries. The study looked at the EPU index’s capacity to predict crypto gains in nations with the most Bitcoin bumps, such as the United States, Germany, France, the Netherlands, Singapore, Canada, the United Kingdom, China, Russia, and Japan. It was revealed that in the short run, EPU indicates favourable bitcoin returns while they may lose their operative hedge and safe haven capacity against other financial assets during turbulence (Cheema et al., Citation2020).
Given the inconclusive findings from the above-mentioned adopted uncertainty metrics, one could argue against the efficiency of the EPU, which is limited in coverage as a measure of the crypto uncertainty index. Furthermore, the last quarter of 2022 saw the crypto market lurch into chaos. For instance, the Bloomberg investment hub as reported by Kharif (Citation2022) revealed an accumulated crypto market forfeiture of over $900 billion, with Bitcoin and Ethereum among the most significant cryptos further recording a whopping daily trailing value of approximately 13%. In lieu of this chaos and observed market fragility, and considering their pertinence to investors, several studies have been explored with various uncertainty measures introduced. For instance, studies such as Aharon et al. (Citation2022), Almeida et al. (Citation2022), Al-Shboul et al (Citation2022, Citation2023) and Bashir and Kumar (Citation2023), among others, have considered diverse indicators such as the role of investor sentiments through access to sensitive information and internal factors leading to the espousal of a twitter-based and crypto-specific (price and policy) uncertainty index as introduced by Lucey et al. (Citation2022). For instance, Al-Shboul et al. (Citation2022) presented a contention on the crypto market’s fragility to spillovers, particularly in light of the prevailing plague, and further reinforced the eclectic makeup of the ecosystem. The pandemic was the primary issue that garnered attention, and the study employed the recently created crypto (price and policy) uncertainty index as an uncertainty metric. It was discovered that Bitcoin (and Litecoin) lost (gained) their potential offsetting capacity during the pandemic, and it was also discovered that the pandemic had an enormous effect on the swings of the crypto market. This was modified by Aharon et al. (Citation2022), who appraised how Twitter- and industry-induced apprehension altered the digital currency market. Adopting, among others, the QR and causality tests, it was concluded that Bitcoin in particular is susceptible to media-based incertitude and that there is a causal link between the crypto market and the aforementioned uncertainty index. This was amiss given that these virtual currencies were perceived as efficient asset classes, particularly in light of the recent market declines.
In a quest to refute or substantiate the above series of findings and featuring Shannon’s entropy as a metric of scrutiny, Almeida et al. (Citation2022) accentuated investor sentiments regarding risk and scepticism in seven selected cryptos. The study identified Tether as the only crypto with a diverse pattern of uncertainty and risk, while the remainder of the sample, including Bitcoin and Ethereum, projected an identical structure. It emerged that the pandemic played an integral role in the market’s riskiness; nevertheless, the market’s risk spectrum dwindled during the elevated level of uncertainty, indicating the safe-haven potential of these categories of securities. The aforementioned results partly supplement yet concurrently refute prior results pertaining to the versatility of cryptosystems as havens. Inured by the pandemic and the recent plunges, Al-Shboul et al. (Citation2023) and Bashir and Kumar (Citation2023) validated the spillage of impulsivity into the crypto market by evaluating the safe-haven aptitude for a range of cryptos. These studies experimented with EPU and Twitter-induced uncertainty as uncertainties and vulnerability metrics, respectively. Al-Shboul et al. (Citation2023) reiterated EPU-induced trickling in the Bitcoin market, especially during the pandemic. Despite rising spillage in the crypto market, the study endorsed the industry’s safe-haven perspective, aligning with those of Aharon et al. (Citation2022) and Al-Shboul et al. (Citation2022), among others. Bashir and Kumar (Citation2023) laid out the fragmented implications of media-induced uncertainty on investor attitudes and confirmed that, while crypto volatility is favourably compromised by these uncertainty measures, its constituent returns are impaired, particularly during turbulence. These discoveries underscore the tenuous state of the crypto market’s safe haven and hedge prospects.
Given the above-mentioned limitation, coupled with the weaknesses earlier highlighted in the use of the conventional OLS as a measure of the crypto-uncertainty nexus, the basis of this study could only be appreciated. Previous studies on the crypto returns and uncertainty nexus have either focused on Bitcoin as the sole crypto due to market capitalisation or adopted the EPU as a measure of uncertainty. In the following line, we highlight the similarities and relevant deviations from previous studies. This study’s key deviations from previous studies are embedded in the use of the WUI instead of the EPU as a measure of economic uncertainty and considering an expansive number of cryptos other than focusing on Bitcoin. Our study is similar to studies by Shaikh (Citation2020), Colon et al. (Citation2021), Kyriazis (Citation2021), Demir et al. (Citation2018), and Mokni et al. (Citation2020) in measuring the crypto-uncertainty nexus and considering the hedge, the diversifier, and the safe-haven properties of cryptos amid global turmoil. Our study deviates from these studies by using seven (7) cryptos, including Bitcoin, and further proxying uncertainty with the comprehensive WUI instead of the limited EPU index. This was due to the fact that the crypto market is expanding with other cryptos gaining recognition amid the recent hikes in value. Therefore, considering Bitcoin as the sole crypto has limitations in terms of coverage. Similar to this limitation is the use of EPU as an uncertainty proxy. Considering the nature of cryptos as a universal asset, it could be argued that factors that affect their value are not limited to developed economies; therefore, there is a need to consider an expansive measure like the WUI that addresses such limitations. Nevertheless, Mokni et al. (Citation2020) and Colon et al. (Citation2021) deviate from this study only in the use of EPU as an uncertainty proxy.
Crypto returns and the uncertainty nexus are important to crypto investors, and an examination of this relationship using a WUI is as important as the value of these assets. These factors, as well as the diversity of findings across regions, further stimulate and rationalise the current study’s attempt to address these imperative research queries in contemporary contexts. To address the limitations mentioned above in previous studies, this study investigated the crypto-uncertainty nexus using QR and QQR and rather employed the conventional OLS as a benchmark in assessing the superiority of the earlier stated model while also using the WUI as an uncertainty proxy. Furthermore, the study considers the influence of investor sentiments and overall market volatility to unveil the genuine effects of the WUI on the sampled cryptos.
3. Data and methodology
3.1. Estimation models
We begin with a conventional linear regression and further adopt the QR approach in anticipation of revealing the nexus between economic uncertainties and crypto returns and diverse quantile-based segments. EquationEquation (1)(1)
(1) is an expression of classical regression, which exemplifies the nexus between the response and predictor variables linearly.
Where epitomises the noise term;
and
are the crypto returns and returns on global uncertainty respectively.
Accordingly, only one concern in this study can be addressed using the classical rectilinear reversion idealised structure in EquationEquation (1)(1)
(1) , and that is whether or not global uncertainties are relevant for crypto returns (Bouri et al., Citation2017; Colon et al., Citation2021). A crucial question, such as whether or not changes in uncertainties might have an impact on crypto returns subject to diverse market circumstances, may be unaddressed by the OLS model (Chang et al., Citation2020; Cheema et al., Citation2020). The quantile-based reversion framework offers an exact assessment of the effect of the predictor on the response variable than classical linear and non-linear regression approaches (Owusu Junior et al., Citation2020; Sim & Zhou, Citation2015). Given the limitations and submission mentioned above, we use QR to respond to this important query.
3.2. The quantile regression and QQR approach
QR approximates the contingent median of the predicting variable through diverse values of the predictor parameter, whereas the least squares approach emphasises the contingent mean of the response across values of the causal variables. The QR provides a more rigorous insight into the relationship than the OLS by establishing the relationship at distinctive quantiles of the regress and providing a reliable outcome on the studied variable’s nexus under precise market settings like normal, bullish, or bearish (Colon et al., Citation2021; Das et al., Citation2022; Owusu Junior et al., Citation2020). The QR is employed as an extension of the OLS subject to its failure to meet the stringent linearity requirements. Estimates based on QR are more resistant to outliers in response measurements. Because of its use and robustness, QR is recommended over regular least squares regression (Chang et al., Citation2020; Das et al., Citation2022). Since its invention, QR analysis has promised to be a prevalent technique for enormously modelling the varying structures and extents of dependence (Bashir & Kumar, Citation2023; Bouri et al., Citation2017; Das et al., Citation2022). It entails a set of varying segment-based reversion curvatures of the restrictive distributions of the predicting parameter across different quantiles, with the quantiles capturing different moments of the response variable (Chang et al., Citation2020, Demir et al., Citation2022; Das et al., Citation2022).
With QR, an idealised structure of the contingent segment of the explained component is generated for definite values of
. Therefore, the contingent QR model for
given the predicting variable (
) and accounting for other established relevant factors (
) found to affect the crypto markets, could be articulated as:
where the contingent quantile of the regressand,
, is epitomised by
, the intercept is characterised as
, and permissible to be subject to
. A trajectory of the
th related factors is defined as
, and
,
, and
, defines a vector of regressors, which in this study is restricted to returns on the GU, volatility uncertainty index (VIX), and general market volatility (OVX).
on the other hand, epitomises the noise term.
The quantile connection is scrutinised to match to the conservative OLS model defined in EquationEquation (1)(1)
(1) as it examines the upshot of uncertainties on crypto returns with QR estimations.
By means of the QR model in (3), the paper examines the conceivable uncertainty-crypto connection in nine (9) quantiles defined as ( = 0.05, 0.10, 0.25, 0.50, 0.60, 0.70, 0.80, 0.90, 0.95). Conditions will be attributed to the above-stated quantiles with the median quantile considered a normal market condition and with the quantiles below and above deemed bearish and bullish market conditions respectively. Given the broad spectrum of the WUI and its capacities to capture significant phenomena such as political polarisations and catastrophes, among others, and subject to the findings of Al-Shboul et al (Citation2022, Citation2023). Almeida et al. (Citation2022), Assaf et al. (Citation2023), Bashir and Kumar (Citation2023), and Yousaf and Ali (Citation2020) on the adverse contributing bearings of events such as the pandemic and polarisations on the crypto market, we will similarly analogise incidents such as the COVID-19 pandemic and the recent crypto market immersion to substantial economic phenomena that traverse the market into disarray and mimic an untenable market scenario, granted their promulgating adverse bearing as earlier stated.
The QR approach has the restraint of not being able to fully represent dependence (Bouri et al., Citation2017; Owusu Junior et al., Citation2020). In distinctive, the QR approach eliminates the reality that the type of uncertainty may also influence how crypto is related to GU. Given the limitations in the original QR approach, this study further adopts the QQR slant by Sim and Zhou (Citation2015) and further adopted by Bouri et al. (Citation2017). The nexus could evolve contingent on respective dispersion by modeling quantiles of the chosen crypto’s returns as a function of the quantile of the WUI using the QQR approach. The QQR technique is used by considering a set of quantiles of the independent variable (global uncertainties) and analysing the possible effects of each quantile on the diverse variates of response variables (crypto returns). The study extends the solitary model per Sim and Zhou (Citation2015) and further adopts it as adopted by Bouri et al. (Citation2017).
Accordingly, the QQR slant, on the other hand, is a modified form of standard QR that combines nonparametric estimation with QR to reveal the distinct quantile-based link between both endogenous and exogenous variables (Sim & Zhou, Citation2015). The framework offers substantiation for the conditional quantile linkage between multiple variables (Owusu Junior et al., Citation2020; Sim & Zhou). We extended the approach in this study with the following explanations:
Let Ø superscript represent the quantile of crypto returns designated by We presume a Ø-quantile-based framework for the selected crypto as function of its prior lags
and the economic uncertainty, which measure by the
Index. Formally, this can be expressed as:
where and
denote the crypto returns and global uncertainties at current period
,
is the Øth-variate of the provisional distribution of cryptos and
is the conditional quantile-based error variate with value conditioned to be zero and
(.) represents the slope of this relationship. Given that we do not know how crypto returns and global uncertainties are related, we assume that the connection function
(.) is unspecified, which is, in our opinion, a plausible assumption. In scrutinising the nexus between the conditional-based quantile of cryptos and the
th-variate of the GU Index, represented by
, we regularise the factor
through an extended first-order Taylor’s
(.) around
, with the following results:
We can further redefine (
) and
(
) and
and
respectively and to complement Sim and Zhou (Citation2015) and Bouri et al. (Citation2017). Our second equation can further be transformed as in (6):
Consequently, we substitute (6) into (4) to obtain the following:
where (*) springs the conditional variate of ɸ th of GUI. Also, it highlights the relation between the median value of crypto returns () and the variate of GUI (
) of
0 and
1 with ɸ and ɸ indices. The QQR is based on linear regression in the locality of
at which point stationarity can be relaxed (Owusu Junior et al., Citation2020; Sim & Zhou, Citation2015). Also, the QQR can capture series non-stationarity while describing the unevenness between low and high yields (Chang et al., Citation2020). Furthermore, we can infer the nexus between GU and cryptos over the short, intermediate, and extended term by quantifying the relationship between GU and cryptos utilising QR and QQR. The QR and QQR technique have proven to be efficient in examining the uncertainty-crypto nexus and it was established in studies such as Chang et al. (Citation2020), Colon et al. (Citation2021), Hazgui et al. (Citation2021) and Mokni et al. (Citation2020).
Following the estimated parameters of aforementioned model and the interpretations coined in the study by Wu et al. (Citation2019), which were adopted from the explanations of Baur and Lucey (Citation2010) on safe haven and hedge and further explored by Mokni et al. (Citation2022) and Colon et al. (Citation2021) the hedging and safe haven properties of cryptos could be verified as follows: If cryptos are positively allied (or uncorrelated) with the variation in uncertainty on middling, then we label crypto as a strong (or weak) hedge against uncertainty. We characterise cryptos as a stout (or frail) haven if they tend to correlate (or uncorrelated) with the average change in uncertainty during turmoil. Furthermore, if on average then crypto market is a hedge against GU. On the other hand, if
at extreme market conditions with lesser variates (
) and higher quantiles (
), then the crypto market is a safe haven at lower and higher GU conditions respectively. Note that the predictor (GU) values are used to estimate each quantile of the response variable (crypto returns) in the QR.
3.3. Variable and data description
The monthly-based empirical data for this study spans from January 2015 through December 2022, yielding 96 crypto-uncertainty observations. The seven (7) sampled cryptos were primarily conditioned on market capitalisation, representational ability, and significance of the currency, given the difficulty in analysing currencies with insignificant values, while the sample period is determined by data availability and variational susceptibility of the selected cryptos and also covering eras of global uproar such as the recent dreadful COVID-19 pandemic. Prices for each crypto which is treated as the predicting variable is obtained from https://coinmarketcap.com/ and quoted in USD. The prices of the selected cryptos are transitioned to returns by employing the initial monotonic differences of crypto prices. The monthly WUI data and those of CBOE OVX and VIX, which were respectively treated as predicting and interacting variables and proxies for global uncertainty, investor sentiments, and general market volatility, were respectively sourced from the databases of the National Bureau of Economic Research and Fred Economic Research. Instead of the EPU, we proxied global uncertainty with the WUI as the main predictor variable of interest. The WUI measures uncertainty related terms which are frequently harnessed by the economic expert intellectual unit country report. Furthermore, as espoused by Al-Yahyaee et al. (Citation2019) and Umar et al. (Citation2023), the VIX index, functioning as a gauge of international investor risk, engenders profound ramifications for the assessment of portfolio risks. This is due to the discernment of investors who craft their anticipations and investment tactics predicated upon the magnitude of peril unveiled by the sentiments. Furthermore, Das et al. (Citation2022) further underscored the significance of the fact that volatility within the realm of the oil market (OVX) exerts an effect encompassing virtually every facet of the financial markets, thereby necessitating its incorporation in studies exploring the domain of uncertainty.
Similar to preceding papers (Colon et al., Citation2021; Wang et al., Citation2019; Yarovaya et al., Citation2021), this study implements the logarithmic transition on the monthly prices to compute returns for crypto in month
, as the main dependent variable. The log-returns of cryptos are expressed as
whiles that of global uncertainty is captured as
. Where
and
characterise the incessantly heightened returns of crypto and global uncertainties,
epitomises the crypto value or index of global uncertainties in the contemporary period,
, and
signifies the crypto price or index of global uncertainties in the preceding period.
3.4. Preliminary analysis
Graphical analysis reveals that, with a few notable exceptions, all of the chosen cryptos and the uncertainty indexes had a volatile trend. The uncertainty indexes began to trend upward in August 2016 and later took a downward trend in February 2018, following which it stabilised with little volatility. After a severe decrease between February 2018 and March 2019 and a subsequent rebound in August 2019, the bitcoin market was perceived to be fairly volatile in terms of cryptos. The bitcoin market and uncertainties move in an opposite direction indicating uncertainties have a negative effect on bitcoin. Throughout the study period, there were periods of upward and downward movement in the Monero market. The market started moving upward in February 2018, experienced another surge in August 2018, and has since experienced turbulence. Despite a number of fluctuations, the Monero market has proven to be more stable than the Bitcoin market, confirming its hedge status (Owusu Junior et al., Citation2020). Litecoin, Dashcoin, XRP, and Neo after the piercing waning in the uncertainty measure between February and August 2018 apprehended ascendent inclinations interspersed with few explosiveness. The Ethereum market rather took an opposite trend with an upward trend coinciding with rising uncertainty and vice versa. Surprisingly, the period (2019–2022) featured an array of peaks and troughs in crypto prices, as well as uncertainty metrics. This implies the pandemic exerted a bearing on the crypto price series. This complements the findings of Aharon et al. (Citation2022), Al-Shboul et al. (Citation2022, Citation2023), and Bashir and Kumar (Citation2023), all of whom observed an intriguing impact of the COVID-19 pandemic on both conventional and virtual currencies. In essence, the crypto market could be deemed highly volatile given the inconsistencies in surge with only minor stability, this notwithstanding the prior evidence (Aharon et al., Citation2022; Al-Shboul et al., Citation2023; Bashir & Kumar, Citation2023; Kyriazis, Citation2021; Mokni et al., Citation2022) which endorses the crypto market as either a hedge, safe haven or diversifier amid uncertainties. Furthermore, a visual assessment of the market with inconsistencies in the trends of crypto return and price series shown in Figure could attest to the findings of Vidal-Tomás and Ibañez (Citation2018), Tiwari et al. (Citation2018), and Kurihara and Fukushima (Citation2017) on the market’s near sturdy efficiency. There were series of upward and downward shift in the uncertainty measures with the sample metrics (independent and control variables) achieving their highest value during the pandemic period. This further indicate the bearing of the global health crisis in terms of uncertainties.
3.5. Descriptive statistics
Table highlights the descriptive statistics of the return series employed in the study. The results reveal that, overall, the returns on the selected cryptos are highly skewed to the right and leptokurtic. This “high peak and fat tail” distribution is typical in the crypto literature (Chowdhury et al., Citation2021; Colon et al., Citation2021; Wu et al., Citation2019). We performed a battery of tests on the fundamental prerequisite for pecuniary series analysis, which further affirmed our model’s choice. We utilised the augmented version of the Dickey-Fuller and Phillip-Perron tests to diagnose the existence of unit roots in the return series, as this is the fundamental requirement for time-series analysis. The findings imply that the null hypothesis that unit roots exist in every series across the sampled period is rejected. The Jarque-Bera statistic demonstrates that all of the variables significantly deviate from normality, with the majority exhibiting fat tails or extreme values, while the Ljung-Box test indicates the absence of autocorrelation among the series except for the Monero. Furthermore, the statistics point to non-normality in the dataset, which validates our selection of quantile estimates and yields more accurate findings than traditional linear models (see Chowdhury et al., Citation2021; Colon et al., Citation2021; Troster et al., Citation2018). On average, the Ethereum return is slightly superior to that of the remaining cryptos, including Bitcoin, with the lowest average returns observed in Ripple. The selected cryptos exhibit a positive average return over the study period, with the opposite detected in the uncertainty measure. Ripples exhibited the highest precariousness throughout the period, with VIX showing the least volatility.
Table 1. Descriptive statistics and preliminary analysis
The correlation matrix exhibited a blend of weak and strong relation between crypto returns and global uncertainty, with the highest (lowest) correlations observed in Ethereum (VIX), respectively. There was a combination of both negative and positive correlation between sampled uncertainties and the selected cryptos, indicating that while some cryptos directly co-move with variations in global uncertainty, others exhibited the reverse. Also, there was a strong, near-perfect, diverse directional correlation among the majority of sampled cryptos, with the highest coefficient (0.989) recorded between the Dashcoin and Neo pairs. The interaction between the majority of the response and the predictor variable and those among the predictor variables is said to be significant at 1%, while the remaining pairs remain insignificant. This indicates that the majority of the sampled crypto market and the uncertainty measure are informed about each other’s dynamics. This is not surprising given the comprehensive nature of the WUI as an uncertainty measure (Ahir et al., Citation2022), yet the startling findings remain the insignificant correlation that exists between Bitcoin and global uncertainty. The study, consequently, highlights the summarised pairwise correlation matrix between the study variables in Table .
Table 2. Correlation matrix of global uncertainties and cryptocurrencies
4. Empirical results
4.1. Global uncertainty and the cryptocurrency market: OLS results
The QR (conventional OLS) model results, as highlighted in Equation 9 (1), are summarised in Tables , respectively. Table reports estimates by regressed crypto returns, with the GU being the main independent variable. The estimated coefficient is positioned in the initial column for each model, as is the relevant OLS diagnostic test. It is pertinent to acknowledge the values in the parenthesis as the estimates’ respective standard errors. The findings from the classical OLS model point to a blend of positive significant (insignificant) nexus between GU and crypto returns, except for Monero (Ripple), which exhibited a blend of negative significant (insignificant) nexus with GU. These OLS model results do not exclusively but moderately conform with the findings of Aharon et al. (Citation2022), Al-Shboul et al. (Citation2023), Colon et al. (Citation2021), Foglia and Dai (Citation2021), and Kyriazis (Citation2021), who respectively examined the safe haven and diversification features of cryptos amid uncertainty (EPU and Twitter-based) and indicated that the aforementioned uncertainty measures impact crypto returns positively. On the other hand, the negative significant (insignificant) nexus for the few selected cryptos is, however, partly consistent with those of Bashir and Kumar (Citation2023), Hazgui et al. (Citation2021), Shaikh (Citation2020), Mokni et al. (Citation2020), and Wu et al. (Citation2019), who all discovered a negative EPU bearing on crypto returns. This study complements the plethora of research demonstrating the effects of economic upheavals on the crypto market that already exists. Also, the diagnostics (autocorrelation, homoscedasticity), as shown in Table , revealed that all the estimates meet the majority of the stringent uniformity requirements with a minor exception. Nevertheless, the limitation of OLS in capturing extreme events further necessitates the QR model for such an effect. For instance, as established by Koenker and Hallock (Citation2001) and further substantiated by the subsequent studies carried out by Das et al. (Citation2022) and Owusu Junior et al. (Citation2020), the QR approach exhibits remarkable resilience in addressing difficulties associated with outliers, which are mostly inherent in time-series data. Accordingly, this resilience stems from the QR method’s utilisation of the conditional median, which deviates from the static mean, thereby offering a more robust alternative. Consequently, these findings underscore the significance of employing QR despite its acknowledged limitations, as previously posited.
Table 3. OLS regression estimation results for crypto-uncertainty nexus
Table 4. Nexus between cryptocurrencies and global uncertainty via quantile regression
4.2. Quantile regression results
The QR provides a more rigorous insight into the relationship than the OLS by establishing the relationship at distinctive quantiles of the regress and providing a reliable outcome on the studied variable’s under precise market conditions like normal, bullish, or bearish (Colon et al., Citation2021; Owusu Junior et al., Citation2020). Likewise, this permits us to comprehend the means by which uncertainty-driven incidents featuring the COVID-19 pandemic, political polarisations, and underlying market disruptions alter crypto markets and the dispersion of returns, as well as how these implications transition periodically (Colon et al., Citation2021; Yarovaya et al., Citation2021). Subject to the aforementioned suppositions, we assess imbalances in crypto returns in response to GU disruptions by computing coefficients spanning various quantiles and subsequently utilising the QR framework. Thus, we analyse how the crypto market reacts to variations in uncertainty around certain quantiles (0.05, 0.10, 0.25, 0.50, 0.60, 0.70, 0.80, 0.90, and 0.95). Our analysis is dissected into two parts to incorporate the influences of investor sentiment (VIX) and general market volatility (OVX) on the nexus. Consequently, the outcomes of the initial (subsequent) examination, which take into consideration the absence (presence) of the control variables in the crypto-uncertainty relationship, are succinctly presented in Panel B of Table (Table ). Generally, the discoveries in both series of the QR estimation divulge an overlap between the crypto-uncertainty nexus, with a comparatively larger influence at lower quantiles.
Table 5. Nexus between cryptocurrencies and global uncertainty with VIX and OVX as control variables
The QR results from Table reveals the existence of strong hedging capacity in the crypto market in most cases, as characterised by a significant positive nexus between most of the selected cryptos (Dashcoin, Ethereum, and Neo) and GU at normal market conditions ( = 0.50, 0.60, and 0.70), with minor exceptions (Monero and Ripple). Also, we find the relationship to be significant at the 1% level, with the exception of Bitcoin, which experiences a positive but insignificant nexus with GU shocks. This implies that the cryptos (Dash, Ethereum, and Neo) might act as a potent hedge in a normal market. These results corroborate those of Cheema et al. (Citation2020), Demir et al. (Citation2018), and Yen and Cheng (Citation2021), who found the crypto market to be more of a source of hedging instruments than diversifiers. The findings confirm that, during normal times, investors can profit by investing in the aforementioned cryptos since they are less vulnerable to shocks from the GU given the direct acclimatisation among them. The Bitcoin market, on the other hand, could, to some extent, function as a weak hedge against GU shocks in normal market scenery (Colon et al., Citation2021; Wu et al., Citation2019). Monero (Ripple), on the other hand, exhibited a negative significant (insignificant) coefficient against GU at normal market conditions, indicating that they cannot act as a hedge against normal GU shocks but only as a diversification asset in a portfolio (Kyriazis, Citation2021; Papadamou et al., Citation2021).
Comparatively, the most significant cryptos in terms of capitalisation, with the exception of Ethereum, could not act as hedge instruments against GU in the normal market. This could as well mean that the hedging ability of an asset could not be limited to its significance but also its stability, as instruments with such an ability proved to serve the hedging properties. Given that we likened the normal market to period absent any chaos, we could conclude that, even in the face of stability absent travesty, cryptos such as Monero and Ripple could not act as hedge instrument against GU shocks but only as diversifiers. The results further revealed that is significant at higher quantiles (
= 0.80, 0.90, and 0.95) for the same markets that were significant in the normal periods except for the Bitcoin and Ripple markets which rather exhibited significant but adverse coefficients. This implies that, regardless of the realisation that their import scale has shifted, they are unable to function as a hedge against GU disruptions, but instead as diversifiers. This suggests that they could only be injected into holdings for the purpose of diversification. Bitcoin’s coefficient and resilience shifted from a weak hedge in a conventional market to a diversifier instead of a hedge in a bullish market, which was a staggering development. This proves the existence of a variational structure in the crypto (Bitcoin) market. The remaining cryptos exhibited strong hedging potential against GU in a bullish market against higher GU shocks (Cheema et al., Citation2020; Demir et al., Citation2018; Yen & Cheng, Citation2021). Also, except for Bitcoin, which displayed a pattern of directional shift, the remaining cryptocurrencies preserved their trajectory, signalling that investors should be wary of greater discrepancies and fluctuation in the Bitcoin market. Given the discrepancy of its swings in instances of unpredictability, this could additionally clarify the rationale behind the higher daily diminution in its value during periods of chaos. Therefore, in contrast to the findings of Kyriazis and Papadamou et al. (Citation2021) and partially conflicting with the findings of Colon et al. (Citation2021), Demir et al. (Citation2018), and Yen and Cheng (Citation2021), who respectively found cryptos as either diversifiers or weak hedges both in normal and bullish markets, this study has proven that not all the crypto market has the same pattern of capacity and direction, and further proved that cryptos (Dash, Ethereum, and Neo) could act as strong hedges both in normal and bullish markets.
The safe-haven capabilities of the crypto market were evaluated with the lower quantiles ( = 0.05, 0.10, and 0.25) which depict bearish market conditions, or rather pandemonium, such as the initially aligned plague, plunges, and political polarisations, among others. In recognition of their affirmative significant coefficients in almost all the more bearishly juxtaposed quantiles, it emerged that all cryptos, regardless of their hedging potential in both normal and euphoric market scenarios, could operate as viable havens, despite also acknowledging that the Ripple market has a limited capacity as a safe haven across all adverse quantiles (Colon et al., Citation2021; Wu et al., Citation2019). In addition, notwithstanding the fact that cryptos such as Bitcoin and Litecoin, which failed to hedge against GU shocks in normal market conditions, had haven prospects in a bear market, their abilities were found to be inconsistent, with a blend of weak and strong haven prospect across the bear quantiles. This validates their initial variational capacities, which speculators must recognise due to their import and the fact that they cannot rely exclusively on them during investment decisions. Across all bearish-embedded quantiles, Ripple (Dash, Ethereum, and Neo) maintained consistently frail (strong) safe-haven capacities, signalling their consistency. This also suggests that the cryptos (Dash, Ethereum, and Neo) could withstand extraneous market disruptions such as the COVID-19 pandemic and polarisations, among others, during periods of heightened distress.
Given the study’s findings, we could conclude that, on average, the selected cryptos could serve as both a strong (or weak) safe haven against GU shocks in terms of a crisis, given the large series of positive and significant (or insignificant) coefficients exhibited in most markets, with Monero being the only exception. These results corroborate the discoveries of Aharon et al. (Citation2022), Almeida et al. (Citation2022), Al-Shboul et al. (Citation2023), Colon et al. (Citation2021), Paule-Vianez et al. (Citation2020), Wang et al. (Citation2020), and Wu et al. (Citation2019), who independently argued that the crypto market can act as a frail (Colon et al., Citation2021; Wu et al., Citation2019) and stout (Aharon et al., Citation2022; Almeida et al., Citation2022; Al-Shboul et al., Citation2023; Paule-Vianez et al., Citation2020; Wang et al., Citation2020) safe haven against GU shocks during periods of economic ambiguities including pandemic, market plunges and political polarisation. The findings contradict those of Bashir and Kumar (Citation2023), Bouri et al. (Citation2017), Chen et al. (Citation2021), Cheema et al. (Citation2020), Demir et al. (Citation2018), Shaikh (Citation2020), and Yen and Cheng (Citation2021), which argued on the contrary, and Kyriazis (Citation2021), Mokni et al. (Citation2020), and which both concluded that the crypto market could only act as an alternative asset during global uncertainty. In essence, during instances of turmoil, investors could incorporate cryptos (Dash, Ethereum, and Neo) into their portfolios to mitigate exposure and loss.
Table , conversely, presents the results of the crypto-uncertainty nexus while controlling for the impact of investor sentiment (VIX) and market volatility (OVX). Analogous to the outcomes observed in the crypto-uncertainty nexus absent controls, cryptos (Dash, Ethereum, and Neo) exhibited a positive nexus with GU both in normal, bullish, and bearish market conditions, which indicates that even absent volatility and investor sentiment, the aforementioned crypto can act both as a hedge and a safe haven in both normal and bearish market. Nevertheless, the magnitude of significance for the above nexus was comparatively higher than the analysis without control variables, implying that the impact of general uncertainty on Dash, Ethereum, and Neo holds relatively substantial importance in the absence of investor sentiment and market volatility.
In a similar vein, Monero demonstrated a noteworthy negative correlation with GU, thus reinforcing its lack of capability to function as a hedge or safe haven in both normal and bearish market conditions, as previously established. Conversely, cryptos such as Bitcoin, Litecoin, and Ripple exhibited a comparable pattern of nexus with GU, differing only in terms of the level of significance. Thus, the aforementioned nexus was determined to be statistically insignificant across all quantiles while still maintaining its directional relationship, as elucidated in Table . This implies that these cryptos can only offer a feeble level of hedging (safe haven) during normal (turbulent) periods, contrary to the findings of the earlier analysis. Interestingly, it was further discovered that despite the lack of a significant nexus between the aforementioned cryptos (Bitcoin, Litecoin, and Ripple) and GU, they were significantly influenced by VIX across all quantiles. This further substantiates our earlier conjectures, as well as those of Das et al. (Citation2022) and Umar et al. (Citation2023), regarding the impact of investor sentiment on the crypto market. It suggests that both GU and VIX could be simultaneously employed as measures of uncertainty in the realm of cryptos, given their respective divergent capacities as uncertainty indicators.
4.3. QQR results
To improve our understanding of the QR results, we further evaluate the crypto-uncertainty nexus utilising the QQR to affirm or refute the notion that GU plays a role in the restrictive transmission conduct of crypto yields and their variant occurrences. The QQR is a non-parametric method that generates no statistical significance for the regression coefficients but disintegrates QR projections into precise quantiles of the explanatory variables (Owusu Junior et al., Citation2020; Sim & Zhou, Citation2015). The QR, on the other hand, can be used to surmise the QQR’s plausibility. Conversely, the salience of the QQR model lies in the intrinsic capacity of its average coefficients to manifest values that encapsulate the values of the corresponding QR coefficients. Hence, the fundamental rationale behind the utilisation of the QQR model is to reinforce the authenticity and reliability of the system within the purview of the study. The QQR results are concisely presented in Table .
Table 6. Crypto-GU estimations via QQR
The results show that the QQR and QR projections are somewhat identical but also exhibit slight diverging trends and a mixture of assimilation and disintegration across certain segments, as shown in Table . Also, in the trivial portion of the distribution, QR is largely greater than QQR with minor exceptions; thus, while the QR case generally outperforms the QQR at lower frequencies of crypto returns, the relationship is rather mixed, with the majority being positive, with the key exception being Monero (Ethereum and Neo), where the relationship was found to be negative (where QR projections virtually edged out QQR estimations at all scales). This further confirms our earlier submission about Monero’s (Ethereum and Neo’s) inability (ability) to hedge in normal market and is consequently presented graphically in the robust visualisation, which further validates the robustness of the QQR estimation. In addition, both the QR and QQR estimates in the visualisation were somewhat unstable across all quantiles, while comparatively, the QR exhibited some level of stability, especially for Ethereum and Monero. Also, it is pertinent to acknowledge that the QQR analysis is subjected to the same analysis with regards to diversification, hedging, and safe-haven as earlier applied to QR projections, with the only difference being magnitudes. Furthermore, cryptos’ hedge capacity against uncertainty is restrictive to the market (bullish or bearish) and the level of uncertainty (low or high).
In summary, the empirical evidence conveys a differing influence across the sampled crypto-GU quantile, with certain commonalities applicable to particular crypto segments and market dynamics, as earlier established in the QR estimates. It merits acknowledging that the procedure is hardly conceivable to deduce the degree of significance associated with the coefficients owing to the non-parametric mechanism implemented in QQR projections. However, the subsequent robustness test shows that our QR outcome metrics have validated these estimates.
4.4. Robustness test for QQR
Since the QQR is a non-parametric algorithm, identifying the statistical significance associated with the metrics it discloses is complicated (Bouri et al., Citation2017; Owusu Junior et al., Citation2020). Therefore, the QR approximations can be utilised to validate the QQR outputs while assisting in the comprehension of the QR’s interactions (Bouri et al., Citation2017; Sim & Zhou, Citation2015; Owusu Junior et al., Citation2021). The line-based visualisations highlighting the QR and QQR coefficients in Figure perform several functions, which comprise imagining the QR projections, signifying the extent to which GU returns are becoming more prevalent or hampering and how that corresponds with the variations in crypto returns, and affirming the QQR by contrasting it to the QR projections. The inclination of the diagonal (horizontal) dimension of the visualisations represents the quantile projections of QQR (QR). Musters of grey and black align with QQR and QR estimates spanning diverse quantiles. Following the visualisations in Figure , the QR and QQR estimates for the vast majority of fragments are coherent. With a slight variation in the severity of the outcome at certain quantiles, movements are in the same direction as indicated by quantile estimates. Regardless of this, the QRR estimates are validated by the QR estimates.
4.5. Further robustness test
To further establish the robustness of the QR results and limit any possibility of bias estimation, we further employ a battery of tests, including the test of autocorrelation in the residuals of the sample quantiles as outlined in Table . This algorithm follows previous studies (Archer et al., Citation2022; Koenker, Citation2005). Notably, it was evident that the residuals across various quantiles for the majority of the most significant nexus models do not exhibit autocorrelation, with probability values exceeding 5%. Notably, only a few, including XRP and BTC, exhibited a glimpse of autocorrelation across some of the sample quantiles. Consequently, our model is deemed robust in elucidating the multifaceted nexus between cryptos and global uncertainties.
Table 7. Results of Residual-based LaGrange multiplier (LM) autocorrelation test for QR
Given the observed autocorrelation in a few of the sampled normal quantiles, we proceed by conducting the causality in quantile test to further establish the extent of causal relation among the sample and also affirm the robustness of the model. This extra step is taken because we acknowledge that the existence of an autocorrelation in the quantile residuals could potentially influence the accuracy of our results and raise the issue of spurious correlation.
4.6. Causality test
To validate the study’s robustness, we additionally present a non-linear causality-in-quantile analysis, building upon prior research (Archer et al., Citation2022; Balcilar et al., Citation2017). The pertinence of this analysis is inherent in the model’s ability to capture varying causal relation flowing from the uncertainty measures towards the conditional distributions of crypto returns unlike the conventional Granger causality test which only examines the average distribution. Therefore, this method may demonstrate how causality works in both low and high energy returns. In general, the outcomes of the aforementioned causality test, both in terms of mean and variance are depicted in and numerically backed by the test statistics in Table . From Figure and Figure , the test statistics are matched against the vertical axis with the quantiles on the horizontal axis in each plot. The 5% significance level, which corresponds to a critical value (CV) of 1.96, is depicted by the horizontal solid line. The null hypothesis states that a change in global uncertainty does cause changes in crypto returns and volatility. Contrary to the QR analysis, we reject the null hypothesis of no causal relation in the lower quantiles of the crypto-uncertainty nexus, while we fail to reject the null at the normal and upper quantiles with few exceptions, especially in the case of Neo. Despite the inconsistencies in the mean causal relation, the results for the causality in variance rather indicate that GU remains the most significant metric that drives the volatility of the crypto market across all quantiles, with few exceptions. This demonstrates that, comparably, the global uncertainty measure rather exerts a noteworthy causal impact on the transmission of risk within the crypto markets than the returns, as portrayed in Table and . These results underscore the robustness of our findings, with the causality-in-quantile analysis proving to be dependable in this regard.
Table 8. Results of causality in mean and variance test
5. Conclusions
This study complements prior research through an extension of the dialogue on the nexus between economic uncertainty and the crypto market to identify hedging and safe haven possibilities in the response variables. We demonstrate the active variational reactions of the crypto market against global uncertainty with and absent the impact of investor sentiments and market volatility, and we further conclude on the semi-strong efficiency of the market given the inconsistencies in the trends of crypto price and return series as established in the literature. We used monthly return series of both the predictor and response variables while also employing QR techniques in model estimations. The QR captures the unevenness in the connection at different quantiles of the return dispersion. Our sampled period spanned January 2015 and December 2022. We found QR a valid model to examine the crypto-GU nexus across diverse quantiles.
Subject to the conditions of private and safe haven, we found the selected cryptos (Dash, Ethereum, and Neo) to function as a strong hedge (strong safe-haven) against GU shocks during normal (turbulence) market scenery. On the other hand, cryptos (Bitcoin, Litecoin, and Ripple) were found to possess only the capacity to function as diversifiers (weak safe havens) in normal (turbulent market scenery, which was characterised as a period with intense pandemics, polarisations, and crypto market crashes) market scenery against GU shocks. The results further revealed that most of the selected crypto markets could function as safe-haven assets, except for Monero. On average, the crypto market could serve as a safe haven against GU amid the turmoil. These results corroborate the discoveries of Aharon et al. (Citation2022), Almeida et al. (Citation2022), Al-Shboul et al. (Citation2023), Colon et al. (Citation2021), Paule-Vianez et al. (Citation2020), Wang et al. (Citation2020), and Wu et al. (Citation2019), who independently argued that the crypto market can act as a frail (Colon et al., Citation2021; Wu et al., Citation2019) and stout (Aharon et al., Citation2022; Almeida et al., Citation2022; Al-Shboul et al., Citation2023; Paule-Vianez et al., Citation2020; Wang et al., Citation2020) safe haven against GU shocks during precariousness. The findings contradict those of Bashir and Kumar (Citation2023), Bouri et al. (Citation2017), Chen et al. (Citation2021), Cheema et al. (Citation2020), Demir et al. (Citation2018), Shaikh (Citation2020), Yen and Cheng (Citation2021), which argued on the contrary, and Kyriazis (Citation2021), Mokni et al. (Citation2020), which both concluded that the crypto market could only act as an alternative asset during global uncertainty. Similarly, the results accounting for the impact of investor sentiments (VIX) and market volatility (OVX) further corroborate the initial results, with the only exception being the significance of Bitcoin, Litecoin, and Ripple. Thus, the results further revealed that the nexus between GU and the aforementioned markets was insignificant across all quantiles. Nevertheless, these markets were rather significantly affected by investor sentiment, which corroborates the findings of Das et al. (Citation2022) and Umar et al. (Citation2023). Furthermore, the QQR and causality-in-quantile results complement the QR results, with some exceptions concerning magnitude.
Furthermore, theoretically, the MPT and AMH were confirmed, among other things, within the scope of dynamic asymmetric hedge and safe haven attributes as opposed to the EMH, given the established diversification prospect for some of the selected cryptos (Dash, Ethereum, and Neo) both in normal and turbulence, with the key exception being Monero (MPT). The directional variations of the crypto-uncertainty nexus across diverse quantiles, coupled with the exhibited varying degree of adaptability, further confirm the adaptive nature of crypto market participants given diverse conditions (Ghazani & Jafari, Citation2021; Khuntia & Pattanayak, Citation2018).
5.1. Recommendation and policy implications
Investors should only consider the cryptos (Dash, Ethereum, and Neo) for hedge and haven purposes, while the cryptos (Bitcoin, Litecoin, Monero, and Ripple) should only be added to a portfolio for diversification purposes other than hedge and safe haven assets, given the above findings. Also, given that there is a substantial nexus between the crypto market and the WUI, it is recommended that investors consider the dynamics of this index in their choice of cryptos to inject into their portfolios, especially when not all the sampled cryptos possess the abilities to hedge or act as havens in normal and turbulence. Also, even though some of the selected cryptos were found to be haven and hedge assets, it is relevant to acknowledge that the crypto market, in general, is full of peril, as exhibited by the yield and peril metrics and considering the consistent crash in the market. On the other hand, decisions to invest in such a market will largely depend on the investor’s level of tolerance for risk if augmented profits are to be relied upon.
Furthermore, policymakers, especially in technologically advanced economies where cryptos serve as a means of transaction, are to critically trail GU variations since precariousness in their movements substantially influences the crypto markets, and likewise organise relevant education for their citizens to enable them to appreciate the dynamics of the market before investing in these asset classes. Thus, information flows throughout industries must be thoroughly regulated to prevent investors from making impulsive decisions. Since strong hedges and safe havens are only viable in some of the crypto markets, with the majority exhibiting a mixture of variational capacities, it may not be viable to invest in a random crypto market without considering how they relate to uncertainty. Furthermore, regulatory authorities, including the government, should be engaged in regulating the market by devising strategies to strengthen it, as suggested by Spithoven (Citation2019).
5.2. Limitations and direction for future research
The outcomes of this inquiry were confined to the sampled cryptos and the all-encompassing WUI. Therefore, the generalisability of these outcomes could be impeded due to the existence of numerous comparable cryptos with analogous uncertain characteristics that were excluded from the study, and the employed uncertainty index may not encompass all pertinent worldwide phenomena, particularly those that are specific to the market. Notwithstanding the reasons for the sampled data, it is important to acknowledge this constraint. In forthcoming studies, researchers could amplify the quantity of sampled cryptos and consider employing distinct uncertainty indices in addition to global uncertainty to guarantee a proper comparison. For instance, a confluence of world and crypto market uncertainty indices could be utilised. Given the dissimilarities between the present study’s findings and those of previous literature, it may be worthwhile to undertake a concomitant comparative analysis of a broader spectrum of cryptos and diverse uncertainty indices to corroborate or disprove prior results in a solitary study. Furthermore, it may be beneficial for future researchers to explore whether the newly developed all-inclusive uncertainty index could impact other assets apart from cryptos, which could be advantageous for portfolio diversification and investor preferences.
Disclosure statement
No potential conflict of interest was reported by the author(s).
References
- Adediran, I. A., Yinusa, O. D., & Lakhani, K. H. (2021). Where lies the silver lining when uncertainty hang dark clouds over the global financial markets? Resources Policy, 70, 101932. https://doi.org/10.1016/j.resourpol.2020.101932
- Aharon, D. Y., Demir, E., Lau, C. K. M., & Zaremba, A. (2022). Twitter-based uncertainty and cryptocurrency returns. Research in International Business and Finance, 59, 101546. https://doi.org/10.1016/j.ribaf.2021.101546
- Ahir, H., Bloom, N., & Furceri, D. (2022). The world uncertainty index (no. w29763). National Bureau of Economic Research https://www.nber.org/papers/w29763
- Almeida, D., Dionísio, A., Vieira, I., & Ferreira, P. (2022). Uncertainty and risk in the cryptocurrency market. Journal of Risk and Financial Management, 15(11), 532. https://doi.org/10.3390/jrfm15110532
- Al-Shboul, M., Assaf, A., & Mokni, K. (2022). When bitcoin lost its position: Cryptocurrency uncertainty and the dynamic spillover among cryptocurrencies before and during the COVID-19 pandemic. International Review of Financial Analysis, 83, 102309. https://doi.org/10.1016/j.irfa.2022.102309
- Al-Shboul, M., Assaf, A., & Mokni, K. (2023). Does economic policy uncertainty drive the dynamic spillover among traditional currencies and cryptocurrencies? The role of the COVID-19 pandemic. Research in International Business and Finance, 64, 101824. https://doi.org/10.1016/j.ribaf.2022.101824
- Al-Yahyaee, K. H., Rehman, M. U., Mensi, W. & Al-Jarrah, I. M. W. (2019). Can uncertainty indices predict bitcoin prices? A revisited analysis using partial and multivariate wavelet approaches. The North American Journal of Economics & Finance, 49, 47–33. https://doi.org/10.1016/j.najef.2019.03.019
- Archer, C., Junior, P. O., Adam, A. M., Asafo-Adjei, E., & Baffoe, S. (2022). Asymmetric dependence between exchange rate and commodity prices in Ghana. Annals of Financial Economics, 17(2), 2250012. https://doi.org/10.1142/S2010495222500129
- Assaf, A., Mokni, K., Yousaf, I., & Bhandari, A. (2023). Long memory in the high frequency cryptocurrency markets using fractal connectivity analysis: The impact of COVID-19. Research in International Business and Finance, 64, 101821. https://doi.org/10.1016/j.ribaf.2022.101821
- Baker, S. R., Bloom, N., & Davis, S. J. (2016). Measuring economic policy uncertainty. The Quarterly Journal of Economics, 131(4), 1593–1636. https://doi.org/10.1093/qje/qjw024
- Balcilar, M., Bekiros, S., & Gupta, R. (2017). The role of news-based uncertainty indices in predicting oil markets: A hybrid nonparametric quantile causality method. Empirical Economics, 53(3), 879–889. https://doi.org/10.1007/s00181-016-1150-0
- Bariviera, A. F. (2017). The inefficiency of Bitcoin revisited: A dynamic approach. Economics Letters, 161, 1–4. https://doi.org/10.1016/j.econlet.2017.09.013
- Bashir, H. A., & Kumar, D. (2023). Investor attention, Twitter uncertainty and cryptocurrency market amid the COVID-19 pandemic. Managerial Finance, 49(4), 620–642. https://doi.org/10.1108/MF-09-2021-0414
- Baur, D. G., & Lucey, B. M. (2010). Is gold a hedge or a safe haven? An analysis of stocks, bonds and gold. Financial Review, 45(2), 217–229. https://doi.org/10.1111/j.1540-6288.2010.00244.x
- Bouri, E., Gupta, R., Tiwari, A. K., & Roubaud, D. (2017). Does Bitcoin hedge global uncertainty? Evidence from wavelet-based quantile-in-quantile regressions. Finance Research Letters, 23, 87–95. https://doi.org/10.1016/j.frl.2017.02.009
- Chang, B. H., Sharif, A., Aman, A., Suki, N. M., Salman, A., & Khan, S. A. R. (2020). The asymmetric effects of oil price on sectoral Islamic stocks: New evidence from quantile-on-quantile regression approach. Resources Policy, 65, 101571. https://doi.org/10.1016/j.resourpol.2019.101571
- Cheema, M. A., Szulczyk, K., & Bouri, E. (2020). Cryptocurrency returns and economic policy uncertainty: A multicountry analysis using linear and quantile-based models. SSRN Electronic Journal. https://doi.org/10.2139/ssrn.3567635
- Chen, T., Lau, C. K. M., Cheema, S., & Koo, C. K. (2021). Economic policy uncertainty in China and Bitcoin returns: Evidence from the COVID-19 period. Frontiers in Public Health, 9, 140. https://doi.org/10.3389/fpubh.2021.651051
- Chez, B. (February. 2022). Cryptocurrency market capitalisation. Retrieved from https://coinmarketcap.com/
- Chowdhury, M. S. R., & Damianov, D. S. (2022). Uncertainty and bubbles in cryptocurrencies: Evidence from newly developed uncertainty indices. SSRN Electronic Journal, Available at SSRN 4301540. https://doi.org/10.2139/ssrn.4301540
- Chowdhury, M. A. F., Meo, M. S., & Aloui, C. (2021). How world uncertainties and global pandemics destabilized food, energy and stock markets? Fresh evidence from quantile-on-quantile regressions. International Review of Financial Analysis, 76, 101759. https://doi.org/10.1016/j.irfa.2021.101759
- Colon, F., Kim, C., Kim, H., & Kim, W. (2021). The effect of political and economic uncertainty on the cryptocurrency market. Finance Research Letters, 39, 101621. https://doi.org/10.1016/j.frl.2020.101621
- Das, D., Dutta, A., Jana, R. K., & Ghosh, I. (2022). The asymmetric impact of oil price uncertainty on emerging market financial stress: A quantile regression approach. International Journal of Finance & Economics, 28(4), 4299–4323. https://doi.org/10.1002/ijfe.2651
- Das, D., & Kannadhasan, M. (2018). Do global factors impact bitcoin prices? Evidence from wavelet approach. Journal of Economic Research, 23(3), 227–264.
- Demir, E., Gozgor, G., Lau, C. K. M., & Vigne, S. A. (2018). Does economic policy uncertainty predict the Bitcoin returns? An empirical investigation. Finance Research Letters, 26, 145–149. https://doi.org/10.1016/j.frl.2018.01.005
- Foglia, M., & Dai, P. F. (2021). “Ubiquitous uncertainties”: spillovers across economic policy uncertainty and cryptocurrency uncertainty indices. Journal of Asian Business and Economic Studies, 29(1), 35–49. https://doi.org/10.1108/JABES-05-2021-0051
- Ghazani, M. M., & Jafari, M. A. (2021). Cryptocurrencies, gold, and WTI crude oil market efficiency: A dynamic analysis based on the adaptive market hypothesis. Financial Innovation, 7(1), 1–26. https://doi.org/10.1186/s40854-021-00246-0
- Hazgui, S., Sebai, S., & Mensi, W. (2021). Dynamic frequency relationships between bitcoin, oil, gold and economic policy uncertainty index. Studies in Economics and Finance, 39(3), 419–443. https://doi.org/10.1108/SEF-05-2021-0165
- Kharif, O. (November. 2022). How a series of crypto meltdowns is reshaping the industry. Retrieved from https://www.bloomberg.com/news/
- Khuntia, S., & Pattanayak, J. K. (2018). Adaptive market hypothesis and evolving predictability of bitcoin. Economics Letters, 167, 26–28. https://doi.org/10.1016/j.econlet.2018.03.005
- Khursheed, A., Naeem, M., Ahmed, S., Mustafa, F., & McMillan, D. (2020). Adaptive market hypothesis: An empirical analysis of time–varying market efficiency of cryptocurrencies. Cogent Economics & Finance, 8(1), 1719574. https://doi.org/10.1080/23322039.2020.1719574
- Koenker, R.(2005). Quantile regression. In Econometric Society Monographs. Cambridge University Press.
- Koenker, R. & Hallock, K. F.(2001). Quantile regression. Journal of Economic Perspectives, 15(4), 143–156.
- Kurihara, Y., & Fukushima, A. (2017). The market efficiency of Bitcoin: A weekly anomaly perspective. Journal of Applied Finance & Banking, 7(3), 57.
- Kyriazis, N. A. (2021). The nexus of sophisticated digital assets with economic policy uncertainty: A survey of empirical findings and an empirical investigation. Sustainability, 13(10), 5383. https://doi.org/10.3390/su13105383
- Lo, A. W. (2004). The adaptive markets hypothesis: Market efficiency from an evolutionary perspective. The Journal of Portfolio Management, 30(5), 15–29. https://doi.org/10.3905/jpm.2004.442611
- Lucey, B. M., Vigne, S. A., Yarovaya, L., & Wang, Y. (2022). The cryptocurrency uncertainty index. Finance Research Letters, 45, 102147. https://doi.org/10.1016/j.frl.2021.102147
- Mokni, K., Ajmi, A. N., Bouri, E., & Vo, X. V. (2020). Economic policy uncertainty and the Bitcoin-US stock nexus. Journal of Multinational Financial Management, 57, 100656. https://doi.org/10.1016/j.mulfin.2020.100656
- Mokni, K., Youssef, M., & Ajmi, A. N. (2022). COVID-19 pandemic and economic policy uncertainty: The first test on the hedging and safe haven properties of cryptocurrencies. Research in International Business and Finance, 60, 101573. https://doi.org/10.1016/j.ribaf.2021.101573
- Mushinada, V. N. C. (2020). Are individual investors irrational or adaptive to market dynamics? Journal of Behavioral and Experimental Finance, 25, 100243. https://doi.org/10.1016/j.jbef.2019.100243
- Neely, C. J., Weller, P. A., & Ulrich, J. M. (2009). The adaptive markets hypothesis: Evidence from the foreign exchange market. Journal of Financial and Quantitative Analysis, 44(2), 467–488. https://doi.org/10.1017/S0022109009090103
- Noda, A. (2021). On the evolution of cryptocurrency market efficiency. Applied Economics Letters, 28(6), 433–439. https://doi.org/10.1080/13504851.2020.1758617
- Owusu Junior, P., Adam, A. M., Tweneboah, G., & McMillan, D. (2020). Connectedness of cryptocurrencies and gold returns: Evidence from frequency-dependent quantile regressions. Cogent Economics & Finance, 8(1), 1804037. https://doi.org/10.1080/23322039.2020.1804037
- Papadamou, S., Kyriazis, N. A., & Tzeremes, P. G. (2021). Non-linear causal linkages of EPU and gold with major cryptocurrencies during bull and bear markets. The North American Journal of Economics & Finance, 56, 101343. https://doi.org/10.1016/j.najef.2020.101343
- Paule-Vianez, J., Prado-Román, C., & Gómez-Martínez, R. (2020). Economic policy uncertainty and Bitcoin. Is Bitcoin a safe-haven asset? European Journal of Management and Business Economics, 29(3), 347–363. https://doi.org/10.1108/EJMBE-07-2019-0116
- Qin, M., Su, C. W., & Tao, R. (2021). BitCoin: A new basket for eggs? Economic Modelling, 94, 896–907. https://doi.org/10.1016/j.econmod.2020.02.031
- Shaikh, I. (2020). Policy uncertainty and Bitcoin returns. Borsa Istanbul Review, 20(3), 257–268. https://doi.org/10.1016/j.bir.2020.02.003
- Sim, N., & Zhou, H. (2015). Oil prices, US stock return, and the dependence between their quantiles. Journal of Banking & Finance, 55, 1–8. https://doi.org/10.1016/j.jbankfin.2015.01.013
- Spithoven, A. (2019). Theory and reality of cryptocurrency governance. Journal of Economic Issues, 53(2), 385–393. https://doi.org/10.1080/00213624.2019.1594518
- Tiwari, A. K., Jana, R. K., Das, D., & Roubaud, D. (2018). Informational efficiency of bitcoin—an extension. Economics Letters, 163, 106–109. https://doi.org/10.1016/j.econlet.2017.12.006
- Troster, V., Shahbaz, M., & Uddin, G. S. (2018). Renewable energy, oil prices, and economic activity: A Granger-causality in quantiles analysis. Energy Economics, 70, 440–452. https://doi.org/10.1016/j.eneco.2018.01.029
- Umar, M., Shahzad, F., Ullah, I., & Fanghua, T. (2023). A comparative analysis of cryptocurrency returns and economic policy uncertainty pre-and post-covid-19. Research in International Business and Finance, 65, 101965. https://doi.org/10.1016/j.ribaf.2023.101965
- Urquhart, A. (2016). The inefficiency of Bitcoin. Economics Letters, 148, 80–82. https://doi.org/10.1016/j.econlet.2016.09.019
- Vidal-Tomás, D., & Ibañez, A. (2018). Semi-strong efficiency of Bitcoin. Finance Research Letters, 27, 259–265. https://doi.org/10.1016/j.frl.2018.03.013
- Vidal-Tomás, D., Ibáñez, A. M., & Farinós, J. E. (2019). Weak efficiency of the cryptocurrency market: A market portfolio approach. Applied Economics Letters, 26(19), 1627–1633. https://doi.org/10.1080/13504851.2019.1591583
- Wang, G. J., Xie, C., Wen, D., & Zhao, L. (2019). When Bitcoin meets economic policy uncertainty (EPU): Measuring risk spillover effect from EPU to Bitcoin. Finance Research Letters, 31. https://doi.org/10.1016/j.frl.2018.12.028
- Wu, S., Tong, M., Yang, Z., & Derbali, A. (2019). Does gold or Bitcoin hedge economic policy uncertainty? Finance Research Letters, 31, 171–178. https://doi.org/10.1016/j.frl.2019.04.001
- Yarovaya, L. Matkovskyy, R. & Jalan, A.(2021). The effects of a “black swan” event (COVID-19) on herding behavior in cryptocurrency markets. Journal of International Financial Markets, Institutions and Money, 75, 101321. https://doi.org/10.1016/j.intfin.2021.101321
- Yen, K. C., & Cheng, H. P. (2021). Economic policy uncertainty and cryptocurrency volatility. Finance Research Letters, 38, 101428. https://doi.org/10.1016/j.frl.2020.101428
- Yousaf, I., & Ali, S. (2020). The COVID-19 outbreak and high frequency information transmission between major cryptocurrencies: Evidence from the VAR-DCC-GARCH approach. Borsa Istanbul Review, 20, S1–S10. https://doi.org/10.1016/j.bir.2020.10.003
- Yousaf, I., Nekhili, R., & Gubareva, M. (2022). Linkages between DeFi assets and conventional currencies: Evidence from the COVID-19 pandemic. International Review of Financial Analysis, 81, 102082. https://doi.org/10.1016/j.irfa.2022.102082
- Yousaf, I., & Yarovaya, L. (2022). Static and dynamic connectedness between NFTs, Defi and other assets: Portfolio implication. Global Finance Journal, 53, 100719. https://doi.org/10.1016/j.gfj.2022.100719