ABSTRACT
Machine learning algorithms have recently emerged as a tool to generate force fields which display accuracies approaching the ones of the ab-initio calculations they are trained on, but are much faster to compute. The enhanced computational speed of machine learning force fields results key for modelling metallic nanoparticles, as their fluxionality and multi-funneled energy landscape needs to be sampled over long time scales. In this review, we first formally introduce the most commonly used machine learning algorithms for force field generation, briefly outlining their structure and properties. We then address the core issue of training database selection, reporting methodologies both already used and yet unused in literature. We finally report and discuss the recent literature regarding machine learning force fields to sample the energy landscape and study the catalytic activity of metallic nanoparticles.
Graphical abstract
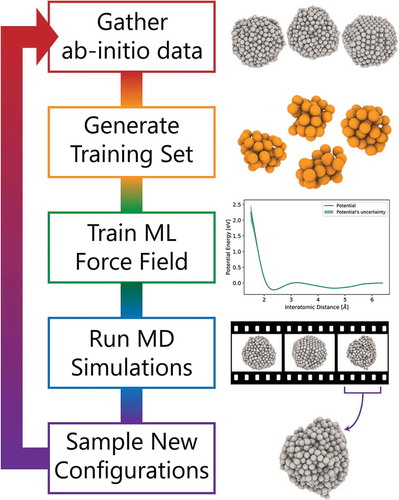
Acknowledgments
CZ and AG acknowledge funding by the Engineering and Physical Sciences Research Council (EPSRC) through the Centre for Doctoral Training Cross-Disciplinary Approaches to Non-Equilibrium Systems (CANES, Grant No. EP/L015854/1) and by the Office of Naval Research Global (ONRG Award No. N62909-15-1-N079). We are grateful to the UK Materials and Molecular Modelling Hub for computational resources, which is partially funded by EPSRC (EP/P020194/1). FB acknowledges financial support from the UK Engineering and Physical Sciences Research Council (EPSRC), under Grants No. EP/GO03146/1 and No. EP/J010812/1. KR acknowledges financial support from EPSRC, Grant No. ER/M506357/1. KR and FB acknowledge the financial support offered by the Royal Society under project number RG120207.
The authors would like to dedicate this review to their mentor, colleague, and friend prof. Alessandro “Sandro” De Vita, passed away too early.
Disclosure statement
No potential conflict of interest was reported by the authors.