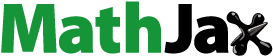
ABSTRACT
Ion channels are fundamental biological devices that act as gates in order to ensure selective ion transport across cellular membranes; their operation constitutes the molecular mechanism through which basic biological functions, such as nerve signal transmission and muscle contraction, are carried out. Here, we review recent results in the field of computational research on ion channels, covering theoretical advances, state-of-the-art simulation approaches, and frontline modeling techniques. We also report on few selected applications of continuum and atomistic methods to characterize the mechanisms of permeation, selectivity, and gating in biological and model channels.
GraphicalAbstract
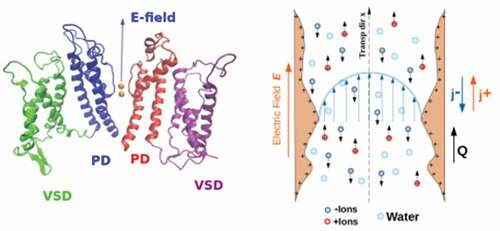
1. Introduction
Ion channels are proteins delimiting pores that allow a regulated flow of water and ions across biological membranes. The first ion channel to be structurally characterized was, in 1998, KcsA, a potassium ion channel [Citation1] (, left panel). Ion channels are involved in key biological functions including control of homeostasis, muscle contraction, and the propagation of nerve signals [Citation2]. The importance of ion channels cannot be overstated when considering that they represent the molecular basis of sensory perception and human thought. Their biomedical relevance is related both to their role in channelopathies [Citation3] and to their role as drug targets for a wide range of diseases.
Figure 1. Left) Crystal structure of KcsA in the closed state (PDB ID: 1J95 [Citation6],); two diagonally opposite monomers are shown for clarity, in cartoon representation. Potassium ions at the binding sites S1-S4 are represented as spheres using their van der Waals radius. Extracellular (EC) and intracellular (IC) boundaries of the lipid bilayer are also indicated. Protein sketch created using VMD [Citation7]. Right) Cartoon illustrating the complex electrokinetic phenomenology occurring in a wide pore (biological or solid-state) under the effect of an external electric field. The walls of the pore can acquire surface charge, the field generates a current () of positive and negative ions. The unbalanced flow of ions, in turn, can result in a net transport of water molecules,
, (electroosmosis). The arrow profile in the middle of the pore, represents the presence of the electroosmotic flow, or any other flow generated, e.g. by external gradients.
![Figure 1. Left) Crystal structure of KcsA in the closed state (PDB ID: 1J95 [Citation6],); two diagonally opposite monomers are shown for clarity, in cartoon representation. Potassium ions at the binding sites S1-S4 are represented as spheres using their van der Waals radius. Extracellular (EC) and intracellular (IC) boundaries of the lipid bilayer are also indicated. Protein sketch created using VMD [Citation7]. Right) Cartoon illustrating the complex electrokinetic phenomenology occurring in a wide pore (biological or solid-state) under the effect of an external electric field. The walls of the pore can acquire surface charge, the field generates a current (j±) of positive and negative ions. The unbalanced flow of ions, in turn, can result in a net transport of water molecules, Q, (electroosmosis). The arrow profile in the middle of the pore, represents the presence of the electroosmotic flow, or any other flow generated, e.g. by external gradients.](/cms/asset/1a5fbdef-1633-483e-87b6-c5f6f91f0420/tapx_a_2080587_f0001_oc.jpg)
Ion channels are characterized by three main features [Citation2]: (i) high permeability, that allows conduction rates close to the free diffusion limit; (ii) high selectivity, that allows discrimination between ions with the same or different charge; (iii) pore gating, that is, the highly regulated opening and closing of the pore in response to specific stimuli. Most research on ion channels focuses on the clarification of the molecular basis of these properties or how they can be modulated by ligands. In this review, we present current theoretical and numerical approaches to address this challenge.
The high conduction rates of ion channels (107–108 ions/second for KcsA [Citation4]) are somehow counterintuitive, given the narrow pore connecting the two sides of the membrane. The high complexity of the permeation mechanism which allows for this extraordinary efficiency is exemplified by the case of voltage-gated potassium channels (see [Citation5]). The Selectivity Filter (SF) of K+ channels is formed by the interface of four subunits where the carbonyl groups of five or six residues point toward the center of the pore defining four binding sites (labeled S1 to S4 from the outermost to the innermost, see , left panel), which can be occupied by potassium ions.
Traditionally the anomalous scattering data from the bacterial K+ channels KcsA from Streptomyces lividans were interpreted [Citation8] as a superposition of ion- and water-occupied states leading to a model of cotranslocations of ions and water. This model was further supported by numerous simulation studies [Citation9–14]. However, this model has more recently been questioned [Citation15,Citation16], and an alternative view has been proposed, with ions passing through the selectivity filter in direct contact with each other without intervening water molecules. This interpretation directly challenged the classical view on permeation: the direct ion–ion interactions had been so far ruled out on account of an excessive electrostatic repulsion. While the issue is far from settled, this example simultaneously highlights the complexity of structural biology and electrophysiology data whose interpretation is far from trivial, and the power of computational approaches, which serve as a bridge between the two, providing molecular-level dynamical information.
Ion channel selectivity is another area of active research. It is again instructive to consider the case of KcsA that conducts K+ and Na+ ions with a ratio 150:1 [Citation17]. Again, this property is surprising since K+ ions are larger than Na+ and selection cannot be explained by simple sieving based on ion size. This pattern was originally explained with a snug fit model [Citation1,Citation18] according to which the channel was potassium-selective because the K+ ion perfectly fitted into the SF, but Na+ was too small to favourably interact with the pore walls. This model, however, became untenable when it was discovered that the SF of this channel was capable of fluctuations larger that the difference in radius between the potassium and sodium ions [Citation19]. The model was thus updated with a more sophisticated one also accounting for the electrostatic repulsion between the carbonyls of the SF [Citation20,Citation21] and limitations on the number and motion of coordinating carbonyls [Citation22–24] as well as the interactions between multiple ions in the pore [Citation25]. The emerging modern view of ion selectivity was reviewed in Ref [Citation26]. In general, the size-exclusion model does not explain the counter-intuitive evidence that often large channels are selective to small ions while small channels are selective to large ions [Citation27]. This pattern emerges as a result of the hydration shell that surrounds the ions. A small ion can cross a large pore keeping its hydration shell almost intact. Conversely, both small and large ions are massively dehydrated when crossing narrow pores but the energy penalty paid by a large ion is normally smaller than that of a small ion that interacts more tightly with the surrounding water. The redistribution of water around an ion crossing a nanopore is thus a topic of great relevance in bio- and nano-technology and can be studied computationally using, for example, Kirkwood approximation [Citation28] (see Section 2.4).
The high selectivity of ion channels is seemingly at odds with their high permeation rates, leading to the so-called conductivity-selectivity paradox. The traditional argument [Citation29] relies on the scheme that, if the channel is selective to a given ion, there should be a strong ion-binding site, with a deep energy well. However, the deeper the well, the slower the release of the ion, which leads to the prediction of small permeation rates in disagreement with experimental data. Many research efforts via theoretical and simulation-based approaches are currently underway to try and reconcile selectivity and permeability of ion channels [Citation4,Citation30,Citation31]. A possible solution to the paradox relies on the presence of two or more neighbouring binding sites [Citation32], or small energy steps that allow an ion to escape the deep energy well [Citation33]. More research efforts are required in order to understand which solution of the paradox applies to each of the different families of ion channels.
Another key characteristics of ion channels is their gating capability. Traditionally [Citation2] ion channels were classified as ligand-gated and voltage-gated, according to whether their opening is triggered by the binding of a ligand or by a change in the membrane potential. Further complex fine-tuning mechanisms of control, including allosteric modulators, can participate in the gating control. Recently, other gating mechanisms have been identified, based on channels sensitivity to pressure or temperature [Citation34–37]. In general, how the information is transmitted to the pore gate and how gating can be influenced by a variety of other subtle factors remains unclear. Furthermore, there is growing interest to identify the physical means by which the pore blocks ion permeation: is steric occlusion the only one? For example, in the concept of hydrophobic gating [Citation38], a pore with hydrophobic lining can be closed by the formation of a bubble that functionally occludes the pore even in the absence of steric block. This mechanism was originally observed in model nanopores [Citation39,Citation40] but it has been recently identified in an increasing number of biological channels [Citation13,Citation41–43]. Physically, it corresponds to a process of evaporation in hydrophobic nanoconfinement [Citation44,Citation45] which gives rise to bubble formation, see Sections 2.5 and 6.6. Hydrophobic gating implies that the analysis of the radius profile is no longer sufficient to classify a channel structure as open or closed. New criteria for the annotation of newly resolved channel structures are called for [Citation46]. Both equilibrium and enhanced sampling simulations are proving to be efficient tools for the study of hydrophobic gating. A case study [Citation47] of hydrophobic gating in artificial nanopores can be found in Section 6.6.
Even a well-established mechanism like voltage-gating is far from being fully understood. While the gating mechanism of domain-swapped K+ channels seems consolidated [Citation48], the gating mechanism of non-domain-swapped channels is still the object of debate. Domain-swapped channels are characterized by a long linker helix (L45) that connects the Voltage Sensor domain (VSD) with the Pore domain (PD). It is currently accepted [Citation48] that the displacement of the sensor helix S4 pushes down the L45 linker that acts as a mechanical lever on the pore helix S6, extending it and closing the pore. In non-domain-swapped channels, however, the linker element L45 is too short to act as a mechanical lever and the gating mechanism is still under investigation. Voltage-gated ion channels have recently been classified as allosteric machines [Citation48] since they are characterized by a long-range coupling between the VSD and PD. This suggested the opportunity to use network theoretical approaches already used in the study of allosteric enzymes [Citation49,Citation50]. These techniques allow one to identify pathways of motion propagation from the VSD to the PD. Recent studies [Citation51,Citation52] applying this methodology identified two main pathways, the former reminiscent of the mechanism operating in domain-swapped channels, and the latter corresponding to a new, non-canonical mechanism important not only for activation/deactivation, but possibly also for inactivation [Citation53]. A case study can be found in Section 6.5.
Ion channels have not only a biomedical relevance but they are also extremely important in nanotechnology and engineering. Currently, it is possible to embed ion channels or other nanopores in natural or artificial bilayers and use them as devices for single-molecule manipulation. One of the most successful applications is the use of -hemolysin for DNA sequencing [Citation54] which is currently commercially exploited. Ion channels have also been engineered to respond to specific stimuli [Citation55–57]. Unfortunately, ion channels are extremely fragile molecules that tend to unfold and lose their properties when placed outside their natural biological environment. This has motivated massive research efforts to develop artificial solid-state nanopores [Citation58,Citation59]. Unfortunately, so far synthetic nanopores are much less performing than their biological counterparts; in particular, they typically lack two distinguishing properties of ion channels, that is, selectivity and gating. The goal of much research in the field is thus to design biomimetic artificial nanopores [Citation60–62].
As an example, a promising material to realise biomimetic nanopores is represented by graphene [Citation63]. The lithography and beam irradiation techniques allow the creation of nanoscale pores in graphene mono-layers, which opens the way to a wide range of applications, from wastewater treatment to desalination [Citation64,Citation65]. In order to use graphene as a molecular sieve, it is necessary to enforce a uniformly small diameter of the pores. Small pores with a diameter up to 5.5 Å are naturally selective due to steric exclusion [Citation66], but their fabrication is still a challenge [Citation67]. Larger pores are easier to manufacture but they are not selective. However, even large nanopores can be made selective through an appropriate functionalization [Citation62]. The quest for the ideal functionalization simultaneously allowing high selectivity and high permeation rates of the selected solute brings back to biological models. In a landmark study [Citation68], Corry and coworkers created graphene nanopores functionalized with four carbonyl groups arranged so as to reproduce the geometry of the SF of the K+ channel KcsA: the synthetic pores indeed were potassium selective. The performance of biological channels, however, does not only depend on the arrangement of the SF groups. Indeed, graphene nanopores with four carboxylate groups, engineered to mimic the SF of the Na+ channel NavAb, resulted in pores not selective to sodium [Citation68]. However, a voltage-tunable ion selectivity could be enforced using only three carboxylate groups.
Computational modelling has always been an integral part of the research on ion channels. For instance, Hodgkin and Huxley [Citation69] described the onset and propagation of the action potential modeling the membrane as a non-linear electric circuit. It is notable that Hodgkin and Huxley, who won the 1963 Nobel Prize in Physiology or Medicine for this work, did not know about the existence of ion channels in the same way as Mendel did not know about the existence of genes when he discovered his laws of transmission and segregation of inherited traits. The discovery of specific proteins acting as pathways for ionic currents across the membranes dates back to the patch clamp studies by Sakmann and Neher [Citation70] who were awarded the 1991 Nobel Prize in Physiology or Medicine. The first structural resolution of an ion channel, however, had to wait until McKinnon (2003 Nobel laureate in Chemistry) determined the X-ray structure of the KcsA channel [Citation1]. We are currently living in a particularly favourable historical period for the computational study of ion channels. On the one hand, cryo-electron microscopy is providing a large number of high-resolution structures of ion channels [Citation71]. On the other hand, hardware improvements are providing the scientific community with increasing computing power [Citation72]: as of November 2021 the computing speed of Fugaku, the fastest supercomputer, is ca. 442 petaflops [Citation73], that is, more than 1017 floating point operations per second. Finally, researchers can now rely on a rich toolbox of advanced computing techniques that are the topic of this review together with theoretical advancements in the field.
Notwithstanding the recent advancements, the characterization of ion channels still remains a theoretical and computational challenge; accordingly, the choice of the approach must be performed with care based on the task at hand. The calculation of electrostatic potential profiles or ion flows highly benefits from continuum approaches where protein, water, and membrane are modeled as continuous dielectric media while ions are described by a continuous density distribution. This approach, traditionally relying on the Poisson-Boltzmann equation [Citation74] and on Poisson-Nernst-Planck (PNP) equation [Citation75], is computationally convenient and extremely flexible. Indeed, combination of PNP equation with the Navier-Stokes equation allows to include hydrodynamic effects yielding the system of electrokinetic equations [Citation76] presented in Section 2. The continuum approach, however, does not account for fluctuations, ion correlations, polarization effects, and finite-size effects. When these effects become relevant, atomistic approaches are the tool of choice, see Section 6. In particular, Molecular Dynamics (MD) simulations can be considered as a computational microscope [Citation77] with a time resolution of the order of the femto-second and a space resolution of the order of the hydrogen atom. The main limitation of MD is that, due to the constraint to use a femto-second time-scale, the simulations are normally limited to a few hundreds of nanoseconds (although the growing use of GPUs [Citation78] or dedicated hardware like the Anton supercomputer [Citation79] allow for longer simulations). This limitation is particularly serious because many phenomena of interest in biology can be classified as rare events [Citation80], see Section 4.2. Rare events are processes associated with the overcoming of an energy barrier higher than the thermal energy . As a result, the system lingers for a very long time in the pre-barrier minimum before a fluctuation endows it with sufficient kinetic energy to overcome the barrier. Rare events are thus characterized by long waiting times, but when they finally occur, the barrier crossing is very rapid and the sampling of the region near the barrier is very poor.
In order to overcome this limitation, one is forced to develop tools that enhance the sampling of MD simulations or bias them in a controllable way (see section 4.2), to focus on unlikely and yet important regions of the free-energy landscape. Methods like Replica Exchange Molecular Dynamics [Citation81] do this by overcoming free-energy barriers thermally through exchanges with high-temperature replica simulations. These can suffer, however, from needing a very large number of replica simulations. Alternatively, biases can be applied to an appropriate set of collective variables, or CVs, to characterize those regions and to define the corresponding free-energy landscape. Choosing appropriate CVs, is however, a non-trivial task. A clever approach is to create hybrid algorithms where the strengths of Replica Exchange and CV-based algorithms (e.g. metadynamics) can be combined [Citation82,Citation83]. As an alternative, if the goal is not the reconstruction of the whole free-energy landscape but just the calculation of the free energy profile along the transition of interest, it is possible to use methods like Transition Path Sampling [Citation84] or the String Method [Citation85]. Finally, Machine Learning is disclosing the opportunity to train a deep neural network to automatically choose good CVs [Citation86], for instance, those along the direction of maximal auto-correlation [Citation87,Citation88], see Section 7.
This Review ensues from the talks and the scientific discussions held in February 2021 at the conference ‘Frontiers in ion channels and nanopores: theory, experiments, and simulation’ [Citation89]; while the overview of the approaches attempts to be general, the selection of applications reflects its origin. The Review has the following main organization. Section 2 is devoted to continuum methods and Section 3 to their applications; these Sections introduce a general physical framework, which is useful to understand the fundamental transport phenomena occurring in ion channels but also in synthetic nanopores; while the continuum approach is highly succinct and informative, it requires some formalism to be introduced. We propose a number of selected applications referring to the use of electrokinetic equations, the methods to compute hydration patterns, and the use of density functional theory. Section 4 of the review covers atomistic simulation approaches of ion channels which provide a detailed picture of the same phenomena and whose treatment benefits from a less formal introduction: after an overview, we introduce the different families of methods for the study of rare events and we review a hybrid continuum/atomistic approach: Brownian Dynamics. In Section 6, we discuss few selected applications of atomistic methods to characterize selectivity, permeation, and gating in biological and model channels. In Section 7, we discuss a number of forefront techniques that are still in a development stage but have a potential to revolutionize the field. In particular, we focused on polarizable force fields, hybrid enhanced sampling algorithms, multi-resolution approaches, and machine learning. In the final section, we draw the conclusions of the work.
2. Continuum methods
The transport of solutes, including ions, across narrow pores is not only important for ion channel research, but is also at the core of many nanotechnology applications in the field of nanopore sensing [Citation90], nanofiltration [Citation91], and nanoporous energy materials [Citation92]. Two main computational approaches are useful to address this general problem: atomistic/coarse-grained simulations and continuum methods. While the former provides a detailed (atomistic) or approximated (coarse-grained) description of chemical interactions occurring at relatively short-time scales, continuum theories, which neglect the granular nature of matter, allow to explore transport mechanisms on longer time scales, moreover yielding, in some cases, analytical estimates of the relevant observables characterising transport. In the following, we provide a rather general continuum framework to study the said transport phenomena and we specialise them for ion channel research.
2.1. Electrokinetic equations
In the continuum approach, the complexity of the confined electrolyte transport within artificial or biological nanopores, such as that in , right panel, is drastically reduced by using local densities and fields associated to each component of the system shown in , left panel. For instance, the solvent (e.g. water) is generally treated as a homogeneous medium with a constant electric permittivity , the ion species are described by their local concentrations, and channel walls contribute with a surface charge density. Within this framework, the transport of ions is determined by electrokinetic equations [Citation93,Citation94], that for systems with axial symmetry, read [Citation94]
EquationEquation (1a)(1a)
(1a) is the continuity equation specifying that the variation of the density,
, of positive/negative ions in a volume is related to their fluxes across the surface of that volume. EquationEquation (1b)
(1b)
(1b) is the Navier-Stokes equation governing the evolution of the velocity profile of a solution of density
and viscosity
, driven by external forces
and, possibly, pressure gradients
. Finally, EquationEquation (1c)
(1c)
(1c) defines the incompressibility condition for the solution, an approximation usually well satisfied by common salt solutions. The physical nature of the ion transport is assigned by specifying the constitutive equation of the ion fluxes in EquationEquation (1a)
(1a)
(1a) . A traditional approach employs the so-called Nernst-Planck current
where is the coordinate along the longitudinal axis of the channel (transport direction) and
indicates the radial coordinate, see , right panel. EquationEquation (2)
(2)
(2) expresses the ion currents
in terms of advection by the velocity
, diffusion, and electromigration due to the gradient of the electrochemical potential, which includes ion concentration and charge effects
where is the diffusivity of the ions,
denotes their valence,
is the elementary charge, and
, with
the Boltzmann constant and
the absolute temperature. The electrostatic potential
in the channel is obtained by solving the Poisson equation
relating to the concentrations
of two symmetric ion species;
is the permittivity of the solvent taken as an homogeneous medium. The typical size of ion channels leads to vanishing small values of the Reynolds numberFootnote1 and hence the Navier-Stokes EquationEquation (1b)
(1b)
(1b) reduces to the (steady-state) Stokes equation:
EquationEquation (4)(4)
(4) and (Equation5
(5)
(5) ) are subject to standard channel impermeability and no-slip boundary conditions for the flow (
at the channel walls) as well as
or
at the walls, which account for the dielectric (with surface charge density
) or conductive (with
potential) nature of the walls [Citation76], respectively, with
the normal to the wall surface.
Analytical insight into the steady-state solution of EquationEquation (1a)(1a)
(1a) and (Equation4
(4)
(4) ) can be obtained by assuming that, along the radial direction, the charge densities attain their equilibrium profile
and by linearising the charge density [Citation76]
where is the inverse of the Debye length
which measures the length scale at which the re-distribution of mobile ions screens an electric field, with the bulk concentration of the salt. In the case of smoothly-varying channel profile, a linear-response theory can be applied. In this regime, the total pressure varies solely along the pore axis, such that its gradient is
, where
is the
component of the geometrically induced local pressure gradient which is determined by the boundary conditions and by fluid incompressibility, while
indicates the pressure drop across the channel length
. Moreover, within such a regime,
is the electrostatic driving force.
Within the linear response approach, the fluxes are proportional to the generalized forces and the transport coefficient can be derived by the relaxation of equilibrium fluctuations, see Ref [Citation94]. Within these approximations, the transport along the channel axis is captured by the following fluxes
which can be induced by different forcing. Clearly, an electric current can be generated by an external electric field while a solvent current
by a pressure drop. However, also cross phenomena may appear where, e.g. an electric current can be prompted by applying a pressure drop on the solvent. In order to capture these non-trivial couplings it is insightful to write down the linear system of equations governing the relevant currents in the following way:
where are the coefficients of the Onsager matrix [Citation95] associated with the drops across the channel of electrostatic potential
, chemical potential
, and pressure
and where we have introduced
in order to make the matrix symmetric. The symmetry of the Onsager matrix, that relies on the microscopic time reversibility of the underlying Hamiltonian dynamics [Citation94], has a crucial practical outcome: the cross-terms, such as
, can be computed in two ways: either measuring
upon applying a chemical potential drop
or by measuring the excess solute flow
upon applying an electrostatic potential,
. This approach is convenient in numerical simulations where applying chemical potential gradients requires to simulate large reservoirs (hence raising the computational time) whereas electrostatic forcing can be simulated with (computationally more convenient) periodic boundary conditions. Finally, we remark that
in EquationEquation (12)
(12)
(12) is due to the Debye-Hückel assumption [Citation76] and that one expects
for the case of the fully non-linear Poisson-Boltzmann equation.
In the case of smoothly varying channels, it is also possible to derive closed formulas for the coefficients elements of the Onsager matrix. Interestingly, the transport coefficients are particularly sensitive to the geometry and the conductive properties of the channel walls when the Debye length is comparable to the channel width. In this regime, one pair of off-diagonal Onsager matrix elements increases with the corrugation of the channel transport, in contrast to all other elements, which are either unaffected by or decrease with increasing corrugation [Citation76].
2.2. Poisson-Nernst-Planck equations
A usual scenario is the one in which the flow of solvent is absent, . Indeed, in such a regime the (Navier-)Stokes equations are trivially fulfilled and Equation (1) simplify much reducing to the so-called Poisson-Nernst-Planck electrodiffusion equation in three-dimensional space (3d-PNP) governing the motion of positive and negative ions [Citation96–98]:
where is the solution of the Poisson EquationEquation (4)
(4)
(4) with the Boltzmann assumption, EquationEquation (6)
(6)
(6) . At first, a one-dimensional reduced model was used to describe ion permeation (1d-PNP) [Citation99], but eventually, treatments based on the full three-dimensional (3d-PNP) theory became possible [Citation96,Citation97]. In the absence of ion flux, the 3d-PNP theory reduces to the standard non-linear equilibrium Poisson-Boltzmann equation. As mentioned in the previous section, the 3d-PNP equation is based on a mean-field approximation that overlooks non-electrostatic ion–ion interactions (e.g. excluded volume) as well as polarization and ion correlation effects. This is why this equation is not well suited to treat highly charged molecules (like DNA) at high ion concentration or single file diffusion along a narrow pore. However, due to the low charge density of membrane systems, the PB equation is often used to compute the electrostatic potential profile along the pore of ion channels. The equation, allowing the comparison of relative stabilities of protonated and unprotonated residues, is also used for pKa calculations.
In practice, PNP is based on several simplifications: rigid channel structure, structureless dielectric solvent, and mean-field ion–ion interactions, which are of unknown validity in the context in which they are used. If one is to adopt a continuum electrodiffusion approach, such simplifications are necessary in order to have partial differential equations that can be solved numerically. The accuracy of the 3d-PNP approach for ion channels was discussed in a few studies [Citation97,Citation100,Citation101], where 3d-PNP simulations were contrasted with Brownian dynamics. Results showed that 3d-PNP approach can predict the reversal potential for wide pores, but breaks down in narrow ion channels with radii smaller than the Debye length. Further extension of 3d-PNP to finite ion sizes [Citation102], multiple ion species [Citation103], and including dielectric repulsion terms [Citation104] have been proposed, as well as a combination of one-dimensional (1d) PNP with classical density functional theory (see Section 2.5) [Citation105]. However, depending on the situation, 3d-PNP may, or may not, be sufficiently accurate. Ultimately, the significance of that picture should not be expected to exceed that of the physical approximations upon which it is built.
2.3. Capture process of suspended particles
In many biologically and technologically relevant applications, such as drug delivery of molecular therapies, nanopore sensing, testing the impact of anesthetics on ion channels, etc., it is fundamental to characterize the capture of small and large molecules (analytes) from their bulk solution to narrow paths (channels and nanopores). An efficient capture, for instance, is crucial to obtain a correct behaviour of nanopore-sensing devices [Citation106,Citation107] which work detecting the ion-current variation produced by the passage of analytes into channels [Citation108–111]. In this case, Equation (1) should be complemented with the equations governing the evolution of the density of analytes. Clearly, the density of analytes is coupled to that of the electrolyte and of the solute, hence the Onsager matrix will become a matrix including novel diagonal and cross terms.
If the analytes are highly diluted and weakly charged (or highly screened by the salt solution), their presence is assumed to mildly affect the electrostatics and the velocity profile, such that, they can be modelled as passive tracers. Accordingly, Equation (1) are solved and then the velocity profile and the electrostatic potential are used as external fields for the evolution of the density of analytes.
In the context of capture mechanism, Equation (1) are crucial to characterize and control the main electrokinetic effects that are known to drive the analytes to the capture regions [Citation112–114]: electrophoresis (EP), dielectrophoresis (DEP), and electroosmosis (EO). EP is the motion of charged molecules relative to a solvent induced by a uniform electric field. DEP is the transport of neutral particles carrying a permanent or induced dipole in inhomogeneous electric fields, in which dipoles not only undergo a torque, but also a translational force. EO is the flow of an electroneutral solvent (e.g. water) through a membrane triggered by the motion of ions in electric fields; this flow can drag even large macromolecules [Citation111]. The deep entanglement and competition of these three effects can also be seen from the structure of the Onsager matrix in Equation (14).
The continuum approach so far described allows deriving the dependence of the capture rates on the above effects. In the presence of an external electric field, the capture problem can be formulated in terms of a transport equation [Citation115] for a diluted analyte concentration that is a function of the distance
from the center of the nanochannel entrance
where the molecule flux , pointing to the pore, has three components: i) diffusive, ii) advective due to the solvent motion,
, for example, induced by electroosmosis, and iii) phoretic due to external fields.
denotes the molecule diffusivity. As compared to Equation (1), the presence of electrolyte solution manifests only through an EO flux and the dielectric constant.
It is important to notice that Equation (1) focus directly on the dynamics of the electrolytes and of the solution while in EquationEquation (14a)(14a)
(14a) -(Equation14b
(14b)
(14b) ) the electrolyte solution is approximated just as a background fluid, with an assigned dielectric constant, where macromolecules are transported by external fields and flows. This justifies the absence of Poisson and Navier-Stokes equations.
According to the hemi-spherical electrode approximation [Citation116], the external voltage generates, far from the electrodes, a radial electric field of modulus directed towards the pore center.
is the ion current due to the applied voltage. Thus, the problem EquationEquation (14a)
(14a)
(14a) -(Equation14b
(14b)
(14b) ) acquires a radial symmetry with respect to the center of the pore entrance (origin).
The stationary solution of EquationEquation. (14a)
(14a)
(14a) -(Equation14b
(14b)
(14b) ) in the shell between
(entrance radius) and
is determined by the boundary conditions,
, meaning that the molecule concentration is constant far from the channel, and
, indicating that the channel entrance is partially absorbing, that is, only a fraction of the incoming molecules actually enter.
Once is known, the flux density
can be easily derived via EquationEquation (14b)
(14b)
(14b) and the resulting capture frequency
(number of molecules adsorbed per unit time) is nothing but
,
where is the dimensionless effective potential, which accounts for the radial EP and DEP effects as well as for the contribution of the EO flow [Citation115]. EquationEquation (15)
(15)
(15) , written in the form
, shows that the capture frequency splits in two contributions,
, accounting for the approach to the pore, and
, associated with the molecules actually entering it. The entrance frequency reads
, and depends on the parameter
, whose precise determination requires an atomistic description of the system. Nevertheless, in the same approximation of EquationEquation (14a)
(14a)
(14a) -(Equation14b
(14b)
(14b) ), we can attempt an Eyring equation for the rate
where is the transmission coefficient and
the Planck constant.
indicates the free-energy barrier, generally positive, which molecules need to cross to enter the pore, see Section 4 for methods to compute free-energy barriers of ion permeation and Section 6 for examples of atomistic calculations of free-energy barriers. High values of
reduce exponentially the adsorption rate.
The simple expression (15) shows how continuum approaches are useful to derive estimates of the capture frequency that can be used to establish the order of magnitude of the capture rates and their dependence on the experimental conditions. Moreover, the Smoluchowski theory allows an immediate assessment of the competition among electroosmosis and various phoretic contributions involved in experiments [Citation117]. Moreover, the approach allows to determine the Mean First Passage time [Citation118] and the permeability [Citation119] (see Section 3.2) in corrugated channels under the action of a chemical potential difference at the channel ends.
2.4. Kirkwood approximation: ionic hydration patterns in nanopores
Transport through nanopores is affected not only by ion-pore and ion–ion interactions, but also by the hydration barrier originating from the re-distribution of the water molecules when an ion traverses the nanopore. In addition, water distributions are also necessary to assess water-mediated ion-pore interactions. To address this issue Barabash et al. [Citation28] extended an approach designed to characterize the DNA hydration [Citation120,Citation121] to analytically predict the ionic hydration patterns inside nanopores, using the radial distribution functions (RDFs) evaluated in the bulk electrolyte. Using a rigorous statistical mechanical correspondence between the potential of mean force (PMF) and RDFs, the density of water-oxygen atoms can be rewritten via the multi-particle distribution functions [Citation122]. By truncating the PMF decomposition at the 2-particle terms, one arrives at the Kirkwood approximation [Citation123] involving only a product of the RDFs
Here, is the position of interest,
are the coordinates of the fixed atoms of the nanopore (
in total),
is the location of the ion,
indicates the bulk water density,
and
are the ion-oxygen and nanopore-oxygen free bulk RDFs, respectively.
EquationEquation (17)(17)
(17) shows that the complex water patterns originate from the interference between the ionic hydration shells (
) and the hydration cloud around the nanopore (
). Since the method requires the bulk RDFs
to be measured only once under given system structure and composition, a 102–104 speedup is achieved as compared to enhanced sampling all-atom MD simulations [Citation28,Citation120]. The truncation of the decomposition to 2-particle terms overlooks the interactions between the components of individual water molecules and their orientations, leading to overestimated densities near the pore walls. Nevertheless, the proposed analytical description generally agrees well with the results of molecular dynamics simulations for K+, Na+, and Cl – ions (see ) and predicts the locations of the trapped water molecules [Citation28]. Hence, the method provides fundamental insights into the electrostatics, the dielectric properties, and the dynamics of ions in nanopores. A tentative analytical way of connecting the hydration patterns and the single-ion equilibrium PMF is proposed as well [Citation28].
Figure 2. Three-dimensional ionic hydration patterns. Water distribution around a K+ ion (purple sphere) at 0.4 nm above the pore in a graphene lattice (black ball-and-stick representation), obtained from (a) MD simulations and (b) theory, EquationEquation (17)(17)
(17) . The layered structure of the hydration around the ion and the graphene lattice emerges due to the chosen isovalue of 1.15. A 5-point smoothing window has been applied to the original data in both panels. Reproduced without changes from Ref [Citation28]. under the Creative Commons CC BY 4.0 license.
![Figure 2. Three-dimensional ionic hydration patterns. Water distribution around a K+ ion (purple sphere) at 0.4 nm above the pore in a graphene lattice (black ball-and-stick representation), obtained from (a) MD simulations and (b) theory, EquationEquation (17)(17) ρw(r)ρw,0=giw(|r−ri|)∏j=1Npgpw(|r−rjp|).(17) . The layered structure of the hydration around the ion and the graphene lattice emerges due to the chosen isovalue of 1.15. A 5-point smoothing window has been applied to the original data in both panels. Reproduced without changes from Ref [Citation28]. under the Creative Commons CC BY 4.0 license.](/cms/asset/8a18d4c7-8ff1-4ad0-9c6e-a58c7456e6c3/tapx_a_2080587_f0002_oc.jpg)
Moreover, the approach allows to study the effects on the hydration patterns imposed by external strain (stretching, skewing, bending, twisting) and chemical features (pore isomers, type and charge of the rim atoms, numbers of lattice layers, layer offset eclipse). Such flexibility should prove useful in designing the hydration pattern via the multi-parameter optimisation leading to pre-defined properties of the nanopores.
2.5. Classical density functional theory and ion channels
Classical density functional theory (C-DFT) [Citation124] is a powerful approach, in the grand canonical ensemble, that allows, through a variational principle, to compute the structure and the energetics of a system in equilibrium and the evolution of the density distribution for systems out of equilibrium [Citation125–127]. The starting point for C-DFT is the functional of the grand potential that, for a one-component system, takes the form
where is the intrinsic Helmholtz free-energy functional, which can be split into an exactly known ideal gas part
and an excess (over the ideal gas) contribution
, which contains all the information about the particle–particle interactions [Citation124]. In EquationEquation (18)
(18)
(18) ,
is the external and
the chemical potential. For practically all systems of interest,
is unfortunately known only approximately. The equilibrium density profile
can be calculated from the fact that
is minimal in equilibrium [Citation124], which can be expressed as
Once the equilibrium density profile is known, the grand potential of the system follows immediately
In the context of ion channels, C-DFT can be viewed as complementary to molecular dynamics, since it provides configurations that are averaged over the grand-ensemble rather than single frames. This can be important when system states are characterized by rare events or large fluctuations. C-DFT allows the exploration of larger time scales than those typically accessible to MD, even if the implementation of force fields is less obvious.
There are several studies of ion channels within the framework of C-DFT so far, related to gating, selectivity, and ion transport. In gating, the relationship between the gate configuration and the probability of a bubble formation was studied in Refs. [Citation44,Citation128]. The gate configuration was accounted for by the external potential and the bubble formation followed from the density profile
of a water-like simple fluid. In order to illustrate the application of C-DFT to gating in an ion channel, we show in ) the simplified geometry of a channel and its change from a closed configuration in (a) to an open one in (c). This geometrical change of the channel geometry is employed as an external potential
in the DFT calculation. A cut through the resulting density profiles of a closed and an open channel is also shown in (a) and (c) [Citation128]. While in the open configuration the water density in the channel remains liquid-like (c), a clear bubble is formed in the narrow region of the closed configuration (a). In addition to the structure, one obtains information about the energetics of the channel. In this application, we used
, the radius of the gate, as a control parameter to describe the geometrical configuration. For each value of
, we can ask, on the one hand, what the structure of the equilibrium configuration of water in the channel or the gate is. On the other hand, we can ask what is the structure of an open and of a closed configuration for a given value of
, that is, a density distribution with a liquid-like density, say
, and one with a bubble in the gate, say
. Once these density profiles are known, it is straightforward to compute the corresponding grand potentials
and
and their difference
. If
then the open state of the gate is the equilibrium configuration and the closed one is meta-stable and vice versa. Assuming a two-state system with probability
for being in the open state and
for being in the closed state, we can easily calculate from
the probability of finding the channel open:
Figure 3. (a) The geometry of a simple model channel, that enters a C-DFT calculation as external potential and a cut through the corresponding equilibrium density profile of a closed configuration of the gate, that is blocked by a bubble. (b) The probability of finding the channel open as function of the gate radius . (c) The geometry and the corresponding equilibrium density profile of an open channel that is filled with a liquid-like water density. Reprinted (abstract/excerpt/figure) with permission from [Citation128]. Copyright 2017 by the American Physical Society.
![Figure 3. (a) The geometry of a simple model channel, that enters a C-DFT calculation as external potential and a cut through the corresponding equilibrium density profile of a closed configuration of the gate, that is blocked by a bubble. (b) The probability of finding the channel open as function of the gate radius R2. (c) The geometry and the corresponding equilibrium density profile of an open channel that is filled with a liquid-like water density. Reprinted (abstract/excerpt/figure) with permission from [Citation128]. Copyright 2017 by the American Physical Society.](/cms/asset/716ecf07-5e4e-4128-b418-57819706d73d/tapx_a_2080587_f0003_oc.jpg)
The shape of this probability as function of is shown in ).
Here, the accurate knowledge of the state point of water in relation to the bulk-phase diagram has proven beneficial to study the formation of a bubble and the probability of opening and closing of the gate. The influence of hydrophobic gases and hydrostatic pressure was studied due to the relatively small computational costs [Citation44]. Within this framework, a more complete model of an ion channel was formed that included voltage sensors and a hydrophobic gate [Citation129].
Selectivity can be driven by specific (chemical) interactions or by physical mechanisms, such as a competition between the electrostatic interactions and entropy. In the latter case, C-DFT can help to understand the physics involved in selectivity by providing structure and energetics of the process [Citation130–132]. Coupling classical dynamical DFT (C-DDFT)[Citation125,Citation127] and the electrochemical gradient across a membrane allows one to study the characteristics of ion flux through channels [Citation105] adding microscopic details to the more macroscopic approaches described in the previous sections.
3. Continuum approaches: applications
Continuum methods, despite their limitations, lend themselves to a variety of applications owing to their flexibility. A complete survey of the possible applications to ion channels is impossible within the extent of this review, thus we will limit the discussion to a few selected examples without any claim to be exhaustive.
3.1. Capture models
The continuum model for capture (EquationEquation (14a)(14a)
(14a) -(Equation14b
(14b)
(14b) )) can be used to interpret some experiments in synthetic nanopores, for example, those of Larkin et al. [Citation117] in which two globular proteins, ProK and RNase A, are captured by a solid-state hafnium dioxide (HfO2) pore immersed in a 1 M KCl solution at pH 8.1 at which the HfO2 pore is slightly negatively charged. The surface charge induces an EO flow, which superimposes to the EP of the external field. EquationEquation (15)
(15)
(15) can be used to estimate the capture frequency of the two proteins in a wide range of voltages.
, left panel shows the capture frequency when the entrance contribution was neglected, i.e. and
, for protein concentration
nM. The points refer to EquationEquation (15)
(15)
(15) that includes EO, EP, and DEP, whereas lines indicate the case in which DEP is set to zero. The small displacement of symbols with respect to the solid lines suggests that, in the experiment, dielectrophoresis is negligible. Moreover, as the pore is negatively charged, for positive
, EO is directed towards the pore so that it cooperates with EP. Theoretical capture frequencies are 4–5 times smaller than the ones observed in the experiments (see of Ref [Citation117]), which is remarkable given that no fitting parameters were introduced.
Figure 4. Left) Capture frequency for the experiment of Larkin et al. [Citation117]. Circles and squares refer to the theoretical capture frequency calculated via EquationEquation (15)(15)
(15) for RNase A and Protein K. Lines refer to the capture frequency when DEP is neglected. In both cases, protein bulk concentration is
nM and complete adsorption is assumed (
= 0 and
, transport limited regime). The pore is slightly negatively charged at the experimental pH = 8.1 while both proteins are positive, i.e. EO and EP cooperate at
. Protein sketches are created using VMD [Citation7]; blue and red colors identify to positively and negatively charged residues, respectively. Right) Experimentally observed capture frequency
, black points. The V-shaped plot indicates that capture occurs at both positive and negative voltages. The dashed line refers to the theoretical capture frequency estimated via EquationEquation (15)
(15)
(15) with
in the Arrhenius formula for the absorbing rate
(see text), reproducing the typical V-shaped behaviour. Figures are adapted with permission from [Citation115]. Copyright 2020 American Chemical Society.
![Figure 4. Left) Capture frequency for the experiment of Larkin et al. [Citation117]. Circles and squares refer to the theoretical capture frequency calculated via EquationEquation (15)(15) ν=2πρp,∞DDkre2eϕ(re)+∫re∞dρeϕ(ρ)ρ(15) for RNase A and Protein K. Lines refer to the capture frequency when DEP is neglected. In both cases, protein bulk concentration is ρp,∞=1 nM and complete adsorption is assumed (τe = 0 and ν=νa, transport limited regime). The pore is slightly negatively charged at the experimental pH = 8.1 while both proteins are positive, i.e. EO and EP cooperate at ΔV>0. Protein sketches are created using VMD [Citation7]; blue and red colors identify to positively and negatively charged residues, respectively. Right) Experimentally observed capture frequency f, black points. The V-shaped plot indicates that capture occurs at both positive and negative voltages. The dashed line refers to the theoretical capture frequency estimated via EquationEquation (15)(15) ν=2πρp,∞DDkre2eϕ(re)+∫re∞dρeϕ(ρ)ρ(15) with ΔF0=22kBT in the Arrhenius formula for the absorbing rate k (see text), reproducing the typical V-shaped behaviour. Figures are adapted with permission from [Citation115]. Copyright 2020 American Chemical Society.](/cms/asset/f400b892-5368-41fe-bba3-5ea37209fbe9/tapx_a_2080587_f0004_oc.jpg)
The analytic capture model, based on the continuum equations of Section 2.3, developed by Chinappi et al. [Citation115] also allows the interpretation of an experiment of DEP capture into an hemolysin channel (aHL) of a -hairpin engineered to carry a permanent dipole, while remaining electrically neutral at pH 7. In practice, the peptide is a dumbbell of length
nm, with zero global charge, and dipole
nm. , right panel shows the capture frequency into the aHL obtained from the ion current traces, highlighting that capture occurs independently of the voltage polarity. The figure shows that the experimental data are in agreement with the predictions of DEP capture model.
3.2. Channel permeability
Another application of the presented continuum theory, in particular, of Smoluchowski equation, deals with the computation of channel permeability when local inhomogeneity and chemical heterogeneity of the channel makes the assumptions of constant diffusion coefficient not viable. This is the typical case for ion channels. The passive transport of ions/molecules through a pore in the limit of dilute molecule concentration (as in the previous discussion) can be written in the simplest form as the Fick’s law (see Section 2.1 and Ref [Citation94].):
where is either the ion or molecule density and
is the permeability coefficient containing all geometrical and chemical channel properties but the dependence from the concentration. An expression for the permeability coefficient can be derived starting again from the Smoluchowski diffusion-drift equation with respect to the axial transport coordinate, the position of the particle along the pore axis
, and the Kramers relations [Citation119,Citation133]
where is the length of the pore,
the local diffusion constant,
the PMF characterizing the ion/molecule effective interactions with the pore. This expression is based on the assumption that the permanent ions/molecules crossing the membrane are uncorrelated and that there is no saturation of the occupancy of the pore. This assumption is reasonable at low concentrations, but may be violated if the pore is narrow and there are high-affinity binding sites along the pore.
EquationEquation (23)(23)
(23) shows two important features of transport inside a nanopore: its non-locality, due to the presence of an integral over the pore length
, and its statistical nature, due to the presence of the potential of mean force
. This means that any local method, such as docking, would predict permeation only in a few limited cases, such as a single binding site. Furthermore, one needs to thermodynamically average all other variables for a correct estimation of the
profile. Reliable methods to evaluate
to be used in EquationEquation (23)
(23)
(23) are based on advanced sampling techniques combined with classical molecular dynamics (see Section 4.2). EquationEquation (23)
(23)
(23) is valid only at dilute concentrations or far from saturation, presumably with the pore occupied by a single independent particle. In case of saturation conditions, there are additional methods that can be applied to correctly estimate the flux, such as the Markov state model [Citation134,Citation135].
While for ions diffusing through specific channels the approximations of EquationEquation (23)(23)
(23) are quite severe due to strong correlations among ions and multiple occupancy, the method works well for small molecules diffusing through channels (weak molecule–pore interactions, single occupancy). Electrophysiology data provided a good test for EquationEquation (23)
(23)
(23) in the case of a charged particle, the beta-lactam inhibitor avibactam. Its permeability through the general channel OmpF was measured with the reversal potential method and compared with that calculated via EquationEquation (23)
(23)
(23) . Metadynamics was used to quantify
, and the agreement was remarkable [Citation136].
3.3. Hydration patterns
The analytical approach to characterise the hydration patterns in nanopores [Citation28] and based on EquationEquation (17)(17)
(17) opens an avenue for a number of potential applications. For example, one can hypothesise that the ionic transport rate and the permeation pathway can be tailored by iteratively choosing the pore geometry and the level of externally applied strain. First, such engineering of the selective and conductive properties appears of high demand in water desalination technologies, where the maximisation of the output water quality and energy efficiency have to meet the environmental sustainability criteria [Citation137]. Secondly, the maximised energy-yield during blue energy harvesting has to exceed 5 Watt/m2 to be economically viable while minimising Joule heating [Citation138,Citation139]. Thirdly, next-generation fast DNA sequencing would benefit by applying the same logic of optimisation to slow down and guide the DNA during its translocation through a nanopore [Citation140,Citation141]. In that regard, the classical Coulter-principle-based nucleobase detection could be improved by maximising the current blockage optimising the geometrical features of the nanopore. Fourthly, the Materials Genome Initiative would benefit from cataloguing 2D and 3D nanopore isomers [Citation142]. Finally, transverse (corrugated) waves, travelling along a carbon nanotube (CNT), would invoke the ionic motion against the applied electrochemical gradient, thus realising a nanoscale ionic pump [Citation143]. Thus, the method supports the design and optimization of controllable nanoionic devices with on-demand selective and conductive properties, finding its applications in biophysics, industry, and nanotechnology.
4. Atomistic approaches
4.1. Overview of the methods
Intrinsic to the continuum approaches introduced in Section 2 is the mean field approximation, which neglects all fluctuations and correlations which might be relevant at the scale of ion channels. In particular, ion–ion correlations beyond the mean-field approach of Poisson-Boltzmann (see Section 2.1) are particularly important in narrow channels: a notable example is the selectivity filter of potassium channels discussed in the Introduction. Although, in principle, the geometrically and chemically complex molecular structure of proteins may be accounted for in a continuum model, it is generally preferred to directly resort to particle-based approaches, which more naturally encompass both fluctuations and the atomic structure. The modelling at the atomistic level of ion channels is the most refined available description, providing all the biochemical details necessary to understand complex phenomena such as gating [Citation144], hydrophobic control [Citation145], effect of mutations [Citation146], protein–membrane interaction [Citation147], and the pivotal role of water molecules through H-bonds network formation and local dielectric screening [Citation145].
Atomistic methods explicitly including all atoms and all inter-atomic interactions allow a spatial resolution of a single hydrogen atom and the time-resolution of a single femto-second, so that Molecular Dynamics (MD) simulations [Citation148] can be considered as an atomic resolution microscope [Citation77,Citation149,Citation150]. Of particular interest is the capability of MD to bridge structural information on ion channels with their dynamics and, with some challenges, to their function, with spatial and temporal resolution which is yet unmatched in experimental approaches [Citation151]. In the following, we describe the conceptual framework of MD, showing the equations of motion, the definition of the force field, which prescribes the interactions between atoms, and the challenges specific to ion channel simulations.
Three main ingredients are contributing to the success of MD as extremely refined and powerful tool to investigate conformational structure and changes and the relationship between structure and function in proteins. First, the development of highly parallelized and optimized hardware and software tools allows the simulation of systems of increasing dimensions and with increasing trajectory lengths. The second ingredient is the development and refinement of dedicated force fields, including not only amino acid groups but also lipids, solvent molecules, and specific substances as glycans, whose fundamental role has emerged clearly, for example, in the case of the glycosylate spike Covid-19 protein [Citation152]. Last, but not least, the development and improvement of a wealth of methods and algorithms to improve the statistical sampling of the conformational space (see Section 4.2).
The mathematical framework of MD is conceptually simple: for each of the atoms (the same holds in the case of coarse grained models), MD trajectories are generated by integrating Newton’s equations of motionFootnote2:
relating the mass and acceleration of the -th particle to the force acting on it, with
the vector of the
cartesian atomic coordinates and
a classical empirical potential function. In this representation, interactions are established between atom nuclei, while the electronic degrees of freedom are averaged out. A typical analytical expression of the interaction potential
reads
where the first four terms represent bonded interactions due to covalent bonds, which enforce chain connectivity as well as the secondary structure, while the latter two, the Lennard-Jones and Coulomb potentials, quantify non-bonded interactions of van der Waals and electrostatic nature. In particular, the first two terms are harmonic potentials allowing only small oscillations around the specified equilibrium values (,
) of bond lengths and bond angles, respectively. The intensity of these restraining potentials is quantified by the force constants
and
. In the third term, the torsional potential,
and
represent the barrier height and the multiplicity, respectively, while
and
are the current and equilibrium value of the torsional angles. In the Lennard-Jones potential,
represents the depth of the energy well whose minimum is located at
. Finally, in the Coulomb term,
and
are the atom charges, while
and
are the local and vacuum dielectric constants, respectively.
The sets of parameters of the empirical potential in EquationEquation (25)(25)
(25) are referred to as force field. Some of them are computed through quantum simulations of small organic molecules exploiting the concept of transferability, while others are estimated so as to match thermodynamic measurements. The most popular force fields for biomolecular simulation include AMBER [Citation153], CHARMM [Citation154], OPLS [Citation155], and GROMOS [Citation156] and, along with amino acids, they also include parameters for nucleic acids, lipids, carbohydrates, and ions. The parameterization of small organic compounds, for example, molecules of pharmaceutical interest, is more challenging, but the task has been simplified thanks to generalized force fields (GAFF [Citation157], CGenFF [Citation158]) and toolkits like Antechamber [Citation159], Paramfit [Citation160], and GAAMP [Citation161] with global optimization of parameters [Citation162]. MD atomistic force fields are continuously revised and assessed [Citation163]. Even water models are of pivotal relevance for a proper description of mechanisms based on hydrophobicity [Citation164].
The integration of EquationEquation (24)(24)
(24) can be performed with a variety of schemes [Citation148]. For instance, the velocity Verlet algorithm updates positions and velocities according to:
The timestep plays a key role, since, to keep the integration stable, it has to be tuned to the period of the fastest oscillating modes in the system. Typically, in biological system, these are associated with the covalent bonds, with
of
or
femtoseconds. Fixing the most rapidly vibrating bonds [Citation165], or reassigning the mass of hydrogens to the associated heavy atoms [Citation166] can allow for
up to 5 femtoseconds.
thus limits the achievable length of the simulated trajectories of biological systems which, currently, is of the order of few microseconds on high-performance supercomputers.
In order to evolve EquationEquation (24)(24)
(24) , initial conditions for the atomic positions and velocities need to be specified. For the latter, a Maxwell-Boltzmann distribution is typically used. For the atomic coordinates and the additional information on the protein topology needed to setup the bonded potentials in EquationEquation (25)
(25)
(25) , one typically resorts to structural data deposited in databases such as the Protein Data Bank [Citation167]. The provided structures also include information on atom types, which is required to setup the non-bonded interactions. Typically, even when the entire sequence of the ion channel is resolved, structural data need to be complemented with appropriate information for (a portion of) the cellular membrane and the surrounding aqueous solution. Initial ‘equilibration’ runs are needed in order to relax the structure in simulated conditions as close to the physiological ones as possible. During these runs, hydration and relaxation of the structures to the locally stable structure are achieved. In principle, equilibrium simulations for timescales comparable to experimentally relevant ones (milliseconds) should yield information on the dynamics of the protein and on its function, for example, the gating process of an ion channel, starting solely from structural information. Such brute force approach, however, is computationally inapproachable, except using ad-hoc supercomputer architectures discussed in Section 8. In addition, atomistic trajectories spend most of the time in well-defined regions of the phase space, only rarely jumping to another basin, which makes brute-force sampling extremely inefficient; the problem of rare events and enhanced sampling techniques are discussed in Section 4.2.
A typical issue in ion channel simulation is the calculation of ion currents and conductances. The importance of performing all-atom simulations is related to the pivotal role that the details of the chemical structure play in determining the behaviour of ion channels, gated by voltage, ions concentration, or ligand binding. Even a point-like mutation, or a residue chain rotation can be able to significantly change the channel conductance, by tuning or even impeding ion passage. In simulations, ions can be driven through the channel with a constant electric field perpendicular to the membrane plane that generates a membrane potential across the channel driving ion movement (as in , left panel). In this method, the potential applied to the membrane is
, where
is the constant field and
is the length of the simulation box in the direction of the membrane normal [Citation168,Citation169]. The constant electric field acts on all charged particles throughout the simulated system and affects their spatial distribution–especially in the bulk solution. This distribution results in a nontrivial transmembrane potential in the region of the pore that differs from
. The usage of periodic boundary conditions means that there is a single compartment because ions passing through the channel can be recycled avoiding charge build-up on either side of the membrane. However, it is also possible to impose appropriate conditions at the edge of the periodic box to maintain asymmetric ionic concentrations, allowing the simulation of the reversal potential yielding zero ionic current [Citation170].
Figure 5. Two typical simulation setups in computational electrophysiology: applying an electrical field (left) and using two reservoirs with different concentrations. Adapted from [Citation173], Copyright 2020, with permission from Elsevier.
![Figure 5. Two typical simulation setups in computational electrophysiology: applying an electrical field (left) and using two reservoirs with different concentrations. Adapted from [Citation173], Copyright 2020, with permission from Elsevier.](/cms/asset/a1fd464d-daf5-4249-9911-a6eb2e2f3ae3/tapx_a_2080587_f0005_oc.jpg)
An alternative method consists in simulating a system with two membranes, dividing the system into two compartments (as in , right panel). By adjusting the ion concentrations in each compartment a net charge imbalance can be created directly to model the desired electrochemical gradient [Citation171–173]. In this method, the potential applied to the membrane is , where
is the charge imbalance and
is the membrane capacitance. In this case, ions must be swapped between compartments after passing through the channel to maintain the desired potential through the charge imbalance.
The membrane potential and the charge imbalance
are conjugated thermodynamic variables [Citation168]. In the constant field method,
is under control and
can fluctuate, whereas in the compartment method,
is under control and
can fluctuate. Provided they are appropriately applied, using an external electric field or a charge imbalance can yield very similar results [Citation174]. Although early simulations examined only one or two permeation events [Citation12], increases in computational power now allow for many permeation events to be captured in multi-ms simulations, see for example [Citation13,Citation16,Citation175]. Agreement with experimentally measured currents cannot be guaranteed, due to many reasons, including the state and condition in which the channel structure was obtained as well as limitations in the MD force fields themselves.
One limit of the atomic description is that its high level of detail currently precludes, for computational limitations, the simulation of mesoscopic biological systems like whole organelles, vesicles, or viral particles. For instance, the simulation of an action potential would require the explicit simulation of a large stretch of membrane hosting a population of many different types of ion channels. This system is way too large for classical atomistic force fields. One possible approach to the problem is Brownian Dynamics, which is, in a way, a hybrid between mean field and atomistic approaches, see Section 4.3. A different approach more akin to the atomistic one, is that of coarse-grained force fields where the number of interaction centers (and thus the number of interactions) is reduced by lumping several atoms in a single Coarse-Grained (CG) particle (see for example, [Citation176–178]). A particularly successful CG tool for the study of protein/lipid systems is the MARTINI model [Citation179] which combines the speed-up benefits of simplified models with the resolution obtained for atomically detailed models. Indeed, the MARTINI model allows the simulation of very large membrane systems, for example, an entire viral envelope with 29 millions of CG particles [Citation180], allowing to access much larger time scales than atomistic simulations. Despite this high speed-up the MARTINI modeling retains a significant amount of molecular detail. In the MARTINI model on average four heavy atoms plus associated hydrogens are represented by a single interaction center. Mapping of water is consistent with this choice, with four real water molecules mapped to a CG water particle, while ions are modelled as a single particle, which represents both the ion and its first hydration shell. The MARTINI model has four main types of particles: polar, non-polar, apolar, and charged. Within each type, there are sub-types based on hydrogen-bonding capabilities or the degree of polarity, giving a total of 18 particle types or building blocks. These CG particles interact with one another through classical bonded potentials, Lennard-Jones interactions, and Coulomb interactions. The force field parameters have been tuned to reproduce a number of experimental thermodynamic data, in particular, the water/oil partitioning behaviour of a variety of compounds. The MARTINI model has been successfully used in a wide range of lipid-centered applications including the characterization of lipid membrane properties, lipid polymorphism, protein-lipid interplay and membrane protein oligomerization (see [Citation179,Citation181] and references therein), and lipid nanoemulsions [Citation182]. The MARTINI model assumes rigid protein secondary structures. However, recent refinements extends its applicability to cases where large conformational changes are involved, for example, ion channel gating [Citation183], where the Martini 3 force field was used [Citation184] in combination with a G-like model for proteins [Citation185].
It should be noted that both all-atom and coarse-grained approaches encompass some degree of empiricity; for both methods this is true for the force fields which need to be tuned with some ‘first principle’ data. However, moving away from the atomic description inherently requires additional assumptions on the location, interactions, and dynamics of the CG particles. In other words, improvements in the accessible time and length scales come at the price of an additional level of empiricity, which should be assessed on a case-by-case basis.
4.2. Rare events in ion channels
As introduced in Section 4.1, the requirement of a small timestep in the integration of the equations of motion limits the length of the simulated trajectories to a few microseconds. This limitation is particularly problematic in the case of biomolecular systems, such as ion channels, where many important functional processes (such as gating or ligand binding) take place on much longer timescales.
If we associate the different functional states of a channel to distinct minima in an energy landscape, in a standard MD simulation, the trajectory spends most of the time close to the local minimum in which it was initialised, and, only seldom, jumps to a different local minimum owing to sufficiently large thermal fluctuations (see ). If the thermal energy of the system is lower than the energy barrier separating the minima, the activated process happens very infrequently. However, when it occurs, it is quite fast. Sampling such rare events is thus a major challenge for MD simulations, which motivated the development of several specific methods to speed up such events and enhance conformational sampling [Citation80]. The crux is that, according to Eyring EquationEquation (16)(16)
(16) , the waiting time
between rare events scales exponentially with the height of the free-energy barriers
measured in thermal units
. As a result, the trajectories spend most of the time close to minima, while a comparatively short one in the transition state (on top of the barrier). Because of the temporal limitations of MD, normally very few barrier-crossing events can be observed and the sampling of the transition state is very poor.
Figure 6. Left) Illustration of the problem of rare events in biophysical simulations via a cartoon of the protein free-energy landscape: during typical simulations only local barriers can be overcome, while a number of configurational changes or conduction events lies beyond the current computer capabilities. Reproduced from [Citation186], under creative commons licence CC BY 4.0. Right) Conformational changes giving rise to opening and closing of the CRAC channel, see Ref [Citation187].
![Figure 6. Left) Illustration of the problem of rare events in biophysical simulations via a cartoon of the protein free-energy landscape: during typical simulations only local barriers can be overcome, while a number of configurational changes or conduction events lies beyond the current computer capabilities. Reproduced from [Citation186], under creative commons licence CC BY 4.0. Right) Conformational changes giving rise to opening and closing of the CRAC channel, see Ref [Citation187].](/cms/asset/5a1eba93-034f-422d-b2be-093e9928b892/tapx_a_2080587_f0006_oc.jpg)
In the specific case of ion channels, there are three main classes of thermally activated processes of paramount interest: i) structural changes, which switch between the functional states of the channel: open, closed, or inactivated (gating), ii) ion conduction, in which each ion has to overcome a free-energy barrier in order to translocate through the channel, and iii) binding of ligands or drugs to the channel. In the following, we will describe few enhanced sampling techniques developed to overcome the rare event problem and cite some examples on i) and ii). Problem iii), instead, is common to different kinds of proteins and is reviewed elsewhere [Citation188].
Enhanced sampling techniques can be broadly classified into three categories [Citation189]: (i) Techniques that do not focus on specific reaction coordinates; (ii) Techniques that aim to determine the path between known end points; and (iii) Techniques that enhance sampling or reconstruct the free energy along one or more selected reaction coordinates. While not truly an enhanced sampling technique, a final approach that has extensive use in ion channel simulations is that of alchemical perturbation discussed below. The actual implementation of enhanced sampling techniques is currently managed either by built-in modules to common MD codes or by specific packages, such as Plumed [Citation190], which works together with some of the most popular MD engines, as Amber [Citation191], NAMD [Citation192], and Gromacs [Citation193].
4.2.1. Techniques that do not focus on specific reaction coordinates
The first group includes techniques that do not focus on specific reaction coordinates (also referred to as Collective Variables, CVs) but simultaneously heat all the degrees of freedom of the system to increase the frequency of barrier crossing. These approaches have the advantage that they do not require predefined knowledge of the system to define reaction coordinates of interest.
The archetypal techniques of this group are Temperature and Hamiltonian Replica Exchange Molecular Dynamics (T-REMD and H-REMD) [Citation81,Citation194]. In T-REMD [Citation81] a number of replicas are run in parallel at different temperatures, and exchanges are accepted or rejected according to a Metropolis-like criterion (). A replica can thus easily evade from a kinetic trap when it is warmed up due to an exchange of atomic coordinates with a neighbouring replica. This method thus addresses the multiple-minima problem arising from the ruggedness of the free-energy landscape that threats the ergodicity of MD simulations. The most important limit of T-REMD simulations is their poor scaling with the size of the system, as the number of replicas is proportional to the square root of the number of atoms. This occurs because energy is an extensive property so that for large systems, the energy difference between neighbouring replicas will be large imposing a small temperature difference in order to have a sufficiently high overlap in energies of each replica to obtain a high enough acceptance probability. This, however, increases the number of replicas and thus the computational cost of the simulation. Heating only some parts of a system, such as the protein but not the solvent as in replica exchange with solute tempering (REST), can help alleviate this issue but can reduce the enhancement of conformational sampling [Citation195].
This kind of problems can be avoided in Hamiltonian Replica Exchange [Citation194] where replicas are simulated at the same temperature but with different energy functions. The different energy functions can be attained by splitting the energy function in different terms weighted by different scaling coefficients. The terms with unitary weights cancel out in the expression of the acceptance probability that therefore will depend only on the energy of a subset of degrees of freedom. Despite this improvement, H-REMD is computationally demanding for the simulation of membrane proteins and not frequently used in this field. Indeed, this technique has been mainly used for the simulation of peptides and small organic molecules [Citation196–198].
An alternative to running multiple simulations is to alter the potential energy function of the entire system so as to speed up transitions as in accelerated MD (aMD) [Citation199] or Gaussian accelerated MD (GaMD) [Citation200]. In these, the potential energy of wells below a certain threshold value are increased, while those above are not. This speeds up transitions between energy wells. In theory, re-weighting of the simulation trajectories can be achieved to reproduce the unbiased results. Re-weighting is prone to noise, something that is reduced in GaMD.
4.2.2. Techniques to determine the path between known end points
The most known of these approaches is transition path sampling (TPS) [Citation84]. In TPS, a statistical description of reactive trajectories is introduced via the definition of the transition path ensemble as the set of trajectories connecting the end states of a transition. Based on this description, reactive trajectories are directly obtained via specifically designed sampling procedures, and analyzed together to obtain information on the reactive process.
A key quantity in the approach is the reactive path probability, that is, the probability that a dynamical trajectory started in a metastable set (A) at time zero will end up in a different metastable set (B) at a successive time
, defined as
In (27) ] is the probability of the dynamical path,
and
are the characteristic functions of the corresponding regions (i.e.
= 1 if
and
= 0 otherwise),
and
are the states of the trajectory at times 0 and
, respectively, and
is a normalization factor.
Various deterministic and stochastic methods have been proposed over the years to sample this probability and, thus, the reactive path ensemble. The most commonly used rely on a Monte Carlo importance sampling procedure in the space of paths: starting from a given transition path, a new one is generated and accepted or rejected according to a Metropolis rule based on the reactive path probability. In practice, it is of relevance how the new paths are generated, since the acceptance rate, and thus the efficiency of a transition path sampling, will depend on how this is done. The most widely used is the so-called shooting algorithm, whose basic idea is to randomly pick a point along the old path, perturb the momenta of the system and propagate it forward and backward in time until it reaches the reactant or product state.
Another set of approaches to directly generate reactive trajectories is that of the string method and its variants, a class of algorithms based on the Transition Path Theory developed by E and Vanden-Eijnden [Citation201]. The zero-temperature string method [Citation202,Citation203] allows to identify the minimum energy path (MEP) in the potential landscape of a system, that is, the curve
that connects two minima of
via a saddle point and whose tangent is everywhere parallel to the force, that is
An efficient procedure to obtain the MEP is to discretize an initial path (the string) into a number of points (called images) and evolve them according to steepest descent dynamics, while enforcing a chosen parametrization of the whole curve. Examples of commonly used parametrization schemes are by equal or energy-weighted arc-length.
A different algorithm, named finite-temperature string method [Citation204,Citation205], was designed to calculate the so-called principal curve, that is, the curve joining the mean positions of the system on the hyperplanes perpendicular to the curve itself. Because of its definition, the location of this curve depends on specific features of the underlying energy landscapes, and is thus appropriate to be used when the landscape is rugged, or variations in the width of the reaction channel are relevant. The curve can be obtained via successive iterations of i) constrained sampling in the orthogonal hyperplanes, as in the original formulation [Citation204], or sampling in the Voronoi tessellation of the space generated by the discretized images along the curve itself [Citation205], ii) update of the images to the new centers of the distributions, and iii) a reparametrization step.
While the zero- and the finite-temperature string methods were first formulated in the space of the Cartesian coordinates of the system , versions using CVs have also been developed [Citation85,Citation205]. These allow to calculate the minimum free-energy path (MFEP), or the principal curve, in free-energy space, and permit the use of a large number of CVs, being thus useful for applications involving complex systems with many degrees of freedom such as ion channels.
Over the years, variants of the string method in CVs have been developed, such as the on-the-fly [Citation206], the swarms of trajectories [Citation207], or the dynamical string method [Citation208]. From a theoretical perspective, these algorithms calculate different paths [Citation201,Citation209,Citation210], and as a consequence, they also differ on the technique used to evolve the images. Concerning ion channels, applications of the string methods include the conformational transition of a potassium channel voltage sensor domain [Citation211] and the gating of the bacterial GLIC channel [Citation42,Citation212].
4.2.3. Techniques that employ selected reaction coordinates
Perhaps, the most commonly applied category of enhanced sampling techniques in ion channel simulations are those that aim to enhance sampling or reconstruct the free-energy (or Potential of Mean Force, PMF) landscape of the system with respect to a selected set of CVs, defined as
where are
CVs and
is a set of their values.
These techniques can be further classified into equilibrium (like Umbrella Sampling [Citation213] or the Blue Moon Ensemble technique [Citation214]), out-of-equilibrium (like Steered Molecular Dynamics [Citation215] and Targeted Molecular Dynamics [Citation216]), and close-to-equilibrium techniques (where the bias is small and incremental), like Metadynamics [Citation217] or Adaptive Biasing Force [Citation218] to cite but a few. In the last two techniques, the biasing potential is history-dependent, and favours the exploration of previously unvisited regions of the conformational space.
Good CVs should be low-dimensional descriptors of the functional dynamics of the system (often captured by the slow modes) [Citation86]. If a system is biased to explore the whole range of values of the CV, it will perform a uniform sampling of all relevant metastable states as well as the Transition State Ensembles separating them. Given the structural complexity of biomolecular systems, as membrane-embedded ion channels, the choice of the most appropriate CV is not trivial at all. A direct solution would be to bias a huge number of CVs to make sure to include the relevant ones. Unfortunately, the volume of the space of CVs that the simulation needs to explore grows exponentially with the number of CVs so that, for practical purposes no more than 2–3 CVs can be simultaneously biased. To properly identify the CVs, knowledge is required of the system degrees of freedom that are relevant for the free-energy barrier separating the minima. In complex biochemical systems, this choice is typically performed in a system-dependent manner. Yet, these approaches are widely used for the study of conformational transitions in proteins. For the specific case of ion channels, they are useful both to investigate conformational transitions and to evaluate the PMF for ion translocation.
Umbrella Sampling and Metadynamics have proven to be particularly useful in the field of ion channel research. In the first approach, the interval of CVs values to be explored is discretized, and several copies of the system are simulated, each restrained via biasing potentials to the different values. In this way, equally accurate sampling can be obtained across the whole CV range, including high-free energy regions. The CV distributions obtained from the restrained simulations are then unbiased and combined together to yield the proper full distribution, and hence the free energy as a function of the CV, for example, using the Weighted Histogram Analysis Method (WHAM) [Citation219]. Umbrella sampling has been frequently used to study ion permeation and selectivity [Citation10,Citation25,Citation220–222] and ligand binding [Citation223,Citation224], for example.
In metadynamics, the energy function is supplemented with an history-dependent biasing potential composed by a sum of Gaussian functions centered in the visited regions of the CV space. In this way, the biasing potential discourages the visit of regions already explored and pushes the simulation towards unexplored areas of the conformational space. In the limit of a very long simulation, the biasing potential fills all energy minima and the free-energy profile can be simply attained by reversing the sign of the biasing potential. Ideally, a metadynamics simulation should be stopped when all energy minima have been filled. Since it is difficult to identify this moment, there may be problems of overfilling that limit the accuracy of the free energy calculation. This kind of problems can be avoided by using well-tempered metadynamics [Citation225] where the height of the energy Gaussians decreases exponentially during the simulation and it is possible to tune the fraction of the energy basins that will be filled. Metadynamics has found multiple applications in ion channel research, for example, in studying permeation [Citation226,Citation227], gating related conformational changes [Citation228], and ligand binding [Citation229,Citation230]
Collective Variables (CVs) allow for a reduced and physically meaningful representation of the process under investigation. If few CVs satisfactorily describe a process it is possible to use them to reconstruct a low-dimensional free-energy landscape, for example, via umbrella sampling or metadynamics, or to drive an accelerated exploration of the phase-space [Citation231,Citation232]. For ion channels, this approach can be pursued only in some special cases, for example, ion permeation [Citation233] or inactivation [Citation234]. Unfortunately, in the general case of gating, it is not easy to find simple descriptors for the complex structural rearrangements undergone by ion channels. High-dimensional CVs, however, preclude the reconstruction of large portions of their free-energy space. In those cases, path methods, such as the string method in collective variables [Citation85] and related ones mentioned above, are more efficient because they entail a local optimization of the path. This efficiency, on the other hand, comes at the cost of exploring only a region close to the initial guess path. Overall, determination of CVs in gating problems is still an open challenge: in Section 6 we make some examples, which explore several rare event problems in ion channel research and discuss the choice of suitable descriptors.
4.2.4. Techniques that employ alchemical perturbations
Alchemical perturbations, such as the addition or removal of atoms, or the mutation of one amino acid residue to another during a simulation can be considered as an example of techniques that exploit the knowledge of end states to compute the free-energy difference between them. The most commonly employed approaches for this are the related methods of Free-Energy Perturbation (FEP) [Citation235] and Thermodynamic Integration (TI) [Citation236]. The approach exploits the fact that free energy is a state function so that the free-energy difference between end states can be attained as the sum of the free energies of a number of reactions arranged so as to close a thermodynamic cycle. Moreover, since the path is of no interest here, some or all of these steps may be un-physical which justifies the name of alchemical methods. In practice, the transition is broken into multiple steps (characterised by the parameter that varies from 0 to 1), each of which is run as a separate stage of the simulation or as separate simulations (i.e. atoms slowly appear or disappear across this transition). The total free-energy change can be summed from the individual steps.
A typical application of alchemical methods is drug design, in which a key parameter is either the absolute free energy of binding of a ligand to a protein, or the relative-binding free energy of two different ligands [Citation224,Citation237]. In the former case, the reaction of interest is the migration of the ligand from bulk to the protein-binding site, which can be calculated from summing the free energy of ligand annihilation in bulk and ligand creation in the site. For relative free energies, the annihilation of one ligand in the binding site can be done simultaneously with the creation of the other. This approach has also found significant value in understanding the basis of ion selectivity, where the free energy to swap one ion type to another is computed at different points in the permeation pathway or in model-binding sites [Citation20,Citation238].
For the sake of completeness, we will cite an alternative method for the computation of free energies of binding, MM-PBSA or MM-GBSA [Citation239]. In these methods, molecular mechanics energies (representing binding energies in vacuo) are combined with solvationfree energies (of ligand, receptor, and complex) computed by solving the linearized Poisson-Boltzmann or generalized Born equation. Using these contributions it is possible to build a thermodynamic cycle to compute binding free energies in solution. Of note, the direct calculation of the binding free energy of solvated reactants would be highly inaccurate because energy would be dominated by solvent–solvent interactions and the fluctuations of the total energy would be an order of magnitude larger than the binding energy. MM-PBSA and MM-GBSA can be considered intermediate in both accuracy and computational effort between empirical scoring and strict alchemical perturbation methods. In the last years, these approaches have been applied to a large number of systems with varying success [Citation240–242].
4.3. Hybrid methods: Brownian Dynamics
Brownian Dynamics can be considered as a compromise between the computational efficiency of continuum methods and the molecular accuracy of atomistic ones. Finite size and ion–ion correlation effects are recovered in Brownian Dynamics (BD) [Citation243], a technique where ions are explicitly modeled as particles while water, lipids, and proteins are still treated as continuous dielectric media. BD allows to observe statistically relevant numbers of permeation events in a time range from the microsecond to the millisecond and it thus represents the method of election for the computation of ion currents. Ion trajectories are computed integrating Langevin equation [Citation244]:
The right-hand side of EquationEquation (30)(30)
(30) represents the forces acting on particle
. The term
is an effective potential, whose negative gradient with respect to
is the force stemming from the interaction with other particles, with the fixed charge distribution of the protein and membrane, and with the reaction field. The role of the solvent is implicitly accounted for by the friction term
and the gaussian fluctuating force
, which models random collisions.
The dynamics of ions in water is well approximated by the over-damped regime where the inertial term can be neglected and the Langevin equation reduces to
where is the ion diffusion coefficient and
is a Gaussian random noise. In principle, the diffusion coefficient should be position-dependent but most simulation works employ a single position-independent diffusion coefficient for all atoms of the same species. The effective potential
is split in a number of contributions that account for ion–ion interactions as well as the interactions of the ion with the pore, solution, and other biomolecules [Citation97,Citation245]:
where the first contribution on the RHS models ion–ion interactions and includes a Lennard-Jones and a Coulomb term, the second contribution is a confinement potential that models the geometry of the pore representing the ion-accessible region, represents the static field, that is, the electrostatic potential generated by the fixed charge distribution of proteins and lipids, and
is the reaction field, that is, the electrostatic potential generated by the polarization of the dielectric boundaries [Citation246]. Notable extensions of the basic BD algorithm include that of Roux and coworkers [Citation97,Citation247], who designed a Grand Canonical Monte Carlo/Brownian Dynamics scheme that allows to model a variable number of ions and asymmetric ion concentrations, and that of Chung and colleagues [Citation248], who included mobile protein elements.
5. Brownian Dynamics: applications
The accuracy of Brownian Dynamics can be enhanced if the effective potential is computed from fully atomistic Molecular Dynamics simulations. Using this approach Berneche and Roux [Citation249] analyzed the conduction mechanism in the KcsA potassium channel. In this work, the effective potential was considered as a function of the axial coordinates of the three ions occupying the selectivity filter and it was decomposed as
where is the equilibrium potential that was derived from all-atom umbrella sampling simulations and
is the transmembrane potential profile that was computed with Poisson-Boltzmann equation. In particular, the Brownian simulation was implemented as a continuous-time Markov chain with discrete states corresponding to the ions positions. Forward and backward transition rates were computed as
Using this approach the authors measured a maximal conductance of the channel in excellent agreement with experimental data [Citation17] (550 and 360 pS for outward and inward fluxes, respectively). More importantly, the large statistics of crossing events, accrued during the long BD simulations, enabled the authors to clarify the mechanism of outward permeation which proceeds along the sequence of states , where
with
identifies the binding sites inside the selectivity filter of KcsA. Inward permeation was found to occur in the reverse order. When this information was integrated with the free energy maps it became clear that the key step of the process was the
transition because configuration
was less stable than
. This finding thus justified the difference between the outward and inward conductance, challenging the traditional view of isoenergetic states and barrierless conduction.
The use of ion channels and nanopores for DNA sequencing is another application where the use of MD simulations to tune the parameters of Brownian Dynamics can lead to invaluable results. The idea to sequence DNA exploiting the signature currents of the four nucleotides as the chain is electrophoretically pulled through a nanopore, dates from the beginning of the 2000s [Citation250,Citation251]. However, it soon became clear that the current cannot depend on a single base but more likely a stretch of a few nucleotides whose sequence varies in time in a sequence-dependent manner. Since no experimental device can currently image the conformation of a DNA chain as it is pulled through a nanopore, Molecular Dynamics algorithms have become the tool of election. Unfortunately, the computational measurement of the small currents flowing through ion channels requires prohibitively long simulations. For instance, a typical 100 pA ion current corresponds to the observation of approximately 104 ion crossing events which requires a 16 s MD simulation. Simulations of this length can be routinely run using Brownian Dynamics but the accuracy of the simulation requires a careful tuning of the effective potential using information deriving from atomistic MD. In the protocol designed by Comer and Aksimentiev [Citation252] (named Atomic Resolution Brownian Dynamics – ARBD) the effective potential is decomposed in an ion–ion interaction term and a term of interaction of the ions with nanopore and DNA:
The term was computed through three sets of Umbrella Sampling simulations that determined the PMF of the K+-K+, K+-Cl – and Cl–-Cl – pairs as a function of the distance between the two ions. The
were also computed from Umbrella sampling simulations where a single potassium or chloride ion was restrained to the center of the cells of a hexagonal lattice surrounding a single nucleotide restrained to the typical B-form of DNA and immersed in a water box. Comer and Aksimentiev showed that the 3D-PMFs generated from isolated nucleotides can be combined together to generate the PMF of large systems like oligonucleotides and base pairs. As an example, the reconstructed PMF of the A
T base-pair,
, can be computed from the PMF of the isolated A and B nucleotides (
and
respectively) as:
where and
are the rigid-body transformations that map the coordinates
and
of the isolated A and B nucleotides to the coordinates
of the same nucleotides in the A
T base-pair. In a similar fashion, Comer and Aksimentiev showed that the PMF of a single-stranded DNA chain (ssDNA) can be attained by summing the PMF of the individual component nucleotides (with appropriate geometric transformations). Moreover, if a nucleotide is replaced with another one, the PMF of the ssDNA can be updated by appropriately replacing the PMF of the mutated base. The limit of this approach is that it cannot be used to build the PMF of double-stranded DNA (dsDNA) because the interaction of potassium ions for the minor groove is significantly under-estimated. The PMF of dsDNA must therefore be computed through atomistic umbrella sampling simulations. Mutations, however, can then be applied just as with ssDNA. It is important to stress that the computational efficiency of the method by Comer and Aksimentiev is critically reliant on the ability to combine elementary PMFs to generate the PMF of larger systems. Indeed, despite the power of Brownian Dynamics, there will be no computational saving if for each new system an expensive umbrella sampling simulation must be run to produce the effective potential. The accuracy test showed that ARBD generates ion distributions consistent with those predicted by atomistic simulations. Moreover, at low salt concentrations, ARBD computes currents in quantitative and qualitative agreement with those of atomistic MD. Finally, at higher concentrations, the agreement between ARBD and atomistic simulations is only qualitative. The authors, however, observed that the currents computed by ARBD in bulk solution are in better agreement with experimental data than the currents predicted by atomistic simulations. It remains to be tested whether this better agreement is also preserved in the confined environment of nanopores partially occluded by nucleotide chains.
6. Atomistic approaches: applications
The most notable properties of ion channels are ion selectivity, high permeation rates, and gating which allow them to orchestrate complex biological functions, such as neuron firing and muscle contraction. Because of the problem of rare events explained in Section 4.2, the characterisation of each of these properties requires enhanced sampling techniques. In the following we review few selected examples in which different techniques were used to assess permeability, selectivity, and gating in ion channels or models thereof. These examples are by no means exhaustive and reflect the authors’ expertise.
6.1. Permeability and selectivity of NaChBac
Guardiani et al. [Citation253] used metadynamics to study the permeability of a bacterial voltage-gated sodium channel, NaChBac. Interestingly, while a PMF derived from a long equilibrium simulation exhibited four minima (in agreement with a study by Catterall and coworkers on NavAb [Citation254]), the PMF derived from a metadynamics simulation biasing a single Na+ ion to explore the Selectivity Filter (SF) featured a single minimum so deep that the ion would remain trapped inside. The mismatch turned out to be due to an energy barrier in the middle of the SF that an ion could overcome only after receiving an electrostatic kick from a second incoming ion. Indeed, this knock-on mechanism was clearly identified when the metadynamics simulation was repeated biasing two ions instead of one. These results clearly highlight a requirement common to metadynamics and all collective-variable driven methods, including umbrella sampling [Citation213], i.e., these approaches need some preliminary knowledge of the number and kind of collective variables relevant to describe the process at hand; in this case, this meant that some rough idea of the permeation mechanism had to be known a priori.
Metadynamics is a powerful tool also for the study of selectivity. Indeed, equilibrium and metadynamics simulations [Citation255] revealed that a single calcium ion could access the SF of NaChBac but then remained stuck inside. This is due to the larger binding free energy of Ca2+ as compared to Na+ and to the unlikelihood of a Ca2+/Ca2+ knock-on mechanism. Indeed, in metadynamics calculations with a fixed and a mobile ion, the free-energy profile experienced by the incoming ion featured a very high barrier due to the electrostatic repulsion of the resident ion.
6.2. Bias exchange metadynamics simulations of NavAb
The NaChBac simulation study reported in Section 6.1 highlights a limit of metadynamics that is shared by many other enhanced sampling techniques: the necessity of a preliminary knowledge of the type and number of ions involved in the permeation mechanism. As also mentioned in Section 7, in Bias Exchange (BE) metadynamics several replicas of the system are run in parallel. Each replica runs a metadynamics simulation with bias on different collective variables, and structural exchanges are attempted at regular time intervals. The exchanges are accepted with probability where
. This technique naturally lends itself to the study of multi-ion permeation processes. Indeed, if
is the maximal number of ions that are expected to be involved in permeation, it is sufficient to use
replicas each biasing the axial position of a single ion (plus a neutral replica where no biasing potential is applied). Since ions are free to leave or remain inside the pore, the number of ions taking part in conduction will also vary. Moreover, while each replica enhances the dynamics along the single biased CV, the exchanges between replicas enhance the sampling along the whole set of CVs biased in all the replicas. Domene et al [Citation256] applied the method to the study of a simplified model of the NavAb sodium channel. Indeed BE-metadynamics yielded 1D- and 2D-PMF in excellent agreement with those generated by umbrella sampling at a fraction of the computational cost of the latter technique: while fullfree energy convergence took 300 ns in BE-metadynamics, more than 1
s was required by umbrella sampling. Furthermore, in BE-metadynamics, the number and type of ions involved in conduction events did not need to be predefined, and conduction events where different numbers of ions are involved could be analyzed and compared using a single simulation.
6.3. Structure of claudin-based paracellular channels
Recently, computational studies on claudins, transmembrane proteins that are part of tight-junctions between adjacent epithelial or endothelial cells, have been reported. Using structural modelling and all-atoms molecular dynamics simulations Alberini et al [Citation257] refined the channel architecture proposed by Suzuki et al [Citation258,Citation259]. To validate the model, the authors verified its experimentally known selectivity properties for cations [Citation260] by employing the Voronoi-tessellated Markovian Milestoning method for free energy and kinetics calculations [Citation205,Citation261]. Milestoning allows reconstructing the long-time dynamics of a system from the crossing statistics of its trajectory through a set of hypersurfaces (the milestones) placed along the reaction coordinate [Citation262,Citation263]. It can be employed to obtain mean-first-passage-times and in turn permeability coefficients (compare with Section 3.2) across membranes or channels, even without relying on the so-called inhomogeneous solubility-diffusion model [Citation264]. Voronoi-tessellated Markovian Milestoning is a version of the method that leverages independent simulations confined within a set of cells spanning the reaction coordinate. Transition path theory [Citation265] is then used to obtain the kinetic properties of the full reaction from hitting statistics at the cell boundaries, identified as milestones.
6.4. Structures and permeability of nAChR
Enhanced sampling techniques are also very important for the functional annotation of Ligand Gated Ion channels (LGIC) that requires the investigation of the ion translocation through the pore crossing high free-energy barriers. Within this framework Chiodo et al [Citation266–269] investigated the 7 nicotinic acetylcholine receptor (nAChR), a widely expressed LGIC of the brain related to schizofrenia and Alzheimer’s disease [Citation270,Citation271]. Performing analysis of the profile and hydration in extensive molecular dynamics simulations of a homology model, the authors were able to associate different conformations to four different functional states: the open active state [Citation266], a desensitized state [Citation267], a closed-locked, non-conductive state [Citation268] and the apo-resting state [Citation268]. A comparison with the recent experimental structures of the channels in different states [Citation272,Citation273] confirms the accuracy of the models.
To provide a refined description of the process, the ion permeation across the all-atoms full-length (TMD+LBD) human 7 channel model, was studied both in native and in the E-1’A mutant [Citation274]. The single-ion PMF (i.e. the PMF of one ion while no other ions are present in the pore, see
in EquationEquation (23)
(23)
(23) ) and the ion translocation kinetics were reconstructed for sodium and chloride by using the milestoning method with Voronoi tessellation [Citation205,Citation261].
The 1D PMF profiles shown in allowed to identify the structural determinants of ion translocation, i.e. the key residues responsible for the formation of energy barriers and kinetic traps, along the protein structure represented in background in . The mean first passage time to traverse the full channel is smaller for sodium than chloride consistent with the experimentally determined cationic nature of wild type 7. Moreover, results indicate that the free-energy barriers in the transmembrane domain play the major role in ion permeation, in agreement with results from simulations on GLIC [Citation275].
Figure 7. PMF for the permeation of sodium (black) and chloride (red) ions through the full 7 native channel. The curves are shifted along the y axis so that their values match at the intracellular end (on the left). On the background: two protein subunits are shown, represented in cartoon, for the sake of clarity. Lipids are in line representation; water represented with points. Dots indicate the centers of the Voronoi cells. Figure reproduced with permission from [Citation274]. Copyright 2020 American Chemical Society.
![Figure 7. PMF for the permeation of sodium (black) and chloride (red) ions through the full α7 native channel. The curves are shifted along the y axis so that their values match at the intracellular end (on the left). On the background: two protein subunits are shown, represented in cartoon, for the sake of clarity. Lipids are in line representation; water represented with points. Dots indicate the centers of the Voronoi cells. Figure reproduced with permission from [Citation274]. Copyright 2020 American Chemical Society.](/cms/asset/230ddd9c-0b87-4122-b4b7-de64c61db2e1/tapx_a_2080587_f0007_oc.jpg)
6.5. Gating of hERG
So far examples focused on permeation and selectivity but ion channels exhibit another key property, gating, i.e. the ability to open and close in response to specific stimuli. A particularly interesting case is represented by hERG, a potassium channel involved in the repolarization of cardiomyocytes [Citation276], whose mutations and nonspecific interactions with a wide range of drugs leads to the Long QT Syndrome [Citation277,Citation278]. In order to limit the incidence of this disease, the FDA has promoted the Comprehensive in vitro proarrhytmia assay [Citation279], a screening program to exclude from the pharmaceutical development pipeline drugs exhibiting unintended interactions with hERG.
From the structural point of view hERG is formed by four identical subunits each comprising a Voltage Sensor Domain (VSD), a Pore Domain (PD), and a carboxy-terminal domain [Citation280], see ). hERG responds to changes in the membrane potential with a movement of helix S4 of the VSD. This motion is somehow transduced to the PD where the gate is located. In order to clarify the mechanism of electro-mechanical coupling Costa et al [Citation52] used a network theoretical approach already applied to the study of allosteric enzymes [Citation49,Citation50] and ion channels [Citation51,Citation281,Citation282]. The channel is modeled as a network where each node corresponds to a residue, arcs correspond to interactions between residues and edge weights quantify the efficacy of motion propagation. Once the network is built, the minimal paths connecting all residues of a source and a sink region are computed using Dijkstra’s algorithm. Using this approach two families of VSD-PD pathways were identified, see ). Interestingly, several mutations known to affect hERG gating could be mapped on the discovered paths suggesting that mutations act by interrupting the electro-mechanical coupling between VSD and PD.
Figure 8. Structure of the hERG channel (b) with definition of the VSD, PD, and CTD (a). Pathways connecting the VSD and the PD (c) identified for a representative subunit by a network theoretical approach, which considers the residues as the nodes and the distances related to their correlations. Figures reproduced with permission from [Citation52] under CC BY 4.0 license.
![Figure 8. Structure of the hERG channel (b) with definition of the VSD, PD, and CTD (a). Pathways connecting the VSD and the PD (c) identified for a representative subunit by a network theoretical approach, which considers the residues as the nodes and the distances related to their correlations. Figures reproduced with permission from [Citation52] under CC BY 4.0 license.](/cms/asset/9ba8d886-8723-424c-a6f5-99bae4d28d50/tapx_a_2080587_f0008_oc.jpg)
6.6. Hydrophobic gating in model channels
While hERG exhibits the typical voltage-gated steric occlusion of the channel, in other channels ion currents are blocked by hydrophobic gating [Citation38,Citation44,Citation283,Citation284]. Hydrophobic gating involves the formation of a vapour phase – a bubble – nucleating from the liquid phase inside the hydrophobic portion of the cavity of the biological channel [Citation268]. Bubble formation in confinement has been shown to be deeply dependent on several characteristics of the nanopore, such as hydrophobicity, geometry, and size [Citation45].
Simplified models of ion channels are a useful tool to disentangle the competing factors affecting the energetics of bubble formation in ion channels [Citation44,Citation283] and to provide microscopic interpretations of experimental results on nanoporous materials [Citation285]. An example of this physical approach to hydrophobic gating is the study of the drying mechanism of model nanopores in the presence of an hydrophobic solute dissolved in water [Citation47]. A cylindrical nanopore with the typical hydrophobicity of nonpolar aminoacids was studied by means of a rare event method, Restrained Molecular Dynamics [Citation231], in order to characterize the kinetics of drying. Two collective variables were used: one controlling the water filling of the pore and the other the axial position of the gas. The bubble gating process was shown to be crucially modified by the physical presence of the hydrophobic solute inside the channel, see . In particular, a single atom of argon was enough to abate the drying free-energy barrier between the wet and the dry state of the hydrophobic nanopore, causing the instantaneous drying of the whole nanopore for sizes comparable to the inner section of biological channels. Moreover, it was shown that the gas particle stabilizes the dry state of the nanopore and that the most probable mechanism of drying for this system corresponds to the infiltration of the gas particle inside the wet nanopore followed by the drying event. Such mechanism becomes relevant in connection to the context of general anesthetics, and in particular of volatile anesthetics, such as noble gases [Citation286] and to the hypothesis that bubble formation may be involved in general anesthetics [Citation44].
Figure 9. Illustration of the effect of a single hydrophobic particle on the dewetting of a model nanopore: the dewetting barrier is abated when an Argon atom enters the water-filled pore, thus accelerating the process of hydrophobic gating. Reproduced with permission from [Citation47]. Copyright 2020 American Chemical Society.
![Figure 9. Illustration of the effect of a single hydrophobic particle on the dewetting of a model nanopore: the dewetting barrier is abated when an Argon atom enters the water-filled pore, thus accelerating the process of hydrophobic gating. Reproduced with permission from [Citation47]. Copyright 2020 American Chemical Society.](/cms/asset/4cf60835-97e0-4f06-9b26-591344715be8/tapx_a_2080587_f0009_oc.jpg)
6.7. Identification of functional states of Kv and Nav channels
Voltage-gated ion channels undergo conformational changes in response to membrane potential, cycling between closed and open states. While X-ray crystallographic structures of Kv1.2, Kv1.2/Kv2.1 chimera and NavAb channels [Citation254,Citation287,Citation288] in the active state became available already in the early 2000s, the resting state structure of the VSD resisted for very long to experimental determination. As of 2022 the situation has not particularly improved. In 2014, the VSD of Ciona intestinalis was crystallized in both the active and resting states [Citation289], but the resting states of most other Kv and Nav channels are still unknown.
This experimental bottleneck prompted massive computational efforts to predict the resting state. Early models were obtained using the Rosetta program [Citation290] and refined through atomistic simulations [Citation291]. Other models were generated through restrained MD simulations enforcing a wide array of constraints deriving from experiments of mutagenesis, cross-linking, fluorescence, and resonance-energy transfer. These techniques indeed identify residue–residue interactions that can be readily translated into distance constraints. A notable work in this field was performed by Vargas et al [Citation292] who computationally engineered metal bridges between pairs of residues experimentally predicted to be in close proximity. An alternative approach is based on simulations incorporating the effect of the membrane potential. In this case, the ambition is not only to predict the resting state of the VSD but also to reconstruct the complete sequence of events of the active resting conformational transition induced by membrane hyperpolarization. A particularly important contribution was given by Jensen et al [Citation14] who ran hundreds of microseconds of MD simulations using a non-physiological potential (−750 mV) to accelerate the transition.
Remarkably, despite the wide variety of computational methods used by these investigators, the research results were largely in agreement with each other, yielding a ‘low-resolution’ consensus model [Citation293] (see ) that can be used as a benchmark to discuss the idealized gating mechanisms proposed in the literature [Citation294–297]. The consensus model [Citation293] of the resting state of the VSD lends strong support to the helical-screw/sliding-helix model [Citation294,Citation295]. Indeed, all the resting state models show a significant roto-translation of helix S4 that undergoes a helical displacement of 10 Å. This displacement is significantly smaller than that predicted by the paddle model (20–25 Å) [Citation296], but larger than that implied by the transporter-like model [Citation297]. Even if extreme caution should always be exercised when dealing with computational predictions, the consensus model clearly shows the power of computational modeling and MD simulations in the solution of key problems of membrane physiology.
Figure 10. Main elements of secondary structure of the VSD in the active and resting state. (a) The VSD in the active conformation (taken from the x-ray structure; PDB accession no. 3LUT). (b) A superposition of different models of the resting- state configuration of the VSD obtained by independent research teams using different constraints and methodologies. The four helices, S1 (gray), S2 (yellow), S3 (red), and S4 (blue), are displayed. The spheres correspond to the C atoms of E1 (Glu226) and E2 (Glu236) along S2, and R1 (Arg294) along S4. ©(2012) Vargas et al. Originally published in Journal of General Physiology [Citation293].
![Figure 10. Main elements of secondary structure of the VSD in the active and resting state. (a) The VSD in the active conformation (taken from the x-ray structure; PDB accession no. 3LUT). (b) A superposition of different models of the resting- state configuration of the VSD obtained by independent research teams using different constraints and methodologies. The four helices, S1 (gray), S2 (yellow), S3 (red), and S4 (blue), are displayed. The spheres correspond to the Cα atoms of E1 (Glu226) and E2 (Glu236) along S2, and R1 (Arg294) along S4. ©(2012) Vargas et al. Originally published in Journal of General Physiology [Citation293].](/cms/asset/bcbce4d5-eef4-4840-886c-bd7337d41b05/tapx_a_2080587_f0010_oc.jpg)
7. Outlook and perspectives
The possibility to perform biomolecular simulations critically relies on the availability of good-quality structures. This necessity can be summarized in the statement: no structure, no simulation. This problem is particularly serious in the case of membrane proteins that are too large and hydrophobic to be resolved through NMR spectroscopy and are notoriously difficult to crystallize [Citation298]. Indeed, membrane proteins represent between 20 and 30% of the proteome of most organisms, yet, to date, less than 1% of the entries of the Protein Data Bank are membrane proteins, and only a small fraction of these are eukaryotic. Eukaryotic membrane proteins cannot be expressed in prokaryotic systems because they require specific eukaryotic machinery for post-translational modifications and to be trafficked to the membrane. This is why eukaryotic membrane proteins cannot be produced recombinantly through standard genetic engineering techniques but they need to be purified from native sources. This, however, leads to another problem, because membrane proteins are unstable [Citation299] even when treated with the mildest detergents needed for their extraction. The necessity to use detergents leads to high-volume crystals containing large amounts of solvent. These crystals are fragile, diffract at low resolution and suffer from irradiation damage. Over the last few years, cryo-electron microscopy (cryo-EM) has emerged as a powerful alternative to X-ray diffraction for the structural resolution of membrane proteins [Citation71]. Indeed, cryo-EM requires small volumes and lower concentrations so that it can be applied to samples purified from natural sources. Moreover, cryo-EM does not require removal of flexible loops or the addition of stabilizing substrates to induce crystallization. Finally, while X-ray structures may suffer from artifacts due to crystal packing forces, the rapid quenching to the temperature of liquid nitrogen required by cryo-EM freezes the protein in a set of conformations more likely to represent functional states [Citation300].
Although the overall number of available ion channel structures is still underrepresented as compared to other protein families, the improvement in the experimental techniques provided in the past five years has led to a remarkable increase in their availability. This is, for example, the case of human nicotinic receptors and voltage gated sodium channels for which a wealth of eukaryotic structures have been very recently deposited [Citation272,Citation273,Citation301–309].
The scarcity of experimental ion channel structures prompted in the years the development of a series of computational tools to predict ion channels directly from protein sequences. Homology modeling combined with atomistic simulations provides a valuable approach to investigate the structure-dynamics-function relationship in several ion channels [Citation310,Citation311]. Methods based on machine learning algorithms have been recently developed in ion channel research, from sequence-based predictions to the topology of the transmembrane region, including structure-activity relationship [Citation312–314]. Moreover, machine learning shows further promise for structure prediction, see the AlphaFold [Citation315] successes at CASP13 and CASP14.
Nowadays the most popular technique for the computational study of membrane proteins is represented by Molecular Dynamics simulations. Despite impressive improvements over the last few years, MD simulations still suffer from a number of limitations that in 1990 were highlighted by Karplus and Petsko [Citation316] in a sentence that still holds true today: ‘Two limitations in existing simulations are the approximations in the potential energy functions and the lengths of the simulations. The first introduces systematic errors and the second statistical errors’.
In order to overcome the approximations of the potential energy functions, massive efforts are being performed in the development of more accurate force fields. A major limitation of most currently used force fields is that they employ fixed atomic charges corresponding to the electrostatic environment of bulk solvent. This assumption is not always correct. For instance, non-polarizable force fields under-estimate by a factor of 2 the dielectric constant of the lipid bilayer [Citation317] doubling the energy barrier of an ion crossing the membrane. The lack of lipid polarization is reflected in the free-energy profile of an ion crossing the gramicidin A channel [Citation318–320]. Non-polarizable force fields also lack accuracy in the modelling of divalent cations like Ca2+ and Mg2+ [Citation321] that, owing to their high charge density, tend to polarize the surrounding water shell. At the moment, the most commonly used polarizable force fields are the Drude oscillator [Citation322,Citation323] and the AMOEBA force field [Citation324]. Even if promising results have been reported, the development of these force fields is still a work in progress hampered by the limited availability of model parameters and the increased computational cost [Citation325]. For instance, using NAMD the computational cost of a simulation with a polarizable force field is twice that of the non-polarizable counterpart [Citation326,Citation327]. The increasing use of GPU cards for biomolecular simulations will likely provide new momentum to the development of polarizable force fields [Citation328]. Besides the issue of computational cost, the modeling of electrostatic interactions also needs further refinement. Polarizable force fields are more accurate than classical force fields in the simulation of heterogeneous environments featuring regions with different dielectric constants or different hydrophobicity.
The problem of the short length of MD trajectories, already highlighted by Karplus and Pesko [Citation316], lies at the heart of any simulation method. Indeed, MD simulations have the ambition to connect structure with function through dynamics but, while current trajectories routinely cover hundreds of nanoseconds, relevant biological phenomena occurs in milliseconds to seconds. For instance, this is the case of electrophysiological recordings that are measured over several milliseconds. In order to rationalize at the molecular level the experimental patterns, MD simulations need to reach longer time-scales. A brute force approach to overcome this limitation is to increase computing power. While in the 1980s supercomputers contained just a few powerful Central Processing Units (CPUs) in the 1990s the trend emerged to combine several CPUs into a cluster [Citation72]. Nowadays supercomputers of top high-performance computing centres may contain hundreds of thousands to millions of CPU cores. Another trend involves the use of Graphical Processing Units (GPUs) originally designed for gaming [Citation78]. This hardware is particularly well suited for highly repetitive operations like the force calculations that represent the most demanding part of MD simulations. The bottleneck here is the necessity to translate MD codes from Fortran or C to a more appropriate programming language like CUDA. However, given the small cost of GPUs it can be foreseen that in the near future ordinary researchers will afford to buy multi-GPU workstations providing the opportunity to do high-performance computing at their desk. An interesting alternative to CPU- and GPU-based general purpose computers is the design of a special purpose machine with an architecture tailored for MD simulations like the Anton supercomputer [Citation79]. Anton was named after the early microscopist Anton van Leeuwenhoek with the declared ambition to develop a computational microscope with a resolution unattainable by any experimental method. Anton, that owes part of its high performance to a parallel architecture and optimized inter-node communications, has led to several important discoveries. For instance, Anton has helped to clarify the mechanism of transition between open and closed states in voltage gated potassium channels [Citation13] and has allowed simulations of drug-binding in G-protein coupled receptors [Citation329]. Moreover, the long simulations allowed by Anton revealed the flaws of a force field that would remain latent in shorter simulations. The third generation of Anton supercomputers has been announced in 2021 [Citation330], which claims to perform on 512 nodes s per day for a million-atoms system.
In many cases, even the use of high-performance hardware does not enable sufficient sampling – anyway not efficient – calling for advanced enhanced sampling techniques. In section 4.2 we described several enhanced sampling approaches. Two of them, Metadynamics and Replica Exchange Molecular Dynamics have proven to be particularly effective, but they still present a number of limitations. The most critical problem of metadynamics is represented by hidden degrees of freedom, which may not be accurately described by the chosen CVs and can frustrate the exploration of the phase space, sometimes limiting the extent of convergence and accuracy of results, see, for example, the rightmost panel in in which biasing along a single CV, say S1, does not allow the correct reconstruction of the free-energy landscape. REMD simulations, on the other hand, do not need any preliminary choice of CVs and they enhance the sampling of all degrees of freedom through high temperatures. Unfortunately, the necessity to use a number of replicas proportional to the square root of the number of atoms, makes the technique too computationally demanding for the simulation of large systems as a membrane-embedded ion channel. It is thus clear that the strengths and weaknesses of CV-based methods and REMD are complementary and their combination generates a new class of promising methods, see also [Citation331,Citation332]. A first combination of the two techniques is represented by Parallel Tempering Metadynamics [Citation82]. In this algorithm, a number of metadynamics simulations biasing the same CV are run in parallel at different temperatures and exchanges between neighbouring replicas are performed with the Metropolis rule as in REMD. In this scheme, metadynamics ensures an effective sampling along the chosen CV while the exchanges with high-temperature replicas allow sampling along orthogonal CVs. Another hybrid algorithm combining metadynamics and REMD is Bias Exchange Metadynamics [Citation83] where a number of replicas are run in parallel with bias on different CVs and exchanges are performed at regular time interval with the Metropolis criterium.
Figure 11. Illustration of machine learning applications to the analysis and enhancement of MD simulations. High dimensional data, e.g. the protein trajectory, is used as the input of an artificial neural network (ANN). The ANN is trained to project the input to a low dimensional space minimising a loss function. Depending on the structure of the ANN and on the loss function, the low dimensional representation captures different features that are considered to be important, such as slow modes. In some methods, the features learnt are used to further enhance the sampling of MD simulation as shown by the arrow in the bottom. Reproduced from [Citation340], Copyright 2020, with permission from Elsevier.
![Figure 11. Illustration of machine learning applications to the analysis and enhancement of MD simulations. High dimensional data, e.g. the protein trajectory, is used as the input of an artificial neural network (ANN). The ANN is trained to project the input to a low dimensional space minimising a loss function. Depending on the structure of the ANN and on the loss function, the low dimensional representation captures different features that are considered to be important, such as slow modes. In some methods, the features learnt are used to further enhance the sampling of MD simulation as shown by the arrow in the bottom. Reproduced from [Citation340], Copyright 2020, with permission from Elsevier.](/cms/asset/8cb42977-a2e4-4b70-8859-06eab8fde06b/tapx_a_2080587_f0011_oc.jpg)
Powerful hybrid techniques arise not only from the combination of different enhanced sampling techniques but also from the combination of simulation methods with different resolutions. These methods are particularly important when a part of the system needs to be treated with atomistic detail while the rest of the system is very large and just acts as the environment of the relevant part, so that it is more convenient to treat it at a coarse-grained level. This situation is particularly relevant for the study of ion channels. For instance, the simulation of a whole vesicle is computationally very expensive so that membrane lipids could be described using a CG model while the ion channel or the few membrane proteins of interest could be described at atomistic level. This would also account for the fact that the MARTINI force field currently models lipids more accurately than proteins. Such a framework is provided, e.g., by the adaptive resolution scheme (AdResS) in its different flavours [Citation333–335] where the atomistic and coarse grained domains are connected by an intermediate region that allows for a continuous transition between the two domains. The passage between regions modeled at different resolutions is described by a sigmoid transition function that grows monotonically from zero (CG region) to one (atomistic region) taking values
in the hybrid region. Assuming a mono-dimensional case, the transition function allows the calculation of the force acting on residue
:
where the atomistic and coarse grained force
are just the negative gradients of the corresponding potentials
with and
the position vectors of atom
of residue
and atom
of residue
while
and
are the position vectors of the center of mass of residues
and
. It is important to note that the equations of state of the atomistic and coarse grained systems are very different so that a given value of the density corresponds to different values of the pressure. Therefore, in order to simulate the multiscale system at uniform density without dissipating the pressure gradient between the atomistic and coarse grained domains, it is necessary to add a thermodynamic force (the term
in EquationEquation (36)
(36)
(36) ). This force is determined iteratively starting from the initial choice
. The method has been initially used [Citation336] for the calculation of chemical potentials and solvation-free energies of molecular systems. For instance, a characterization of the solvation shell of fullerenes revealed that surface-induced structuring of water does not go beyond the second hydration layer. The AdResS method, however, is extremely flexible and recent applications include quantum-classical coupling [Citation337], non-equilibrium systems [Citation338], and advanced free-energy calculations [Citation339].
A class of techniques that is finding increasing application in the arena of biomolecular simulation is represented by Machine Learning (ML). ML is leading a revolution in all fields of science and technology with applications ranging from image and speech recognition to the prediction of drug action. In particular, we will discuss the application of deep feedforward neural networks [Citation341]. A neural network is just a set of activating functions called neurons and organized in layers (). A neuron in a layer receives as input the weighted sum of the outputs of the previous layer, transforms this total input through a non-linear function, and passes the output to the next layer. The most important feature of deep neural networks is that, through a training stage, they can automatically ‘learn’, that is, adjust their weights to fit any function of interest. Indeed, the error function, quantifying the distance between the solution proposed by the machine and the correct one, can be seen as a multidimensional function of the weights and can be minimized through an algorithm similar to steepest descent called stochastic gradient descent [Citation342]. In order to apply machine learning to biomolecular calculations the neural network must be fed with inputs respecting the physical constraints [Citation343]. For instance, if we consider an oxygen molecule in vacuo with a six-dimensional coordinate vector , the energy will be invariant to roto-translation, while the force will be equivariant:
where is a translation vector and
is a rotation matrix. In order to retain the invariances and equivariances, the network should not be fed with a vector of cartesian coordinates but with a set of distances and angles which significantly reduces the dimensionality of the problem. Other restraints that should be accounted for are: permutational invariance, for example, of solvent molecules, probability conservation, and detailed balance. ML can be applied to a wide range of problems of interest for biomolecular simulation. The list we provide below has no claim to be exhaustive.
Calculation of potential energy surfaces. A number of classical methods already exists [Citation344,Citation345] to fit energy values derived from quantum mechanical calculations, but these often suffer from poor transferability. A viable alternative is to train a deep neural network to minimize an energy or a force loss function [Citation346,Citation347]:
where is the energy predicted by the network for configuration
using parameter vector
, while
is the energy computed ab initio for the same conformation. In a similar way,
is the force predicted by the neural network and
is the force computed through quantum mechanics. This approach can be used to optimize the parameters of a force field.
(2) Calculation of free-energy surfaces. This problem is formally similar to the estimate of Potential Energy Surfaces, but the training is done on classical MD trajectories of the rare event of interest. Indeed, the neural network [Citation348] must be trained to minimize the free-energy loss
where is the free energy predicted by the network using parameter vector
at point
in the space of collective variables while
is the free energy of the same configuration computed with a method able to sample the equilibrium distribution
:
.
(3) Coarse graining. ML can be used to learn the effective energy of a coarse grained molecular model [Citation349,Citation350]. Let us consider a transformation
that associates a vector of cartesian coordinates
to a vector of coordinates of the coarse grained particles
with
. The transformation is assumed to be linear because normally the CG coordinates are a subset of the atomistic ones. For instance the CG particle representing a residue is normally placed in the position of the
atom of that residue. The effective energy of the CG model is given by the free energy as a function of variables
:
. If the coarse grained model is to be thermodynamically consistent with the atomistic one, the force predicted by the neural network
must match the force on CG particles
computed from the atomistic forces
. The loss function that the network must learn to minimize is thus
where the sum runs over all conformations of a training set.
(4) Automatic discovery of Collective Variables. As discussed, many enhanced sampling methods critically rely on the appropriate choice of CVs. It is thus important to clarify what is meant by ‘good’ collective variables [Citation86]. Good CVs should correspond to the important dynamical motions of the system so that a simulation biased along these CVs should overcome the free-energy barriers (rarely sampled in unbiased simulations) separating the relevant functional states. Given the complexity of biomolecular systems, identifying the good CVs through chemical intuition is often a daunting task. This is why a number of numerical techniques have been designed for the automatic identification of good CVs. A first group of techniques seeks to project a MD trajectory in a lower dimensional space spanned by base vectors aligned along the directions of maximal variance. This is the case, for example, of the popular Principal Component Analysis (PCA) technique [Citation351]. A second class of methods looks for a low-dimensional CV space spanned by vectors aligned along the directions of maximal auto-correlation. These directions correspond to the slowest molecular motions most likely capturing the functional dynamics of the protein. A typical representative of this class of techniques is the Time-lagged Independent Component Analysis (TICA) [Citation352]. Both families of techniques can benefit from the fitting power of deep neural networks.
An example of application of neural networks to the discovery of high-variance CVs is the Molecular Enhanced Sampling with Autoencoders (MESA) [Citation353]. The MESA method requires a neural network with a special architecture including an encoder that maps the 3N-dimensional vector of cartesian coordinates to a lower dimensional vector of CVs,
, and a decoder that approximates the reverse mapping from
to
. The network is trained to reconstruct its input, that is, the weights are tuned so that
. The algorithm performs cycles of CV identification and biased simulations using the discovered CVs until the value of the CVs remains stable. The method was successfully applied to the alanine dipeptide and Trp-cage systems [Citation353,Citation354].
We now provide an example of discovery of maximally autocorrelated CVs. This approach was originally developed by Ferguson and coworkers [Citation87] who named it State-free Reversible Variational Approach to Markov Processes Networks, and further improved by Parrinello’s group (deepTICA) [Citation88]. The approach is based on the observation that a Molecular Dynamics simulation can be considered as a dynamical process that evolves a density distribution at time
towards the Boltzmann distribution
. Mathematically, the evolution of the density distribution is performed by the transfer operator
. The eigenfunctions associated to the largest eigenvalues of the transfer operator are characterized by the slowest relaxation to equilibrium and are thus good CV candidates. In complex biomolecular systems, the analytical diagonalization of the transfer operator is not possible. However, exploiting a variational approach, it is possible to compute lower bound approximations
of the true eigenvalues
and the corresponding approximate eigenfunctions
. To further simplify the calculation, the variational eigenfunctions
are expressed as a linear combination of trial descriptors
and the set of parameters is sought that maximizes their autocorrelation (thus also maximizing
). This leads to a generalized eigenvalue problem
where
The neural network is fed with pairs of time-lagged descriptors ,
that are mapped to their latent space counterparts
and
, where
is a set of parameters. The solution of the generalized eigenvalue problem using the latent space variables leads to an estimate of the
parameters. The network is then trained to minimize the loss function
. Once the loss function is optimized, the deep-TICA collective variables can be computed as
and an enhanced sampling simulation can be run biasing these CVs.
8. Conclusions
The discussion in this review shows that simulation methods are powerful tools for the analysis of biomolecular systems and, in particular, for ion channels. Specifically, molecular dynamics simulations just need three ingredients: an initial state, a reliable force field, and suitable algorithms for the numerical integration of Newton’s equations of motion. In principle, these few elements should suffice to fully reproduce the dynamical behaviour of biomolecules and their function, in agreement with the 1812 statement by Pierre Simon de Laplace [Citation355]:
… an intelligence which could comprehend all the forces by which nature is animated and the respective positions of the beings which compose it, if moreover this intelligence were vast enough to submit these data to analysis … to it nothing would be uncertain, and the future as the past would be present to its eyes.
Unfortunately, as discussed above, both continuum and atomistic methods suffer from a number of limitations and Laplace’s optimism ought to give way to a more cautious attitude along the lines of the 1929 statement by Dirac [Citation356]:
The underlying physical laws necessary for the mathematical theory of a large part of physics and the whole of chemistry are thus completely known, and the difficulty is only that the exact application of these laws leads to equations much too complicated to be soluble. It therefore becomes desirable that approximate practical methods of applying quantum mechanics should be developed, which can lead to an explanation of the main features of complex atomic systems without too much computation.
What Dirac said about quantum mechanics also applies to classical molecular simulations and still resists the test of time, with the notable exception that much computer power seems always in high demand. This review, highlighting strengths and weaknesses of the different methods in computational ion channels research, can guide the reader in the choice of the most appropriate approach to the problem of interest. Moreover, we selected a few rapidly developing research lines ranging from hybrid enhanced sampling methods to multi-scale algorithms and machine learning that will enable to by-pass the problem of ‘too much computation’. While a perfect knowledge of ‘the future as the past’ is still precluded to mankind even in the restricted area of quantitative sciences, a clever application of the approaches discussed here will certainly push forward the boundary of our quantitative understanding of biology.
Acknowledgments
We thank G. Ciccotti for inspiring discussions at FICN2021 and for encouragement. MLB is grateful to W.A.T. Gibby, D.G. Luchinsky, P.V.E. McClintock, and A. Smolyanitsky for valuable discussions. LC, GC, and LM thank T. Malliavin for the long and fruitful collaboration on the 7 nicotinic receptor. CG and AG thank F. Costa for useful discussions on the hERG channel.
Disclosure statement
No potential conflict of interest was reported by the author(s). Nundefined
Additional information
Funding
Notes
1. We recall that the Reynolds number quantifies the relative magnitude of inertial to viscous forces.
2. These equations refer to a system with constant number of particles, volume, and energy. Interactions of the (small) simulated system with external thermal baths (constant temperature), pistons (constant pressure), mass exchange, etc. can be accounted for by suitably modifying the equations of motion such that the correct probability distribution is sampled [Citation357].
References
- Doyle D, Morais Cabral J, Pfuetzner R, et al. The structure of the potassium channel: molecular basis of K+ conduction and selectivity. Science. 1984;280:69–74.
- Hille B. Ionic channels of excitable membranes. Sunderland Massachussets: Sinauer Associates Inc. Publishers; 1992.
- Imbrici P, Liantonio A, Camerino GM, et al. Therapeutic approaches to genetic ion channelopathies and perspectives in drug discovery. Front Pharmacol. 2016;7:121.
- Morais-Cabral J, Zhou Y, MacKinnon R. Energetic optimization of ion conduction rate by the K+ selectivity filter. Nature. 2001;414:37–42.
- Nogueira J, Corry B. Ion channel permeation and selectivity. In: The Oxford handbook of neuronal ion channels. Edited by Arin Bhattacharjee, Oxford University Press, Oxford, UK. 2019.
- Zhou M, Morais-Cabral JH, Mann S, et al. Potassium channel receptor site for the inactivation gate and quaternary amine inhibitors. Nature. 2001;411:657–661.
- Humphrey W, Dalke A, Schulten K. VMD: visual molecular dynamics. J Mol Graphics. 1996;14:33–38.
- Zhou Y, MacKinnon R. The occupancy of ions in the K+ selectivity filter: charge balance and coupling of ion binding to a protein conformational change underlie high conduction rates. J Mol Biol. 2003;333:965–975.
- Aqvist J, Luzhkov V. Ion permeation mechanism of the potassium channel. Nature. 2000;404:881–884.
- Berneche S, Roux B. Energetics of ion conduction through the K+ channel. Nature. 2001;414:73–77.
- Chung SH, Allen TW, Kuyucak S. Conducting-state properties of the KcsA potassium channel from molecular and Brownian dynamics simulations. Biophys J. 2002;82:628–645.
- Khalili-Aragi F, Tajkhorshid E, Schulten K. Dynamics of K+ ion conduction through Kv1.2. Biophys J. 2006;91:L72–L74.
- Jensen M, Borhani D, Lindorff-Larsen K, et al. Principles of conduction and hydrophobic gating in K+ channels. Proc Natl Acad Sci USA. 2010;107:5833–5838.
- Jensen MO, Jogini V, Borhani DW, et al. Mechanism of voltage gating in potassium channels. Science. 2012;336:229–233.
- Furini S, Domene C. Atypical mechanism of conduction in potassium channels. Proc Natl Acad Sci USA. 2009;106:16074–16077.
- Kopfer D, Song C, Gruene T, et al. Ion permeation in K+ channels occurs by direct Coulomb knock-on. Science. 2014;346:352–355.
- LeMasurier M, Heginbotham L, Miller CK. KcsA: It’s a potassium channel. J Gen Physiol. 2001;118:303–313.
- Bezanilla F, Armstrong C. Negative conductance caused by entry of sodium and cesium ions into the potassium channels of squid axons. J Gen Physiol. 1972;60:588–608.
- Bernèche S, Roux B. Molecular dynamics of the KcsA K+ channel in a bilayer membrane. Biophys J. 2000;78:2900–2917.
- Noskov S, Berneche S, Roux B. Control of ion selectivity in potassium channels by electrostatic and dynamic properties of carbonyl ligands. Nature. 2004;431:830–834.
- Dixit PD, Merchant S, Asthagiri D. Ion selectivity in the KcsA potassium channel from the perspective of the ion binding site. Biophys J. 2009 ;96:2138–2145.
- Thomas M, Jayatilaka D, Corry B. The predominant role of coordination number in potassium channel selectivity. Biophys J. 2007;93:2635–2643.
- Bostick DL, Brooks CL. Selectivity in K+ channels is due to topological control of the permeant ion’s coordinated state. Proc Natl Acad Sci USA. 2007;104:9260–9265.
- Yu H, Noskov SY, Roux B. Two mechanisms of ion selectivity in protein binding sites. Proc Natl Acad Sci USA. 2010 ;107:20329–20334.
- Thompson AN, Kim I, Panosian TD, et al. Mechanism of potassium-channel selectivity revealed by Na+ and Li+binding sites within the KcsA pore. Nat Struct Mol Biol. 2009;16:1317–1324.
- Roux B, Bernèche S, Egwolf B, et al. Ion selectivity in channels and transporters. J Gen Physiol. 2011 ;137:415–426.
- Corry B. Mechanisms of selective ion transport and salt rejection in carbon nanostructures. MRS Bull. 2017;42:306–310.
- Barabash ML, Gibby WAT, Guardiani C, et al. Origin and control of ionic hydration patterns in nanopores. Commun Mater. 2021;2:65.
- Horn R, Roux B, Aqvist J. Permeation redux: thermodynamics and kinetics of ion movement through potassium channels. Biophys J. 2014;106:1859–1863.
- Gibby WAT, Barabash ML, Guardiani C, et al. Physics of selective conduction and point mutation in biological ion channels. Phys Rev Lett. 2021;126:218102.
- Fowler P, Tai K, Sansom M. The selectivity of K+ ion channels: testing the hypotheses. Biophys J. 2008;95:5062–5072.
- Tang L, Gamal El-Din T, Payandeh J, et al. Structural basis for Ca2+ selectivity of a voltage-gated calcium channel. Nature. 2014;505:56–61.
- Dang T, McCleskey E. Ion channel selectivity through stepwise changes in binding affinity. J Gen Physiol. 1998;111:185–193.
- Bass R, Strop P, Barclay M, et al. Crystal structure of Escherichia coli MscS, a voltage-modulated and mechanosensitive channel. Science. 2002;298:1582–1587.
- Lamas J, Rueda-Ruzafa L, Herrera-Perez S. Ion channels and thermosensitivity: TRP, TREK, or both? Int J Mol Sci. 2019;20:2371.
- Caterina MJ, Schumacher MA, Tominaga M, et al. The capsaicin receptor: a heat-activated ion channel in the pain pathway. Nature. 1997;389:816–824.
- Coste B, Mathur J, Schmidt M, et al. Piezo1 and Piezo2 are essential components of distinct mechanically activated cation channels. Science. 2010;330:55–60.
- Aryal P, Sansom M, Tucker S. Hydrophobic gating in ion channels. J Mol Biol. 2015;427:121–130.
- Beckstein O, Biggin P, Sansom M. A hydrophobic gating mechanism for nanopores. J Phys Chem B. 2002;105:12902–12905.
- Allen R, Melchionna S, Hansen J. Intermittent permeation of cylindrical nanopores by water. Phys Rev Lett. 2002;89:175502.
- Anishkin A, Sukharev S. Water dynamics and dewetting transitions in the small mechanosensitive channel MscS. Biophys J. 2004;86:2883–2895.
- Anishkin A, Sukharev S. Pore opening and closing of a pentameric ligand-gated ion channel. Proc Natl Acad Sci USA. 2010;107:19814–19819.
- Jia Z, Yazani M, Zhang G, et al. Hydrophobic gating in BK channels. Nat Commun. 2018;9:3408.
- Roth R, Gillespie D, Nonner W, et al. Bubbles, gating, and anesthetics in ion channels. Biophys J. 2008;94:4282–4298.
- Giacomello A, Roth R. Bubble formation in nanopores: a matter of hydrophobicity, geometry, and size. Adv Phys X. 2020;5:1817780.
- Rao S, Klesse G, Stansfeld P, et al. A heuristic derived from analysis of the ion channel structural proteome permits the rapid identification of hydrophobic gates. Proc Natl Acad Sci USA. 2019;116:13989–13995.
- Camisasca G, Tinti A, Giacomello A. Gas-induced drying of nanopores. J Phys Chem Lett. 2020;11:9171–9177.
- Barros F, Pardo L, Dominguez P, et al. New structures and gating of voltage-dependent potassium (Kv) channels and their relatives: a multi-domain and dynamic question. Int J Mol Sci. 2019;20:248.
- Ghosh A, Vishveshwara S. A study of communication pathways in methionyl-tRNA synthetase by molecular dynamics simulations and structure network analysis. J Phys Chem B. 2007;104:15711–15716.
- Pasi M, Tiberti M, Arrigoni A, et al. xPyder: a PyMOL plugin to analyze coupled residues and their networks in protein structures. J Chem Inf Model. 2012;52:1865–1874.
- Fernandez-Marino A, Harpole T, Oelstrom K, et al. Gating interaction maps reveal a noncanonical electromechanical coupling mode in the shaker K+ channel. Nat Struct Mol Biol. 2018;25:320–326.
- Costa F, Guardiani C, Giacomello A. Molecular dynamics simulations suggest possible activation and deactivation pathways in hERG channel. Commun Biol. 2022;5:165.
- Carvalho-de Souza J, Bezanilla F. Noncanonical mechanism of voltage sensor coupling to pore revealed by tandem dimers of Shaker. Nat Commun. 2019;10:1–12.
- Maglia G, Rincon Restrepo M, Mikhailova E, et al. Enhanced translocation of single DNA molecules through α-hemolysin nanopores by manipulation of internal charge. Proc Natl Acad Sci USA. 2019;105:19720–19725.
- Koçer A, Walko M, Meijberg W, et al. A light-actuated nanovalve derived from a channel protein. Science. 2005;309:755–758.
- Trick JL, Wallace EJ, Bayley H, et al. Designing a hydrophobic barrier within biomimetic nanopores. ACS Nano. 2014;8:11268–11279.
- Cosentino C, Alberio L, Gazzarrini S, et al. Engineering of a light-gated potassium channel. Science. 2015;348:707–710.
- Dekker C. Solid-state nanopores. Nat Nanotechnol. 2007;2:209–215.
- Siwy ZS, Howorka S. Engineered voltage-responsive nanopores. Chem Soc Rev. 2010;39:1115–1132.
- Hou X, Guo W, Jiang L. Biomimetic smart nanopores and nanochannels. Chem Soc Rev. 2011;40:2385–2401.
- Kocer A, Tauk L, Dejardin P. Nanopore sensors: from hybrid to abiotic systems. Biosens Bioelectron. 2012;38:1–10.
- Lepoitevin M, Ma T, Bechelany M, et al. Functionalization of single solid state nanopores to mimic biological ion channels: a review. Adv Colloid Interface Sci. 2017;250:195–213.
- Guardiani C, Gibby W, Barabash M, et al. Exploring the pore charge dependence of K+ and Cl− permeation across a graphene monolayer: a molecular dynamics study. RSC Adv. 2019;9:20402.
- Sint K, Wang B, Kral P. Selective ion passage through functionalized graphene nanopores. J Am Chem Soc. 2008;130:16448–16449.
- Sint K, Wang B, Kral P. Selective ion penetration of graphene oxide membranes. ACS Nano. 2013;7:428–437.
- Cohen-Tanugi D, Grossman J. Water desalination across nanoporous graphene. Nano Lett. 2012;12:3602–3608.
- O’Hern S, Jang D, Bose S, et al. Nanofiltration across defect-sealed nanoporous monolayer graphene. Nano Lett. 2015;15:3254–3260.
- He Z, Zhou J, Lu X, et al. Bioinspired graphene nanopores with voltage-tunable ion selectivity for Na+ and K+. ACS Nano. 2013;7:10148–10157.
- Hodgkin A, Huxley A. A quantitative description of membrane current and its application to conduction and excitation in nerve. J Physiol. 1952;117:500–544.
- Sakmann B, Neher E. Patch clamp techniques for studying ionic channels in excitable membranes. Annu Rev Physiol. 1984;46:455–472.
- Cheng Y, Glaeser R, Nogales E. How cryo-EM became so hot. Cell. 2017;171:1229–1231.
- Sterling T. Beowulf clusters computing with Linux. Cambridge, Massachusetts: MIT Press; 2001.
- TOP500. 2021 Nov [cited 2022 Jan 03]. Available from: https://www.top500.org/lists/top500/2021/11/2021
- Dill K, Bromberg S. Molecular driving forces: statistical thermodynamics in biology, chemistry, physics, and nanoscience. 2nd ed. New York: Garland Science; 2010.
- Jackson M. Molecular and cellular biophysics. New York, Melbourne, Madrid, Cape Town, Singapore, Sao Paulo: Cambridge; 2006. Cambridge University Press.
- Malgaretti P, Janssen M, Pagonabarraga I, et al. Driving an electrolyte through a corrugated nanopore. J Chem Phys. 2019;151:084902.
- Dror RO, Dirls RM, Grosssman JP, et al. Biomolecular simulation: a computational microscope for molecular biology. Annu Rev Biophys. 2012;41:429–452.
- Harvey M, Giupponi G, De Fabritiis G. ACEMD: accelerating biomolecular dynamics in the microsecond timescale. J Chem Theory Comput. 2009;5:1632–1639.
- Shaw D, Deneroff M, Dror R, et al. Anton, a special-purpose machine for molecular dynamics simulation. Commun ACM. 2008;51:91–97.
- Bonella M, Meloni S, Ciccotti G. Theory and methods for rare events. Eur Phys J B. 2012;85:97.
- Sugita Y, Okamoto Y. Replica-exchange molecular dynamics method for protein folding. Chem Phys Lett. 1999;314:141–151.
- Bussi G, Gervasio F, Laio A, et al. Free-energy landscape for beta hairpin folding from combined parallel tempering and metadynamics. J Am Chem Soc. 2006;128:13435–13441.
- Piana S, Laio A. A bias-exchange approach to protein folding. J Phys Chem B. 2007;111:4553.
- Bolhuis P, Chandler D, Dellago C, et al. Transition paths sampling : throwing ropes over rough mountain passes, in the dark. Annu Rev Phys Chem. 2002;53:291–318.
- Maragliano L, Fischer A, Vanden-Eijnden E, et al. String method in collective variables: minimum free energy paths and isocommittor surfaces. J Chem Phys. 2006;125:024106.
- Sidky H, Chen W, Ferguson AL. Machine learning for collective variable discovery and enhanced sampling in biomolecular simulation. Mol Phys. 2020;118:e1737742.
- Chen W, Sidky H, Ferguson A. Nonlinear discovery of slow molecular modes using state-free reversible VAMPnets. J Chem phys. 2019;150:214114.
- Bonati L, Piccini G, Parrinello M. Deep learning the slow modes for rare events sampling. Proc Natl Acad Sci USA. 2021;118:e2113533118.
- Frontiers in ion channels and nanopores: theory, experiments, and simulation; 2021 [cited 2022 Jan 10]. Available from: https://sites.google.com/uniroma1.it/ficn2021
- Shi W, Friedman AK, Baker LA. Nanopore sensing. Anal Chem. 2017;89:157–188.
- Humplik T, Lee J, O’Hern S, et al. Nanostructured materials for water desalination. Nanotechnology. 2011;22:292001.
- Eroshenko V, Regis RC, Soulard M, et al. Energetics: a new field of applications for hydrophobic zeolites. J Am Chem Soc. 2001;123:8129–8130.
- Bruus H. Theoretical microfluidics. Vol. 18. New York: Oxford university press; 2007.
- de Groot SR, Mazur P. Non-equilibrium thermodynamics. New York: Dover; 1984.
- Wendt R. Simplified transport theory for electrolyte solutions. J Chem Educ. 1974;51:646.
- Kurnikova M, Coalson R, Graf P, et al. A lattice relaxation algorithm for three-dimensional Poisson-Nernst-Planck theory with application to ion transport through the gramicidin A channel. Biophys J. 1999;76:642–656.
- Im W, Roux B. Ion permeation and selectivity of OmpF porin: a theoretical study based on molecular dynamics, Brownian dynamics, and continuum electrodiffusion theory. J Mol Biol. 2002;322:851–869.
- Coalson RD, Kurnikova MG. Poisson-Nernst-Planck theory approach to the calculation of current through biological ion channels. IEEE Trans Nanobioscience. 2005;4:81–93.
- Chen D, Lear J, Eisenberg R. Permeation through an open channel: Poisson-Nernst-Planck theory of a synthetic ionic channel. Biophys J. 1997;72:97–116.
- Corry B, Kuyucak S, Chung SH. Tests of continuum theories as models of ion channels. II. Poisson–Nernst–Planck theory versus brownian dynamics. Biophys J. 2000;78:2364–2381.
- Noskov SY, Im W, Roux B. Ion permeation through the alpha-hemolysin channel: theoretical studies based on Brownian dynamics and Poisson-Nernst-Planck electrodiffusion theory. Biophys J. 2004;87:2299–2309.
- Liu JL, Eisenberg B. Poisson-Nernst-Planck-Fermi theory for modeling biological ion channels. J Chem Phys. 2014;141:22D532.
- Liu W. One-dimensional steady-state Poisson–Nernst–Planck systems for ion channels with multiple ion species. J Differ Equations. 2009;246:428–451.
- Corry B, Kuyucak S, Chung SH. Dielectric self-energy in Poisson-Boltzmann and Poisson-Nernst-Planck models of ion channels. Biophys J. 2003;84:3594–3606.
- Gillespie D, Nonner W, Eisenbers R. Coupling Poisson-Nernst-Planck and density functional theory to calculate ion flux. J Phys. 2002;14:12129.
- Howorka S, Siwy Z. Nanopore analytics: sensing of single molecules. Chem Soc Rev. 2009;38:2360–2384.
- Chinappi M, Cecconi F. Protein sequencing via nanopore based devices: a nanofluidic perspective. J Phys. 2018;30:204002.
- Bayley H, Braha O, Gu LQ. Stochastic sensing with protein pores. Adv Mater. 2000;12:139–142.
- Cressiot B, Greive S, Si W, et al. Porphyrin-assisted docking of a thermophage portal protein into lipid bilayers: nanopore engineering and characterization. Adv Mater. 2017;11:11931–11945.
- Zhang S, Huang G, Versloot RCA, et al. Bottom-up fabrication of a proteasome–nanopore that unravels and processes single proteins. Nat Chem. 2021;13:1192–1199.
- Muthukumar M. Polymer translocation. Taylor & Francis group, Boca Raton, USA: CRC press; 2016.
- Asandei A, Schiopu I, Chinappi M, et al. Electroosmotic trap against the electrophoretic force near a protein nanopore reveals peptide dynamics during capture and translocation. ACS Appl Mater Interfaces. 2016;8:13166–13179.
- Huang G, Willems K, Soskine M, et al. Electro-osmotic capture and ionic discrimination of peptide and protein biomarkers with frac-nanopores. Nat Commun. 2017;8:935.
- Boukhet M, Piguet F, Ouldali H, et al. Electrophoresis versus electroosmosis. Nanoscale. 2016;8:18352–18359.
- Chinappi M, Yamaji M, Kawano R, et al. Analytical model for particle capture in nanopores elucidates competition among electrophoresis, electroosmosis, and dielectrophoresis. ACS nano. 2020;14:15816–15828.
- Grosberg A, Rabin Y. DNA capture into a nanopore: interplay of diffusion and electrohydrodynamics. J Chem Phys. 2010;133:10B617.
- Larkin J, Henley R, Muthukumar M, et al. High-bandwidth protein analysis using solid-state nanopores. Biophys J. 2014;106:696–704.
- Malgaretti P, Pagonabarraga I, Rubi J. Entropically induced asymmetric passage times of charged tracers across corrugated channels. J Chem Phys. 2016;144:034901.
- Malgaretti P, Pagonabarraga I, Rubi J. Geometrically tuned channel permeability. Macromol Symp. 2015;357:178.
- Hummer G, Soumpasis DM. Computation of the water density distribution at the ice-water interface using the potentials-of-mean-force expansion. Phys Rev E. 1994;49:591–596.
- Hummer G, Garca AE, Soumpasis DM. Hydration of nucleic acid fragments: comparison of theory and experiment for high-resolution crystal structures of RNA, DNA, and DNA-drug complexes. Biophys J. 1995;68:1639–1652.
- Gray CG, Gubbins KE. Theory of molecular fluids. Vol. 1. Oxford, UK: Oxford University Press; 1984.
- Kirkwood JG. Statistical mechanics of fluid mixtures. J Chem Phys. 1935;3:300–313.
- Evans R. The nature of the liquid-vapour interface and other topics in the statistical mechanics of non-uniform, classical fluids. Adv Phys. 1979;28:143–200.
- Marini Bettolo Marconi U, Tarazona P. Density functional theory of fluids. J Chem Phys. 1999;110:8032.
- Schmidt M, Brader J. Power functional theory for Brownian dynamics. J Chem Phys. 2013;138:214101.
- te Vrugt M, Löwen H, Wittkowski R. Classical dynamical density functional theory: from fundamentals to applications. Adv Phys. 2020;69:121–247.
- Gußmann F, Roth R. Bubble gating in biological ion channels: a density functional theory study. Phys Rev E. 2017;95:062407.
- Peyser A, Gillespie D, Roth R, et al. Domain and interdomain energetics underlying gating in Shaker-type Kv channels. Biophys J. 2014;107:1841–1852.
- Roth R, Gillespie D. Physics of size selectivity. Phys Rev Lett. 2005;95:247801.
- Gillespie D, Nonner W, Henderson D, et al. A physical mechanism for large-ion selectivity of ion channels. Phys Chem Chem Phys. 2002;4:4763–4769.
- Gillespie D, Xu L, Meissner G. Selecting ions by size in a calcium channel: the ryanodine receptor case study. Biophys J. 2014;107:2263–2273.
- Bodrenko I, Salis S, Acosta-Gutierrez S, et al. Diffusion of large particles through small pores: from entropic to enthalpic. J Chem Phys. 2019;150:211102.
- Coines J, Acosta-Gutierrez S, Bodrenko I, et al. Glucose transport via the pseudomonad porin OprB: implications for the design of Trojan Horse anti-infectives. Phys Chem Chem Phys. 2019;21:8457–8463.
- Bodrenko IV, Milenkovic S, Ceccarelli M. Diffusion of molecules through nanopores under confinement: time-scale bridging and crowding effects via Markov state model. Biomol Concepts. 2022;13:207–219.
- Ghai I, Pira A, Scorciapino M, et al. General method to determine the flux of charged molecules through nanopores applied to β-lactamase inhibitors and OmpF. J Phys Chem Lett. 2017;8:1295–1301.
- Lee A, Elam JW, Darling SB. Membrane materials for water purification: design, development, and application. Environ Sci. 2016;2:17–42.
- Graf M, Lihter M, Altus D, et al. Transverse detection of DNA using a MoS2 nanopore. Nano Lett. 2019;19:9075–9083.
- Macha M, Marion S, Nandigana VVR, et al. 2D materials as an emerging platform for nanopore-based power generation. Nat Rev Mater. 2019;4:588–605.
- Heerema SJ, Dekker C. Graphene nanodevices for DNA sequencing. Nat Nanotechol. 2016;11:127–136.
- Yuan Z, Liu Y, Dai M, et al. Controlling DNA translocation through solid-state nanopores. Nanoscale Res Lett. 2020;15:1–9.
- de Pablo JJ, Jackson NE, Webb MA, et al. New frontiers for the materials genome initiative. Npj Comput Mater. 2019;5:41.
- Marbach S, Dean DS, Bocquet L, et al. Transport and dispersion across wiggling nanopores. Nat Phys. 2018;14:1108–1113.
- Martin NE, Malik S, Calimet N, et al. Un-gating and allosteric modulation of a pentameric ligand-gated ion channel captured by molecular dynamics. PLoS Comput Biol. 2017;13:e1005784.
- Rao S, Klesse G, Lynch CI, et al. Molecular simulations of hydrophobic gating of pentameric ligand gated ion channels: insights into water and ions. J Phys Chem A. 2021;125:981–994.
- Jekhmane S, Medeiros-Silva J, Li J, et al. Shifts in the selectivity filter dynamics cause modal gating in K+ channels. Nat Commun. 2019;10:1–12.
- Ulmschneider JP, Ulmschneider MB. Molecular dynamics simulations are redefining our view of peptides interacting with biological membranes. Acc Chem Res. 2018;51:1106–1116.
- Allen M, Tildesley D. Computer simulation of liquids. Oxford: Clarendon Press; 1991.
- Dror RO, Jensen MØ, Borhani DW, et al. Exploring atomic resolution physiology on a femtosecond to millisecond timescale using molecular dynamics simulations. J Gen Physiol. 2010;135:552–562.
- Carnevale V, Delemotte L, Howard RJ. Molecular dynamics simulations of ion channels. Trends Biochem Sci. 2021;46:621–622.
- Flood E, Boiteux C, Lev B, et al. Atomistic simulations of membrane ion channel conduction, gating, and modulation. Chem Rev. 2019;119:7737–7832.
- Casalino L, Gaieb Z, Goldsmith JA, et al. Beyond shielding: the roles of glycans in the SARS-CoV-2 spike protein. ACS Cent Sci. 2020;6:1722–1734.
- Ponder J, Case D. Force fields for protein simulations. Adv Prot Chem. 2003;66:27–85.
- Huang J, MacKerell ADJ. CHARMM36 all-atom additive protein force field: validation based on comparison to NMR data. J Comput Chem. 2013;34:2135–2145.
- Jorgensen WL, Maxwell DS, Tirado-Rives J. Development and testing of the OPLS all-atom force field on conformational energetics and properties of organic liquids. J Am Chem Soc. 1996;118:11225–11236.
- Schmid N, Eichenberger A, Choutko A, et al. Definition and testing of the GROMOS force-field versions 54A7 and 54B7. Eur Biophys J. 2011;40:843–856.
- Wang J, Wolf R, Caldwell J, et al. Development and testing of a general amber force field. J Comput Chem. 2004;25:1157–1174.
- Vanommeslaeghe K, Hatcher E, Acharya C, et al. CHARMM general force field (CGenFF): a force field for drug-like molecules compatible with the CHARMM all-atom additive biological force fields. J Comput Chem. 2010;31:671–690.
- Wang J, Wang W, Kollman P, et al. Automatic atom type and bond type perception in molecular mechanical calculations. J Mol Graphics Modell. 2006;25:247260.
- Betz R, Walker RP. Automated optimization of force field parameters for molecular dynamics simulations. J Comput Chem. 2014;36:79–87.
- Huang L, Roux B. Automated force field parameterization for non-polarizable and polarizable atomic models based on ab initio target data. J Chem Theory Comput. 2013 ;9:3543–3556.
- Boulanger E, Huang L, Rupakheti C, et al. Optimized Lennard-Jones parameters for druglike small molecules. J Chem Theory Comput. 2018 ;14:3121–3131.
- Furini S, Domene C. Critical assessment of common force fields for molecular dynamics simulations of potassium channels. J Chem Theory Comput. 2020;16:7148–7159.
- Lynch CI, Klesse G, Rao S, et al. Water nanoconfined in a hydrophobic pore: molecular dynamics simulations of transmembrane protein 175 and the influence of water models. ACS nano. 2021;15:19098–19108.
- Hess B, Bekker H, Berendsen HJC, et al. LINCS: a linear constraint solver for molecular simulations. J Comp Chem. 1997;18:1463–1472.
- Hopkins CW, Grand SL, Walker RC, et al. Long-time-step molecular dynamics through hydrogen mass repartitioning. J Chem Theory Comput. 2015;11:1864–1874.
- Berman H, Westbrook J, Feng Z, et al. The protein data bank. Nucleic Acids Res. 2000;28:235–242.
- Roux B. The membrane potential and its representation by a constant electric field in computer simulations. Biophys J. 2008;95:4205–4216.
- Gumbart J, Khalili-Aragi F, Sotomayor M, et al. Constant electric field simulations of the membrane potential illustrated with simple systems. BBA - Biomembr. 2012;1818:294–302.
- Khalili-Araghi F, Ziervogel B, Gumbart JC, et al. Molecular dynamics simulations of membrane proteins under asymmetric ionic concentrations. J Gen Physiol. 2013;142:465–475.
- Sachs J, Crozier P, Woolf T. Atomistic simulations of biologically realistic transmembrane potential gradients. J Chem Phys. 2004 ;121:10847–10851.
- Kutzner C, Grubmuller H, de Groot B, et al. Computational electrophysiology: the molecular dynamics of ion channel permeation and selectivity in atomistic detail. Biophys J. 2011;101:809–817.
- Cerdan AH, Cecchini M. On the functional annotation of open-channel structures in the glycine receptor. Structure. 2020;28:690–693.
- Kopec W, Kopfer D, Vickery O, et al. Direct knock-on of desolvated ions governs strict ion selectivity in K+ channels. Nat Chem. 2018;10:813–820.
- Jensen MO, Jogini V, Eastwood M, et al. Atomic-level simulation of current–voltage relationships in single-file ion channels. Proc Natl Acad Sci USA. 2013;141:619–632.
- Guardiani C, Livi R, Cecconi F. Coarse grained modeling and approaches to protein folding. Curr Bioinf. 2010;5:217–240.
- Guardiani C, Cencini M, Cecconi F. Coarse-grained modeling of protein unspecifically bound to DNA. Phys Biol. 2014 11;11:026003DOI:.
- Guardiani C, Di Marino D, Tramontano A, et al. Exploring the unfolding pathway of maltose binding proteins: an integrated computational approach. J Chem Theory Comput. 2014;10:3589–3597.
- Marrink S, Tieleman D. Perspective on the Martini model. Chem Soc Rev. 2013;42:6801–6822.
- González-Arias F, Reddy T, Stone JE, et al. Scalable analysis of authentic viral envelopes on FRONTERA. Computing Sci Eng. 2020;22:11–20.
- Chavent M, Duncan A, Sansom M. Molecular dynamics simulations of membrane proteins and their interactions: from nanoscale to mesoscale. Curr Opin Struct Biol. 2016;40:8–16.
- Machado N, Bruininks BMH, Singh P, et al. Complex nanoemulsion for vitamin delivery: droplet organization and interaction with skin membranes. Nanoscale. 2022;14(2): 506–514 .
- Nemchinova M, Melcr J, Wassenaar TA, et al. Asymmetric CorA gating mechanism as observed by molecular dynamics simulations. J Chem Inf Model. 2021;61:2407–2417.
- Souza PC, Alessandri R, Barnoud J, et al. Martini 3: a general purpose force field for coarse-grained molecular dynamics. Nat Methods. 2021;18:382–388.
- Mahmood MI, Poma AB, Okazaki K. Optimizing Go-MARTINI coarse-grained model for F-BAR protein on lipid membrane. Front Mol Biosci. 2021;8, Article 619381, (pp 10) doi:10.3389/fmolb.2021.619381 https://www.frontiersin.org/article/10.33/fmolb.2021.619381 .
- Göbl C, Tjandra N. Application of solution NMR spectroscopy to study protein dynamics. Entropy. 2012;14:581–598.
- Guardiani C, Sun D, Giacomello A. Unveiling the gating mechanism of CRAC channel: a computational study. Front Mol Biosci. 2021;8:1205.
- Limongelli V. Ligand binding free energy and kinetics calculation in 2020. Wiley Interdiscip Rev Comput Mol Sci. 2020;10:e1455.
- Harpole T, Delemotte L. Conformational landscapes of membrane proteins delineated by enhanced sampling molecular dynamics simulations. BBA - Biomembr. 2018;1860:909–926.
- Bonomi M, Branduardi D, Bussi G, et al. PLUMED: a portable plugin for free energy calculations with molecular dynamics. Comput Phys Commun. 2009;180:1961.
- Case DA, Aktulga HM, Belfon K, et al. Amber 2021. San Francisco: University of California Press; 2021.
- Phillips JC, Braun R, Wang W, et al. Scalable molecular dynamics with NAMD. J Comput Chem. 2005;26:1781–1802.
- Abraham MJ, Murtola T, Schulz R, et al. GROMACS: high performance molecular simulations through multi-level parallelism from laptops to supercomputers. SoftwareX. 2015;1-2:19–25.
- Wang I, Friesner R, Berne B. Replica exchange with solute scaling: a more efficient version of replica exchange with solute tempering REST2). J Phys Chem B. 2011;115:9431–9438.
- Liu P, Kim B, Friesner RA, et al. Replica exchange with solute tempering: a method for sampling biological systems in explicit water. Proc Natl Acad Sci USA. 2005;102:13749–13754.
- Guardiani C, Marsili S, Procacci P, et al. Fragment 101-108 of myelin oligodendrocyte glycoprotein: a possible lead compound for multiple sclerosis. J Am Chem Soc. 2009;131:17176–17184.
- Guardiani C, Signorini G, Livi R, et al. Conformational landscape of N-glycosylated peptides detecting autoantibodies in multiple sclerosis, revealed by Hamiltonian replica exchange. J Phys Chem B. 2012;116:5458–5467.
- Guardiani C, Procacci P. The conformational landscape of tartrate-based inhibitors of the TACE enzyme as revealed by Hamiltonian Replica Exchange simulation. Phys Chem Chem Phys. 2013;15:9186–9196.
- Hamelberg D, Mongan J, MacCammon J. Accelerated molecular dynamics: a promising and efficient simulation method for biomolecules. J Chem Phys. 2004;120:11919–11929.
- Miao Y, Feher VA, McCammon JA. Gaussian accelerated molecular dynamics: unconstrained enhanced sampling and free energy calculation. J Chem Theory Comput. 2015;11:3584–3595.
- E W, Vanden-Eijnden E. Transition-path theory and path-finding algorithms for the study of rare events. Annu Rev Phys Chem. 2010;61:391–420.
- E W, Ren W, Vanden-Eijnden E. String method for the study of rare events. Phys Rev B. 2002;66:052301.
- E W, Ren W, Vanden-Eijnden E. Simplified and improved string method for computing the minimum energy paths in barrier-crossing events. J Chem Phys. 2007;126:164103.
- E W, Ren W, Vanden-Eijnden E. Finite temperature string method for the study of rare events. J Phys Chem A. 2005;109:6688–6693.
- Vanden-Eijnden E, Venturoli M. Markovian milestoning with Voronoi tessellations. J Chem Phys. 2009;130:194101.
- Maragliano L, Vanden-Eijnden E. On-the-fly string method for minimum free energy paths calculation. Chem Phys Lett. 2007;446:182–190.
- Pan AC, Sezer D, Roux B. Finding transition pathways using the string method with swarms of trajectories. J Phys Chem A. 2008;112:3432–3440.
- Johnson ME, Hummer G. Characterization of a dynamic string method for the construction of transition pathways in molecular reactions. J Phys Chem A. 2012;116:8573–8583.
- Maragliano L, Roux B, Vanden-Eijnden E. Comparison between mean forces and swarms-of-trajectories string methods. J Chem Theory Comput. 2014;10:524–533.
- Roux B. String method with swarms-of-trajectories, mean drifts, lag time, and committor. J Phys Chem A. 2021;125:7558–7571.
- Lacroix JJ, Pless SA, Maragliano L, et al. Intermediate state trapping of a voltage sensor. J General Physiol. 2012;140:635–652.
- Lev B, Murail S, Poitevin F, et al. String method solution of the gating pathways for a pentameric ligand-gated ion channel. Proc Nat Acad Sci. 2017;114:E4158–E4167.
- Torrie GM, Valleau JP. Nonphysical sampling distributions in Monte Carlo free-energy estimation: umbrella sampling. J Comp Phys. 1977;23:187–199.
- Carter E, Ciccotti G, Hynes JT, et al. Constrained reaction coordinate dynamics for the simulation of rare events. Chem Phys Lett. 1989;156:472–477.
- Izrailev S, Stepaniants B, Isralewitz B, et al. Steered molecular dynamics. In: Deuflhard P, Hermans J, Leimkuhler B, et al. editors. Computational Molecular Dynamics: Challenges, Methods, Ideas. Berlin, Heidelberg: Springer; 1999. p.39–65.Proceedings of the 2nd International Symposium on Algorithms for Maromolecular Modelling, Berlin, May 2124 1997.2124 1997.
- Schlitter J, Engels M, Kruger P. Targeted molecular dynamics: a new approach for searching pathways of conformational transitions. J Mol Graph. 1994;12:84–89.
- Laio A, Parrinello M. Escaping free energy minima. Proc Natl Acad Sci USA. 2002;99:12562–12566.
- Darve E, Rodriguez-Gomez D, Pohorille A. Adaptive biasing force method for scalar and vector free energy calculations. Proc Natl Acad Sci USA. 2008;128:144120.
- Kumar S, Rosenberg J, Bouzida D, et al. The weighted histogram analysis method for free-energy calculations on biomolecules. i. the method. J Comput Chem. 1992;13:1011–1021.
- Egwolf B, Roux B. Ion selectivity of the KcsA channel: a perspective from multi-ion free energy landscapes. J Mol Biol. 2010;401:831–842.
- Corry B, Thomas M. Mechanism of ion permeation and selectivity in a voltage gated sodium channel. J Am Chem Soc. 2012;134:1840–1846.
- Medovoy D, Perozo E, Roux B. Multi-ion free energy landscapes underscore the microscopic mechanism of ion selectivity in the KcsA channel. Biochim Biophys Acta. 2016 ;1858:1722–1732.
- Baştuğ T, Chen P, Patra S, et al. Potential of mean force calculations of ligand binding to ion channels from Jarzynski’s equality and umbrella sampling. J Chem Phys. 2008;128:155104.
- Deng Y, Roux B. Computations of standard binding free energies with molecular dynamics simulations. J Phys Chem B. 2009;113:2234–2246.
- Barducci A, Bussi G, Parrinello M. Well-tempered metadynamics: a smoothly converging and tunable free-energy method. Phys Rev Lett. 2008;100:020603.
- Ceccarelli M, Danelon C, Laio A, et al. Microscopic mechanism of antibiotics translocation through a porin. Biophys J. 2004;87:58–64.
- Furini S, Domene C. Computational studies of transport in ion channels using metadynamics. Biochim Biophys Acta. 2016;1858:1733–1740.
- Delemotte L, Kasimova M, Klein M, et al. Free-energy landscape of ion-channel voltage-sensor–domain activation. Proc Natl Acad Sci USA. 2014;112:124–129.
- Martin L, Corry B. Locating the route of entry and binding sites of benzocaine and phenytoin in a bacterial voltage gated sodium channel. PLOS Comp Biol. 2014;10:e1003688.
- Comitani F, Limongelli V, Molteni C. The free energy landscape of GABA binding to a pentameric ligand-gated ion channel and its disruption by mutations. J Chem Theory Comput. 2016;12:3398–3406.
- Maragliano L, Vanden-Eijnden E. A temperature accelerated method for sampling free energy and determining reaction pathways in rare events simulations. Chem Phys Lett. 2006;426:168–175.
- Abrams JB, Tuckerman ME. Efficient and direct generation of multidimensional free energy surfaces via adiabatic dynamics without coordinate transformations. J Phys Chem A. 2008;112:15742–15757.
- Oakes V, Furini S, Domene C. Insights into the mechanisms of K+ permeation in K+ channels from computer simulations. J Chem Theory Comput. 2019;16:794–799.
- Li J, Shen R, Reddy B, et al. Mechanism of C-type inactivation in the hERG potassium channel. Science Adv. 2021;7:eabd6203.
- Chipot C, Pohorille A. Calculating free energy differences using perturbation theory. In: Chipot C, Pohorille A editors. Free energy calculations. Chapter 2. Berlin, Heidelberg, New York: Springer; 2007. p. 33–75. Springer series in chemical physics 86.
- Straatsma TP, Berendsen HJC. Free energy of ionic hydration: analysis of a thermodynamic integration technique to evaluate free energy differences by molecular dynamics simulation. J Chem Phys. 1988;89:5876.
- Wan S, Bhati A, Zasada S, et al. Rapid, accurate, precise and reproducible ligand–protein binding free energy prediction. Interface Focus. 2020;10:20200007.
- Thomas M, Jayatilaka D, Corry B. Mapping the importance of four factors in creating monovalent ion selectivity in biological molecules. Biophys J. 2011;100:60–69.
- Genheden S, Ryde U. The MM/PBSA and MM/GBSA methods to estimate ligand-binding affinities. Expert Opin Drug Discov. 2015;10:449–461.
- Xu X, Guardiani C, Yan C, et al. Opening pathways of the DNA clamps proliferating cell nuclear antigen and Rad9-Rad1-Hus1. Nucl Acids Res. 2013;41:10020–10031.
- Wang C, Greene D, Xiao L, et al. Recent developments and applications of the MMPBSA method. Front Mol Biosci. 2018;4:87.
- Sahakyan H. Improving virtual screening results with MM/GBSA andMM/PBSA rescoring. J Comp-Aided Mol Des. 2021;35:731–736.
- Schuss Z. Brownian dynamics at boundaries and interfaces. New York, Heidelberg, Dordrecht, London: Springer; 2013.
- Li S, Hoyles M, Kuyucak S, et al. Brownian dynamics study of ion transport in the vestibule of membrane channels. Biophys J. 1998;74:37–47.
- Corry B, Allen TW, Kuyucak S, et al. Mechanisms of permeation and selectivity in calcium channels. Biophys J. 2001;80:195–214.
- Im W, Roux B. Brownian Dynamics solutions of ions channels: a general treatment of electrostatic reaction fields for molecular pores of arbitrary geometry. J Chem Phys. 2001;115:4850–4861.
- Im W, Seefeld S, Roux B. A grand canonical Monte Carlo–Brownian dynamics algorithm for simulating ion channels. Biophys J. 2000;79:788–801.
- Chung S, Corry B. Conduction properties of KcsA measured using Brownian dynamics with flexible carbonyl groups in the selectivity filter. Biophys J. 2007;93:44–53.
- Berneche S, Roux B. A microscopic view of ion conduction through the K+ channel. Proc Natl Acad Sci USA. 2003;100:8644–8648.
- Akeson M, Branton D, Kasianowicz J, et al. Microsecond time-scale discrimination among polycytidylic acid, polyadenylic acid, and polyuridylic acid as homopolymers or as segments within single RNA molecules. Biophys J. 1999;77:3227–3233.
- Deamer D, Akeson M. Nanopores and nucleic acids: prospects for ultrarapid sequencing. Trends Biotechol. 2000;18:147–151.
- Comer J, Aksimentiev A. Predicting the DNA sequence dependence of nanopore ion current using atomic-resolution Brownian dynamics. J Phys Chem C Nanometer Interfaces. 2012;116:3376–3393.
- Guardiani C, Rodger P, Fedorenko O, et al. Sodium binding sites and permeation mechanism in the NaChBac channel: a molecular dynamics study. J Chem Theory Comput. 2017;13:1389–1400.
- Payandeh J, Scheuer T, Zheng N, et al. The crystal structure of a voltage-gated sodium channel. Nature. 2011;475:353–358.
- Guardiani C, Fedorenko O, Roberts S, et al. On the selectivity of the NaChBac channel: an integrated computational and experimental analysis of sodium and calcium permeation. Phys Chem Chem Phys. 2017;19:29840–29854.
- Domene C, Barbini P, Furini S. Bias-exchange metadynamics simulations: an efficient strategy for the analysis of conduction and selectivity in ion channels. J Chem Theory Comput. 2015;11:1896–1906.
- Alberini G, Benfenati F, Maragliano L. A refined model of claudin-15 tight junction paracellular architecture by molecular dynamics simulations. PLoS One. 2017;12:e0184190.
- Suzuki H, Nishizawa T, Tani K, et al. Crystal structure of a claudin provides insight into the architecture of tight junctions. Science. 2014;344:304–307.
- Suzuki H, Tani K, Tamura A, et al. Model for the architecture of claudin-based paracellular ion channels through tight juctions. J Mol Biol. 2015;427:291–297.
- Alberini G, Benfenati F, Maragliano L. Molecular dynamics simulations of ion selectivity in a claudin-15 paracellular channel. J Phys Chem B. 2018;122:10783–10792.
- Maragliano L, Vanden-Eijnden E, Roux B. Free energy and kinetics of conformational transitions from Voronoi tessellated milestoning with restraining potentials. J Chem Theory Comput. 2009;5:2589–2594.
- Faradjian A, Elber R. Computing time scales from reaction coordinates by milestoning. J Chem Phys. 2004;120:10880–10889.
- Elber R. Milestoning: an efficient approach for atomically detailed simulations of kinetics in biophysics. Annu Rev Biophys. 2020;49:69–85.
- Votapka L, Lee C, Amaro R. Two relations to estimate membrane permeability using milestoning. J Phys Chem B. 2016;120:8606–8616.
- Vanden-Eijnden E. Transition path theory. In: Ferrario M, Ciccotti G, Binder K, editors. Computer simulations in condensed matter systems: from materials to chemical biology volume 1. (Lecture Notes in Physics. Vol. 703, Berlin, Heidelberg: Springer; 2006. p. 453–493.
- Chiodo L, Malliavin T, Maragliano L, et al. A structural model of the human α7 nicotinic receptor in an open conformation. PLoS ONE. 2015;10:e0133011.
- Chiodo L, Malliavin T, Maragliano L, et al. A possible desensitized state conformation of the human α7 nicotinic receptor: a molecular dynamics study. Biophys Chem. 2017;229:99–109.
- Chiodo L, Malliavin T, Giuffrida S, et al. Closed-locked and apo-resting state structures of the human α7 nicotinic receptor: a computational study. J Chem Inf Model. 2018;58:2278–2293.
- Hosseini Naveh Z, Malliavin T, Maragliano L, et al. Conformational changes in acetylcholine binding protein investigated by temperature accelerated molecular dynamics. PLoS ONE. 2014;9:e88555.
- Changeux JP. The nicotinic acetylcholine receptor: the founding father of the pentameric ligand-gated ion channel superfamily. J Biol Chem. 2012;287:40207–40215.
- Bouzat C, Lasala M, Nielsen B, et al. Molecular function of α7 nicotinic receptors as drug targets. J Physiol. 2018;596:1847–1861.
- Noviello C, Gharpure A, Mukhtasimova N, et al. Structure and gating mechanism of the a7 nicotinic acetylcholine receptor. Cell. 2021;184:2121–2134.
- Zhao Y, Liu S, Zhou Y, et al. Structural basis of human α7 nicotinic acetylcholine receptoractivation. Cell Res. 2021;1–4.
- Cottone G, Chiodo L, Maragliano L. Thermodynamics and kinetics of ion permeation in wild-type and mutated open active conformation of the human α7 nicotinic receptor. J Chem Inf Model. 2020;60:5045–5056.
- Song C, Corry B. Ion conduction in ligand-gated ion channels: Brownian dynamics studies of four recent crystal structures. Biophys J. 2010;98:404–411.
- Trudeau MC, Warmke JW, Ganetzky B, et al. HERG, a human inward rectifier in the voltage-gated potassium channel family. Science. 1995;269:92–95.
- Curran ME, Splawski I, Timothy KW, et al. A molecular basis for cardiac arrhythmia: hERG mutations cause long QT syndrome. Cell. 1995;80:795–803.
- Sanguinetti MC, Tristani-Firouzi M. hERG potassium channels and cardiac arrhythmia. Nature. 2006;440:463–469.
- Cavero I, Guillon JM, Ballet V, et al. Comprehensive in vitro Proarrhythmia Assay (CiPA): pending issues for successful validation and implementation. J Pharmacol Toxicol Methods. 2016;81:21–36.
- Wang W, MacKinnon R. Cryo-EM structure of the open human ether-à-go-go-related K+ channel hERG. Cell. 2017;169:422–430.
- Kang P, Westerlund A, Shi J, et al. Calmodulin acts as a state-dependent switch to control a cardiac potassium channel opening. Sci Adv. 2020;6:eabd6798.
- Costa F, Guardiani C, Giacomello A. Exploring Kv1.2 channel inactivation through MD simulations and network analysis. Front Mol Biosci. 2021;8:784276.
- Beckstein O, Biggin P, Sansom M. A hydrophobic gating mechanism for nanopores. J Phys Chem B. 2001;105:12902–12905.
- Zhu F, Hummer G. Drying transition in the hydrophobic gate of the GLIC channel blocks ion conduction. Biophys J. 2012;103:219–227.
- Tinti A, Giacomello A, Grosu Y, et al. Intrusion and extrusion of water in hydrophobic nanopores. Proc Natl Acad Sci USA. 2017;114:E10266–E10273.
- Franks NP. General anaesthesia: from molecular targets to neuronal pathways of sleep and arousal. Nat Rev Neurosci. 2008;9:370–386.
- Long S, Campbell E, MacKinnon R. Voltage sensor of Kv1.2: structural basis of electromechanical coupling. Science. 2005;309:903–908.
- Long S, Campbell E, MacKinnon R. Atomic structure of a voltage-dependent K+ channel in a lipid membrane-like environment. Nature. 2007;450:376–382.
- Li Q, Wanderling S, Paduch M, et al. Structural mechanism of voltage-dependent gating in an isolated voltage-sensing domain. Nat Struct Mol Biol. 2014;21:244–252.
- Pathak M, Yarov-Yarovoy V, Agarwal G, et al. Closing in on the resting state of the shaker K+ channel. Neuron. 2007;56:124–140.
- Khalili-Araghi F, Jogini V, Yarov-Yarovoy V, et al. Calculation of the gating charge for the Kv1.2 voltage-activated potassium channel. Biophys J. 2010;98:2189–2198.
- Vargas E, Bezanilla F, Roux B. In search of a consensus model of the resting state of a voltage-sensing domain. Neuron. 2011;72:713–720.
- Vargas E, Yarov-Yarovoy V, Khalili-Araghi F, et al. An emerging consensus on voltage-dependent gating from computational modeling and molecular dynamics simulations. J Gen Physiol. 2012;140:587–594.
- Catterall WA. Molecular properties of voltage-sensitive sodium channels. Annu Rev Biochem. 1986;55:953–985.
- Guy HR, Seetharamulu P. Molecular model of the action potential sodium channel. Proc Natl Acad Sci U S A. 1986 Jan;83:508–512.
- Jiang Y, Ruta V, Chen J, et al. The principle of gating charge movement in a voltage-dependent K+ channel. Nature. 2003;423:42–48.
- Chanda B, Asamoah O, Blunck R, et al. Gating charge displacement in voltage-gated ion channels involves limited transmembrane movement. Nature. 2005;436:852–856.
- Carpenter E, Beis K, Cameron A, et al. Overcoming the challenges of membrane protein crystallography. Curr Opin Struct Biol. 2008;18:581–586.
- Slowik D, Henderson R. Benchmarking the stability of human detergent-solubilised voltage-gated sodium channels for structural studies using eel as a reference. Biochim Biophys Acta. 2015;1848:15451551.
- Venien-Bryan C, Li Z, Vuillard L, et al. Cryo-electron microscopy and x-ray crystallography: complementary approaches to structural biology and drug discovery. Acta crystallogr F. 2017;73:174–183.
- Shen H, Zhou Q, Pan X, et al. Structure of a eukaryotic voltage-gated sodium channel at near-atomic resolution. Science. 2017;355:6328.
- Yan Z, Zhou Q, Wang L, et al. Structure of the Nav1.4-β1 Complex from Electric Eel. Cell. 2017;170:470–482.
- Pan XZL, Zhou Q, Shen H, et al. Structure of the human voltage-gated sodium channel Nav1.4 in complex with β1. Science. 2018;362(6412) :eaau2486.
- Pan X, Li Z, Huang X, et al. Molecular basis for pore blockade of human Na+ channel Nav 1.2 by the μ-conotoxin KIIIA. Science. 2019;363(6433):1309–1313.
- Shen H, Liu D, Wu K, et al. Structures of human Nav1.7 channel in complex with auxiliary subunits and animal toxins. Science. 2019;363:1303–1308.
- Jiang D, Shi H, Tonggu L, et al. Structure of the cardiac sodium channel. Cell. 2020;180:122–134.
- Li Z, Jin X, Wu T, et al. Structural basis for pore blockade of the human cardiac sodium channel Nav1.5 by the antiarrhythmic drug quinidine. .Angew. Chem. Int Ed. Engl. 2021;60(20):11474–11480.
- Li Z, Jin X, Wu T, et al. Structure of human Nav1.5 reveals the fast inactivation-related segments as a mutational hotspot for the long QT syndrome. Proc Natl Acad Sci USA. 2021;118:e2100069118.
- Pan X, Li Z, Jin X, et al. Comparative structural analysis of human Nav1.1 and Nav1 5 reveals mutational hotspots for sodium channelopathies. Proc Natl Acad Sci USA. 2021;118:e2100066118.
- Capener CE, Kim HJ, Arinaminpathy Y, et al. Ion channels: structural bioinformatics and modelling. Hum Mol Genet. 2002;11:2425–2433.
- Giorgetti A, Carloni P. Molecular modeling of ion channels: structural predictions. Curr Opin Chem Biol. 2003;7:150–156.
- Menkea J, Maskria S, Kocha O. Computational ion channel research: from the application of artificial intelligence to molecular dynamics simulations. Cell Physiol Biochem. 2021;55:14–45.
- Ashrafuzzaman M. Artificial intelligence, machine learning and deep learning in ion channel bioinformatics. Membranes (Basel). 2021;11:672.
- Han K, Wang M, Zhang L, et al. Predicting ion channels genes and their types with machine learning techniques. Front Genet. 2019;10:399.
- Jumper J, Evans R, Pritzel A, et al. Highly accurate protein structure prediction with alphafold. Nature. 2021;596:583–589.
- Karplus M, Petsko G. Molecular dynamics simulations in biology. Nature. 1990;347:631–639.
- Vorobyov I, Anisimov V, MacKerell AJ. Polarizable empirical force field for alkanes based on the classical Drude oscillator model. J Phys Chem B. 2005;109:18988––18999.
- Allen TW, Andersen OS, Roux B. Energetics of ion conduction through the gramicidin channel. Proc Natl Acad Sci. 2004;101:117–122.
- Patel S, Davis JE, Bauer BA. Exploring ion permeation energetics in gramicidin A using polarizable charge equilibration force fields. J Am Chem Soc. 2009 Oct;131:13890–13891.
- Ngo V, Li H, MacKerell JAD, et al. Polarization effects in water-mediated selective cation transport across a narrow transmembrane channel. J Chem Theory Comput. 2021;17:1726–1741.
- Yu H, Whitfield T, Harder E, et al. Simulating monovalent and divalent ions in aqueous solution using a Drude polarizable force field. J Chem Theory Comput. 2010;6:774––786.
- Lemkul JA, Huang J, Roux B, et al. An empirical polarizable force field based on the classical drude oscillator model: development history and recent applications. Chem Rev. 2016 05;116:4983–5013. DOI:10.1021/acs.chemrev.5b00505
- Lin FY, Huang J, Pandey P, et al. Further optimization and validation of the classical Drude polarizable protein force field. J Chem Theory Comput. 2020 May;16:3221–3239.
- Shi Y, Xia AZ, Zhang J, et al. Polarizable atomic multipole-based Amoeba force field for proteins. J Chem Theory Comput. 2013;9:4046––4063.
- Wang A, Zhang Z, Li G. Higher accuracy achieved in the simulations of protein structure refinement, protein folding, and intrinsically disordered proteins using polarizable force fields. J Phys Chem Lett. 2018;9:7110––7116.
- Jiang W, Hardy DJ, Phillips JC, et al. High-performance scalable molecular dynamics simulations of a polarizable force field based on classical Drude oscillators in NAMD. J Phys Chem Lett. 2011;2:87–92.
- Inakollu V, Geerke D, Rowley C, et al. Polarisable force fields: what do they add in biomolecular simulations? Curr Opin Struct Biol. 2020;61:182––190.
- Huang J, Lemkul JA, Eastman PK, et al. Molecular dynamics simulations using the Drude polarizable force field on GPUs with OpenMM: Implementation, validation, and benchmarks. J Comp Chem. 2018;39:1682–1689.
- Dror R, Pan A, Arlow D, et al. Pathway and mechanism of drug binding to G-protein-coupled receptors. Proc Natl Acad Sci USA. 2011;108:13118–13123.
- Shaw DE, Adams PJ, Azaria A, et al. Anton 3: twenty microseconds of molecular dynamics simulation before lunch. In: Proceedings of the International Conference for High Performance Computing, Networking, Storage and Analysis; 2021. p. 1–11.
- Orlandini S, Meloni S, Ciccotti G. Combining rare events techniques: phase change in Si nanoparticles. J Stat Phys. 2011;145:812–830.
- Giacomello A, Meloni S, Chinappi M, et al. Cassie–Baxter and Wenzel states on a nanostructured surface: phase diagram, metastabilities, and transition mechanism by atomistic free energy calculations. Langmuir. 2012;28:10764–10772.
- Praprotnik M, Delle Site L, Kremer K. Adaptive resolution scheme for efficient hybrid atomistic-mesoscale molecular dynamics simulations of dense liquids. Phys Rev E. 2006;73:066701.
- Potestio R, Fritsch S, Espanol P, et al. Hamiltonian adaptive resolution simulation for molecular liquids. Phys Rev Lett. 2013;110:108301.
- Cortes-Huerto R, Praprotnik M, Kremer K, et al. From adaptive resolution to molecular dynamics of open systems. Eur Phys J. 2021;94:1–22.
- Lambeth B, Junghans C, Kremer K, et al. On the locality of hydrogen bond networks at hydrophobic interfaces. J Chem Phys. 2010;133:221101.
- Boereboom J, Potestio R, Donadio D, et al. Toward Hamiltonian adaptive QM/MM: accurate solvent structures using many-body potentials. J Chem Theory Comput. 2016;12:3441.
- Heidari M, Cortes-Huerto R, Potestio R, et al. Steering a solute between coexisting solvation states: revisiting nonequilibrium work relations and the calculation of free energy differences. J Chem Phys. 2019;151:144105.
- Heidari M, Kremer K, Cortes-Huerto R, et al. Spatially resolved thermodynamic integration: an efficient method to compute chemical potentials of dense fluids. J Chem Theory Comput. 2018;14:3409–3417.
- Wang Y, Ribeiro JML, Tiwary P. Machine learning approaches for analyzing and enhancing molecular dynamics simulations. Curr Opin Struct Biol. 2020;61:139–145.
- LeCun Y, Bengio Y, Hinton G. Deep learning. Nature. 2015;521:436–444.
- Bottou L, Bousquet O . The tradeoffs of large scale learning. In: Platt J, Koller D, Singer Y, editors. Proceeding Advances in Neural Information Processing Systems. Red Hook, NY, USA: Curran Associates, Inc; 2007. p. 161–168.
- Noe F, Tkatchenko A, Mueller KR, et al. Machine learning for molecular simulation. Annu Rev Phys Chem. 2020;71:361–390.
- Lindorff-Larsen K, Maragakis P, Piana S, et al. Systematic validation of protein force fields against experimental data. PLoS ONE. 2012;7:e32131.
- Huang J, Rauscher S, Nawrocki G, et al. CHARMM36: an improved force field for folded and intrinsically disordered proteins. Nat Methods. 2016;14:71–73.
- Chmiela S, Tkatchenko A, Sauceda H, et al. Machine learning of accurate energy-conserving molecular force fields. Sci Adv. 2017;3:e1603015.
- Chmiela S, Sauceda H, Mueller K, et al. Towards exact molecular dynamics simulations with machine-learned force fields. Nat Commun. 2018;9:3887.
- Schneider E, Dai L, Topper R, et al. Stochastic neural network approach for learning high-dimensional free energy surfaces. Phys Rev Lett. 2017;119:150601.
- Zhang L, Han J, Wang H, et al. DeePCG: constructing coarse-grained models via deep neural networks. J Chem Phys. 2018;149:034101.
- Wang J, Olsson S, Wehmeyer C, et al. Machine learning of coarse-grained molecular dynamics force fields. ACS Cent Sci. 2019;5:755–767.
- Ichiye T, Karplus M. Collective motions in proteins: a covariance analysis of atomic fluctuations in molecular dynamics and normal mode simulations. Proteins Struct Funct Bioinf. 1991;11:205–217.
- Perez-Hernandez G, Paul F, Giorgino T, et al. Identification of slow molecular order parameters for Markov model construction. J Chem Phys. 2013;139:015102.
- Chen W, Ferguson A. Molecular enhanced sampling with autoencoders: on-the-fly collective variable discovery and accelerated free energy landscape exploration. J Comput Chem. 2018;39:2079–2102.
- Chen M. Collective variable-based enhanced sampling and machine learning. Eur Phys J B. 2021;94:1–17.
- Laplace P. Introduction to “Oeuvres, vol. VII, theorie analytique de probabilites”. Paris: Gauthier-Villars; 1812.
- Dirac P. Quantum mechanics of many-electron systems. Proc R Soc A. 1929;123:714–733.
- Tuckerman M. Statistical mechanics: Theory and molecular simulation. Oxford: Oxford University Press; 2010.