ABSTRACT
This research contributes to an emerging evidence base that considers a possible relationship between exposure to road traffic noise and mental distress. This study aimed to determine whether chronic exposure to road traffic noise was associated with quality of life or various measures of mental distress. We spatially linked high-quality modelled noise exposure data for the cities of Dublin and Cork in Ireland to The Irish Longitudinal Study on Ageing, allowing an examination of these health outcomes among older adults while adjusting for socio-demographic and behavioural characteristics. Exposure to air pollution was also considered in the analysis, allowing any associations between noise and either quality of life or mental distress that were independent of this other stressor to be isolated. While the study did not detect evidence of an association between noise exposure and depression, anxiety, stress, or worry, it identified a negative association between exposure to road traffic noise and quality of life that was independent of a range of socio-demographic and behavioural factors. Moving from the highest quintile of noise exposure to the lowest was associated with an increase on the CASP-12 quality of life scale of 1.08 of a standard deviation.
Introduction
Environmental noise is defined by the World Health Organization (WHO) as ‘noise emitted from all sources, except noise at the industrial workplace,’ and includes ‘road, rail, air traffic, industries, construction and public work, and the neighbourhood’ (WHO Citation2011). It is an omnipresent feature of daily life, particularly in cities and urban areas. Urban transportation is a key source of environmental noise. For example, one study found high average levels of noise exposure on all transport modes around Paris (Kreuzberger et al. Citation2019), while unhealthy levels of noise pollution were recorded at bus stops in Chennai (Mahesh Citation2021). Unlike some other environmental stressors in cities, such as second-hand smoke, dioxins and benzenes, population exposure to environmental noise from road traffic is increasing in Europe (WHO Citation2011, European Environment Agency Citation2020, Murphy and King Citation2022). Increasing levels of mobility and road traffic linked with unbalanced urban development have been identified as particular factors in this increase (Silva and Mendes Citation2012). Overall, environmental noise has been associated with significant harmful effects on health (Faulkner and Murphy Citation2022) and it is now considered a key environmental and societal concern in cities, in both developed and developing countries (WHO Citation2018, Xu et al. Citation2020).
A recent literature review on links between transportation and health noted that noise had been identified as a pathway through which urban transportation can have a negative effect on health (Glazener et al. Citation2021). Noise is considered a psychological stimulus that induces a central nervous system response, which can in turn disrupt homeostasis, the optimal physical and chemical balance in the human organism (Recio et al. Citation2016). In terms of its pathology, the physiological reaction to noise is understood to include the hyperarousal of the sympathetic nervous and endocrine systems, and the secretion of stress hormones including cortisol and catecholamines through the hypothalamic-pituitary-adrenal and sympathetic-adrenal-medullary axes (Ader Citation2000). The biological defensive response is thus exacerbated and extended by exposure to noise (Aich and Potter Citation2009). Chronic exposure to environmental noise is hypothesised to impact negatively on quality of life, wellbeing and mental health through a prolonged activation of these physiological responses (Clark and Paunovic Citation2018). Night-time noise interfering with sleep has also been proposed as a mechanism through which noise could negatively affect quality of life or mental wellbeing (Clark and Paunovic Citation2018).
Despite the high prevalence of environmental noise such as road traffic noise, previous research has tended to focus on the auditory effects of once-off extreme impulse noise or chronic exposure to noise in an occupational setting on human health (Basner et al. Citation2015). Two systematic reviews of environmental noise studies concluded that there is a relative lack of evidence linking noise exposure to quality of life, wellbeing and mental health (Clark and Paunovic Citation2018, Clark et al. Citation2020). The earlier review informed the WHO Environmental Noise Guidelines for the European Region (WHO Citation2018). While in general no effects were found, the quality of the evidence was considered low, so the authors could not definitively rule out associations between road traffic noise and these outcomes (Clark and Paunovic Citation2018). The more recent review found evidence of a harmful effect, but also deemed this evidence to be of low quality (Clark et al. Citation2020). Both reviews found similar evidence using antidepressant or anxiolytic medication as a proxy for depression or anxiety. In terms of quality of life, the Clark and Paunovic (Citation2018) review noted several studies that utilised self-rated measures of quality of life and general health, but again with only low-quality evidence of no substantial effect.
Two groups highlighted as potentially vulnerable in relation to noise exposure and other health outcomes, such as cognitive performance or cardiovascular health, are children and older adults (Hygge Citation2003, van Kamp and Davies Citation2013). However, while several studies of noise and mental wellbeing or mental distress have focused on children, there appears to be a relative dearth of evidence that specifically considers older adults (Clark and Paunovic Citation2018, Clark et al. Citation2020, Douglas and Murphy Citation2020). One recent study empirically examined possible associations between road traffic noise exposure and cognitive health among older adults and found some evidence of an association between noise and executive function (Mac Domhnaill et al. Citation2021).
The current paper focuses on quality of life, mental distress and exposure to road traffic noise among older adults in Ireland. It contributes to an emerging evidence base on the impact of road traffic noise on mental health with a representative, cross-sectional study of older adults using regression models on microdata on health and wellbeing from The Irish Longitudinal Study on Ageing (TILDA). The study aimed to determine whether chronic exposure to road traffic noise was associated with quality of life and various measures of mental distress, including depression, anxiety, stressand worry.
Methods and data
The Irish Longitudinal Study on Ageing (TILDA)
TILDA is a nationally representative longitudinal study of over 8,000 persons aged 50 and over in Ireland (Kearney et al. Citation2011, Donoghue et al. Citation2018). At each wave of data collection (every two years), all members of sample households aged 50 years and over provide information on multiple aspects of their lives, including health, economic and social circumstances. To date, five waves of data collection have been completed. TILDA is part of an international network of longitudinal ageing studies, and therefore the survey content is harmonised with other studies, such as the Survey of Health, Ageing and Retirement in Europe, the English Longitudinal Study of Ageing and the Health and Retirement Survey in the US. At the first wave of data collection, the geo-code of each TILDA respondent’s home address was recorded, allowing the dataset to be linked with other geo-coded spatial data. Three different methods are utilised to collect data for TILDA. In the first instance, Computer Assisted Personal Interviewing is used by trained interviewers who call to respondents’ homes. Second, at the home interview, participants are given a self-completion questionnaire (to gather information on more sensitive issues, such as alcohol consumption, quality of relationships, etc.) which they then return by post to TILDA. Finally, at the first and third waves of data collection, respondents attended a health assessment at specialised Health Assessment Centres, or a modified partial assessment in their homes if travel to a centre was not practicable. These assessments were carried out by trained nurses. Written informed consent was obtained for all TILDA participants at each wave of data collection. Ethical approval for each wave of data collection was granted by the Faculty of Health Sciences Research Ethics Committee at Trinity College Dublin. The study was carried out according to the guidelines of the Declaration of Helsinki.
The current study employed data from the third wave of TILDA, which was collected between March 2014 and October 2015 from 6,396 individuals aged 54 and over. Data gathered from the Computer Assisted Personal Interviewing and self-completion questionnaire was used. Data on estimated noise exposure was matched to respondents living in the Dublin and Cork agglomerations, which are subject to noise mapping under the EU Environmental Noise Directive. Twelve respondents whose residences were exposed to railway (Environmental Protection Agency Citation2018a) or aircraft noise (Environmental Protection Agency Citation2018b) above 45 dB at night were removed from the analysis to isolate any associations between road traffic noise specifically and the outcome variables, giving a sample size of 1,706 for analysis. A flow chart detailing the sample size is provided in Appendix A. This sample size varied by outcome variable due to varying response rates in TILDA.
Noise exposure: modelling exposure to road traffic noise
Modelled levels of exposure to road traffic noise were spatially linked to TILDA using a Geographic Information System (GIS) platform, QGIS 3.10. This assigned a modelled level of noise exposure to each respondent at their residence. Noise exposure levels were estimated in decibels, dB(A), at the façades of residential buildings in Dublin and Cork, using input data collated between 2012 and 2016 including traffic flow and composition, building height and geometry, ground cover and topology as well as meteorological information. The Predictor-LimA Advanced V2019.02 software package was employed to model road traffic noise using the ‘Common Noise Assessment Methods in the European Union’ (CNOSSOS-EU) methodology, a common methodological framework recently developed for EU Member States (see Murphy et al. Citation2020). This study used Lnight, the EU indicator of annual average (A-weighted) noise level for night-time periods, taken at the most exposed receiver point on the most exposed façade as the noise exposure variable. ‘Night-time’ is defined for Lnight as an eight-hour period between 23:00 and 07:00. illustrates modelled values of Lnight for Dublin and Cork based on this methodology, and descriptive statistics for the Lnight variable are included in .
Figure 1. Night-time road traffic noise exposure (Lnight) at the most exposed façade or residential buildings, Dublin and Cork.
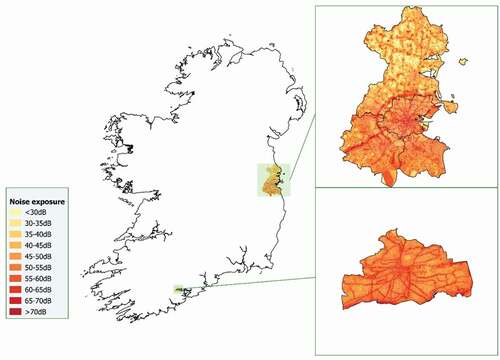
Table 1. Descriptive statistics, pollution exposure variables.
Previous literature was followed in spatially linking a single value of noise exposure estimated at the most exposed façade of the residence for each TILDA respondent (Tzivian et al. Citation2017). While the study had no data on the times at which TILDA respondents are usually in their residence, it is reasonable to assume that most respondents spend this overnight period at home, and that Lnight is thus a good measure of a respondent’s actual exposure to road traffic noise. In addition, sleep interference has been identified as a possible mechanism for a negative effect of noise exposure on quality of life or mental health, indicating the importance of studying Lnight as an exposure variable (Clark and Paunovic Citation2018). Noise exposure was categorised using quintiles to protect anonymity (as a condition of linking noise exposure data with TILDA) and to allow for possible non-linear effects in any relationships between noise and the outcome variables. Noise exposure is measured on a logarithmic scale, and quintiles allow for the logarithmic form of the exposure variable by imposing no functional form restriction on the modelled relationship.
Outcome variables: quality of life and mental distress
A comprehensive picture of the mental health of respondents is established in TILDA by a rich set of questionnaire-based variables measuring quality of life and various forms of mental distress, including depression, anxiety, stress and worry. Descriptive statistics for the variables examined are presented in .
Table 2. Descriptive statistics and outcome variables.
Quality of life was determined in wave 3 of TILDA across four domains using the twelve-item Control, Autonomy, Self-realisation and Pleasure (CASP-12) scale, with a higher score indicating a better quality of life (Hyde et al. Citation2003). To test for depression, TILDA employed the eight-item Center for Epidemiologic Studies Depression (CES-D) scale, which scored respondents on a scale from 0 to 24 with a score of 9 or above indicative of clinical depression (Radloff Citation1977). The anxiety subscale of the Hospital Depression and Anxiety scale (HADs-A) was used to test for anxiety, ranging from 0 to 21 with a score of 11 or above considered indicative of a case of anxiety (Zigmond and Snaith Citation1983). Stress and worry were tested using the Perceived Stress Score (PSS; Cohen et al. Citation1983), and the Penn State Worry Questionnaire (PSWQ; Meyer et al. Citation1990), respectively, with higher scores indicating greater levels of stress or worry.
In addition, details of any medication regularly taken by respondents were recorded, including antidepressant and anxiolytic medication, offering an alternative medication-based method of detecting the presence of a depression or anxiety disorder.
Covariates: socio-demographics and behaviour
Adjusting regression models for potentially confounding socio-demographic or behavioural characteristics suggested by the literature and collected in TILDA allowed the study to identify any associations between environmental noise and quality of life or mental distress that were independent of these individual-level characteristics. presents descriptive statistics of these covariates.
Table 3. Descriptive statistics and potentially confounding characteristics.
Models were adjusted for age, gender, socioeconomic status, employment status, residential density within a 1-km radius, alcohol consumption, physical activity, general health status and social connectedness. Net household income and level of education were both used to measure socioeconomic status. Alcohol consumption was included using the outcome of the CAGE problematic alcohol scale (Ewing Citation1984), and physical activity was captured using the International Physical Activity Questionnaire (Craig et al. Citation2003). General health status was measured as having a long-term health limitation and by the use of five or more medications (‘polypharmacy’) on a regular basis. Finally, the Berkman-Syme Social Network Index (Berkman and Syme Citation1979) was used to measure social connectedness.
Covariate: ambient air quality
Models were further adjusted for exposure to ambient air pollution in order to isolate any independent associations between environmental noise specifically and the outcome variables. Ground-level nitrogen dioxide (NO2) exposure was modelled in micrograms per cubic metre air (μg m−3) for 2015 by the Irish Environmental Protection Agency (Aves and Williams Citation2019), and this measure of NO2 was employed in this study as a proxy for exposure to air pollution. Descriptive statistics for this variable are presented in . As with the noise exposure variable, exposure to air pollution was categorised using quintiles to protect anonymity and to allow for potential non-linear effects. Data on air quality was only available for a sub-sample of observations in central Dublin, and this investigation was thus limited to adjusting models for air pollution in a sub-sample analysis of 568 respondents. This sub-sample was not representative of the wider sample, however, as these observations reside in the more densely populated centre of Dublin, while the wider study area also encompasses less densely populated areas of both the Dublin and Cork agglomerations.
In this sub-sample analysis, alternative models that included each of PM2.5 and PM10 as measures of air pollution instead of NO2 were also run. These measures were also modelled for 2015 by the Environmental Protection Agency (Aves and Williams Citation2019).
Model specification
With this cross-sectional data, the study used an ordinary least squares linear regression model for the CASP-12 scale, and negative binomial regression models for the CES-D, HADs-A, PSS, and PSWQ scales, with standard errors clustered at the household level in each model. The choices of linear and negative binomial specifications were based on the forms of the respective outcome variables and empirically on a comparison of values for the Akaike Information Criterion and Bayesian Information Criterion for Gaussian, Poisson and negative binomial identities for each outcome variable, with Gaussian preferred for the CASP-12 scale and negative binomial for all other outcomes.
As an alternative measure to the CES-D and HADs-A scales for depression and anxiety, respectively, logistic regression models were employed to test whether noise exposure was independently associated with the use of antidepressant or anxiolytic medication.
As a sensitivity check of any results that indicated a possible association with noise exposure, a separate regression model was run that included Lden, an EU indicator of annual average (A-weighted) noise exposure over a 24-h period, as the noise exposure variable in place of Lnight. As with Lnight, this Lden indicator was generated as part of the study’s noise modelling process.
For each outcome variable, these quantitative techniques tested the hypothesis that environmental noise was associated with the outcome. For each model, a backward selection process using F-tests was employed to remove covariates that did not impact the model. Statistical analysis was conducted using Stata 14.
Results are presented in the form of average marginal effects for quintiles of noise exposure relative to the reference category, the highest quintile of exposure. The average marginal effect associated with a quintile of noise exposure is the average of predicted changes in the outcome variable relative to the highest quintile of noise exposure, holding all other covariates constant. P-values, indicating the probability of obtaining results as extreme as the observed results under the null hypothesis, are also reported. Outcome variables were standardised using z-scores and reported average marginal effects can thus be interpreted as proportions of a standard deviation.
Results
Results of this study’s main regression models, fully adjusted for socio-demographic and behavioural covariates, are summarised for the noise exposure variable in . A complete set of results for the CASP-12 model is provided as Model I of in Appendix B.
Table 4. Average marginal effects of noise exposure.
indicates a negative association between exposure to road traffic noise and quality of life, as measured by the CASP-12 scale. Moving from the highest quintile of noise exposure to the second lowest is associated with an increase on the CASP-12 scale of 1.07 of a standard deviation, and an increase of 1.08 of a standard deviation moving from the highest quintile of exposure to the lowest. By way of comparison, these average marginal effects are larger than the average marginal effect of moving from having completed only primary or no education to having completed secondary education. The p-values that correspond to these average marginal effects at lower quintiles of noise exposure are low at 0.01. This association is independent of the socio-demographic and behavioural characteristics considered in this investigation.
A separate linear regression model of the CASP-12 scale was run including Lden (24-h noise exposure) as the noise exposure variable to test the sensitivity of this finding to the choice of noise exposure indicator. Results for this regression, shown in (Model II) in Appendix B, are broadly reflective of the main CASP-12 results.
However, the main results do not indicate any such association between noise exposure and depression, anxiety, stress or worry as measured by the respective questionnaire-based scales, the CES-D, HADs-A, PSS and PSWQ scales. The results also show no evidence of an association with depression or anxiety when employing the use of antidepressant or anxiolytic medications as outcome variables.
When focusing on the sub-sample of 568 respondents who live in central Dublin and for whom data on ambient air quality was available to the study, however, there is no evidence of a relationship between noise exposure and respondents’ score on the CASP-12 scale. Summary results of this sub-sample analysis are presented in in Appendix B. There is also no evidence of a relationship between exposure to air pollution and the CASP-12 variable among this sub-sample.
Discussion
Employing a questionnaire-based measure of quality of life that scores respondents across four different domains, the CASP-12 scale, this study detects a negative association between exposure to road traffic noise and quality of life that is independent of a range of socio-demographic and behavioural factors. The association is also robust to the choice of Lnight over Lden as the noise exposure variable. This indicates that respondents with lower exposure to road traffic noise achieve a higher quality of life score on the CASP-12 scale relative to the respondents most exposed to noise.
When focusing on a sub-sample in central Dublin to further adjust the model for exposure to ambient air pollution, no evidence of an association between noise and quality of life was found. Interpreting this result is difficult, as the sub-sample for which air pollution data was available was not representative of the wider sample, which is a limitation of the study. It may also be the case that the study did not have the statistical power to identify and disentangle any true associations with noise and air pollution in this sub-sample analysis. There is a moderate correlation of 0.69 between the study’s measures of noise and NO2 pollution, suggesting the possibility that multicollinearity may be an issue, which could mask a true association from detection. Alternatively, it may be an indication that the result for quality of life in the main model is spurious. Overall, however, due to the reduced sample size, results from the sub-sample analysis are not directly comparable with the main analysis. The 2018 review of previous research (Clark and Paunovic Citation2018) suggested that there is no substantial effect of noise on quality of life, although the measures of quality of life considered in these studies, such as the Short Form Health Survey or the General Health Questionnaire, were not as comprehensive as the CASP-12 scale in relation to quality of life.
No evidence was found of an association between noise exposure and depression or anxiety, measured using questionnaire-based scales, the CES-D and HADs-A, or measured through the use of antidepressant and anxiolytic medication. As discussed in the Introduction, the physiological reaction to noise exposure includes the secretion of stress hormones, and stress can thus be considered a potential mechanism for any effect on quality of life and mental distress. However, the study finds no evidence of an association between noise and either stress or worry, also measured utilising questionnaire-based scales, the PSS and PSWQ. Therefore, the null hypothesis of there being no relationship between road traffic noise exposure and either depression, anxiety, stress or worry cannot be rejected in this study. It is possible that testing for relationships between noise exposure at the most exposed façade of the respondent’s residence and their quality of life or mental health may impede the clear detection of true effects of noise on mental distress. The earlier review of research in this area had suggested that there was evidence of road traffic noise having no effect on depression or anxiety (Clark and Paunovic Citation2018), although the more recent review noted some emerging longitudinal evidence of a harmful effect on various measures of depression and anxiety (Clark et al. Citation2020).
Conclusions
This paper contributes to an emerging evidence base that considers a possible relationship between exposure to road traffic noise and quality of life or mental distress. As environmental noise is an important feature of daily living in cities and urban areas, robust evidence on this issue is necessary in a global context of increasing urbanisation. While the study does not find any evidence of an association between road traffic noise exposure and depression, anxiety, stress or worry, it finds some evidence of a negative association between exposure to road traffic noise and quality of life as measured by the CASP-12 scale that is independent of a range of socio-demographic and behavioural factors.
This study’s findings should be interpreted in the context of certain limitations. First, while this paper broadens the evidence base in relation to older adults, its findings cannot be generalised to the whole population. Subject to data availability, further research into different aspects of mental health that considers environmental noise as well as a wide range of socio-demographic and behavioural covariates for different population groups would be useful. Second, as with many studies in this literature, this investigation is cross-sectional in nature, and therefore causality cannot be inferred. Subject to data availability, future research could exploit longitudinal data to improve on this, although causality would still remain elusive given the possibility of confounding by unobservable time-varying factors.
Due to limitations in data availability, data on road traffic noise exposure was modelled based on input data collated between 2012 and 2016, while data on cognitive health, socio-demographic and behavioural variables were collected by TILDA during 2014 and 2015. It is considered unlikely, however, that the noise modelling input data changed significantly during this period, and the quantitative effect of this limitation is therefore likely to be small. Data limitations also dictated that models of quality of life or mental distress could only be adjusted for exposure to ambient air pollution for a smaller sub-sample in central Dublin. Applying this model of quality of life including both noise and air pollution to a larger sample could be useful.
Subject to the availability of a larger sample size, another potential avenue for further research is to explore whether the results of this study differ by other demographic characteristics, such as gender or socio-economic status among older adults. We have adjusted for these characteristics in this study such that results are independent of them, but it may be the case that there is a stronger association between noise exposure and quality of life among further sub-groups. Further research into possible mechanisms underlying a relationship between noise exposure and quality of life would also be beneficial.
This study makes several valuable contributions to the literature. First, high-quality modelled noise exposure data from two cities, based on the new CNOSSOS-EU standard, was spatially linked to TILDA. This allowed the study to examine quality of life and various forms of mental distress through well-established questionnaire-based measures, while adjusting for a wide range of socio-demographic and behavioural characteristics. In addition, the study focused on older adults, a group potentially vulnerable to noise exposure that has thus far received little attention in the literature. Furthermore, exposure to air pollution was considered in a sub-sample analysis, allowing the study to test for any associations between noise and the outcome variables that are independent of this other environmental stressor.
Acknowledgements
The authors are grateful to The Irish Longitudinal Study on Ageing (TILDA) for providing access to the microdata files, and to the EPA for air quality data. Researchers who wish to use TILDA data may access the data here www.tilda.ie.
Disclosure statement
No potential conflict of interest was reported by the author(s).
Additional information
Funding
Notes on contributors
Ciarán Mac Domhnaill
Mr Ciarán MacDomhnaill is currently a PhD student in Economics at the University of St Andrews in the UK, and was a Research Assistant at the Economic and Social Research Institute (ESRI) in Dublin at the time the research was conducted.
Owen Douglas
Dr Owen Douglas is currently an EU Project Officer at the Eastern and Midland Regional Assembly in Dublin, and was a Postdoctoral Research Fellow in the School of Architecture, Planning and Environmental Policy at University College Dublin (UCD) at the time the research was conducted.
Seán Lyons
Dr Seán Lyons is an Associate Research Professor in Economics at the ESRI in Dublin, and an Associate Professor in Economics at the Department of Economics, Trinity College Dublin (TCD).
Enda Murphy
Professor Enda Murphy is the Principal Investigator of the NOISE-HEALTH project and Professor of Planning at the School of Architecture, Planning and Environmental Policy at UCD.
Anne Nolan
Dr Anne Nolan is an Associate Professor in Economics at the ESRI in Dublin, an Associate Professor in Economics at the Department of Economics, TCD and a Research Affiliate at the Irish Longitudinal Study on Ageing at TCD.
References
- Ader, R., 2000. On the development of psychoneuroimmunology. European journal of pharmacology, 405, 167–176. doi:10.1016/S0014-2999(00)00550-1
- Aich, P. and Potter, G., 2009. Modern approaches to understanding stress and disease susceptibility: a review with special emphasis on respiratory disease. International journal of general medicine, 19. 10.2147/IJGM.S4843
- Aves, C. and Williams, M., 2019. Urban air quality modelling of Dublin (Technical Report). Cambridge Environmental Research Consultants.
- Basner, M., et al., 2015. ICBEN review of research on the biological effects of noise 2011-2014. Noise & health, 17, 57. doi:10.4103/1463-1741.153373
- Berkman, L.F. and Syme, S.L., 1979. Social networks, host resistance, and mortality: a nine-year follow-up study of Alameda County residents. American journal of epidemiology, 109, 186–204. doi:10.1093/oxfordjournals.aje.a112674
- Clark, C. and Paunovic, K., 2018. WHO Environmental noise guidelines for the European Region: a systematic review on environmental noise and quality of life, wellbeing and mental health. International journal of environmental research and public health, 15 (11), 2400. doi:https://doi.org/10.3390/ijerph15112400
- Clark, C., Crumpler, C., and Notley, H., 2020. Evidence for environmental noise effects on health for the United Kingdom policy context: a systematic review of the effects of environmental noise on mental health, wellbeing, quality of life, cancer, dementia, birth, reproductive outcomes, and cognition. International journal of environmental research and public health, 17, 393. doi:https://doi.org/10.3390/ijerph17020393
- Cohen, S., Kamarck, T., and Mermelstein, R., 1983. A global measure of perceived stress. Journal of health and social behavior, 24, 385. doi:10.2307/2136404
- Craig, C.L., et al., 2003. International physical activity Questionnaire: 12-Country reliability and validity. Medicine & science in sports & exercise, 35, 1381–1395. doi:10.1249/01.MSS.0000078924.61453.FB
- Donoghue, O.A., et al., 2018. Cohort profile update: the Irish Longitudinal Study on Ageing (TILDA). International journal of epidemiology, 47. 1398–1398l. doi:10.1093/ije/dyy163
- Douglas, O. and Murphy, E., 2020. Assessing the treatment of potential effect modifiers informing World Health Organisation guidelines for environmental noise. International journal of environmental research and public health, 17, 315. doi:10.3390/ijerph17010315
- Environmental Protection Agency, 2018a. Noise Round 3 Rail (Lnight).
- Environmental Protection Agency, 2018b. Noise Round 3 Airport (Lnight).
- European Environment Agency, 2020. Environmental noise in Europe - 2020. European Environment Agency, Copenhagen.
- Ewing, J.A., 1984. Detecting alcoholism: the CAGE Questionnaire. Jama, 252, 1905. doi:10.1001/jama.1984.03350140051025
- Faulkner, J.P. and Murphy, E., 2022. Estimating the harmful effects of environmental transport noise: an EU study. The Science of the total environment, 811, 152313. doi:10.1016/j.scitotenv.2021.152313
- Glazener, A., et al., 2021. Fourteen pathways between urban transportation and health: a conceptual model and literature review. Journal of transport & health, 21, 101070. doi:10.1016/j.jth.2021.101070
- Hyde, M., et al., 2003. A measure of quality of life in early old age: the theory, development and properties of a needs satisfaction model (CASP-19). Aging & mental health, 7, 186–194. doi:10.1080/1360786031000101157
- Hygge, S., 2003. Noise exposure and cognitive performance – Children and the elderly as possible risk groups, WHO Report Brussels. University of Gavle, Sweden.
- Kearney, P.M., et al., 2011. Cohort Profile: the Irish Longitudinal Study on Ageing. International journal of epidemiology, 40, 877–884. doi:10.1093/ije/dyr116
- Kreuzberger, N., et al., 2019. Transport-Related noise exposure in a representative sample of Île-de-France residents: a data-enrichment approach. Journal of transport & health, 12, 220–228. doi:10.1016/j.jth.2019.02.002
- Mac Domhnaill, C., et al., 2021. Road traffic noise and cognitive function in older adults: a cross-sectional investigation of the Irish Longitudinal Study on Ageing. BMC public health, 21, 1814. 10.1186/s12889-021-11853-y
- Mahesh, S., 2021. Exposure to fine particulate matter (PM2.5) and noise at bus stops in Chennai, India. Journal of transport & health, 22, 101105. doi:10.1016/j.jth.2021.101105
- Meyer, T.J., et al., 1990. Development and validation of the Penn State Worry Questionnaire. Behaviour research and therapy, 28, 487–495. doi:10.1016/0005-7967(90)90135-6
- Murphy, E., Faulkner, J.P., and Douglas, O., 2020. Current state-of-the-art and new directions in strategic environmental noise mapping. Current pollution reports, 6, 54–64. doi:10.1007/s40726-020-00141-9
- Murphy, E. and King, E.A., 2022. Environmental noise pollution: noise mapping, public health, and policy. 2nd ed. Amsterdam ; Boston: Elsevier.
- Radloff, L.S., 1977. The CES-D scale: a self-report depression scale for research in the general population. Applied psychological measurement, 1, 385–401. doi:10.1177/014662167700100306
- Recio, A., et al., 2016. The short-term association of road traffic noise with cardiovascular, respiratory, and diabetes-related mortality. Environmental research, 150, 383–390. doi:10.1016/j.envres.2016.06.014
- Silva, L.T. and Mendes, J.F.G., 2012. City Noise-Air: an environmental quality index for cities. Sustainable cities and society, 4, 1–11. doi:10.1016/j.scs.2012.03.001
- Tzivian, L., et al., 2017. Associations of long-term exposure to air pollution and road traffic noise with cognitive function—an analysis of effect measure modification. Environment international, 103, 30–38. doi:10.1016/j.envint.2017.03.018
- van Kamp, I. and Davies, H., 2013. Noise and health in vulnerable groups: a review. Noise & health, 15, 153. doi:10.4103/1463-1741.112361
- WHO, 2011. Burden of disease from environmental noise: quantification of healthy life years lost in Europe. World Health Organization Regional Office for Europe, Copenhagen.
- WHO, 2018. Environmental noise guidelines for the European Region.
- Xu, C., et al., 2020. Study on environmental Kuznets Curve for noise pollution: a case of 111 Chinese cities. Sustainable cities and society, 63, 102493. doi:10.1016/j.scs.2020.102493
- Zigmond, A.S. and Snaith, R.P., 1983. The Hospital Anxiety and Depression Scale. Acta psychiatrica Scandinavica, 67, 361–370. doi:10.1111/j.1600-0447.1983.tb09716.x
Appendix A.
Sample size
Appendix B.
Additional tables
Table B1: Average marginal effects, CASP-12 scale
Table B2: Sub-sample analysis average marginal effects, CASP-12 scale