Abstract
Epidemiological modeling is an important problem around the world. This research presents COVID-19 analysis to understand which model works better for different regions. A comparative analysis of three growth curve fitting models (Gompertz, Logistic, and Exponential), two mathematical models (SEIR and IDEA), two forecasting models (Holt's exponential and ARIMA), and four machine/deep learning models (Neural Network, LSTM Networks, GANs, and Random Forest) using three evaluation criteria on ten prominent regions around the world from North America, South America, Europe, and Asia has been presented. The minimum and median values for RMSE were 1.8 and 5372.9; the values for the mean absolute percentage error were 0.005 and 6.63; and the values for AIC were 87.07 and 613.3, respectively, from a total of 125 experiments across 10 regions. The growth curve fitting models worked well where flattening of the cases has started. Based on region's growth curve, a relevant model from the list can be used for predicting the number of infected cases for COVID-19. Some other models used in forecasting the number of cases have been added in the future work section, which can help researchers to forecast the number of cases in different regions of the world.
Disclosure statement
No potential conflict of interest was reported by the author(s).
Additional information
Notes on contributors
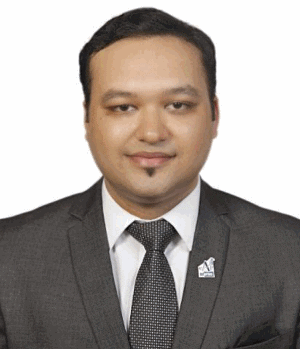
Rajan Gupta
Rajan Gupta is a Research & Data Science Professional working on different analytical problems. He completed his PhD from University of Delhi in Data Science & Information Systems. He is currently serving as Postdoctoral Research Fellow at the Centre of Information Technologies and Applied Mathematics, University of Nova Gorica, Slovenia, and Analytical Consultant to a few global organizations. He has authored four books and published over 75 research papers at various national and international forums. He has served as Assistant Professor of Data Science at University of Delhi, India. He is also a Certified Analytics Professional (CAP) from INFORMS, USA, and holds GStat Accreditation from American Statistical Association. He is a member of Analytics Certification Board (ACB) and CAP Ambassador of Asia Region for INFORMS, USA. He has contributed to the United Nation's report on E-Governance Development Index 2020 and has been an invited speaker at various forums. His area of interest includes Data Science, Machine Learning, Artificial Intelligence, Biostatistics, Information Security, E-Governance, Algorithmic Government, and Healthcare.
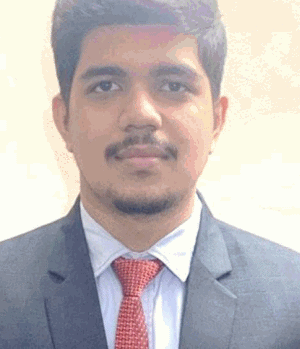
Gaurav Pandey
Gaurav Pandey is a research scholar and has completed his Education and Research Work at The NorthCap University, Gurugram, India. He has interned with ISRO, India, and is currently associated as Junior Data Scientist at THREEXFIVE CONSULTANCY LLP (TCF Consultancy). His area of interest includes Deep Learning, Machine Learning, Artificial Intelligence, and Healthcare.
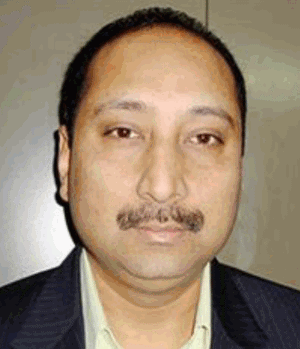
Saibal K. Pal
Saibal K. Pal is a Senior Scientist at Defense Research and Development Organization, Government of India, India. He completed his PhD from University of Delhi in Computer Science and has authored multiple books and over 300 research papers at various national and international forums. His area of interest includes Deep Learning, Distributed and High Performance Computing, Machine Learning, Artificial Intelligence, E-Governance, Algorithmic Government and Security.