ABSTRACT
The purpose was to investigate the effects of travel on performance in the National Rugby League (NRL). A total of 4,704 observations from 2,352 NRL matches (2007–2019) were analysed. The effect of travel on match outcome (i.e., win/loss) was analysed using a generalized linear mixed model, and the points difference using a linear mixed model. For every 1,000 km travelled in the NRL, the estimated probability of winning a match was reduced by −2.7% [−5.7 to 0.3%] and the estimated points difference by −1.1 [−2.0 to −0.2] points. In relation to every 1,000 km travelled, the 2007–2009 seasons had the greatest reduction in the likelihood of winning a match (−2.7% [−4.7 to −0.6%]), with the 2018–2019 seasons having the greatest likelihood (1.1% [−1.2 to 3.3%]). Regarding inter-state travel, teams from the state of Queensland had the greatest reduction in the likelihood of winning a match while the team from the state of Victoria had the greatest likelihood, although there were no clear differences between states. These data suggest that travel has impacted performance in NRL matches although this effect has reduced over time. These findings are useful for practitioners that prepare athletes in sports where frequent short-haul travel is required.
Introduction
Since the inaugural 1998 National Rugby League (NRL) season, there have been various changes to the number of teams involved; however, the current make-up and format of the competition has remained unchanged from 2007 (NRL Citation2019). There are eight matches between the 16 teams each week across Australia and New Zealand, which are typically played throughout Thursday to Monday, with varying kick-off times. Therefore, given the schedule of NRL matches, travel is potentially significant to the performance of teams in the competition.
Teams in the NRL undertake a return journey every two weeks on average during the season and despite travel typically being short-haul (e.g., 1–3 but up to 6 hours), travel fatigue can still occur (Waterhouse et al. Citation2004; McGuckin et al. Citation2014). Different to jet lag, travel fatigue is temporary exhaustion and tiredness that accumulates over time (Waterhouse et al. Citation2007; Samuels Citation2012). The potentially negative effects of travel on performance have previously been assessed in rugby union (George et al. Citation2015; Lo et al. Citation2019), American football (Fullagar et al. Citation2019), netball (Boothby et al., Citation2018; Bishop Citation2004), and Australian football league (Richmond et al. Citation2007). However, similar information for the NRL is currently unknown.
Two studies have previously examined the effects of travel on individual NRL teams (McGuckin et al. Citation2014; Fowler et al. Citation2016). However, no studies have investigated the effects of travel on measures of performance such as match outcome, throughout the entire NRL. Therefore, the purpose of this study was to investigate the effects of travel on performance in all NRL teams over a 13-year period. The study had three research questions; 1) Does travel affect performance in the NRL? 2) Have the effects of travel on performance changed over 13 seasons? 3) Is the performance of certain states or regions of Australia and New Zealand affected more than others by travel?
Methods
Design
Data from 13 seasons (2007–2019) of matches were obtained from the official NRL website (NRL Citation2019) and were analysed using an exploratory retrospective design.
Participants
All 192 matches from each season were initially included in the dataset, providing a total of 4,992 observations (2,496 matches). Due to a relatively small sample (0.5%), 12 drawn matches were excluded, in addition to 132 matches where teams played a home fixture outside of their home city. Play-off matches were not included. There was a total of 4,704 observations from 2,352 league matches used in the analysis. All data were freely available in the public domain and therefore ethics approval was not required. The ethics guidelines and principles of the lead author’s institution were adhered to throughout.
Procedures
Performance was measured using; the binary match outcome of win or loss and the continuous variable of points difference between scored and conceded. Away travel (km) was calculated using a function in Google Maps© (https://www.google.com.au/maps/; Mountain View, CA) that provided a straight line distance between the venue of the match and the away teams home stadium (Fullagar et al. Citation2019). Return travel was not considered as part of the match in question as this occurred following the fixture. The home venue of each team was identified and used as the reference for calculating travel distance.
Statistical analyses
Descriptive statistics are reported as mean (standard deviation) unless stated otherwise. The data were imported into R (version 4.0.2, R Foundation for Statistical Computing, Vienna, Austria) for analysis with the lme4 package (Bates et al. Citation2020). Match outcome was analysed via logistic regression using a generalized linear mixed model, and the points difference was analysed using a linear mixed model. Effects were converted to estimated probability of winning a match or points difference per 1,000 km travelled with uncertainty expressed as 95% confidence intervals (CI). For research question one, linear numeric fixed effects were included for travel distance, away-match disadvantage, turnaround time, and the opposition’s final league ranking and are presented as odds ratios (OR). The random effect was the team identity nested within season. For research questions two and three, interaction effects were added to assess the change/difference in the impact of travel upon performance over time and across states/regions, respectively. The ‘season’ variable was originally explored as a linear effect but after initial inspection, the effect was non-linear and therefore was parsed into five levels (i.e., 2007–2009, 2010–2012, 2013–2014, 2015–2017, 2018–2019) to allow for non-linear changes over time. These models included a random effect for team identity only. The ‘state/region’ variable was treated as categorical (i.e., Auckland (n = 1), New South Wales (n = 11), Queensland (n = 3), Victoria (n = 1)). The emmeans package (Lenth et al. Citation2020) was used to report pairwise contrasts and estimated marginal means.
Results
shows the main effect of travel on performance. For every 1,000 km travelled, the estimated probability of winning a match was reduced by −2.7% [−5.7 to 0.3%] and the estimated points difference was reduced by −1.1 [−2.0 to −0.2] points. Analysis of the covariates demonstrated that the away team had an OR of 0.56 [0.48 to 0.66] of winning a given match, and were estimated to have a points difference of −6.0 [−7.1 to −4.9] points. Every additional day turnaround between matches was associated with an OR of 0.98 [0.97 to 1.02] on winning a match, with an estimated points difference of −0.1 [−0.3 to 0.1] points. Playing a team one position lower in the final league standings was associated with an OR of 1.15 [1.14 to 1.17] in regards to winning the match and was estimated to result in a 1.2 [1.1 to 1.3] points difference. presents the effects of travel for each season category. displays the effects of travel for each state/region.
Figure 1. The effect of travel on the estimated probability of winning a fixture (a) and estimated points difference (b).
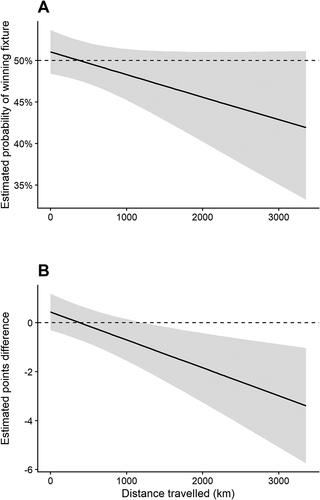
Figure 2. The effects of 1000 km of travel on the estimated probability of winning a fixture (a) and estimated points difference (b) across each season category.
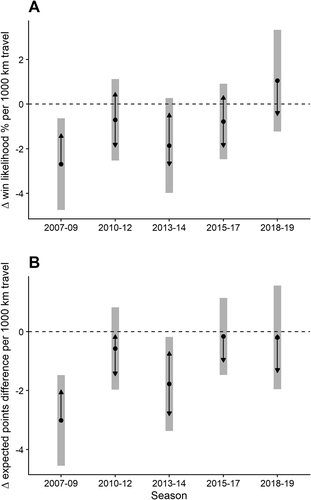
Figure 3. The effects of 1000 km of travel on the estimated probability of winning a fixture (a) and estimated points difference (b) across each state.
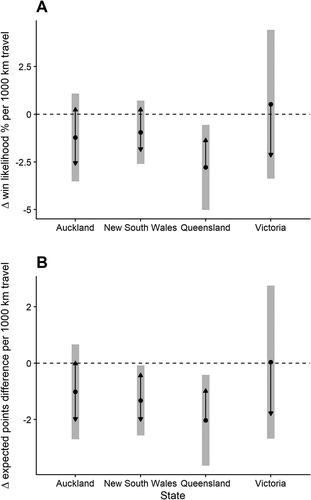
Discussion
The aim of the study was to investigate the effects of travel on performance for all NRL teams over a 13-year period (2007–2019). The main findings were that for every 1,000 km travelled, the estimated probability of winning a match was reduced by −2.7% [−5.7 to 0.3%] and the estimated points difference by −1.1 [−2.0 to −0.2] points. This is the first study to analyse the effects of travel on performance in the entire NRL competition. These findings demonstrate that travel can negatively affect performance and can support practitioners in their preparation of athletes.
On initial inspection, a reduction of −1.1 [−2.0 to −0.2] points and a −2.7% [−5.7 to 0.3%] likelihood in winning per 1,000 km might seem trivial. However, as teams regularly travel over >2000 km for away matches, a resultant −2.2 points and −5.4% winning likelihood can occur. Notably, 15% of the 2,352 matches in the study were decided by two points or less and in 12 of the 13 seasons, making the play-offs was determined by two competition points (i.e., the points allocated for a single win). Thus, when placed into context, changes of this magnitude might take on greater importance.
Playing away from home had an OR of 0.56 [0.48 to 0.66] and therefore is associated with a decrease in the odds ggof winning. Home advantage has previously been shown to exist (Goumas Citation2014; Lo et al. Citation2019; Fullagar et al. Citation2019). Through surveys with NRL players, McGuckin et al. Citation(2015) identified that the home crowd, normal travel/transport and the presence of family/friends were the key factors that players perceived to have a positive influence on performance in home matches. When playing away from home, the same players ranked the different meals and sleeping arrangements as the largest negative influences, which might explain some aspects of the reduction in performance (McGuckin et al. Citation2015).
There was an increase in total travel from 2007 to 2019 in the NRL, although a diminishing effect of travel on performance was found. These findings are similar to that of a study in rugby union (Lo et al. Citation2019), which found that the impact of travel reduced over time, and might be linked to improvements in travel fatigue management, the recovery strategies implemented, or the increasing professionalism and physical development of athletes. The current study also showed that in regards to inter-state travel, there were no clear differences between the states/regions. Notably, the one team based in Victoria showed a 0.5% [−3.4 to 4.4%] increase in the likelihood of winning a given match per 1,000 km travelled and no effect on points difference (0.0 [−2.7 to 2.7] points). Given this team won the league six times in the 13 seasons, this suggests that a teams ability can potentially offset the negative effects of travel on performance. The differing effects of travel on teams within the same state/region might have contributed to the lack of differences and suggests analysis on a team-by-team basis is required.
It is important to acknowledge this study was unable to account for or collect data on all factors associated with travel, and more individualised measures of athlete responses are required. Additionally, travel undertaken by teams or individual players outside of matches was unknown and therefore could not be included.
Practical Applications
Coaches could use the information provided to estimate the potential negative effect of their journey on performance in NRL matches. Consequently, this might inform several aspects of athlete preparation, including; training, travel logistics, sleeping arrangements and nutritional options.
Conclusion
This study investigated the effects of travel in the NRL. For every 1,000 km travelled, the estimated probability of winning a match was reduced by −2.7% [−5.7 to 0.3%] and the estimated points difference was reduced by −1.1 [−2.0 to −0.2] points. In summary, these data suggest that travel has impacted performance in NRL matches although this effect has reduced over time.
Acknowledgements
The authors can confirm no conflict of interest. No external financial support was provided for this study.
Disclosure statement
No potential conflict of interest was reported by the authors.
Additional information
Funding
References
- Bates D, Maechler M, Bolker B, Walker S, Christensen R, Singmann H, Dai B, Grothendieck G. Linear Mixed-Effects Models using “Eigen” and S4. Published online 2020.
- Bishop D. 2004. The effects of travel on team performance in the Australian national netball competition. J Sci Med Sport. 7(1):118–122. doi:https://doi.org/10.1016/S1440-2440(04)80050-1.
- Boothby L, Dawson B, Peeling P, Wallman K, O’Donoghue P. 2018. Effect of travel and fixture scheduling on team performance in the Trans-Tasman Netball League. New Zeal J Sport Med. 45(2):54–63.
- Fowler P, Duffield R, Lu D, Hickmans J, Scott T. 2016. Effects of long-haul transmeridian travel on subjective jet-lag and self-reported sleep and upper respiratory symptoms in professional rugby league players. Int J Sports Physiol Perform. 11(7):876–884. doi:https://doi.org/10.1123/ijspp.2015-0542.
- Fullagar H, Delaney J, Duffield R, Murray A. 2019. Factors influencing home advantage in American collegiate football. Sci Med Footb. 3(2):163–168. doi:https://doi.org/10.1080/24733938.2018.1524581.
- George T, Olsen P, Kimber N, Shearman J, Hamilton J, Hamlin M. 2015. The effect of altitude and travel on rugby union performance: analysis of the 2012 super rugby competition. J Strength Cond Res. 29(12):3360–3366. doi:https://doi.org/10.1519/JSC.0000000000001204.
- Goumas C. 2014. Home advantage in Australian soccer. J Sci Med Sport. 17(1):119–123. doi:https://doi.org/10.1016/j.jsams.2013.02.014.
- Lenth R, Singmann H, Love J, Buerkner P, Herve M emmeans: estimated marginal means, aka least-squares means. Published online 2020.
- Lo M, Aughey R, Hopkins W, Gill N, Stewart A. 2019. Out of your zone? 21 years of travel and performance in super Rugby. J Sports Sci. 37(18):2051–2056. doi:https://doi.org/10.1080/02640414.2019.1620427.
- McGuckin T, Sinclair W, Sealey R, Bowman P. 2014. The effects of air travel on performance measures of elite Australian rugby league players. Eur J Sport Sci. 14(Suppl 1):116–122. doi:https://doi.org/10.1080/17461391.2011.654270.
- McGuckin T, Sinclair W, Sealey R, Bowman P. 2015. Players’ perceptions of home advantage in the Australian rugby league competition. Percept Mot Ski Exerc Sport. 121(3):666–674. doi:https://doi.org/10.2466/06.PMS.121c28x4.
- NRL. NRL Draw. Published 2019. [accessed 2019 Oct 7]. https://www.nrl.com/draw/.
- Richmond L, Dawson B, Stewart G, Cormack S, Hillman D, Eastwood P. 2007. The effect of interstate travel on the sleep patterns and performance of elite Australian rules footballers. J Sci Med Sport. 10(4):252–258. doi:https://doi.org/10.1016/j.jsams.2007.03.002.
- Samuels C. 2012. Jet lag and travel fatigue: a comprehensive management plan for sport medicine physicians and high-performance support teams. Clin J Sport Med. 22(3):268–273. doi:https://doi.org/10.1097/JSM.0b013e31824d2eeb.
- Waterhouse J, Reilly T, Atkinson G, Edwards B. 2007. Jet lag: trends and coping strategies. Lancet. 369(9567):1117–1129. doi:https://doi.org/10.1016/S0140-6736(07)60529-7.
- Waterhouse J, Reilly T, Edwards B. 2004. The stress of travel. J Sports Sci. 22(10):946–966. doi:https://doi.org/10.1080/02640410400000264.