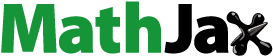
ABSTRACT
Much ink has been spilled to explain how the relative socio-economic position of natives and immigrants might shape anti-immigrant sentiment. However, little effort has been made to pit three competing theories: last place aversion, labour market competition and the welfare state model directly against each other, despite their often-times contradicting implications. This paper tests these alternative theories in Hungary, where the 2015 migration crisis has catapulted a previously hardly salient topic into the forefront of issues on the political agenda. Utilizing a choice-based conjoint experiment, administered on a nationally representative sample of voting-age Hungarians in Spring 2021, aforementioned hypotheses are tested in a country known for its hardline stance on migration. Results suggest fears related to welfare exploits might be the most plausible explanation for widespread anti-immigrant sentiment in the Hungarian public, as the less education fictive refugee profiles showcased, the less likely respondents were to admit them. Contrarily, no evidence corroborating worries associated with labour market competition or last place aversion was found. Future research could explore whether skills mismatch makes natives less wary of labour market competition, as well as the extent to which the 2022 Russian invasion of Ukraine altered Hungarians' attitudes.
Introduction
The 2015 refugee crisis intensified the discourse about immigration in countries previously indifferent to migration. Since then, the religious, ethnic and socio-economic background of refugees arriving to Europe has been subject of heated and politicized debates even in Central Eastern Europe (CEE), where the issue used to be hardly salient before (Lancaster Citation2022). Some countries proved to be more tolerant towards refugees and immigrants, while others remained dismissive of any forms of inclusion. Hungary is considered as an extreme case of rejecting refugees and immigrants, since both the government's communication, as well as the public reaction was outstandingly hostile and prejudicial, despite the low perception rate of immigrants (Czymara Citation2020). Therefore, Hungary is a suitable case for studying attitudes towards immigrants, since anti-immigration sentiments are still salient and prevalent. Exploring the roots of such sentiments in Hungary could bring us closer towards understanding the causes of prejudice and how to mitigate them.
Which are the factors that most effectively drive anti-immigrant attitudes? In this paper, we test theories related to the perceived social position of individuals and refugees. We rely on respondent-refugee comparisons with respect to skills possessed (proxied by level of education), financial situation and education. We test three competing hypotheses: last-place aversion, fears related to labour market competition and the welfare state model to explain anti-immigrant attitudes in Hungary. For this, we conducted a conjoint experiment (e.g. Christensen et al. Citation2021; Hainmueller, Hopkins, and Yamamoto Citation2014), where respondents were asked to choose between two refugee profiles. Varying characteristics of fictive asylum seekers allowed for testing multiple hypotheses simultaneously.Footnote1
In this article, we seek to make three contributions. To our knowledge, this paper constitutes the first direct test of last place aversion in the context of immigration preferences to date. Furthermore, it uses real-world data on asylum seekers' socio-economic background when creating distributions for profile randomization, providing greatly improved external validity (de la Cuesta, Egami, and Imai Citation2022) compared to conjoint analyses reported in the vast majority of recent studies that utilize similar designs on European samples (e.g. Bansak, Hainmueller, and Hangartner Citation2016). Lastly, it uses original data collected after the 2015 refugee crisis, but before the Russian invasion of Ukraine, which could help us to better understand anti-Muslim and anti-immigrant sentiment in Eastern Europe (De Coninck Citation2023).
In the next section, we describe our theoretical framework in more detail and provide a brief justification of case selection. This will be followed by a section on data and methodology. The results and discussion section presents the lessons of the conjoint experiment, whereas the last section concludes and elaborates on the generalizability of findings in the wider European context.
Explaining anti-immigration attitudes
The following section describes the three competing hypotheses in more detail. Each of them highlights the role of self-comparisons within a society based on income and level of education.Footnote2 Broadly speaking, these hypotheses capture various dimensions of perceived competition for resources: group conflicts previously linked to attitudes towards immigrants (Esses, Jackson, and Armstrong Citation1998). Each subsection concludes with the respective operationalized hypothesis. summarizes their observable implications.
Table 1. The hypotheses tested in this paper, the conjoint features (attributes) operationalizing them and their respective formal tests.
Last-place aversion
Last-place aversion (LPA) has its origins in game theory: using experimental and survey evidence, it was demonstrated that people with low-income do not support equal or larger redistribution policies (Kuziemko et al. Citation2014). This is because they are last-place averse, i.e. would like to avoid being last at all costs. Experimental subjects choose to gamble during a money-transfer game if there was any chance to move up from the last place. Kuziemko et al. (Citation2014) also found that people who earn only a little above the minimum wage will be the most likely to oppose raising the minimum wage, as they would lose their advantage vis-á-vis another group that is below them in the social hierarchy.
LPA was also documented in the context of wine choices. Restaurant guests are most likely to choose the second cheapest wine from the menu to avoid the feeling of being last (McFadden Citation1999). Setting up artificial online queue lines, Buell (Citation2021) found experimentally that last-place participants who were shown their rank left the queue, demonstrating last-place averse predispositions. Zhou and Soman (Citation2003) also pointed out that people become calmer if the number of people queuing behind them increased, despite their position remaining unchanged. This behaviour can be explained by individuals basing self-evaluations on downward social comparisons, as comparing themselves to another group which is worse-off contributes to feeling better about one's position in the social hierarchy (Wills Citation1981).
Although there are relatively few empirical attempts to model differences between immigrants and natives with LPA (Alesina, Miano, and Stantcheva Citation2022; Poutvaara and Steinhardt Citation2018), the logic of comparison is worth applying in our research, as last-averse behaviour has been shown to explain voting behaviour and support for welfare retrenchment in Hungary (Gidron and Hall Citation2017; Róna et al. Citation2020). In the context of immigration, LPA would predict that people with limited economic resources will support the arrival of (only) those immigrants who possess even less goods and thus are able to replace them in the perceived last place within the social hierarchy.
H1: Respondents will choose those refugees, who are worse-off than themselves, if this allows them to leave their last place behind. That is, if the asylum seeker is worse-off than a last-place respondent, and consequently the admission of such refugees would move the respondent higher up in the social echelons, the likelihood of the respondent selecting the profile will be higher, compared to profiles that would leave the respondent in the last position.
Labour market competition
Skill level also has a great impact on attitudes toward immigrants. Low-skilled natives dislike immigrants in general, and those who work in high-skilled jobs are more likely to support immigration. One of the reasons for this is high skilled people believing that they will not have to compete with immigrants on the job market (Ortega and Polavieja Citation2012).
Labour market competition (LMC) provides another explanation for how self-comparison might shape preferences on immigration. According to Helbling and Kriesi (Citation2014), low-skilled native people will oppose low-skilled immigrants since they would be their competitor on the labour market. Under the closed economy factor proportions model, local workers' wages go down when similarly skilled migrants join the labour market (Scheve and Slaughter Citation2001). Also, high-skilled native people welcome low-skilled immigrants, since those looking for blue-collar jobs would not be their competitors on the labour market; however, they oppose high-skilled immigrants for similar reasons (Facchini and Maria Mayda Citation2012).Footnote3 We proxy being high-skilled or low-skilled with the level of education. While not identical, these are certainly related theoretical concepts, and this allows for direct comparison between refugees and respondents, while keeping the realism of treatment as high as possible. This is in line with previous literature scrutinizing the impact of immigration on the labour market, which have often used education as a proxy for skill groups (Borjas Citation2003; Hainmueller, Hiscox, and Margalit Citation2015, 194).Footnote4
H2: Respondents will not choose a refugee who possesses the same skills (proxied by educational attainment) as themselves. In other words, both refugee profiles with higher and lower levels of education, relative to the respondent's level of education, will be preferred to profiles showcasing identical levels of schooling as survey takers.
Welfare state model
As an alternative for the LMC model, which compares natives and immigrants with the same skill level, the welfare state model (WSM) describes the relationship between natives and low-skilled immigrants. According to Helbling and Kriesi (Citation2014), high-skilled natives in Switzerland might oppose low-skilled immigrants, because they believe that immigrants would disproportionately use public services, such as health care. They hypothesize (596) that ‘high-skilled immigrants are preferred over low-skilled, as the latter's use of public services especially with regard to welfare assistance and health services is often disproportionally higher than their contribution to tax revenues’. For this reason, high-skilled natives are more likely to favour high-skilled immigrants. As low-skilled migrants are expected to impose an additional tax burden on locals and cause a decrease in per capita transfers (Hainmueller and Hiscox Citation2010; Hanson, Scheve, and Slaughter Citation2007), hostility towards immigrants could be explained by fears of losing social benefits (Aleksynska Citation2011). High-skilled native individuals fear the potential tax raises needed to cover the increased welfare benefits once more immigrants arrive (Facchini and Maria Mayda Citation2009). Indeed, Hero and Preuhs (Citation2007) found that the benefits had been cut in those US states which had higher numbers of immigrants. Immigrants described as having received welfare benefits previously – used as a ‘signal of being a potential burden to the welfare system’ – are also perceived as more threatening (Ruedin Citation2020, 9).
But implications of the WSM are not restricted to a juxtaposition of high-skilled natives versus low-skilled immigrants. Low-skilled natives might also oppose immigrants with little or no qualifications. This is because natives were to expect their government to rather cut welfare expenditures than to raise more taxes (Hanson, Scheve, and Slaughter Citation2007). Therefore, residents are afraid of reduced welfare benefits. Consequently, both low and high skilled natives could oppose low-skilled immigrants. Other studies also demonstrate that natives prefer high-skilled immigrants over low-skilled ones, primarily out of these concerns (Hainmueller, Hiscox, and Margalit Citation2015; Sniderman, Hagendoorn, and Prior Citation2004). To sum it up, WSM expects natives, irrespective of how skilled they are, to prefer skilled immigrants over less skilled ones, potentially out of fears related to exploiting public services. Building on these findings, our third hypothesis conjectures that:
H3: Respondents will not prefer refugees who only have limited education. The hypothesis expects that the two lowest levels of education (haven't finished elementary school/elementary school only) will diminish the likelihood of selecting the profile. That is, changing a refugee's level of educational attainment from any other levels to these two will be associated with lower probabilities of respondents choosing that particular profile.
Hungary: a country of extreme anti-immigration attitudes
Previous research has already documented the long-lasting presence of anti-immigration sentiment in Hungary, even before it was amplified by the Hungarian government's xenophobic campaign (Meuleman, Davidov, and Billiet Citation2009). Governing Fidesz aside, opposition parties were also keen on harnessing exclusionary attitudes (Czymara Citation2020). The Green Party proposed the mandatory tracking of asylum seekers with ankle monitors and a notable share of their voters believes immigrants pose a threat to Hungarian culture (Kovarek and Littvay Citation2019). When elected as mayor, Our Homeland's chairman banned the building of mosques and wearing of the burqua (Kovarek et al. Citation2017). However, the basic motivations of people who reject the arrival and settling of immigrants still remain unclear. Why do most Hungarians refuse to admit refugees? Which refugee characteristics are decisive when preferences are formed regarding admitting them (or denying their entry)?
Despite the ever-present xenophobic tendencies in the Hungarian society, the explicit rejection of Muslim people solidified only recently. Before the 2015 migration crisis, the issue of immigration was hardly salient in Hungary. To regain their electoral support after a decline, Viktor Orbán's government has been implementing an intensive campaign against immigrants and refugees since 2015 (Czymara Citation2020). It ‘enlisted the support of state-run and state-sponsored media, framing the refugee crisis as a cultural threat and the danger of terrorism’ (Wenzel and Zerkowska-Balas Citation2019, 60). The negative campaign, involving billboards and television ads continued even when the number of asylum seekers in Hungary was negligible and meant the most important mobilizing resource for Fidesz in local, national and European Parliamentary elections. Fidesz mayors were also expected to help the government's anti-immigration agenda via petitions, symbolic resolutions and at meetings of inter-municipal organizations (Kovarek and Dobos Citation2023, 109). Based on the policy process analysis of Kriesi et al. (Citation2024), the Hungarian quota referendum of 2016 was the ‘most politicized of all national episodes’ of the entire European refugee crisis.
This political campaign exploited existing and well-documented hostilities of Hungarians towards foreigners (Meuleman, Davidov, and Billiet Citation2009) and intensified negative attitudes towards immigrants (Czymara Citation2020). As a result, the majority of Hungarians developed contradictory prejudices about migrants, believing that they do not intend to work at all while also assuming that they will steal Hungarians' jobs; or that they will exploit the social security system and live off the limited state resources poor Hungarians should be entitled to (Simonovits Citation2020). Unfolding the roots of these predispositions is the main focus of our study. Collecting novel data in Hungary after the 2015 refugee crisis also sets our research apart from previous studies exclusively focusing on the United States (Hainmueller and Hiscox Citation2010; Hainmueller, Hiscox, and Margalit Citation2015), as well as analyses scrutinizing attitudes in Europe that use survey data compiled before 2015 (Dancygier and Donnelly Citation2013; Hellwig and Kweon Citation2016).
Data and methods
We fielded a pre-registered conjoint survey experiment,Footnote5 embedded into a multi-investigator survey, presenting a pair of fictive refugee profiles, randomly varying levels of gender, country of origin, qualification, possessed devices and sum of money. Our main interest is the interplay between respondents' skills (education), as well as tangible possessions (devices, sum of money) and those of refugees. The forced-choice experimental designFootnote6 reports on two refugees, as if they were just found at the Hungarian border by police officers; consequently, we have information about their belongings: whether they had a phone with themselves, whether they were carrying a computer (e.g. in their backpack), as well as how much cash they had in their pockets. These information are presented as attributes and varied randomly, alongside other characteristics needed to establish external validity and to construct realistic profiles, such as level of education, gender and country of origin. Each respondent completed two choice tasks.
To test our hypotheses, we computed average marginal component effects (AMCEs) and measured subgroup differences, where subgroups are primarily defined by (1) individuals' relative status vis-á-vis other respondents (i.e. whether they qualify as ‘last place’) and (2) individuals' relative status vis-á-vis the refugee profiles (e.g. whether they are at equal standing regarding their level of education or whether the refugee qualifies as being in a more difficult financial and economic situation). We operationalize last place as the following: a respondent without phone and computer; a respondent who finished elementary school, but having no further schooling; a respondent with max. 100,000 HUF monthly income (but not without income).Footnote7 We operationalize a worse-off refugee as someone without phone and computer (as other valuable belongings are hard to carry along the journey, hence one can assume a respondent living in Hungary likely possess anything else which would then make them second-to-last following the refugee being admitted), a refugee without elementary schooling, as well as a refugee without any cash.
The order in which profile attributes appear were randomized across respondents (but were the same for the same respondents). The study manipulates fictive refugee characteristics: whether they appear as male or female, whether their country of origin is Afghanistan, Iraq, Pakistan, Iran or Syria; whether they lack elementary schooling, has only elementary schooling, finished high school or has a college degree; whether they have a phone, a phone and a computer, or neither; whether they have no cash, 100,000, 150,000, 200,000 or 500,000 HUF with them when interrogated by police officers. Attribute levels were randomly selected; for level of education, devices and sum of money we used a uniform distribution. Conversely, we used real-world distributions when existing data allowed us to do so (de la Cuesta, Egami, and Imai Citation2022).
Marginal distributions of actual asylum seekers' characteristics were available for country of origin and gender. De la Cuesta, Egami, and Imai (Citation2022) emphasize how randomization based on marginal distributions ‘greatly improves the external validity of conjoint analysis’ (31) and the ‘need to collect relevant data for as many factors as possible when building the target distribution’ (40) already in the design phase. The respective distributions were the following: 57% male and 43% female; 45% came from Afghanistan, 39% from Iraq, 6% from Pakistan, 5% from Iran and 5% from Syria. These values were based on data from Menedék Hungarian Association for Migrants and the Hungarian Central Statistical Office.Footnote8 The variable capturing sender countries justifies incorporating information on our target population of interest, as target profile distributions very different from a uniform distribution will push uniform AMCES far away from population AMCEs (similarly to interactions among profile attributes). With respect to country of origin, sticking to a uniform distribution would have yielded an AMCE that gives equal weights to all conjoint profiles, despite nearly half of all asylum seekers coming from Afghanistan, but very few arriving from Syria or Iran.Footnote9
Data was collected by an external survey firm, TÁRKI Social Research Institute. The probability sample of 1008 persons is constructed using Computer-assisted Personal Interviews (CAPI), applies random selection sampling and is representative of the Hungarian adult population.Footnote10 Each respondent had to complete two choice tasks, increasing the effective sample size to N = 2016.
Dichotomous variables capture (1) when the level of education of respondents and refugee profiles are the same; (2) when allowing the refugee to enter Hungary would move the respondent up from the last place; (3) when the respondent is classified as currently (i.e. before admitting the fictive refugee) occupying the last place.
The analysis obtains average marginal component effects (AMCEs) and marginal means (Hainmueller, Hopkins, and Yamamoto Citation2014; Leeper, Hobolt, and Tilley Citation2020) by using OLS regression.Footnote11 As standard in conjoint analysis, we cluster standard errors by respondents, to account for the fact that each respondent completed two choice tasks. The outcome variable for our regression analysis captures the chosen refugee profile, whereas profile attributes serve as predictors. We relied on the cregg package for obtaining our estimates (Leeper Citation2020). Differences in marginal means were calculated to obtain differences in preferences for particular levels of attributes between respondent subgroups. Pairwise difference in means tests compares unadjusted marginal means to provide a formal test of subgroup differences in favourability for each level of conjoint attributes. This is followed by an omnibus F-test of the null hypothesis that all interaction terms are equal to zero; in other words, we run a nested model comparison of the restricted model (no interactions) and the one allowing for interaction between conjoint attribute levels and respondent subgroups. Interactions, as specified above, test the hypotheses using the relative position of respondents and fictive refugees, regarding the devices possessed, income or cash, and level of education.
Results and discussion
We start our analysis by obtaining AMCEs () and marginal means () for the entire sample.Footnote12 These are not direct tests of any of our hypotheses, but rather informative with respect to the main drivers of anti-immigration sentiment in Hungary. AMCEs summarize the overall effect of an attribute across other attributes of refugee profiles. Unadjusted marginal means measure favourability toward attributes (profile features) and are calculated for each feature level. When it comes to population (or subgroup) preferences, that latter is preferred, as marginal means are estimated for all attribute levels, whereas AMCEs by design do omit producing estimates for the reference category (Leeper, Hobolt, and Tilley Citation2020).
For testing last place aversion, we calculated differences in marginal means for three separate models. The first distinguishes between respondents' and refugees' income level, the second relates to education. As we can see in and , none of the estimates are significantly different, meaning that we are unable to corroborate the respective hypothesis. According to our results, the desire to avoid the last place in society's hierarchy does not influence citizens' choice on immigrants' fictive profiles. A similar null result, i.e. the absence of meaningful differences between respondent subgroups, characterizes our third hypothesis on devices possessed, such as computers and phones (not shown visually). We test subgroup differences formally via nested model comparisons, computing an F-statistic that compares the model fit of restricted versus not restricted models (Leeper, Hobolt, and Tilley Citation2020). Latter models allow for interactions between attribute levels and subgroup dummies. In all three cases, respective omnibus F-tests also suggest the lack of subgroup differences expected by LPA.Footnote13
Figure 3. Differences in marginal means between respondents who are last with respect to education (i.e. finished elementary school only) and those who are not.
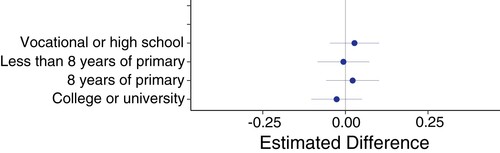
Figure 4. Differences in marginal means between respondent groups, distinguished by being last (i.e. earning a monthly salary of max. 100,000 HUF) and not last based on their income level.
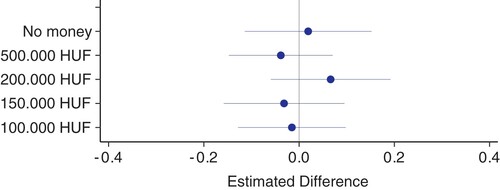
– both suggest that male asylum seekers face a disadvantage, whereas the Hungarian population demonstrates a preference for female refugees. In causal terms, presenting a male asylum seeker profile, all else constant, decreased the probability that the profile is chosen by 0.09 percentage points (SE = 0.02). This is in line with how young men were depicted in Hungarian (and Polish media) at the height of the Syrian refugee crisis, ‘portrayed as cowards who did not want to fight for their country’, op-eds and politicians alike arguing for ‘admit[ting] only women and children’ (Goździak and Márton Citation2018, 138). Contrarily, AMCEs (or marginal means) are statistically indistinguishable from zero (or 0.5) for any levels of country of origin, devices owned or cash possessed by notional refugees. Asylum seekers' level of education packs significant explanatory power: selecting less than 8 years of primary as baseline, all other levels (i.e. having received more education) increase the probability of the particular profile being chosen. suggest secondary and post-secondary education provide an advantage when it comes to preferences regarding admitting asylum-seekers, whereas refugees without a high school diploma are the least-liked category, irrespective whether they finished primary school or not. Bonuses (and penalties) associated with education are larger than the relative importance of gender. Changing a fictive asylum seeker's educational attainment from less than 8 years of primary school to college or university yields an average change of 0.32 percentage point increase (SE = 0.02) in the probability that the refugee will be admitted. We revisit this finding in more detail later, when formally testing Hypothesis 3.
One potential explanation for this could be our respondents having had trouble believing that these refugees will help them to leave rock bottom behind. Whether this has to do with the relatively low share of immigrants in Hungary (hence respondents expecting these refugees to immediately move further to Western Europe once granted asylum), or doubts about sincerity of claims describing their wealth , i.e. consuming news from government-sponsored media outlets could make one believe that refugees are stashing up cash and hiding it from authorities, or that their monthly allowance, received from UNHCR to ‘anonymous credit cards’, covers a lifestyle more comfortable than respondents' own. Further studies can look into which of these two explanations are more plausible – as their normative implications are vastly different.
We proceed by testing the labour market competition hypothesis; for this, we split respondents into two groups, based on their educational attainment. We proxy skill with level of education, similarly to previous research on the topic (Helbling and Kriesi Citation2014; Scheve and Slaughter Citation2001). A dichotomous variable operationalizes whether respondents had the same level of education as the fictive refugee (as opposed to it being either lower or higher). suggests these respondent subgroups do not differ significantly; contrary to what LMC would posit, asylum seekers possessing the same level of education (hence potentially becoming competitors of natives in job seeking) are not rejected more often than immigrants who are better (or worse) qualified, posing no immediate threat for natives' employability and labour market chances.
Figure 5. Differences in marginal means between respondents who possess the same level of education as fictive refugees and respondents with either more or less schooling.
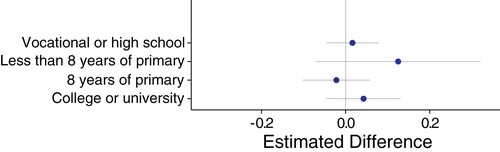
Therefore, our second hypothesis about respondents preferring immigrants whose level of education is not similar to theirs, was not corroborated either, as we were unable to reject the associated null hypothesis, expecting no significant differences between subgroups.Footnote14 This suggests that anti-immigrant sentiments harboured by Hungarians do not stem from fears related to an increasingly competitive labour market; in spite of the government's infamous ‘If you come to Hungary, don't take the jobs of Hungarians!’ billboards, an immigrant who received the same education is not disfavoured more.
We find remarkable differences in preferences regarding refugee profiles, however, in the direction expected by the welfare state model. To describe population preferences, presents raw marginal means as a test of the welfare state model hypothesis. These provide evidence for Hungarians rejecting immigrants without a high school diploma, whereas those with secondary education or higher (i.e. college or university) are strongly favoured based on our estimates. Refugees with the lowest level of education are the least likely to be selected.
Figure 6. Unadjusted (raw) marginal means indicating preferences for skill/education level of refugees.
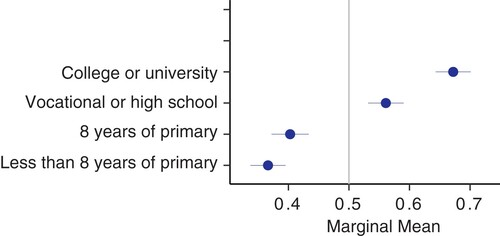
Our findings go in the same direction of recent literature on work values and cultural capital in Hungary (Kovarek and Sata Citation2021), highlighting that Hungarians value education to a great extent, which remains the most important source of social mobility. These results also mirror preferences in the USA and in Slovakia (Findor et al. Citation2022; Hainmueller and Hopkins Citation2015) and resonate with earlier findings on Hungarians being most dismissive of refugees who they think will exploit the welfare system by not being capable of finding jobs because they lack the proper skills (Simonovits Citation2020).
Conclusion
This article set out to capture attitudes on immigration in Hungary. We tested three competing hypotheses: last place aversion (LPA), labour market competition (LMC) and the welfare state model (WSM). We did so while seeking to make three contributions: utilizing real-world data on asylum seekers' socio-economic backgrounds for profile randomization; collecting data after the 2015 refugee crisis and offering a direct test of LPA in the context of immigration preferences. Out of the three aforementioned hypotheses, only fears related to refugees exploiting the welfare system were found to be significant drivers, as the less education refugees have, the less likely respondents were to admit them. These findings contribute to a small, but growing body of literature employing survey experiments to study (anti-)immigration sentiment in Central Eastern Europe (Findor et al. Citation2022; Wenzel and Zerkowska-Balas Citation2019).
Our findings go in the same direction of recent literature on attitudes towards immigrants, highlighting that female asylum seekers are generally preferred over male ones, an experimental result demonstrated in a variety of contexts across Western Europe, Central Eastern Europe and the United States (Bansak, Hainmueller, and Hangartner Citation2016; Findor et al. Citation2022; Hainmueller and Hopkins Citation2015). We expect our findings to generalize to other countries where incumbents (and other political elites) effectively shape individual attitudes on immigration via cueing (Kriesi and Vrânceanu Citation2023), national contexts of Central Eastern Europe where immigration remains salient on the political agenda, irrespective of the actual number of asylum seekers (Strnad Citation2022), and countries where educational attainment does not serve as a heuristic for (higher) likelihood of a refugee speaking the local language.Footnote15
A few caveats are in order. When testing the LMC hypothesis, we assumed that identical skills (proxied by educational attainment) would lead to identical occupational status, but this might not necessarily be the case. More research would be needed to understand whether skills mismatch characterizes immigrants' job prospects in Hungary – and whether this modifies our survey takers' expectations on asylum seekers' future entry to labour market.
While we have focused on testing the WSM, the literature also has much to offer scholars interested in proxying other adjacent but distinct hypotheses, similarly proxied by skills or education. The Deservingness model, as hypothesized by Helbling and Kriesi (Citation2014), captures the idea that high-skilled immigrants are more desirable for natives, because they consider low-skilled immigrants as ‘lazy people’. According to this sentiment, low-skilled immigrants would ‘would be as well off as natives’, if only they were more abitious and ‘tried harder’ (Helbling and Kriesi Citation2014, 598). Should conjectures about the sentiment of migrant undeservingness be true, its empirical implications would be similar to the WSM, as lower levels of education would be a sign of ‘lazyness’ (cf. Cowan, Martinez, and Mendiola Citation1997; Waters Citation1994), and similarly predict future dependence on (or exploitation of) the welfare state.
Further research should try to unpack the specific mechanisms at work behind natives overwhelming preference for high-skilled immigrants. Our research design does not allow for disentangling these two possibilities, but conjoint experiments with individual rating outcome variables (where survey takes would be asked to assess whether they associate fictive immigrant profiles with ‘lazyness’ or a potential burden for the country's tax base) would be well-suited to directly assess this question.
Furthermore, for constructing fictive asylum seeker profiles, we used real-world data on refugees' country of origin. Such figures reflect trends of asylum seekers before the Russian invasion of Ukraine, as our experiment was fielded in 2021. Future studies can look into how this drastic change – just over the course of a month, more than 500,000 Ukrainian refugees have entered Hungary – shaped preferences (De Coninck Citation2023). It is possible that Ukrainians being predominantly Christians would moderate some of the effects uncovered in this paper (Moise, Dennison, and Kriesi Citation2024). Alternatively, as there have been tens of thousands of Ukrainians guest workers in Hungary (infamously dubbed as ‘migrant workers’ by radical right political actors) already in 2019, a replication of this study might corroborate the LMC hypothesis.
Contributing to the growing number of studies seeking to understand individual preferences over immigration (Helbling and Kriesi Citation2014; Ortega and Polavieja Citation2012) with the relative socio-economic position of natives and immigrants, our study introduced last place aversion as mechanism potentially driving (anti-)immigrant sentiment. While leaving the last place behind might come with higher status in the social hierarchy, our null results associated with the respective hypothesis tests (H1) suggest asylum seekers might not be considered as a relevant reference ingroup, as Hungary remains a transit state and most refugees stay only temporarily (Kriesi et al. Citation2024). Further research should test the last place aversion hypothesis and evaluate the degree to which LPA is applicable in the context of destination and frontline states.
Supplemental Material
Download PDF (141.3 KB)Acknowledgments
We thank Dominik Duell, Patrick Mellacher, Natalia Dziadyk, Cagla Deste Ekin Guner, Giacomo Salvarani, Sanja Tepavcevic, Renato De Gaspi and Peter Visnovitz for comments that have improved the analysis presented here.
Disclosure statement
No potential conflict of interest was reported by the author(s).
Notes
1 Note that in the Hungarian public discourse, migrant, immigrant, refugee and asylum seeker are almost always used interchangeably, not independently of the Orbán government's efforts to associate symbolic threats with all sorts of migrants. In the words of Simonovits (Citation2020), the cabinet ‘purposefully refrained from’ using the latter two terms. We asked about ‘asylum seekers’ to justify the experiment's forced-choice nature, but the accompanying instructions also used expressions, which would resonate with Hungarians consuming news that frame all refugees as (illegal) immigrants. See the pre-analysis plan for the exact wording: https://osf.io/jkt9a?view_only=51246c3e43644f35ab11d85cd76e1380
2 In our study, we remain focused on material theories, expecting the relative and perceived social position of individuals to act as drivers of attitudes towards immigrants, consistent with the Sociotropic Economic Threat argument (Valentino et al. Citation2019). Our survey experimental setup is less well-suited to test security and cultural threat perceptions (Wike and Grim Citation2010) or anxiety about crime (Fitzgerald, Curtis, and Corliss Citation2012), and – as the Data and methods section elaborates on it below – we have little variance in terms of refugee profiles' religious background (Krzyzowski and Nowicka Citation2021), as there is no sizeable group of asylum seekers coming to Hungary from predominantly Christian sender countries. Nevertheless, we do not exclude the possibility that they might (just as well) be relevant in the Hungarian context.
3 See Natter, Czaika, and Haas (Citation2020) on how these divergent preferences regarding high versus low-skilled immigrants are represented by partisan actors in the political arena.
4 See also the overview of studies that estimate the labour market effects of immigration across skill groups, operationalized as education and experience (Edo Citation2019, 936).
5 PAP registration was initiated on 27 May 2021. As data was collected by an external survey firm, we had no access to any of the responses until the cleaned dataset was sent to us in June 2021.
6 Reviewing published research employing conjoint designs, Bansak et al. (Citation2021b, 26–27) find that forced-choice outcomes are one of the ‘two most common measures of stated preference in political science’ and have ‘distinct advantages’, such as they incentivize respondents to ‘think more carefully about trade-offs’.
7 This operationalization makes use of the omnibus survey's standard battery of socio-economic items, making direct refugee-respondent comparisons feasible. See Supplementary Material for saliency of these attributes in Hungarian discourse.
8 See https://menedek.hu/sites/default/files/article-uploads/integracio-onkentesalapon.pdf and https://www.ksh.hu/docs/hun/xstadat/xstadat_eves/i_wnvn002b.html, respectively.
9 Our approach mirrors a mixed randomization design, where a uniform distribution is used for the main factors of interest – in our study, cash, device and education –, and target profile distribution for control factors – in our study, country of origin and gender – included for the purposes of background information. This is, of course, less of a purposeful consideration from our side and rather a necessity, given that there was no available data on the marginal distributions for the rest of the attributes. This is, of course, a well-known problem: (de la Cuesta, Egami, and Imai Citation2022, 21) also emphasized how ‘it may not be feasible to obtain the joint distribution of the (potentially many) attributes of immigrants that researchers wish to study’.
10 See Supplementary Material for descriptives.
11 As the nonparametric estimator of the AMCE is the linear combination of the estimated regression coefficients (Hainmueller, Hopkins, and Yamamoto Citation2014), linear regression is generally preferred when estimating AMCEs (Bansak et al. Citation2021b).
12 Although our preferred method is graphical, we also include a tabular presentation of the estimates in Tables A3–A4 in the Supplementary Materials.
13 Results are () for last place as education, (
) as income and (
) as not owning a laptop, respectively.
14 Results of the omnibus F-test is () for labour market competition. Opearatinalizing LMC slightly differently – not testing interactions between a dichotomous variable capturing identical refugee/survey taker educational attainment and notional asylum seekers' level of education, but rather respondents' level of education in general – leaves the results unchanged (
).
15 None of our respondents would assume that an asylum seeker would be more likely to speak Hungarian just because they obtained a degree or finished high school. Consequently, AMCEs associated with (higher) levels of education indeed describe causal quantities of changing refugees' education received, and are not masking (Bansak et al. Citation2021a) the effect of speaking the vernacular. Contrarily, it would be a fair assumption by respondents in the USA (or the United Kingdom) that an immigrant having finished secondary or tertiary education studied English as a second language.
References
- Aleksynska, M. 2011. “Relative Deprivation, Relative Satisfaction, and Attitudes Towards Immigrants: Evidence from Ukraine.” Economic Systems 35 (2): 189–207. https://doi.org/10.1016/j.ecosys.2010.06.002.
- Alesina, A., A. Miano, and S. Stantcheva. 2022. “Immigration and Redistribution.” The Review of Economic Studies 90 (1): 1–39. https://doi.org/10.1093/restud/rdac011.
- Bansak, K., J. Hainmueller, and D. Hangartner. 2016. “How Economic, Humanitarian, and Religious Concerns Shape European Attitudes Toward Asylum Seekers.” Science (New York, NY) 354 (6309): 217–222. https://doi.org/10.1126/science.aag2147.
- Bansak, K., J. Hainmueller, D. J. Hopkins, and T. Yamamoto. 2021a. “Beyond the Breaking Point? Survey Satisfying in Conjoint Experiments.” Political Science Research and Methods 9 (1): 53–71. https://doi.org/10.1017/psrm.2019.13.
- Bansak, K., J. Hainmueller, D. J. Hopkins, and T. Yamamoto. 2021b. “Advances in Experimental Political Science.” In Conjoint Survey Experiments, edited by J. N. Druckman and D. P. Green, 19–41. Cambridge: Cambridge University Press.
- Borjas, G. J. 2003. “The Labor Demand Curve Is Downward Sloping: Reexamining the Impact of Immigration on the Labor Market.” The Quarterly Journal of Economics 118 (4): 1335–1374. https://doi.org/10.1162/003355303322552810.
- Buell, R. W. 2021. “Last-Place Aversion in Queues.” Management Science 67 (3): 1430–1452. https://doi.org/10.1287/mnsc.2020.3619.
- Christensen, H. S., T. Järvi, M. Mattila, and Å. von Schoultz. 2021. “How Voters Choose One Out of Many: A Conjoint Analysis of the Effects of Endorsements on Candidate Choice.” Political Research Exchange 3 (1): 1892456. https://doi.org/10.1080/2474736X.2021.1892456.
- Cowan, G., L. Martinez, and S. Mendiola. 1997. “Predictors of Attitudes Toward Illegal Latino Immigrants.” Hispanic Journal of Behavioral Sciences 19 (4): 403–415. https://doi.org/10.1177/07399863970194001.
- Czymara, C. S. 2020. “Propagated Preferences? Political Elite Discourses and Europeans' Openness Toward Muslim Immigrants.” International Migration Review 54 (4): 1212–1237. https://doi.org/10.1177/0197918319890270.
- Dancygier, R. M., and M. J. Donnelly. 2013. “Sectoral Economies, Economic Contexts, and Attitudes Toward Immigration.” The Journal of Politics 75 (1): 17–35. https://doi.org/10.1017/S0022381612000849.
- De Coninck, D. 2023. “The Refugee Paradox During Wartime in Europe: How Ukrainian and Afghan Refugees Are (Not) Alike.” International Migration Review 57 (2): 578–586. https://doi.org/10.1177/01979183221116874.
- de la Cuesta, B., N. Egami, and K. Imai. 2022. “Improving the External Validity of Conjoint Analysis: The Essential Role of Profile Distribution.” Political Analysis 30 (1): 19–45. https://doi.org/10.1017/pan.2020.40.
- Edo, A. 2019. “The Impact of Immigration on the Labor Market.” Journal of Economic Surveys 33 (3): 922–948. https://doi.org/10.1111/joes.2019.33.issue-3.
- Esses, V. M., L. M. Jackson, and T. L. Armstrong. 1998. “Intergroup Competition and Attitudes Toward Immigrants and Immigration: An Instrumental Model of Group Conflict.” Journal of Social Issues 54 (4): 699–724. https://doi.org/10.1111/josi.1998.54.issue-4.
- Facchini, G., and A. Maria Mayda. 2009. “Does the Welfare State Affect Individual Attitudes Toward Immigrants? Evidence Across Countries.” The Review of Economics and Statistics 91 (2): 295–314. https://doi.org/10.1162/rest.91.2.295.
- Facchini, G., and A. Maria Mayda. 2012. “Individual Attitudes Towards Skilled Migration: An Empirical Analysis Across Countries.” The World Economy 35 (2): 183–196. https://doi.org/10.1111/twec.2012.35.issue-2.
- Findor, A., M. Hruška, P. Jankovská, and M. Pobudová. 2022. “Who Should Be Given an Opportunity to Live in Slovakia? A Conjoint Experiment on Immigration Preferences.” Journal of Immigrant & Refugee Studies 20 (1): 79–93. https://doi.org/10.1080/15562948.2021.1902597.
- Fitzgerald, J., K. A. Curtis, and C. L. Corliss. 2012. “Anxious Publics: Worries About Crime and Immigration.” Comparative Political Studies 45 (4): 477–506. https://doi.org/10.1177/0010414011421768.
- Gidron, N., and P. A. Hall. 2017. “The Politics of Social Status: Economic and Cultural Roots of the Populist Right.” The British Journal of Sociology 68 (S1): S57–S84. https://doi.org/10.1111/bjos.2017.68.issue-S1.
- Goździak, E. M., and P. Márton. 2018. “Where the Wild Things Are: Fear of Islam and the Anti-Refugee Rhetoric in Hungary and in Poland.” Central and Eastern European Migration Review7:125–151.
- Hainmueller, J., and M. J. Hiscox. 2010. “Attitudes Toward Highly Skilled and Low-Skilled Immigration: Evidence from a Survey Experiment.” American Political Science Review 104 (1): 61–84. https://doi.org/10.1017/S0003055409990372.
- Hainmueller, J., M. J. Hiscox, and Y. Margalit. 2015. “Do Concerns About Labor Market Competition Shape Attitudes Toward Immigration? New Evidence.” Journal of International Economics 97 (1): 193–207. https://doi.org/10.1016/j.jinteco.2014.12.010.
- Hainmueller, J., and D. J. Hopkins. 2015. “The Hidden American Immigration Consensus: A Conjoint Analysis of Attitudes Toward Immigrants.” American Journal of Political Science 59 (3): 529–548. https://doi.org/10.1111/ajps.2015.59.issue-3.
- Hainmueller, J., D. J. Hopkins, and T. Yamamoto. 2014. “Causal Inference in Conjoint Analysis: Understanding Multidimensional Choices via Stated Preference Experiments.” Political Analysis 22 (1): 130–141. https://doi.org/10.1093/pan/mpt024.
- Hanson, G. H., K. Scheve, and M. J. Slaughter. 2007. “Public Finance and Individual Preferences Over Globalization Strategies.” Economics & Politics 19 (1): 1–33. https://doi.org/10.1111/ecpo.2007.19.issue-1.
- Helbling, M., and H. Kriesi. 2014. “Why Citizens Prefer High-Over Low-Skilled Immigrants. Labor Market Competition, Welfare State, and Deservingness.” European Sociological Review 30 (5): 595–614. https://doi.org/10.1093/esr/jcu061.
- Hellwig, T., and Y. Kweon. 2016. “Taking Cues on Multidimensional Issues: The Case of Attitudes Toward Immigration.” West European Politics 39 (4): 710–730. https://doi.org/10.1080/01402382.2015.1136491.
- Hero, R. E., and R. R. Preuhs. 2007. “Immigration and the Evolving American Welfare State: Examining Policies in the U.S. States.” American Journal of Political Science 51 (3): 498–517. https://doi.org/10.1111/ajps.2007.51.issue-3.
- Kovarek, D., and G. Dobos. 2023. “Masking the Strangulation of Opposition Parties as Pandemic Response: Austerity Measures Targeting the Local Level in Hungary.” Cambridge Journal of Regions, Economy and Society 16 (1): 105–117. https://doi.org/10.1093/cjres/rsac044.
- Kovarek, D., and L. Littvay. 2019. “Where Did All the Environmentalism Go? ‘Politics Can Be Different’ (LMP) in the 2018 Hungarian Parliamentary Elections.” Environmental Politics 28 (3): 574–582. https://doi.org/10.1080/09644016.2019.1567874.
- Kovarek, D., D. Róna, B. Hunyadi, and P. Krekó. 2017. “Scapegoat-Based Policy Making in Hungary: Qualitative Evidence for How Jobbik and Its Mayors Govern Municipalities.” Intersections. East European Journal of Society and Politics 3 (3): 63–87.
- Kovarek, D., and R. Sata. 2021. “By the Sweat of Your Parents' Brow: Self-Sufficiency of Young Adults and Intergenerational Transmission of Values and Resources in Hungary.” In Intergenerational Transmission and Economic Self-Sufficiency, edited by J. Tosun, D. Pauknerová, and B. Kittel, 209–235. Cham: Palgrave Macmillan.
- Kriesi, H., A. Altiparmakis, Á. Bojár, and I.-E. Oană. 2024. Coming to Terms with the European Refugee Crisis. Cambridge: Cambridge University Press.
- Kriesi, H., and A. Vrânceanu. 2023. “Voter Preferences for EU Asylum Policies: The Role of Government Cues.” Government and Opposition 1–23. https://doi.org/10.1017/gov.2023.41.
- Krzyzowski, L., and M. Nowicka. 2021. “European Solidarity As Boundary-Making: A Conjoint Analysis of Attitudes Towards Islam in the Context of the ‘Refugee Crisis’.” Journal of Sociology 57 (2): 305–324. https://doi.org/10.1177/1440783320902945.
- Kuziemko, I., R. W. Buell, T. Reich, and M. I. Norton. 2014. ““Last-Place Aversion”: Evidence and Redistributive Implications.” The Quarterly Journal of Economics 129 (1): 105–149. https://doi.org/10.1093/qje/qjt035.
- Lancaster, C. M. 2022. “Immigration and the Sociocultural Divide in Central and Eastern Europe: Stasis or Evolution?” European Journal of Political Research 61 (2): 544–565. https://doi.org/10.1111/ejpr.v61.2.
- Leeper, T. J. 2020. cregg: Simple Conjoint Analyses and Visualization. R package version 0.4.0.
- Leeper, T. J., S. B. Hobolt, and J. Tilley. 2020. “Measuring Subgroup Preferences in Conjoint Experiments.” Political Analysis 28 (2): 207–221. https://doi.org/10.1017/pan.2019.30.
- McFadden, D. 1999. “Rationality for Economists?” Journal of Risk and Uncertainty 19 (1–3): 73–105. https://doi.org/10.1023/A:1007863007855.
- Meuleman, B., E. Davidov, and J. Billiet. 2009. “Changing Attitudes Toward Immigration in Europe, 2002–2007: A Dynamic Group Conflict Theory Approach.” Social Science Research 38 (2): 352–365. https://doi.org/10.1016/j.ssresearch.2008.09.006.
- Moise, A. D., J. Dennison, and H. Kriesi. 2024. “European Attitudes to Refugees After the Russian Invasion of Ukraine.” West European Politics 47 (2): 356–381. https://doi.org/10.1080/01402382.2023.2229688.
- Natter, K., M. Czaika, and H. de Haas. 2020. “Political Party Ideology and Immigration Policy Reform: An Empirical Enquiry.” Political Research Exchange 2 (1): 1735255. https://doi.org/10.1080/2474736X.2020.1735255.
- Ortega, F., and J. Polavieja. 2012. “Labor-Market Exposure as a Determinant of Attitudes Toward Immigration.” Labour Economics 19 (3): 298–311. https://doi.org/10.1016/j.labeco.2012.02.004.
- Poutvaara, P., and M. F. Steinhardt. 2018. “Bitterness in Life and Attitudes Towards Immigration.” European Journal of Political Economy 55 (C): 471–490. https://doi.org/10.1016/j.ejpoleco.2018.04.007.
- Róna, D., E. Galgóczy, J. Pétervári, B. Szeitl, and M. Túry. 2020. “The Fidesz Party's Secret to Success: Investigating Economic Voting in Hungary.” https://21kutatokozpont.hu/wp-content/uploads/2020/08/Main_Secret_Fidesz_Success_final-2.pdf.
- Ruedin, D. 2020. “Do We Need Multiple Questions to Capture Feeling Threatened by Immigrants?” Political Research Exchange 2 (1): 1758576. https://doi.org/10.1080/2474736X.2020.1758576.
- Scheve, K. F., and M. J. Slaughter. 2001. “Labor Market Competition and Individual Preferences over Immigration Policy.” The Review of Economics and Statistics 83 (1): 133–145. https://doi.org/10.1162/003465301750160108.
- Simonovits, B 2020. “The Public Perception of the Migration Crisis from the Hungarian Point of View: Evidence from the Field.” In Geographies of Asylum in Europe and the Role of European Localities, edited by B. Glorius and J. Doomernik, 155–176. Cham: Springer International Publishing.
- Sniderman, P. M., L. Hagendoorn, and M. Prior. 2004. “Predisposing Factors and Situational Triggers: Exclusionary Reactions to Immigrant Minorities.” The American Political Science Review 98 (1): 35–49. https://doi.org/10.1017/S000305540400098X.
- Strnad, V. 2022. “Les Enfants Terribles De L'Europe? The ‘Sovereigntist’ Role of the Visegrád Group in the Context of the Migration Crisis.” Europe-Asia Studies 74 (1): 72–100. https://doi.org/10.1080/09668136.2021.1976730.
- Valentino, N. A., S. N. Soroka, S. Iyengar, T. Aalberg, R. Duch, M. Fraile, K. S. Hahn, et al. 2019. “Economic and Cultural Drivers of Immigrant Support Worldwide.” British Journal of Political Science49 (4): 1201–1226. https://doi.org/10.1017/S000712341700031X.
- Waters, M. C. 1994. “Ethnic and Racial Identities of Second-Generation Black Immigrants in New York City.” International Migration Review 28 (4): 795–820. https://doi.org/10.1177/019791839402800408.
- Wenzel, M., and M. Zerkowska-Balas. 2019. “Framing Effect of Media Portrayal of Migrants to the European Union: A Survey Experiment in Poland.” East European Politics and Societies 33 (1): 44–65. https://doi.org/10.1177/0888325418777058.
- Wike, R., and B. J. Grim. 2010. “Western Views Toward Muslims: Evidence from a 2006 Cross-National Survey.” International Journal of Public Opinion Research 22 (1): 4–25. https://doi.org/10.1093/ijpor/edq002.
- Wills, T. 1981. “Downward Comparison Principles in Social Psychology.” Psychological Bulletin 90 (2): 245–271. https://doi.org/10.1037/0033-2909.90.2.245.
- Zhou, R., and D. Soman. 2003. “Looking Back: Exploring the Psychology of Queuing and the Effect of the Number of People Behind.” Journal of Consumer Research 29 (4): 517–530. https://doi.org/10.1086/346247.