ABSTRACT
This study quantified the position-, duration-, and phase-of-play specific peak locomotor characteristics of senior professional rugby league match-play at a multi-club level. Match-play data were collected from 378 male professional rugby league players, from 11 clubs, across two competitive seasons. A total of 9643 match-observations were analysed; 10-Hz instantaneous velocity and acceleration from Catapult S5 microtechnology units were aligned with video footage to determine to the phase-of-play and duration-specific peak locomotor characteristics (average running speed, relative high-speed running [HSR;>5.5 m·s−1], average absolute acceleration). Linear mixed effect models were used to determine positional differences for each dependent variable and differences between phases-of-play. Positional differences for the duration-specific and phase-of-play peak locomotive characteristics were identified. Fullbacks had greater peak HSR during defensive sets (86 ± 70 m·min−1) vs. all other positions (effect size = 0.26 to 0.49, small). Wingers demonstrated the greatest between phase differences with greater peak locomotor characteristics (effect size = 1.23 to 1.65, large) during attacking-defensive set transition vs. defensive sets. The multi-club normative data, and the differences identified, provides practitioners with valuable information for the consideration of training practices; the incorporation of phases-of-play enables greater consideration of technical-tactical factors whilst preparing players for the peak periods of competition.
1. Introduction
Understanding the physical match characteristics of rugby league is of importance given practitioners can use these data to prescribe appropriate exercise intensities via a range of training modalities, such as contact, technical-tactical training and conditioning (Weaving et al., Citation2017a). The appropriate manipulation of external and internal training load is required to elicit a positive training response (Impellizzeri et al., Citation2005; Vanrenterghem et al., Citation2017), ensuring players are adequately prepared for a match. Recently, research has moved beyond “whole-match” characteristics, and focused on the peak physical characteristics of match-play, to ensure technical-tactical training is performed at appropriate intensities, depending on the duration of the drill (Corbett et al., Citation2019; Whitehead et al., Citation2018a). This theoretically provides a physical and, technical and tactical challenge for players in a rugby-specific context (Rennie et al., Citation2019a; Weaving et al., Citation2018; Whitehead et al., Citation2020).
Research quantifying the peak match-play characteristics across the football codes has increased substantially in recent years (Whitehead et al., Citation2018a). In the United Kingdom (UK) the peak characteristics of rugby league match-play has been quantified across the professional (Weaving et al., Citation2018; Whitehead et al., Citation2020, Citation2019a, Citation2018b) and international (Rennie et al., Citation2019; Whitehead et al., Citation2018b) levels. Research at the academy levels have included multiple teams (Whitehead et al., Citation2019a), but at the senior professional level (i.e. Super League [SL]) data exist from only one team during one season (Johnston et al., Citation2019; Weaving et al., Citation2018). The peak 5- and 10-minute average speeds range from ~110 to 119 and ~96 to 103 m∙min−1, respectively, across all positions (Weaving et al., Citation2018). These values are useful for practitioners when comparing either match or training data, and can be used when monitoring technical-tactical training demands in real-time (Weaving et al., Citation2017b).
The practical application of the duration-specific peak characteristics data in isolation has been questioned (Carling et al., Citation2019), highlighting the importance of understanding the tactical requirements alongside the physical data (Carling et al., Citation2019; Malone et al., Citation2020; Whitehead et al., Citation2020, Citation2019b). A recent study in senior international rugby league quantified the physical characteristics of phases of play (i.e. attack, defence, attack-defence transition and ball in/out of play), identifying distinct locomotor characteristics during attacking and defending phases (Rennie et al., Citation2019). Forwards and backs had greater average running speeds during defence but greater high speed distance per minute during attack (Rennie et al., Citation2019). However, only whole-match averages for the phases are identified, and the peak characteristics that are associated within the specific phases of play (e.g. the highest distance covered during an attacking set) are yet to be investigated. Such research, alongside the duration-specific peak locomotor characteristics from the whole-match would provide practitioners with reference data to aid in the prescription of a range of technical-tactical training drills and evaluation of the intensity of training compared to match-play.
A common limitation exists amongst the current research in senior professional rugby league competition, in that studies typically use only one club (Delaney et al., Citation2016, Citation2015; Weaving et al., Citation2018). Whilst Dalton-Barron et al. (Citation2020) found little between-team variability for the locomotor characteristics of the whole-match and average phase of play, single club studies potentially limits the generalisability of the peak characteristics data given the unique tactics of individual clubs as well as individual player physical characteristics which have been found to impact upon the peak locomotor characteristics (Duthie et al., Citation2017). Additionally, the peak characteristics have been found to differentiate between positions (Delaney et al., Citation2016, Citation2015; Weaving et al., Citation2018; Whitehead et al., Citation2019a), however, investigation of each individual position (i.e., back row, second row, prop forward, hooker, half back, centre, winger and fullback) is rarely carried out due to the large sample size needed. A peak characteristics multi-club study has been carried on in the Academy SL (Whitehead et al., Citation2019a), however, this is limited to only one match observation from some clubs. A multi-club microtechnology project currently exists within SL (Project SL-Catapult), whereby all players wear the same type of microtechnology device, which is shared centrally. To date, the match-to-match variability has been quantified of this dataset (Dalton-Barron et al., Citation2020), but the peak characteristics of match-play have not been quantified. Quantification of the peak locomotor characteristics of SL match-play on a league wide basis can provide reference data for all stakeholders involved in rugby league. Therefore, the aims of this study were to quantify the peak locomotor characteristics of senior professional rugby league match-play for specific durations, by position, and by phase-of-play.
2. Methods
2.1. Experimental approach
A longitudinal observational design was used to compare positional duration-specific and phase-of-play (i.e. attack, defence, attack-defence transition and ball-in-play) peak locomotive characteristics from two SL seasons. Microtechnology data were collected from 11 teams participating in the SL competition in the 2018 and 2019 seasons, which were aligned with match-play event data to describe the peak locomotive characteristics of specific phases of play.
2.2. Subjects
Data were analysed for 378 male professional rugby league players, whom were members of a first-team squad competing in the SL competition, providing 9643 match observations which were reduced to 6657 following data pre-processing (detailed below). Participants were stratified according to their primary playing position for each match: back row (n [number of match observations] = 635), centre (n = 869), fullback (n = 438), halves (n = 891), hooker (n = 529), prop forward (n = 1443), second row (n = 995), wing (n = 855). Microtechnology data previously used by Dalton-Barron et al. (Citation2020) (i.e., Project SL-Catapult) were used in this study. However, this study dropped observations with any missing data across any of the phases and peak data, which is why the number of observations are slightly lower in this study compared to Dalton-Barron et al. (Citation2020). As part of the project, clubs provided consent for the data to be used by Super League, Catapult and other authorised users, including research and commercial projects. Since no personal data were accessible by the research team, and only summary statistics are presented, written informed consent was not needed by each participant, thereby conforming with the United Kingdom Data Protection Act, 2018. Ethics approval for the study was granted by Leeds Beckett University Ethics Committee; number 48838.
2.3. Methodology
Players were fitted with microtechnology units (Optimeye S5, Catapult Sports, Melbourne, Victoria), housing a 10-Hz Global Navigation Satellite Systems (GNSS) antenna which were used to quantify the peak locomotive characteristics of match-play. The GNSS provides provides geospatial positioning with global coverage encompassing both Global Positioning System (GPS) and Global Navigation Satellite System (GLONASS) constellations (Malone et al., Citation2017). The validity and reliability of the microtechnology devices for measuring instantaneous velocity have been established (Varley et al., Citation2012b). Participating teams obtained the microtechnology devices in the pre-season of the 2018 season; players wore the devices during training sessions thus were familiarised prior to match-play. Performance staff were educated as to best practice data collection processes (e.g., obtaining satellite lock).
Microtechnology data were initially downloaded by the club users using proprietary OpenFieldTM software (Catapult Sports, Melbourne, Australia). Once the data are downloaded, the OpenfieldTM software automatically applies a number of smoothing algorithms to the raw data which are undisclosed. Clubs then uploaded the data through OpenfieldTM software before being uploaded and stored securely on the cloud. Subsequent querying of the cloud database through an application program interface (API) provided access to microtechnology data in the form of 10 Hz instantaneous speed and acceleration sampling points. These data points were processed for calculation of locomotor variables. Following the calculation of locomotor variables, a synchronisation-point of the precise moment of kick-off was determined through visual inspection of latitude and longitude of each match alongside video footage. The Opta (Leeds, UK) coded match-play phases of play (i.e., attacking sets, defensive sets, attacking-defensive set transition, ball-in-play) were subsequently time synchronised with microtechnology data allowing for further analysis to take place. Good inter-operator reliability have for Opta statistics have been reported in team sports (Liu et al., Citation2013).
Microtechnology inclusion criteria (e.g., number of connected satellites ≥ 10, horizontal dilution of precision [HDOP] ≤ 1, velocity ≤ 10 m∙s−1, acceleration ≤ ± 6 m∙s−2) were applied to each sampling point within the speed and acceleration vectors in order to remove erroneous data (Malone et al., Citation2017; Weston et al., Citation2015). If the duration of consecutive missing sampling points was <10 s then missing speed and acceleration data were imputed via linear interpolation (Rennie et al., Citation2019). Instances where microtechnology signal quality was deemed poor during the data collection phase (i.e. more than 10% of the raw file was filtered; observations = 1588) or match time considered low (i.e. less than 20 minutes on field duration; observations = 326) resulted in the entire observation being removed. The mean number of connected satellites and mean HDOP during the data collection period were 11.5 ± 0.4 and 0.8 ± 0.2, respectively.
The peak locomotive characteristics, i.e., highest total distance covered, high-speed running (HSR; >5.5 m∙s−1) and average absolute acceleration/deceleration (Acc) (Delaney et al., Citation2017a), and duration (s) were determined for each phase of play (attacking sets, defensive sets, attacking-defensive set transition, ball-in-play). The duration-specific peak (1- to 10-minutes) locomotive characteristics were determined via the moving averages method (Varley et al., Citation2012a; Whitehead et al., Citation2018a) and were subsequently used to determine the power law relationship (Delaney et al., Citation2017b) between the moving averages durations and each peak locomotive variable (average running speed, relative HSR and Acc).
2.4. Statistical analysis
All statistical analyses were performed using R statistical software (R. 3.6.3, R Foundation for Statistical Computing, Vienna, Austria). Data were log-transformed prior to analysis to reduce bias and non-uniform error, and subsequently back-transformed post-analysis (Hopkins et al., Citation2009). Descriptive statistics are presented as mean ± standard deviation (SD). Linear mixed-effects models were carried out using the lmerTest package in R (Kuznetsova et al., Citation2017). Individual athletes and match identification were included as nested random effects to account for repeated measures and variability between matches. Positions and phase of play were included as fixed effects to describe their relationship with the dependent variable (i.e., peak locomotive characteristics, power law relationship derived intercepts and slopes). For duration as the dependent variable, phase of play was the only fixed effect. Pairwise comparisons between positions and phases of play were assessed using the Least Squares mean test. The magnitude and the direction of the differences were then determined via effect sizes (ES, i.e., standardised mean difference; 95% confidence intervals), standardised to the between-player, between-match and the residual, and classified as follows: trivial (<0.2), small (0.2 to 0.59), moderate (0.60 to 1.19), large (1.2 to 1.99), very large (2.0 to 4.0) (Hopkins et al., Citation2009). The p-value is set at 0.05, but given the sample size, and need for practical interpretation of data, differences will be discussed based on the ES (Bakker et al., Citation2019; Palesch, Citation2014).
3. Results
The intercept and slope for speed, Acc and HSR for each position are shown in . These values can be used to estimate the peak average speed, Acc and HSR for any duration using Equation 1:
Table 1. Intercept and slope values for estimating the peak average speed, average absolute acceleration/deceleration (Acc) and high-speed running (HSR) for each position, via the equation: peak locomotive characteristic = intercept x timeslope.
intensity = intercept x timeslope
For example, the 10-minute (600-second) peak average speed for a Winger could be estimated as: intensity = 461 x 600-0.25 = 93 m·min−1
The position specific peak locomotive characteristics of the different phases of play are shown in . The whole squad differences in the peak locomotive characteristics between the phases of play are shown in . As demonstrated in the figure very large differences were found between the duration of attack-defence transition and all other phases of play, with the peak average-speed, high-speed running and average absolute acceleration all being greatest in the attack-defence transition phase. For peak average running speed () there was a large difference between transition and attack (289 vs. 164 m·min−1, respectively), moderate differences between transition and defence (289 vs. 187 m·min−1, respectively) and attack and BIP (164 vs. 215 m·min−1, respectively), and small differences between defence and attack (187 vs. 164 m·min−1, respectively) and transition and BIP (289 vs. 215 m·min−1, respectively).
Table 2. The position specific peak locomotive characteristics for attack, defence, attack-defence set transition and ball-in-play phases of play
Figure 1. Pairwise comparisons by phases of play for the whole squad peak A; average-speed (m·min−1), B; high-speed running (m·min−1), C; average absolute acceleration (m·s−2) and D; duration (s)
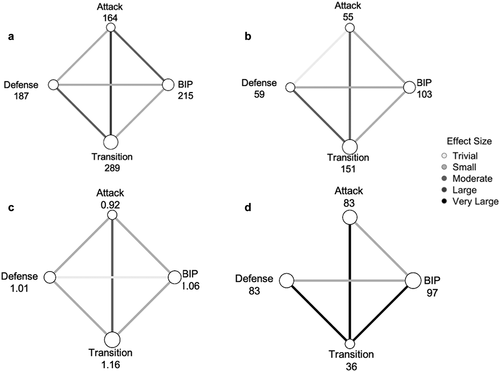
The ES (95% CI) and p-values for each pairwise comparison for the position-specific differences and whole squad phase-of-play differences are shown in Supplementary respectively.
4. Discussion
This study uses the current largest dataset of senior professional rugby league match-play, to quantify the peak phase-of-play and duration-specific locomotive characteristics, consequently providing reference data for practitioners. Positional differences for both the duration-specific and phase-of-play peak locomotive characteristics have been identified, in agreement with previous research on the duration-specific characteristics of match-play (Delaney et al., Citation2016; Whitehead et al., Citation2019a). Furthermore, position-specific differences in the peak phase-of-play locomotive characteristics were identified with the largest difference observed between defensive sets and attacking-defensive transitions for wingers. Such data, and the differences identified, provides practitioners with valuable information when planning and evaluating training practices. Specifically, the incorporation of phases-of-play peak locomotive characteristics enables greater consideration during technical-tactical drills, preparing players for the peak periods of competition.
The duration-specific peak locomotive characteristics of rugby league match play have now been explored widely, with multiple studies investigating senior professional teams (Delaney et al., Citation2015, Citation2015; Duthie et al., Citation2017; Johnston et al., Citation2019; Weaving et al., Citation2018). However, this is the first study to carry out a multi-club analysis of the peak characteristics, from two competitive seasons, resulting in 6657 match observations. The power law relationship derived intercept and slope values are a useful tool for practitioners to estimate the peak “intensity” for a specified duration, permitting practitioners to prescribe and periodise effectively (Jace A. Delaney et al., Citation2017b). Using the intercept and slope values quantified () (Jace A. Delaney et al., Citation2017b) it is evident that the range of values for the peak 10-minute average running speeds (93 to 108 m·min−1) appear similar to those previously reported for senior professional match play (96 to 102 m·min−1 in one SL club [Weaving et al., Citation2018], 98 to 102 m·min−1 in one NRL club [Delaney et al., Citation2016]), and in a multi-club Academy level study (94 to 106 m·min−1)(Whitehead et al., Citation2019b).
It does appear that the peak Acc values identified in this study are lower than previously reported across all positions. For example, the power law relationship derived peak 1-minute Acc for hookers in the current study is ~0.88 m·s−2, compared to ~1.28 m·s−2 (Delaney et al., Citation2016) and ~1.25 m·s−2 (Duthie et al., Citation2017) previously reported in the NRL. This could be due to the use of different filtering algorithms, exclusion criteria and/or hardware (Catapult S5 vs. GPSport HPU microtechnology units), as well as differences in game-play characteristics between SL and NRL (Johnston et al., Citation2019; Woods et al., Citation2018). Similarly, higher duration-specific peak HSR has been identified in the current study in comparison to international match-play (Rennie et al., Citation2019). This could again be due to the different competitions, but given the high variability in HSR between matches (Dalton-Barron et al., Citation2020; Kempton et al., Citation2014), and the limited number of matches investigated by Rennie et al. (Citation2019), further research at the international level including multiple teams is required to quantify the true demands of this level. The positional differences in intercept and slope, and the derived values, are in agreement with previous research (Duthie et al., Citation2017; Whitehead et al., Citation2019a). For example, in the current study, wingers have the greatest speed slope, with small differences between all positions, likely due to the physical qualities of the athletes (i.e., speed and strength) as well as tactical requirements (Duthie et al., Citation2017). This indicates the need for practitioners to expose Wingers to the shorter higher peak HSR periods of match-play within technical-tactical training, for example, maintaining HSR (>5.5 m∙s−1) at 71 m·min−1 for 1-minute ().
The differences between the peak phase-of-play locomotive characteristics are variable and position dependent, demonstrating the need to plan and monitor specific drills effectively to ensure exposure to the appropriate match-intensities of those phases-of-play, for specific positions. Attacking sets exhibit lower Acc compared to the other phases of play (i.e., defensive sets, transition and ball-in-play) (). This may be due to players having to react less to situations during attack compared to other phases of play, as the team have possession of the ball, however this is speculative and requires further research. Similarly, peak attacking average running speed was lower (small) compared to the other phases of play for all positions except for wingers and fullbacks who demonstrate trivial differences compared to peak defensive set speed. This is the first study to investigate the peak locomotive characteristics during these phases of play, yet the apparent differences are in agreement with the current research that has described the average locomotive characteristics of attacking and defensive sets (Gabbett et al., Citation2014; Rennie et al., Citation2019). Rennie et al. (Citation2019) found lower average running speeds for forwards and backs (ES = −1.17 to −2.31) during attacking sets (vs. defensive sets) which is in agreement with the current study. But, conflicting findings in the differences in HSR between attacking and defensive sets is present; in the current study trivial differences were found in HSR for most positions, whereas Rennie et al. (Citation2019) found high HSR during attacking sets vs. defensive, especially for backs (ES = 4.41 [3.37 to 5.45]). Peak speed and HSR during transition sets were higher (small to large differences) than all other phases of play (except trivial differences for props vs. BIP). This is not surprising given the longest duration for these attacking-defensive transition sets is shorter than all other phases of play (ES = 2.17 to 2.53; ).
Within the peak phases-of-play positional differences in the locomotive characteristics have been identified, with some unique differences dependent upon the phase-of-play (). For example, during defensive sets, trivial differences were present between most positions, except for fullbacks who had greater peak HSR (small) compared to all other positions, likely due to their unique tactical requirements (e.g. fullbacks not in the defensive line). Yet, during attacking-defensive set transitions the peak locomotive characteristics for “backs” are greater (small to moderate) compared to all “forward” positions. These differences can be explained by “backs” leading the kick chase or challenging for the ball. Such findings demonstrate the need to consider the phase-of-play peak characteristics when prescribing training for different positions. For example, during defensive drills Fullbacks should be exposed to average running speeds of up to 245 ± 75 m·min−1 for 100 ± 13 s, alongside any concurrent collisions that may occur (Weaving et al., Citation2018), to ensure they are prepared for the peak periods during BIP.
The league wide peak characteristics data identified in the current study provides practitioners and coaches with important information for the prescription of training practices, for the first time considering the peak phase-of-play characteristics. However, it is not without its limitations. Firstly, the study only included locomotive characteristics, further research is required to determine the collision frequency and also the concurrent technical and tactical requirements on a league wide scale, as well as the concurrent physical characteristics of the peak periods (Weaving et al., Citation2018). Additionally, whilst inter-operator reliability of Opta data is available in soccer (Liu et al., Citation2013) the intra- and inter-reliability of Opta data in rugby league is currently unknown, thus further research is required to ensure confidence in these data. Furthermore, the data provided in this study provide practitioners with both the longest duration and the peak locomotive characteristics of each phase which may not happen at the same time. Further research is required to provide a comprehensive analysis of how these peak phases-of-play interact and any effect on technical-tactical characteristics surrounding these peak periods.
In conclusion, this is the first study to quantify the league wide peak phase-of-play and duration-specific locomotive characteristics of senior professional rugby league match-play, across two competitive seasons. The position specific intercepts and slopes identified can be used by practitioners to derive normative data for the duration-specific peak running speeds for the SL competition, to aid in the prescription and monitoring of technical-tactical training. The peak phase-of-play characteristics quantified can be used to build upon this and provide more context to the prescription of training practices, through the consideration of the differences in between the phases-of-play identified as well as the positional differences
Conflict of Interest
SW has no conflicts of interest. NDB receives research and consultancy funding from the RFL, and his PhD was sponsored by Catapult Sports. GR is employed by Catapult Sports. BJ is employed by the RFL in a consultancy capacity, was involved in the conceptualisation of the league-wide GPS agreement between Rugby Football League, Super League and Catapult Sports, and has also received research funding from Catapult Sports. The authors do not see this as a conflict of interest for this study.
Supplemental Material
Download MS Word (14 KB)Supplemental Material
Download MS Word (14 KB)Supplemental Material
Supplemental data for this article can be accessed here
References
- Bakker, A., Cai, J., English, L., Kaiser, G., Mesa, V., & Van Dooren, W. (2019). Beyond small, medium, or large: Points of consideration when interpreting effect sizes. Educational Studies in Mathematics, 102(1), 1–8. https://doi.org/https://doi.org/10.1007/s10649-019-09908-4
- Carling, C., McCall, A., Harper, D., & Bradley, P. S. (2019). Comment on: “The use of microtechnology to quantify the peak match demands of the football codes: A systematic review”. Sports Medicine, 49(2), 343–345. https://doi.org/https://doi.org/10.1007/s40279-018-1032-z
- Corbett, D. M., Sweeting, A. J., & Robertson, S. (2019). A change point approach to analysing the match activity profiles of team-sport athletes. Journal of Sports Sciences, 37(14), 1600–1608. https://doi.org/https://doi.org/10.1080/02640414.2019.1577941
- Dalton-Barron, N., Palczewska, A., McLaren, S. J., Beggs, C., Roe, G., & Jones, B. (2020). A league-wide investigation into variability of rugby league match running from 322 Super League games. Science and Medicine in Football, 5(3), 225-233. . https://doi.org/https://doi.org/10.1080/24733938.2020.1844907
- Delaney, J. A., Cummins, C. J., Thornton, H. R., & Duthie, G. M. (2017a). Importance, reliability and usefulness of acceleration measures in team sports. The Journal of Strength and Conditioning Research, 32(12), 3485–3493. https://doi.org/https://doi.org/10.1519/JSC.0000000000001849
- Delaney, J. A., Duthie, G. M., Thornton, H. R., Scott, T. J., Gay, D., & Dascombe, B. J. (2016). Acceleration-based running intensities of professional rugby league match play. International Journal of Sports Physiology and Performance, 11(6), 802–809. https://doi.org/https://doi.org/10.1123/ijspp.2015-0424
- Delaney, J. A., Scott, T. J., Thornton, H. R., Bennett, K. J., Gay, D., Duthie, G. M., & Dascombe, B. J. (2015). Establishing duration-specific running intensities from match-play analysis in rugby league. International Journal of Sports Physiology and Performance, 10(6), 725–731. https://doi.org/https://doi.org/10.1123/ijspp.2015-0092
- Delaney, J. A., Thornton, H. R., Rowell, A. E., Dascombe, B. J., Aughey, R. J., & Duthie, G. M. (2017b). Modelling the decrement in running intensity within professional soccer players. Science and Medicine in Football, 2(2), 86–92. https://doi.org/https://doi.org/10.1080/24733938.2017.1383623
- Duthie, G. M., Thornton, H. R., Delaney, J. A., McMahon, J. T., & Benton, D. T. (2017). Relationship between physical performance testing results and peak running intensity during professional rugby league match play. The Journal of Strength and Conditioning Research, 34(12), 3506–3513. https://doi.org/https://doi.org/10.1519/JSC.0000000000002273
- Gabbett, T. J., Polley, C., Dwyer, D. B., Kearney, S., & Corvo, A. (2014). Influence of field position and phase of play on the physical demands of match-play in professional rugby league forwards. Journal of Science and Medicine in Sport, 17(5), 556–561. https://doi.org/https://doi.org/10.1016/j.jsams.2013.08.002
- Hopkins, W. G., Marshall, S. W., Batterham, A. M., & Hanin, J. (2009). Progressive statistics for studies in sports medicine and exercise science. Medicine and Science in Sports and Exercise, 41(1), 3–13. https://doi.org/https://doi.org/10.1249/MSS.0b013e31818cb278
- Impellizzeri, F. M., Rampinini, E., & Marcora, S. M. (2005). Physiological assessment of aerobic training in soccer. Journal of Sports Sciences, 23(6), 583–592. https://doi.org/https://doi.org/10.1080/02640410400021278
- Johnston, R. D., Weaving, D., Hulin, B. T., Till, K., Jones, B., & Duthie, G. (2019). Peak movement and collision demands of professional rugby league competition. Journal of Sports Sciences, 37(18), 2144–2151. https://doi.org/https://doi.org/10.1080/02640414.2019.1622882
- Kempton, T., Sirotic, A. C., & Coutts, A. J. (2014). Between match variation in professional rugby league competition. Journal of Science and Medicine in Sport, 17(4), 404–407. https://doi.org/https://doi.org/10.1016/j.jsams.2013.05.006
- Kuznetsova, A., Brockhoff, P. B., & Christensen, R. H. B. (2017). lmerTest package: Tests in linear mixed effects models. Journal of Statistical Software, 82(13), 13. https://doi.org/https://doi.org/10.18637/jss.v082.i13
- Liu, H., Hopkins, W., Gomez, A. M., & Molinuevo, S. J. (2013). Inter-operator reliability of live football match statistics from OPTA Sportsdata. International Journal of Performance Analysis in Sport, 13(3), 803–821. https://doi.org/https://doi.org/10.1080/24748668.2013.11868690
- Malone, J. J., Barrett, S., Barnes, C., Twist, C., & Drust, B. (2020). To infinity and beyond: The use of GPS devices within the football codes. Science and Medicine in Football, 4(1), 82–84. https://doi.org/https://doi.org/10.1080/24733938.2019.1679871
- Malone, J. J., Lovell, R., Varley, M. C., & Coutts, A. J. (2017). Unpacking the black box: Applications and considerations for using GPS devices in sport. International Journal of Sports Physiology and Performance, 12(Suppl 2), S218–s226. https://doi.org/https://doi.org/10.1123/ijspp.2016-0236
- Palesch, Y. Y. (2014). Some common misperceptions about P values. Stroke, 45(12), e244–e246. https://doi.org/https://doi.org/10.1161/STROKEAHA.114.006138
- Rennie, G., Dalton-Barron, N., McLaren, S. J., Weaving, D., Hunwicks, R., Barnes, C., Emmonds, S., Frost, B., & Jones, B. (2019). Locomotor and collision characteristics by phases of play during the 2017 rugby league world cup. Science and Medicine in Football, 4(3), 225-232. https://doi.org/https://doi.org/10.1080/24733938.2019.1694167
- Vanrenterghem, J., Nedergaard, N. J., Robinson, M. A., & Drust, B. (2017). Training load monitoring in team sports: A novel framework separating physiological and biomechanical load-adaptation pathways. Sports Medicine, 47(11), 2135–2142. https://doi.org/https://doi.org/10.1007/s40279-017-0714-2
- Varley, M. C., Elias, G. P., & Aughey, R. J. (2012a). Current match-analysis techniques’ underestimation of intense periods of high-velocity running. International Journal of Sports Physiology and Performance, 7(2), 183–185. https://doi.org/https://doi.org/10.1123/ijspp.7.2.183
- Varley, M. C., Fairweather, I. H., & Aughey, R. J. (2012b). Validity and reliability of GPS for measuring instantaneous velocity during acceleration, deceleration, and constant motion. Journal of Sports Sciences, 30(2), 121–127. https://doi.org/https://doi.org/10.1080/02640414.2011.627941
- Weaving, D., Jones, B., Marshall, P., Till, K., & Abt, G. (2017a). Multiple measures are needed to quantify training loads in professional rugby league. International Journal of Sports Medicine, 38(10), 735–740. https://doi.org/https://doi.org/10.1055/s-0043-114007
- Weaving, D., Sawczuk, T., Williams, S., Scott, T., Till, K., Beggs, C., Johnston, R., & Jones, B. (2018). The peak duration-specific locomotor demands and concurrent collision frequencies of European Super League rugby. Journal of Sports Sciences, 37(3), 322–330. https://doi.org/https://doi.org/10.1080/02640414.2018.1500425
- Weaving, D., Whitehead, S., Till, K., & Jones, B. (2017b). Validity of real-time data generated by a wearable microtechnology device. The Journal of Strength & Conditioning Research, 31(10), 2876–2879. https://doi.org/https://doi.org/10.1519/JSC.0000000000002127
- Weston, M., Siegler, J., Bahnert, A., McBrien, J., & Lovell, R. (2015). The application of differential ratings of perceived exertion to Australian Football League matches. Journal of Science and Medicine in Sport, 18(6), 704–708. https://doi.org/https://doi.org/10.1016/j.jsams.2014.09.001
- Whitehead, S., Till, K., Jones, B., Beggs, C., Dalton-Barron, N., & Weaving, D. (2020). The use of technical-tactical and physical performance indicators to classify between levels of match-play in elite rugby league, 5(2), 121-127. Science and Medicine in Football. https://doi.org/https://doi.org/10.1080/24733938.2020.1814492
- Whitehead, S., Till, K., Weaving, D., & Jones, B. (2018a). The use of micro-technology to quantify the peak match-demands of the football codes: A systematic review. Sports Medicine, 48(11), 2549–2575. https://doi.org/https://doi.org/10.1007/s40279-018-0965-6
- Whitehead, S., Till, K., Weaving, D., Dalton-Barron, N., Ireton, M., & Jones, B. (2019a). The duration-specific peak average running speeds of European Super League Academy rugby league match-play. The Journal of Strength and Conditioning Research, 35(7), 1964-1971. https://doi.org/https://doi.org/10.1519/JSC.0000000000003016
- Whitehead, S., Till, K., Weaving, D., Hunwicks, R., Pacey, R., & Jones, B. (2018b). Whole, half and peak running demands during club and international youth rugby league match-play. Science and Medicine in Football, 3(1), 63–69. https://doi.org/https://doi.org/10.1080/24733938.2018.1480058
- Whitehead, S., Till, K., Weaving, D., & Jones, B. (2019b). Authors’ reply to carling et al: Comment on: “The use of microtechnology to quantify the peak match demands of the football codes: A systematic review”. Sports Medicine, 49(2), 347–348. https://doi.org/https://doi.org/10.1007/s40279-018-1028-8
- Woods, C. T., Leicht, A. S., Jones, B., & Till, K. (2018). Game-play characteristics differ between the European Super League and the national rugby league: Implications for coaching and talent recruitment. International Journal of Sports Science & Coaching, 13(6), 1171–1176. https://doi.org/https://doi.org/10.1177/1747954118788449