Abstract
Air pollution is a major environmental problem in China. Especially, the Beijing–Tianjin–Hebei (BTH) region experienced most serious airborne pollution. In the paper, the spatial data statistical model was employed to analysis the spatiotemporal variability of PM2.5 concentration and spatial agglomeration law within the BTH region. Thus, it can provide considerable benefits when developing environmental policies. Our results indicate that PM2.5 annual average concentrations decreased annually from 2014 to 2016, the days of reaching the standards increased annually and the days of serious pollution decreased annually; the concentration of PM2.5 in BTH region showed significant spatial differentiation, the overall performance from north-west to south-east gradually increased, north and south very different spatial pattern; showing a significant assemble law, hot spots are mainly concentrated in the vast majority of Baoding, Shijiazhuang, Hengshui, Xingtai,Handan and a few of Changzhou county-level cities. Cold spots are mainly concentrated in the part of the county city of Zhangjiakou, Qinhuangdao, Chengde, and Beijing. Compared with 2014, the high concentration of county-level cities in 2016 was slightly narrowed, and the level of agglomeration was reduced.
1. Introduction
China has experienced industrialization and a surge in car usage and urbanization, and these changes have generated severe amounts of particulate matter pollution [1], have brought huge energy consumption as well as a large number of air pollutants emissions. In China, particulate contamination is a serious environmental problem that affects air quality, regional and global climate, and human health (Han, Zhou, & Li, Citation2016; Liao, Shan, & Jie, Citation2017; Xu, Ho, & Cao, Citation2017). Which BTH region is China’s PM2.5 pollution of the heavy disaster area, it formed a large area of continuous PM2.5 serious pollution area. According to the Ministry of Environmental Protection, 2014–2016 national air quality of the worst 10 cities, the BTH region in the past three years accounted for 6–8.
At present, most studies focused on the cause of the pollution of PM2.5, source, and spatiotemporal distribution and the simulation of regional single pollution processes (Chen, Li, & Ge, Citation2015a; Liao et al., Citation2017; Luo, Du, & Samat, Citation2017). Constrained by PM2.5 monitoring data, the existing research in China has analysed the Spatiotemporal distribution pattern of PM2.5 in the national space range from the year, quarter, and month scale the temporal pattern of PM2.5 and the spatial agglomeration characteristics in the Short – term or a short time analysis of a city or region (Chen, Cai, & Gao, Citation2017; Hua, Cheng, & Wang, Citation2015; Li, Ren, & Yu, Citation2016; Li, Zhang, & Zhang, Citation2015a; Wang, Ying, & Hu, Citation2014; Wang et al., Citation2015; Zhang, Wang, & Zhang, Citation2016). There has been a lack of research on the spatiotemporal pattern of PM2.5 concentrations in BTH region using long time series data of ground monitoring. Therefore, our study is to analyse the spatiotemporal pattern of PM2.5 concentrations in BTH region and the spatial clustering characteristics of PM2.5 pollution in county-level cities in recent four years.
The study is beneficial to enhance the cognition of spatial distribution and dynamic change of fine particulate pollutants in this area, and can provide scientific reference for relevant research and policy formulation.
2. Materials and methods
2.1. Research areas and data sources
This study area is located in the BTH region, covers the capital of China (Beijing), Tianjin, and Hebei province as shown in Figure . It is the country’s most important administrative, industrial, and commercial center, but, the most serious PM2.5 pollution in China. The data presented in this study were obtained from the China National Environmental Monitoring Center. The hourly PM2.5 concentrations from 80 monitoring stations in the 13 cities of the BTH region during 12 April 2013 to 12 April 2016 are collected (http://www.pm25.in/). We deleted the values <0 and the abnormal records in the raw data according to the requirements for the validity of the PM2.5 concentration released in GB3095-2012.
Figure 1. The study area and the spatial distribution of the ground stations in the BTH region (The map was Created by ArcMap, version 10.2, PM2.5 stations were collected from the website http://www.pm25.in/).
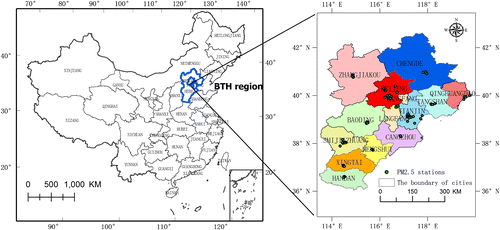
PM2.5 statistics are hourly data, 24 h a day (00: 00-23: 00). “Daily average” refers to an arithmetic average of an average daily average of 24 h, “Monthly mean” refers to the arithmetic mean of the average daily concentration over a calendar month, “Seasonal mean” refers to the arithmetic mean of the average daily concentration in a calendar season, “Average year” means a calendar year each day on average concentration of arithmetic mean. Seasonal division is: March–May for the spring, 6–8 months for the summer, 9–11 months for the fall, 12, 1, February for the winter. In 2013, data were limited to summer, autumn, and April–December.
2.2. Research methods
Some researchers compared the spatial interpolation methods to determine whether they are suitable for reflecting the spatial distribution pattern of PM2.5 and they proved that Ordinary Kriging interpolation method is widely applicable, and it is widely used in the space of the Bureau space, and it can get better precision results in a small amount of uneven distribution data (Tan & Xu, Citation2014; Zhang, Qi, & Jiang, Citation2013). The cubic spline function method is suitable for interpolating contours with larger densities. It has a good interpolating effect on the data of the whole and the larger number of points, and it can get better in a large number of mean distribution data Precision value. The inverse distance weighing (dew) method applies to the overall sample point where the density is relatively large and the distribution of the sample points is relatively uniform. According to the characteristics of existing data, in this paper, Ordinary Kriging interpolation method (Ordinary Kriging Method, OKM) is used to interpolate space to study the spatial distribution and variation characteristics of PM2.5 in BTH region.
2.2.1. Spatial autocorrelation analysis
Spatial autocorrelation analysis describes the potential interdependence of some variables in adjacent spatial units, and explores the spatial agglomeration patterns of factors and finds potential trends which has been widely used in environmental pollution in recent years (Liu, Bi, & Wang, Citation2013). As the diffusion of atmospheric motion and matter, the spatial correlation of PM2.5 concentration in the same area is more significant. In the field of PM2.5 pollution research, this model has been successfully used to demonstrate the PM2.5 spatial pattern of the national scale and the Yangtze River (Wang et al., Citation2014; Wang et al., Citation2015).
The model is composed of global spatial autocorrelation and local spatial autocorrelation Part of the composition.
The global spatial autocorrelation describes the spatial dependence of PM2.5 in the whole area of the region. The global Moran’s I index is used to study the global formula. The formula is as follows:(1)
where ;
; xi, yi, respectively, is the PM2.5 pollution concentration observations of city i, j; the average of all cities; the spatial weight matrix. Moran’s I index is between [−1,1], less than 0 for negative correlation, equal to 0 for unrelated, and greater than 0 for positive correlation.
Using local spatial autocorrelation to determine the specific location of PM2.5 spatial agglomeration, Moran’s I index formula is:(2)
where Ii is Moran’s I index; ; And xi is the attribute of i;
is its average; wij is the spatial weight matrix.
For Global Moran’s I and Local Moran’s I statistical tests can be used z test, which is , at 95% confidence level, if
, it means statistically significant. If Z > 1.96 and the unit and its adjacent unit PM2.5 concentration values are higher (lower) than average, then called “high – high (low – low)” gathering area, if Z < 1.96, there is a spatial negative correlation, that is, high (low) PM2.5 concentration unit is low (high) PM2.5 concentration unit surrounded by high – low (low – high) correlation. When Z = 0, the observed values are independently distributed randomly.
2.2.2. Spatial correlation verification
The spatial autocorrelation analysis of PM2.5 concentration data was carried out by using the spatial analysis model of ArcGIS 10.2 platform. The Gi-Bin field was used to identify the statistical significance of “hot spot” and “cold spot”. The statistical significance of 99% of the statistical confidence is that the research elements are in the confidence interval [−3,3]; the statistical significance of 95% of the statistical significance means that the analysis element is in the confidence interval [−2,2]; The statistical significance of 90% of the statistical significance means that the analysis element is in the confidence interval [−1,1]; the clustering analysis between the confidence values of 0 or the approaching elements is not statistically significant (Wang et al., Citation2014).
The annual, quarterly and monthly mean values of PM2.5 concentrations in 180 cities in 2013–2016 in BTH region were tested by ArcGIS software (Only quarterly and monthly in 2013). Table shows that, Moran’s I index is positive and Z (I) is higher than 2.58, all through the significance test of 0.001, that is, the concentration of PM2.5 in BTH cities has significant clustering characteristics, significant seasons and month-by-month differences.
Table 1. spatial autocorrelation index of PM2.5 concentrations quarterly in the BTH region during 2013–2016.
3. Results
3.1. Temporal and spatial variation of PM2.5 in the BTH region from 2013 to 2016
From 2014 to 2016, the average annual PM2.5 concentration of air quality in BTH region showed a declining trend as a whole. In 2014, the annual average concentration of PM2.5 was 89.15 μg/m3, and it was 74.50 μg/m3 in 2015, and decreased by 14.65 μg/m3; 2016 years PM2.5 annual average concentration of 70.11 μg/m3, down 19.04 μg/fm3. From the point of view of space, the Moran’s I index decreased gradually from 2014 to 2016, which indicated that the spatial clustering of PM2.5 in the county – level cities in Beijing–Tianjin–Hebei region was decreasing in 2014–2010.
Quarterly, the summer and autumn of 2016 have improved compared with the same period in 2013 (Table ). Except for the autumn of 2016 than in the same period in 2015 increased by 4.54 μg/m3 (6.82%), the others fell, which in 2016 compared with the same period in 2014 decreased by 16.78–25.37% between 2016 compared to 2015, the decline in the range of 2.1–15.72%.
Table 2. BTH quarter PM2.5 concentration changes range from 2013 to 2016.
Monthly view, PM2.5 monthly average concentration of U-shaped pattern, that is, in December and January the highest, August, September the lowest during 2014–2016 (Figure ). In July 2014, the average concentration of PM2.5 in BTH region reached 85.24 μg/m3, higher than the same period in 2013, which was consistent with the results released by the Ministry of Environmental Protection. Due to high temperatures in July, less precipitation less airborne pollutants in the poor climatic conditions. In June 2013, the average concentration of PM2.5 in BHT region was also high, reaching 89.77 μg/m3. The area of heavy pollution in BTH region was 21.2% and the main pollutant was PM2.5 (Li, Chen, & Zhao, Citation2015b). The overall trend of change is from January to November in 2016, PM2.5 monthly average of the value of 2014 have decreased, an average decline of 24 μg/m3 (decline rate of 27.27%). Which decreased by 42 μg/m3 (descending ratio of 33.2%), 56 μg/m3 (descending ratio of 45.09%) and 43 μg/m3 (decline rate of 37.66%), respectively, and decreased by 6 μg/m3 in June and October, respectively. The decrease in other months was between 9 and 27 μg/m3, and the average decrease was 20.65%.
December in 2015 and 2016, PM2.5 concentration is high, higher than the same period in 2014 were more than 30 μg/m3, mainly due to this period of BTH region 13 cities except Zhangjiakou other cities have experienced Severe and more polluted weather and mostly in more than ten days. The Ministry of Environmental Protection explained that the 2015 and 2016 EI Nino event had significant effects on air pollution in northern China, including the BTH region, in which air pollution was occurred repeatedly.
In this paper, the trend of daily mean value was analysed by the statistics of 13 cities in BTH, which met the daily limit of 75 μg/m3 of PM2.5 concentration in the second-class environmental functional area. In 2016, PM2.5 average number of days in BTH was 231 days (Compliance rate was 63.11%), which was 33 days higher than that of 198 days (Compliance rate was 54.24%) in 2015, compared with 156 days in 2014 (Compliance rate was 42.73%) increased by 75 days, in 2013, the total number of days in the 242 days was 129 days. In August from 2014 to 2016, the average compliance rate was 76.67% in August, and the lowest in December was 36.72%. Overall, 2014 to 2016 BTH region air quality improved year by year. In the past few years, Zhangjiakou, Chengde and Qinhuangdao are 13 cities in the annual PM2.5 standard days the highest proportion of the highest, are higher than 60%. In 2016, the number of days of severe pollution and severe pollution in 2014 was also reduced. In 2014, the average number of days of heavy pollution in BTH was 41 to 20 days in 2016, and severe pollution dropped from 17 to 8 days.
3.2. Spatial variability of PM2.5 concentration in BTH region from 2013 to 2016
From 2014 to 2016, the spatial distribution of PM2.5 concentration in the BTH region is characterized by increasing spatial pattern from north-west to south-east and very different in north and south. The concentration of PM2.5 in the southern part of Hebei Province is the highest, and the haze is the most serious (Figure ). Ranking from the PM2.5 concentration, Baoding, Xingtai, Hengshui and Handan four cities in the average annual concentration of 13 cities in the top four, affected by the industrial structure, high energy consumption in Hebei Province accounted for a large proportion of energy consumption Fast, PM2.5 sources are mainly sources of industrial and civilian combustion, with the smallest contribution to the source of traffic (Wei, Wang, & Pan, Citation2013; Wang, Xu, & Yang, Citation2012). View the change range, from 2014 to 2016, 13 cities PM2.5 annual average interest rate fell, the larger decline in the city is Baoding, Tangshan, Xingtai (down more than 30%). Nearly three years of BTH region, the overall situation of air pollution has been a certain improvement.
Figure 3. Spatial distribution and change of annual average PM2.5 concentration in BTH region in 2014–2016.
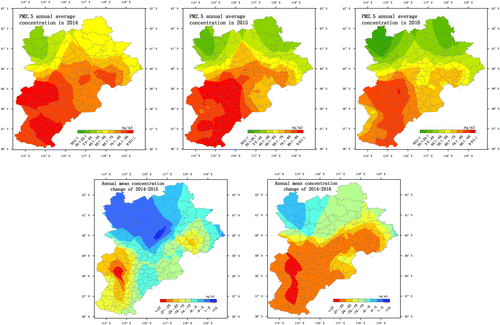
According to GB3095-2012 annual average value of 35 μg/m3 statistics, 2014–2016 3 years in Beijing and Tianjin 13 cities PM2.5 concentrations are exceeded, the average number of days exceeded the number of gradually reduced to 335, 312 and 301 days. In 2014, 11 cities the number of days more than 300 days except Zhangjiakou and Qinhuangdao, the number of days in 2015 less than 300 cities increased Chengde and Beijing, in 2016 and on this basis, increased Lang-fang. According to the PM2.5 concentration of the annual average exceeded the rate of statistics, 2014–2016 13 cities in BTH, the average number of days were 207, 167 and 133 days, gradually decreasing each year. Which in 2014 exceeded the number of days more than 200 days of the city accounted for 8, 2015 reduced to Hengshui, Handan, Xing-tai and Baoding four cities, only Hengshui over the number of days is greater than 200, other cities over the number of days between 53 and 194 in 2016.
The seasonal pollution rate of PM2.5 in BTH region is larger than that in summer, and there is statistical data in autumn, the pollution range of PM2.5 in 2014–2016 showed the most extensive and serious winter, the smallest in summer and the spatial distribution of spring and autumn. The high pollution range from 2014 to 2016 is narrowing. In the winter of 2014 (90 days) PM2.5 daily average of 66.48% (56 days), 63.76% (54 days) in 2015, 48.1% in the winter of 2016 (91 days) Day), excessive rate gradually reduced. 2014 and 2015, except Zhangjiakou, Chengde, Qinhuangdao other 10 cities outside the standard rate of more than 50% to 2016 exceeded the rate of more than 50% of the city only Hengshui, Xingtai, Baoding and Shijiazhuang, other cities exceeded 12–50%. The average over-standard rate in spring was lower year-on-year, from an average of 63.18% (55 days) in the spring of 2014 to 49.96% (43 days) in 2015 to 38.36% (33 days) in 2016, the number of cities of Excessive rate of more than 50% is gradually reduced, 2014, 10 cities to 2016 only Baoding, Handan and Hengshui three cities exceeded the rate of more than 50%. The average exceeded rate in the autumn of 2014 was 52.97% (45 days), 33.25% (29 days) in 2015 and 42.46% (70 days) in 2016. In the autumn of 2014 exceeded the rate of more than 50% of the city is seven cities in Hebei Province, by 2015 only Hengshui, other cities exceeded the standard rate of 9–49%, 2016 Shijiazhuang, Baoding, Xingtai and Tangshan exceeded the rate of more than 50%, other cities in between 8 and 47%. Summer (92 days) exceeded the standard year by year, 44.56% (39 days) in 2014 to 36.33% (32 days) by 2015, 17.17% (15 days) in 2016 and 13 cities in 2016 PM2.5 Daily average exceeded the rate of 40% or less.
Monthly, January 2014 (average daily average exceeded the standard rate of 76.92%, 23 days) BTH region PM2.5 pollution concentration, the largest pollution, 2015 and 2016 is in December, exceeded the rate of 65.26% (20 days) and 70.22% (79 days). January 2014 addition to Chengde and Zhangjiakou average daily average exceeded the rate of 32%, the other cities exceeded the standard rate of 61% or more, and in 2015 and December 2016 except Zhangjiakou, Chengde and Qinhuangdao other cities beyond the standard Rates are more than 60%. However, from 2014 to 2016 the Beijing–Tianjin–Hebei region exceeded the rate of more than 50% of the city gradually reduced.
3.3. Spatial agglomeration law of PM2.5 concentration in BTH region from 2014 to 2016
In the past three years, there are similar clustering characteristics in the BTH region. The heavy disaster area of PM2.5 pollution are mainly distributed in the southwest of Hebei Province, and the areas with relatively good air quality are mainly concentrated in the northern part of Hebei Province and Beijing Part of the area. Specifically, as shown in Figure : hot spots are mainly concentrated in Baoding, Shijiazhuang, Hengshui, Xingtai, Handan most and Cangzhou a few county-level cities, cold spots are mainly concentrated in Zhangjiakou, Qinhuangdao, Chengde and Beijing Part of the county city. Compared with 2014, the 2016 high-polluted county-level urban agglomeration was slightly narrowed, while the Moran’s I index fell by 0.015, indicating a decrease in agglomeration.
Quarterly analysis, PM2.5 pollution of the spatial agglomeration has obvious seasonal differences. The high-polluted hotspots decreased year by year in the spring of 2014–2016, and Moran’s I index decreased by 0.025 and 0.076, respectively, which indicated that PM2.5 pollution was reduced. The summer of 2014 increased by 0.024 compared to 2013, indicating that the concentration of PM2.5 pollution has increased, but in the spring of 2015 and 2016 compared with 2014 but decreased year by year, respectively, down 0.021 and 0.177. The Moran’s I index changed little in 2014 and 2013, and decreased by 0.038 and 0.033, respectively in 2015 and 2016, The hotspot area is gradually changing from south to north and the hotspot area is shrinking. Among them, many counties in Beijing have become non-characteristic points from cold spot. Winter Moran’s I index decreased by 0.005 compared with 2014, hot areas showing a trend from the west to the east, in particular, several county-level cities in Shijiazhuang and Xingtai of Hebei Province have become non-characteristic areas from hotspots, while several county-level cities in western Cangzhou and Baoding have become hot areas from the former non-characteristic areas. Compared to 2014, a few cold spots in the previous cold areas, including Beijing, Tianjin and Langfang, have become a few feature points in 2015. In the winter of 2016, the Moran’s I index rose by 0.02 compared with 2015, while the hot and cold spots were slightly widened.
4. Discussion and conclusions
4.1 Discussion
The results of this study show that although the air quality in the BTH region has improved, the annual average concentration is still higher than the average annual value of GB3095-2012 35 μg/m3, which is still the hardest hit of PM2.5 pollution in China, especially autumn and winter season is a serious period of pollution. Because of its terrain, industrial energy consumption of the larger industrial structure and energy structure of the impact of BTH region PM2.5 pollution on the whole showed low south high north, a slight prominent local situation. For the BTH region, the higher PM2.5 concentration, the stronger influences meteorological factors exert on PM2.5 concentration (Chang, Xu, & Tie, Citation2016; Environmental Protection Department, Citation2015). Furthermore, this research suggests that individual meteorological factors can influence local PM2.5 concentration indirectly by interacting with other meteorological factors (Chen et al., Citation2017). Furthermore, meteorological variables have considerable effects on the dispersion and distribution of pollutants, which also depends on spatial-temporal emission patterns (Ramos, St-Onge, & Blanchet, Citation2015).
Han used Beijing’s meteorological visibility data to assess the relationship between PM2.5 in Beijing and population, urbanization, motor vehicles, and GDP from 1973 to 2013, and demonstrated that the concentration of PM2.5 was positively correlated with population size, GDP, energy consumption and the number of motor vehicles (Hu, Wang, & Ying, Citation2014). H and other research results show that In the entire BTH area, southern Hebei (SHB) represented the largest internal contribution (33%), while the main external contributions came from Shandong (SD) (10%) and Henan (HN) (4%) (Cai, Wang, & Zhao, Citation2016; Chen, Chai, & Yan, Citation2015b; Zhongzhi, Wenxing, & Miaomiao, Citation2017). Yale University has published the Environmental Performance Index (2016 report), which ranks the air quality of 180 countries around the world by measuring the index, and China has become the second largest World PM2.5 exceeded the hardest hit (Yale University, Citation2016). Therefore, the state of air pollution control of the road long way to go, need to consider a wide range of factors, comprehensive management.
4.2. Conclusions
Compared with 2014, the annual average concentration of PM2.5 in 13 cities in BTH region decreased by 16.43% in 2015 and 21.36% in 2016. Among them, the autumn air quality improvement of the largest, the smallest in spring. In the period from 2014 to 2016, the PM2.5 monthly concentration of the BTH region was the highest in August and January, the lowest in August and September, it has a significant ‘U’ shape change law. The concentration of PM2.5 during the heating period of BTH region is several times that of non-heating period [22]. Since 2013, the State Council issued an action plan [23], the Ministry of Environmental Protection to take a variety of measures: a comprehensive start to clean coal instead of clean, focus on comprehensive industry remediation, to strengthen the motor vehicle environmental management, strengthen the environmental law enforcement supervision, regardless of heavy pollution days or pollution levels are declining, air quality has improved. Since 2014, the number of days of pollution has decreased year by year, in 2014 to 207 days to 2015, 167 days, to 2016 down to 133 days. The reasons for the improvement of air quality are diversified, and the data of several years can’t determine the long-term or contingency conclusion of the change of PM2.5 concentration in the study area. In addition to government-man-made measures, air quality is also affected by meteorological conditions. In 2014, the overall influence of cold air in China is weak and the atmospheric pollution is generally poor. The BTH region has a 6% higher capacity (56 days) of the atmospheric environment compared with the same period in the past decade, resulting in air pollution Things continue to gather, resulting in haze weather [24]. Chang L, Xu J, Tie X, et al. [25] pointed out that the changes in weather conditions attributed to the ENSO events could lead to significant effects on the haze occurrences in the NCP and other regions of China. Thus, the BTH region PM2.5 pollution is also closely related to climate factors, the air pollution control results have to be observed through long-term monitoring data.
From the spatial distribution of PM2.5, the spatial distribution of PM2.5 concentration in the Beijing–Tianjin–Hebei region increased from north-west to south-east in 2014–2016, and the haze in the south of Hebei Province was the most serious. 13 cities PM2.5 annual average concentration has fallen, nearly three years of Beijing–Tianjin–Hebei region, the overall situation of air pollution has improved. In the autumn of 2013, the pollution range was larger than that in the summer. The pollution range of PM2.5 in 2014 to 2016 showed the most extensive and severe winter, the smallest in summer and the spatial distribution of spring and autumn. Monthly analysis, 2014–2016, January and December the most polluted and the most extensive but the overall rate of more than 50% of the city gradually reduced.
From the spatial clustering characteristics of PM2.5, the PM2.5 high pollution area in the BTH area is mainly distributed in the south, and the area with relatively good air quality is mainly concentrated in the northern part of Hebei Province and some parts of Beijing. Compared with 2014, the concentration of high-polluting county-level cities in 2016 was slightly narrowed, and the degree of agglomeration was reduced. Quarterly analysis, with significant seasonal differences. In the spring of 2014, the concentration of PM2.5 pollution was reduced. In the spring of 2014, the degree of agglomeration increased by 2013, however, 2015 and 2016 spring than in 2014 year after year decline. The hot spots in the autumn and the autumn of 2016 show a trend of gradual change from south to north and the hotspot area is shrinking. Among them, many counties in Beijing have become non-characteristic points from cold spots. The hot spots in 2014 show a trend from the west to the east in 2015, and several of the cold spots in 2014 include a few county-level cities in Beijing, Tianjin and Langfang that became non-characteristic in 2015 point. 2016 winter hot spots and cold spots slightly expanded, compared with 2014
Disclosure statement
No potential conflict of interest was reported by the authors.
References
- Cai, S., Wang, Y., & Zhao, B. (2016). The impact of the “Air Pollution Prevention and Control Action Plan” on PM2.5 concentrations in Jing-Jin-Ji region during 2012–2020. Science of the Total Environment, 580, 197–209.
- Chang, L., Xu, J., & Tie, X. (2016). Impact of the 2015 El Nino event on winter air quality in China. Scientific Reports, 6, 34275.10.1038/srep34275
- Chen, H., Li, J., & Ge, B. (2015a). Modeling study of source contributions and emergency control effects during a severe haze episode over the Beijing–Tianjin–Hebei area. Science China Chemistry, 58, 1403–1415.10.1007/s11426-015-5458-y
- Chen, J.F., Chai, R.R., & Yan, H. (2015b). PM2.5 pollution source diffusion law and simulation analysis based on the gauss plume model. Systems Engineering,, 153–158.
- Chen, Z., Cai, J., & Gao, B., (2017). Detecting the causality influence of individual meteorological factors on local PM2.5concentration in the Jing-Jin-Ji region. Scientific Reports, 7, 40735.
- Environmental Protection Department. (2015, February 2). The air quality condition of key area and 74 cities in China in 2014. Retrieved from http://www.zhb.gov.cn/gkml/hbb/qt/201502/t20150202_295333.htm
- Han, L., Zhou, W., & Li, W. (2016). Fine particulate (PM2.5) dynamics during rapid urbanization in Beijing, 1973–2013. Scientific Reports, 6, 23604.
- Hu, J., Wang, Y., & Ying, Q. (2014). Spatial and temporal variability of PM 2.5, and PM 10, over the North China Plain and the Yangtze River Delta, China. Atmospheric Environment, 95, 598–609.10.1016/j.atmosenv.2014.07.019
- Hua, Y., Cheng, Z., & Wang, S. (2015). Characteristics and source apportionment of PM 2.5, during a fall heavy haze episode in the Yangtze River Delta of China. Atmospheric Environment, 123, 380–391.10.1016/j.atmosenv.2015.03.046
- Li, M.S., Ren, X.X., & Yu, Y. (2016). Spatiotemporal pattern of ground-level fine particulate matter (PM2.5) pollution in mainland China. China Environmental Science, 2016, 641–650.
- Li, X., Zhang, Q., & Zhang, Y. (2015a). Source contributions of urban PM 2.5, in the Beijing–Tianjin–Hebei region: Changes between 2006 and 2013 and relative impacts of emissions and meteorology. Atmospheric Environment, 123, 229–239.10.1016/j.atmosenv.2015.10.048
- Li, Y., Chen, Q., & Zhao, H. (2015b). Variations in PM10, PM2.5 and PM1.0 in an urban area of the sichuan basin and their relation to meteorological factors. Atmosphere, 6, 150–163.10.3390/atmos6010150
- Liao, T., Shan, W., & Jie, A., (2017). Heavy pollution episodes, transport pathways and potential sources of PM 2.5, during the winter of 2013 in Chengdu (China). Science of the Total Environment, 584–585, 1056–1065.
- Liu, G., Bi, R., & Wang, S. (2013). The use of spatial autocorrelation analysis to identify PAHs pollution hotspots at an industrially contaminated site. Environmental Monitoring & Assessment, 185, 9549–9558.10.1007/s10661-013-3272-6
- Luo, J., Du, P., & Samat, A., (2017). Spatiotemporal pattern of PM2.5 concentrations in mainland china and analysis of its influencing factors using geographically weighted regression. Scientific Reports, 7, 40607.
- Ramos, Y., St-Onge, B., & Blanchet, J.P. (2015). Spatio-temporal models to estimate daily concentrations of fine particulate matter in Montreal: Kriging with external drift and inverse distance-weighted approaches. Journal of Exposure Science & Environmental Epidemiology, 26, 405–414.
- Tan, Q., & Xu, X. (2014). Comparative analysis of spatial interpolation methods: An experimental study. Sensors & Transducers, 165, 155–163.
- Wang, J., Wang, S., Voorhees, A S, Zhao, B., Jang, C., Jiang, J., … Hao, J. (2015). Assessment of short-term PM 2.5 -related mortality due to different emission sources in the Yangtze River Delta, China. Atmospheric Environment, 123, 440–448.10.1016/j.atmosenv.2015.05.060
- Wang, L., Xu, J., & Yang, J. (2012). Understanding haze pollution over the southern Hebei area of China using the CMAQ model. Atmospheric Environment, 56, 69–79.10.1016/j.atmosenv.2012.04.013
- Wang, Y., Ying, Q., & Hu, J. (2014). Spatial and temporal variations of six criteria air pollutants in 31 provincial capital cities in China during 2013–2014. Environment International, 73, 413–422.10.1016/j.envint.2014.08.016
- Wei, W., Wang, L.T., & Pan, X.M. (2013). Simulation study of Haze pollution sources in cities of Hebei Province. Environmental Science & Technology, 2, 24.
- Xu, H., Ho, S.S., & Cao, J., (2017). A 10-year observation of PM2.5-bound nickel in Xi’an, China: Effects of source control on its trend and associated health risks, Scientific Reports, 7, 41132.
- Yale University. (2016). Global metrics for the environment: The 2016 environmental performance index. Retrieved from http://epi.yale.edu/downloads
- Zhang, A., Qi, Q., & Jiang, L. (2013). Population exposure to PM2.5 in the urban area of Beijing. PLoS One, 8(5), e63486.10.1371/journal.pone.0063486
- Zhang, H., Wang, Z., & Zhang, W. (2016). Exploring spatiotemporal patterns of PM 2.5, in China based on ground-level observations for 190 cities. Environmental Pollution, 216, 559–567.10.1016/j.envpol.2016.06.009
- Zhongzhi, Z., Wenxing, W., & Miaomiao, C. (2017). The contribution of residential coal combustion to PM 2.5, pollution over China’s Beijing–Tianjin–Hebei region in winter. Atmospheric Environment, 159, 147–167. doi:10.1016/j.atmosenv.2017.03.054