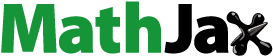
We congratulate the authors for this engaging article. It gives us an opportunity to gaze at the idea of BIC and its generalisations. This article provides several important examples and insightful explorations of the issues of using BIC. To overcome the related problems, the article gives detailed steps on defining the proposed PBIC(PBIC*), and illustrates the use of them. Different from BIC, there are two highlights in the new approaches: (1) PBIC(PBIC*) goes to as
, where
is the marginal likelihood function based on specified prior; (2) corresponding to each transformed parameter based on the non-common parameters in the model selection problem, there is an individualised term determined by the related observed information content and prior in PBIC(PBIC*). (1) shows that PBIC(PBIC*) not only generalises the idea of BIC but also can be directly related to Bayes factor (BF) (without having to ignore a constant term); while (2) allows flexibility for the situations where p depends on n and/or different parameters have different n (the so-called effective sample size). The proposed approach based on PBIC(PBIC*) is applicable for a wide range of problems, and can be a promising statistical inference tool for model selection problems.
Based on the discussions on BIC and PBIC(PBIC*), it turns out that both the sample size ‘n’ and the parameter dimension ‘p’ require careful definitions. According to Giraud (Citation2015), the BIC suffers from two main limitations: (1) the approximation (based on Taylor expansion) is only valid for sample size n much larger than the number p of parameters in the model; (2) the BIC cannot handle complex collections of models as in the variable selection problem in high-dimension. PBIC can be a promising tool to break through both of these limitations. Corresponding to the ‘non-common’ parameters of interest, there are two keys to success: first, a good prior which is meaningful and beneficial for computation; second, a well-defined effective sample size. The second point is especially challenging, and we speculate that the partial reason is that the original definition of effective sample size is for the situation where the observations in the sample are weighted (or correlated), but here, the weights (or correlations) are not always clear.
It is well known that the use of p-values in hypothesis testing problems has been questioned, especially in recent years (see, e.g., Baker, Citation2016; Benjamin et al., Citation2017; Chawla, Citation2017; Nuzzo, Citation2014; Wasserstein & Lazar, Citation2016). Even if ‘classical’ hypothesis testing procedure is used to perform step-wise algorithm, model selection is still difficult (Marden, Citation2000). It is not surprising that statistical inference tools derived from Bayesian side can be good solutions. Among the Bayesian solutions, BF and BIC are widely used and developed to tackle with difficult problems. PBIC(PBIC*) not only generalises BIC but also is closely related to BF. We can expect that, for comparing two models, using PBIC(PBIC*) can provide similar results as using BF under the corresponding priors. It will be interesting to make some comparisons to BF under different choices of priors. More importantly, we note that there have been complaints/doubts in the use of BF. Then, can we use the idea of PBIC(PBIC*) in calculating BF, e.g., approximate by
? We would like to take the opportunity to raise this question for discussion. The authors have more in-depth understandings of the issue and they may well wish to correct us, if we are mistaken or have missed something.
First of all, computation of BF is very often a problem, since original BF is a ratio of integrals. PBIC(PBIC*) has a closed form without integral, and can be used directly for calculations. Second, the proposed prior (in PBIC/PBIC*) has two parts: (a) a constant prior for common parameters; (b) a subjective prior for non-common parameters. Part (a) indicates that the prior is as objective as possible, which is often preferred. Part (b) is data-dependent but only relies on the effective sample size, therefore, we do not worry ‘double use’ the data too much. Last but not least, Lavine Schervish (Citation1999) pointed out the ‘averaging’ in BF has at least two potential drawbacks: ‘first, it requires a prior to average with respect to, and second, it penalises a hypothesis for containing values with small likelihood.’ On the contrary, this article notes that ‘the fat tails of the prior (in PBIC) do result in reasonable answers’ (c.f. Bayarri, Berger, Forte, & García-Donato, Citation2012; Jeffreys, Citation1961). On this issue, this article provides PBIC* as an intermediate solution, where the prior gives more mass to the region of high model likelihood. This in fact may lead to an intermediate solution to the Lindley's Paradox.
Disclosure statement
No potential conflict of interest was reported by the authors.
Additional information
Notes on contributors
Sifan Liu
Sifan Liu received his PhD in Statistics from University of Missouri in 2015. He is a lecturer at Tianjin University Of Finance & Economics. His research interest includes Bayesian methodology, smoothing splines, hypothesis testing, meta-analysis and confidence distribution.
Dongchu Sun
Dongchu Sun received his PhD in Statistics from Purdue University in 1991. He is a professor of Statistics at East China Normal University and University of Missouri. His research interest includes Bayesian methodology, small area estimation, multivariate time series, space-time and longitudinal models, generalized linear mixed models, smoothing splines and statistical computation.
References
- Baker, M. (2016). Statisticians issue warning on P values. Nature, 531, 151. doi: 10.1038/nature.2016.19503
- Bayarri, M. J., Berger, J. O., Forte, A., & García-Donato, G. (2012). Criteria for Bayesian model choice with application to variable selection. The Annals of Statistics, 40, 1550–1577. doi: 10.1214/12-AOS1013
- Benjamin, D. J., Berger, J. O., Johannesson, M., Nosek, B. A., Wagenmakers, E., Berk, R., …Johnson, V. E. (2017). Redefine statistical significance. Nature Human Behaviour, 2, 6–10. doi: 10.1038/s41562-017-0189-z
- Chawla, D. S. (2017). Big names in statistics want to shake up much-maligned P value. Nature, 548, 16–17. doi: 10.1038/nature.2017.22375
- Giraud, C. (2015). Introduction to high-dimensional statistics. Chapman & Hall, CRC.
- Jeffreys, W. H. (1961). Theory of probability (3rd ed.). Oxford, UK: Clarendon Press.
- Lavine, M., & Schervish, M. J. (1999). Bayes factors: What they are and what they are not. The American Statistician, 53, 119–122.
- Marden, J. I. (2000). Hypothesis testing: From p values to Bayes factors. Journal of the American Statistical Association, 95, 1316–1320.
- Nuzzo, R. (2014). Scientific method: Statistical errors. Nature, 506, 150–152. doi: 10.1038/506150a
- Wasserstein, R. L., & Lazar, N. A. (2016). The ASA's statement on p-values: Pontext, process, and purpose. The American Statistician, 70, 129–133. doi: 10.1080/00031305.2016.1154108