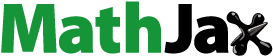
1. General comment
Let me start by acknowledging Dr Jun Shao's (the Editor of the journal) invitation to participate in the discussion of the exciting review paper, and congratulations to Dr Yichun Chi and Dr Jun Cai for their excellent work. It is my honour to have the opportunity to present some of my personal views on the topic of optimal reinsurance design. Dr Chi and Dr Cai's review paper provides an informative summary of the main developments of risk measure minimisation based optimal reinsurance design over the last decade.
A typical optimal reinsurance design model includes the following elements: (1) a risk or reward function adopted as the objective to measure an insurer's position; (2) a principle used to calculate reinsurance premium; (3) an admissible set of ceded loss functions (also called indemnification function). The complexity of the resulting optimisation problem depends on all the three elements. The adopted objectives in the optimal reinsurance design literature include expected utility maximisation, insolvency probably minimisation, variance minimisation, etc., in addition to risk measure minimisation. Risk measure minimisation has been the central paradigm in the literature over the last decade, primarily due to the popularity of risk measures in determining capital adequacy under various modern financial and insurance regulatory frameworks and quantitative risk management paradigms.
From a technical point of view, the optimal reinsurance is a challenging problem because it is an infinite-dimensional optimisation problem, and often it is highly nonlinear by its original form. As introduced by Dr Chi and Dr Cai's review paper, Cai et al. (Citation2008) is the first paper addressing the optimal solution among a class of functions under the risk measures VaR and CVaR. They constructed a subset of ceded loss functions with an explicit representation and managed to show that the subset is dense among the original admissible set; they then focused on the subset for an optimal solution. Because of the nature of the optimisation problem, there is no structured method available for one to follow to obtain optimal solutions for a general optimal reinsurance design model. As I observe, so far the construction method might be the most effective way to study solutions to a variety of reinsurance models under risk measures such as VaR and CVaR. The construction method entails an initial guess on the shape of an optimal solution followed by a formal verification procedure to ensure that the initial guess is indeed a correct optimal solution. Somehow one can also view the method used in Cai et al. (Citation2008) as a construction method because it involves the construction of candidate solutions followed by a formal verification procedure. The major limitation of the construction method lies in its impotence when there is no clue to the shape of the optimal solutions.
In this note, I would like to bring up to readers' attention two approaches for the study of optimal reinsurance other than the construction method. The first one is the marginal indemnity function (MIF) formulation adopted by Zhuang et al. (Citation2016),Footnote1 and the empirical approach proposed by Tan and Weng (Citation2014) and Sun et al. (Citation2017).
2. MIF formulation
The MIF formulation is an effective way to explore the optimal reinsurance among the admissible set (see Dr Chi and Dr Cai's review paper for the definition) under distortion risk measures. It is interesting to consider the combination of the distortion risk measure and the admissible set
. On the one hand, with any ceded loss functions from
, both the insurer's retained loss and the ceded loss are nondecreasing functions of the underlying risk X. This property is useful to discourage immoral behaviours from both contract parties. On the other hand, distortion risk measures are a broad set of risk measures in the field of risk management and include both the VaR and CVaR as special cases.
The MIF formulation is motivated by the observation that every function is absolutely continuous, and thus, it is almost everywhere differentiable, i.e., there exists a Lebesgue integrable function h such that
(1)
(1) Here
is the slope of the ceded loss function f at z, and thus, we must have
for every
. The function
can be interpreted as the ‘marginal indemnification’ from an increase of the loss X. Thus, the function h is referred to as a ‘marginal indemnification function (MIF)’. Obviously, two MIFs only differing from each other over a Lebesgue null set result in the same ceded loss function f everywhere.
With representation (Equation1(1)
(1) ), it is sufficient to determine an MIF in order to obtain an optimal ceded loss function among the admissible set
. With the MIF formulation, we can go with a more general set-up. Define
(2)
(2) where
and
are two constants satisfying
. Let
(3)
(3) Using representation (Equation1
(1)
(1) ), it is easy to check that the admissible set
can be equivalently written as
(4)
(4) If we set
and
, the set
reduces to the set
.
Let us consider the following set of distortion risk measures:
As showed in Lemma 2.1 of Zhuang et al. (Citation2016), given any
and
, there exists
, independent of g, such that
and
(5)
(5) where
is a distortion risk measure with the distortion function g.
If we consider the admissible set , which is more general than
because the constants
and
can be exogenously given, the constrained problem discussed in Section 5 of Dr Chi and Dr Cai's review paper writes:
(6)
(6) where
and
are two distortion functions from
,
is a constant denoting the reinsurance premium budget. Using representation (5), the objective in Problem (6) can be rewritten into
(7)
(7) where
(8)
(8) Since
is a constant, it suffices to analyse the term
for optimal solutions of Problem Equation6
(6)
(6) . As a consequence, Problem Equation6
(6)
(6) can be transformed into an MIF formulation as follows:
(9)
(9) where
.
The MIF formulation brings much convenience to study optimal reinsurance contracts:
MIF formulation (Equation9
(9)
(9) ) is a linear programming problem and, thus, much technically convenient to study the optimal solution. As shown by Zhuang et al. (Citation2016), with the MIF formulation, it becomes feasible to discuss the existence and uniqueness of a solution to the optimal reinsurance model. In contrast, the construction method is impotent to determine whether the solution obtained is unique.
With the MIF formulation, one does not need to develop a conjecture on the shape of optimal reinsurance in solving the problem but one needs to do so by the construction method. As demonstrated by Zhuang et al. (Citation2016), a Lagrangian method combined with a pointwise optimisation procedure can be applied to discuss the optimal solution under the MIF formulation. Such a procedure does not require any pre-analysis on the shape of an optimal solution.
By the definition of the admissible set
, the MIF formulation allows us to consider a more general setup for the optimal reinsurance contracts. In particular, by choosing the constants
and
, one can ensure that both the resulting reinsurance indemnity and the insurer's retain loss are strictly increasing in the underlying risk X. As pointed by Balbás et al. (Citation2015), in practice, reinsurers rarely accept a contract which leads to the lack of incentives of the insurer to verify claims. To rectify this, Balbás et al. (Citation2015) proposed to impose a strictly positive lower bound on the derivative of admissible retained loss functions, which is equivalent to imposing an upper bound on the derivative h of the admissible ceded loss functions. Furthermore, the analysis in Zhuang et al. (Citation2016) can be extended by varying lower and upper bounds
and
, i.e. when
and
are two ordered functions with values within
.
Last but not least, if we adopt the empirical formulation which we will introduce in the next section, the MIF formulation yields a linear programming problem in finite dimension, and thus, it is extremely convenient to use various softwares to obtain an optimal empirical solution.
3. Empirical approach
Dr Chi and Dr Cai have pointed out the uncertainty of the underlying distribution in practice when we consider the problem of optimal reinsurance design. They put in Section 8 of their paper that ‘In most of optimal reinsurance problems, it is assumed that the distributions of the insurer's risks are given or known. However, in practice, the exact distributions of the insurer's risks are difficult to be obtained.’ As a remedy, it is appealing to consider the empirical approach adopted by Tan and Weng (Citation2014) and Sun et al. (Citation2017). The empirical approach formulates an optimal reinsurance model directly on the available data, and thus statistical analysis enters to play a role. Statistical analysis is very scarce in the literature of optimal reinsurance while it is necessary to make an optimal reinsurance model legitimate for practical use; thus, I personally believe it is necessary to bring it up in this note for readers' attention.
Tan and Weng (Citation2014) proposed a general framework to formulate a typical optimal reinsurance model into an empirical formulation. The empirical approach starts with a theoretical model:
(10)
(10) where ρ is an appropriately chosen risk measure. It is possible to consider more constraints with the model.
The empirical approach assumes that an insurer has observed empirically N losses (or claim amounts), denoted , where
corresponds to the ith loss. The method seeks an optimal reinsurance coverage
attached to each loss observation
. Obviously,
is the decision variable and has yet to be determined. Let
. The principle underlying the empirical-based reinsurance model is to formulate the optimisation model involving both
and
directly. More specifically, corresponding to theoretical model (Equation10
(10)
(10) ), the empirical reinsurance model can be formulated in the following symbolic form:
(11)
(11) where the variables
and
can be interpreted as the empirical estimates of
and
in model (Equation10
(10)
(10) ), respectively.
The above formulation of the empirical-based reinsurance model is an optimisation problem of N dimensions. By denoting as the resulting solution to empirical model (Equation11
(11)
(11) ), the optimal ceded loss function is represented as a set of finite points
,
, instead of a ceded loss function
in terms of x. Generally, some standard smoothing techniques such as spline interpolation can always be used if we were interested in a smooth (or piecewise smooth) ceded loss function. Tan and Weng (Citation2014) has discussed in great detail the computational aspect of the resulting empirical model, and showed that for many interesting reinsurance premium principles and objectives, the resulting empirical model can be cast into a second-order-conic programming problem and thus numerically convenient to obtain an accurate optimal solution. The stability and sensitivity of the empirical optimal solutions under interesting risk measures (such as variance and CVaR) have been demonstrated. However, it is yet for us to establish rigorous analysis regarding the consistency and convergence rate of the solutions obtained from an empirical model.
Sun et al. (Citation2017) adopted CVaR to measure the total loss of multiple lines of insurance business and introduced two nonparametric estimation methods to explore the optimal multivariate quota-share reinsurance under a mean-CVaR framework. The general dependence structure makes it almost impossible to explore an optimal solution directly based on a probabilistic model. Following the empirical approach, Sun et al. (Citation2017) formulated the optimal reinsurance design problem on empirical data and made no explicit distributional assumption on the underlying risk vector. The resulting nonparametric reinsurance models are convex and computationally amenable, circumventing the difficulty of computing CVaR of the sum of a generally dependent random vector. Statistical consistency of the resulting estimators for the best CVaR is established for both nonparametric models, allowing empirical data to be generated from any stationary process satisfying strong mixing conditions. Sun et al. (Citation2017) conducted extensive numerical experiments to show that a routine bootstrap procedure can capture the distributions of the resulting risk measures well for independent data.
Finally, it is worth mentioning an additional merit of the empirical approach. The empirical model will maintain the same level of tractability when a background risk is included in the model; on the contrast, it is notoriously challenging to study the optimal reinsurance when the background risk is considered and when it is generally dependent on the underlying insurable risk.
Disclosure statement
No potential conflict of interest was reported by the author(s).
Additional information
Notes on contributors
Chengguo Weng
Chengguo Weng, a professor of actuarial science, has primary research interests in quantitative risk management, finance, and insurance applications.
Notes
1 The MIF formulation was first applied by Assa (Citation2015) for solving an unconstrained optimal reinsurance model.
References
- Assa, H. (2015). On optimal reinsurance policy with distortion risk measures and premiums. Insurance: Mathematics and Economics, 61, 70–75. https://doi.org/10.1016/j.insmatheco.2014.11.007
- Balbás, A., Balbás, B., Balbás, R., & Heras, A. (2015). Optimal reinsurance under risk and uncertainty. Insurance: Mathematics and Economics, 60, 61–74. https://doi.org/10.1016/j.insmatheco.2014.11.001
- Cai, J., Tan, K. S., Weng, C., & Zhang, Y. (2008). Optimal reinsurance under VaR and CTE risk measures. Insurance: Mathematics and Economics, 43(1), 185–196. https://doi.org/10.1016/j.insmatheco.2008.05.011
- Sun, H., Weng, C., & Zhang, Y. (2017). Optimal multivariate quota-share reinsurance: A nonparametric mean-CVaR framework. Insurance: Mathematics and Economics, 72, 197–214. https://doi.org/10.1016/j.insmatheco.2016.11.006
- Tan, K. S., & Weng, C. (2014). Empirical approach for optimal reinsurance design. North American Actuarial Journal, 18(2), 315–342. https://doi.org/10.1080/10920277.2014.888008
- Zhuang, S. C., Weng, C., Tan, K. S., & Assa, H. (2016). Marginal indemnification function formulation for optimal reinsurance. Insurance: Mathematics and Economics, 67, 65–76. https://doi.org/10.1016/j.insmatheco.2015.12.003