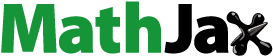
I congratulate the authors on an excellent overview of an important research area. Sufficient dimension reduction methods are based on the model-free driving condition that , where
is multivariate and potentially high-dimensional,
is the projection onto the dimension reduction subspace
. Equipped with variable selection and variable screening techniques, many modern sparse sufficient dimension reduction methods have been developed in the past few years, and they can work really well in the model development stage of high-dimensional data analysis. This review paper is very timely and provides a thorough overview of sparse sufficient dimension reduction methods and sheds lights on future research directions.
Specific contributions of this paper include the following. First of all, it recasts many moment-based sufficient dimension reduction methods as a generalised eigen-decomposition problem and also as a constrained trace optimisation (Equation (2.1) in the paper). These two formulations are crucial for studying sparse sufficient dimension reduction in high-dimensional settings where p>n or . This paper also provides a comprehensive overview of the key developments in sparse sufficient dimension reduction literature, from the first paper by Ni et al. (Citation2005), to the newest theoretical and computational breakthrough in Tan et al. (Citation2020). Various techniques for sparse sufficient dimension reduction are discussed, including the shrinkage and regularised estimation, variable screening and the trace pursuit, and the sequential procedures. Advantages and possible pitfalls of different approaches are well explained by the authors. Finally, the paper provides the theoretical foundations for establishing the minimax rates of convergence for estimating a sparse dimension reduction subspace. Further calculations are also included to provide insights about second-order dimension reduction methods such as SAVE and DR.
This paper is inspiring for substantive research in high-dimensional multivariate statistics, and encouraging for current and future studies in dimension reduction. In what follows, I draw two connections which may be suggestive of future directions.
1. Least squares formulations
In the seminal work of Li and Duan (Citation1989), a well-known connection between the ordinary least square estimator in the regression of Y on and the one-dimensional dimension reduction subspace can be derived. Specifically, consider the following bivariate loss function,
(1)
(1) and define the unique minimiser as follows,
(2)
(2) Then under the linearity condition of dimension reduction subspace, it was shown that
is contained in the central subspace (or any dimension reduction subspace).
A direct consequence of this somewhat surprising result is that one can simply use OLS to extract the direction in nonlinear models of the following form,
(3)
(3) where g is some unknown function, ϵ is the error term, and
spans the central subspace under this model. The model (Equation3
(3)
(3) ) is known as the single-index model when d = 1, and the multiple-index model when d>1. Then Li–Duan Theorem (Li & Duan, Citation1989, Theorem 2.1) implies that the solution
from ordinary least squares estimation, or more generally from (Equation2
(2)
(2) ), lies within the central subspace:
.
For single-index models, this means that from OLS fitting,
, is the same as
in population up to a scalar multiplication (i.e.
for some constant
). As such, we might still use OLS to study regression graphics even when there is a nonlinear relationship between Y and
(Cook, Citation1998). In high-dimensional setting, one may replace OLS with its penalised version such as LASSO regression and achieve consistent variable selection and directional estimation (Neykov, Lin, et al., Citation2016; Neykov, Liu, et al., Citation2016).
For multiple-index models and model-free sufficient dimension reduction, the (sparse) estimation of the central subspace is much more challenging. In high-dimensional sparse sufficient dimension reduction, it is thus desirable to have a penalised least squares formulation. Indeed, as this paper points out, the computationally tractable and rate optimal sparse SIR is eventually obtained by the adaptive estimation scheme based on a least squares formulation (Tan et al., Citation2020). Finally, the paper concludes that ‘Since SAVE and DR can not be rewritten as a least-square formulation, we do not define refined sparse SAVE and DR estimator’. Not surprisingly, developing theoretical solid and computationally feasible methods for high-dimensional SAVE and DR is very challenging and requires substantial efforts.
2. Sparse/constrained canonical correlation
As discussed in Section 3.1 of this paper, sparse sufficient dimension reduction subspace can be obtained by the C method: constrained canonical correlation (Zhou and He, Citation2008). The idea is to estimate the constrained canonical variates between the B-spline basis functions of response transformation,
, where m is the spline order and
is the number of interval knots in B-spline transformation, and the predictor
. Then this procedure can be viewed as an estimation for sparse SIR directions. However, as the authors noted, this method may not be directly applicable to very high-dimensional settings, at least in theory. In the past few years, there are some advances in both the theoretical and computational aspects of high-dimensional canonical correlation analysis. In Mai and Zhang (Citation2019), they solved the sparse canonical correlation analysis (CCA) problem using an iterative penalised least squares approach. This new iterative penalised least squares approach can be directly applied to estimate the sparse sufficient dimension reduction directions when combined with B-spline transformations of the response.
To illustrate the idea, we consider the estimation of the leading CCA directions. For a multivariate and a multivariate
, the leading CCA directions are defined through a pair of linear combinations
and
such that the correlation between them are maximised. When
, the sparse CCA problem assumes that the population solution
and
are both sparse so that we can estimate them with a limited sample size. Then the leading sparse CCA directions can be obtained by solving the following constrained optimisation problem,
(4)
(4) subject to constraints that
and
. Note that the data are centred so that
and
, also that the sample covariance
and
do not need to be positive definite. Then it can be shown that, the above optimisation can be solved by iteratively solving the following two LASSO regression problems. Specifically, the sparse CCA solutions can be obtained as follows,
(5)
(5)
(6)
(6) For sequential sparse CCA directions,
and
,
we can use the similar iterative penalised least squares formulation after deflation of the data from the previous
estimated directions. Theoretical results show that this approach can consistently estimate the population directions (any fix number of pairs
) with an overwhelming probability in ultra-high dimensions
. More importantly, due to its simplicity, the iterative penalised least squares approach can be extremely fast and scalable – even much faster than some existing convex formulations. This approach might be useful for sparse sufficient dimension reduction when the response is multivariate and even high-dimensional.
Disclosure statement
No potential conflict of interest was reported by the author(s).
Additional information
Notes on contributors
Xin Zhang
Xin Zhang is an Associate Professor in Statistics at the Florida State University.
References
- Cook, R. D. (1998). Regression graphics: Ideas for studying regressions through graphics (Vol. 318). John Wiley & Sons.
- Li, K.-C., & Duan, N. (1989). Regression analysis under link violation. The Annals of Statistics, 17(3), 1009–1052. https://doi.org/10.1214/aos/1176347254
- Mai, Q., & Zhang, X. (2019). An iterative penalized least squares approach to sparse canonical correlation analysis. Biometrics, 75(3), 734–744. https://doi.org/10.1111/biom.v75.3 doi: 10.1111/biom.13043
- Neykov, M., Lin, Q., & Liu, J. S. (2016). Signed support recovery for single index models in high-dimensions. Annals of Mathematical Sciences and Applications, 1(2), 379–426. https://doi.org/10.4310/AMSA.2016.v1.n2.a5
- Neykov, M., Liu, J. S., & Cai, T. (2016). L1-regularized least squares for support recovery of high dimensional single index models with gaussian designs. The Journal of Machine Learning Research, 17(1), 2976–3012.
- Ni, L., Cook, R. D., & Tsai, C.-L. (2005). A note on shrinkage sliced inverse regression. Biometrika, 92(1), 242–247. https://doi.org/10.1093/biomet/92.1.242
- Tan, K., Shi, L., & Yu, Z. (2020). Sparse sir: Optimal rates and adaptive estimation. The Annals of Statistics, 48(1), 64–85. https://doi.org/10.1214/18-AOS1791
- Zhou, J., & He, X. (2008). Dimension reduction based on constrained canonical correlation and variable filtering. The Annals of Statistics, 36(4), 1649–1668. https://doi.org/10.1214/07-AOS529