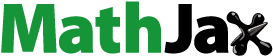
Abstract
To estimate unknown population parameters based on panel data having nonignorable item nonresponse, we propose an innovative data grouping approach according to the number of observed components in the multivariate outcome when the joint distribution of
and associated covariate
is nonparametric and the nonresponse probability conditional on
and
has a parametric form. To deal with the identifiability issue, we utilise a nonresponse instrument
, an auxiliary variable related to
but not related to the nonresponse probability conditional on
and
. We apply a modified generalised method of moments to obtain estimators of the parameters in the nonresponse probability, and a generalised regression estimation to utilise covariate information for efficient estimation of population parameters. Consistency and asymptotic normality of the proposed estimators of the population parameters are established. Simulation and real data results are presented.
1. Introduction
Panel data are collected in many statistical applications, such as sample surveys, clinical trials, economics and social sciences. For example, cluster sampling results in panel data, which occurs in social studies and sample surveys when mutual homogeneity within clusters is evident in the population of interest. Multivariate outcome from a single sampled unit also leads to panel data.
Item nonresponse is a common phenomena in panel data, i.e., some components of the panel, not necessary the entire panel, may be missing. For example, in survey studies, subjects may not respond to all questions; in cluster sampling, some units within a cluster may not respond; in multivariate outcome, some components are measured while the others are not. Estimation and statistical inference without taking nonresponse into consideration could lead to seriously biased estimators and conclusions.
Consider a k-dimensional response or outcome vector of interest that is subject to item nonresponse. Let
be the response indicator vector of
, i.e., the jth component of
is 1 (or 0) if the jth component of
is observed (or not observed),
. Statistical approaches dealing with missing data usually depend on the nonresponse propensity (or mechanism), i.e., the conditional distribution of
given
, denoted by
, where
is a covariate vector associated with
and is always observed. If
, where
is the observed part of
, then nonresponse is ignorable (Little & Rubin, Citation2002; Rubin, Citation1976). Otherwise, nonresponse is nonignorable. While there is a rich literature for valid inference on unknown
(the distribution of
) or
(the conditional distribution of
given
) under ignorable nonresponse (S. X. Chen et al., Citation2008; Little & Rubin, Citation2002; Robins & Rotiv, Citation1997; Rotnitzky & Robins, Citation1997; Rubin, Citation1976), statistical inference faces serious challenges under nonignorable nonresponse when
depends on
as well as some components of
.
We provide a brief review of the progress in research on general nonignorable nonresponse in . Greenlees et al. (Citation1982) proposed to handle nonignorable item nonresponse by maximum likelihood estimation, assuming both
and
are parametric; however, the non-identifiability issue caused by nonignorable nonresponse is not well-addressed and, thus, the result is not rigorous. Besides, a fully parametric approach is sensitive to the parametric model assumptions. Since the population is not identifiable when both
and
are nonparametric (Robins & Rotiv, Citation1997), efforts have been made in situations where one of
and
is parametric or semiparametric. Tang et al. (Citation2003) considered the situation where
is parametric but
is nonparametric, and provided a rigorous treatment of the identifiability issue for the first time; but they assumed that the nonresponse propensity depends only on
, i.e.,
, which may be impractical. This result was extended by Zhao and Shao (Citation2015) to more realistic situation where
and
is a sub-vector of
. While both previously cited papers assumed a parametric
but a unspecified
, parallel results were established by Wang et al. (Citation2014) and J. Shao and L. Wang (Citation2016) under a univariate
(k = 1) with a nonparametric
and a parametric or semi-parametric
, which are particularly useful in sample surveys where it is difficult to find a suitable parametric model for
. Other than the results under a parametric model on
, there is no general result on multivariate
having nonignorable item nonresponse, although Wu and Carroll (Citation1988), Xu and Shao (Citation2009), and Shao and Zhang (Citation2015) obtained some results when the dependence of
on
is through an unobserved random effect
, i.e.,
.
Under nonparametric and
, in this paper we propose an innovative data grouping approach to construct valid estimators of population parameters in the presence of nonignorable item nonresponse in
, assuming the following two main assumptions.
(A1) | Given | ||||
(A2) | Given |
Our main methodology is introduced in Section 2, followed by some simulation results in Section 3 and two real data examples in Section 4. Section 5 contains some technical proofs.
2. Methodology
We use the notation developed in Section 1. Our inference is based on a training sample of size n, ,
, which are independent and identically distributed with
. Values of
are always observed and components of
are observed if and only if the corresponding components of
are equal to 1.
2.1. Grouping
When there is no nonresponse, values in the entire set are exchangeable. But this does not hold in the presence of nonignorable item nonresponse in
. Although
's with the same nonresponse pattern
are exchangeable, there are a total of
different nonresponse patterns when k is the dimension of
. Thus although grouping according to nonresponse patter is natural to achieve within-group homogeneity, each group may not have enough units for efficient estimation or inference.
Our main idea is to divide data into k + 1 groups with within-group homogeneity, using the following key lemma under assumption (A1).
Lemma 2.1.
Let Δ be the number of observed components in . Under (A1),
, i.e., the conditional distribution of
given
is the same as the conditional distribution of
given Δ.
Proof.
Let be the conditional probability of observing a component of
given
. Under (A1),
and Δ follows a binomial distribution with probability π and size k conditioned on
. The result follows from
According to Lemma 2.1, we can partition the whole dataset into k + 1 groups,
,
, where
is the number of observed components in
. Each group
contains exchangeable values and enough units for inference as long as k is much smaller than n.
2.2. Estimation under cluster sampling
We consider the situation where components of have the same distribution (e.g., we have panel data under cluster sampling) and estimation of a parameter in the population of
is our interest. To illustrate, we focus on the estimation of μ, the mean of a component of
. For
and each group with
, the within-group sample mean of observed values is
(1)
(1)
where
and
are the jth components of
and
, respectively, and
is the number of units with
. Each
is an estimator of
. Note that
is not defined.
If is known, then the overall population mean
where
, can be estimated by
(2)
(2)
The proof of the following result is deferred to Section 5.
Theorem 2.1.
Assume (A1) holds and that components of have the same distribution with finite second-order moment Then, as
,
(3)
(3)
where
,
.
Since is usually unknown, however,
is not an estimator and we need to find a way to estimate
. In the group with
, all components of
are missing. Thus some assumption is needed to relate this group with other groups. Under assumption (A2), our idea is to solve this problem using data in the group with
, the group with completely observed
's. From
we obtain the following relationship:
where the second equality follows from (A1)–(A2) and
is defined in (A2), the conditional probability of observing a component of
given
. The ratio
can be estimated by
. If we can obtain an estimator
of θ, then characteristics in
can be estimated using this relationship,
,
, and estimators of characteristics in
with completely observed
.
Thus, can be estimated by
where y is a component of
and
is the empirical distribution based on the data set
.
Once is estimated by
, the overall population mean μ can be estimated by
(4)
(4)
In this way, other population characteristics can be similarly estimated. For example, if we want to estimate the distribution of a component of
at a point t, then we just need to replace
by the indicator of
in the previous discussion. Quantiles of F can then be estimated. Estimators of correlation between two components of
and between
and
can be similarly derived. We can also estimate parameters via estimating equations.
2.3. Estimation of θ in propensity
To complete our proposed methodology, we need to construct an estimator of θ under (A1)–(A2). To estimate θ, we follow the approach of generalised method of moments (GMM) in Wang et al. (Citation2014) for the univariate response, but add a novel modification by utilising the multivariate structure of
.
Define an L-dimensional estimating function
where
is the transpose of a, L is an integer
the dimension of θ and the form of
is specified later. These functions are chosen so that, at the true parameter value θ,
and
is of full rank. Let
If L is the same as the dimension of θ, then we estimate θ by
such that
. If L is larger than the dimension of θ, we apply the two-step GMM (Hall, Citation2005; Hansen, Citation1982) as follows:
Obtain
by minimising
.
Obtain
by minimising
, where
is the inverse of
matrix whose
element is
.
The optimisation can be solved by using the MATLAB function fminsearch, which is applied in our simulation and data analysis in Sections 3 and 4.
It remains to specify the form of . Suppose first that
is discrete and has q categories, say
. A straightforward extension of the approach in Wang et al. (Citation2014) (from univariate response to multivariate
) is using
(5)
(5)
where
is the jth component of the vector
of response indicators and
is a vector whose first q components are indicators of
and the last p components are the p-dimensional covariate vector
in (A2). With this choice of G,
under (A1)–(A2).
However, there are two problems. First, the partially observed responses in are not used in (Equation5
(5)
(5) ), since
if and only if all components of
are observed. Second, a more serious issue is that L may be smaller than the dimension of θ. For example, if
is continuous and
(6)
(6)
where
, α is univariate, β is k-dimensional, and γ is p-dimensional, then the dimension of θ is p + k + 1 and L = p + q; in this case
requires that q>k. That is, we are not able to apply GMM if z does not have more than k categories.
To overcome this difficulty, we consider the following modification. First, we construct k overlapped subsets of the entire data set, where
contains data from units whose
may be missing but all other components are observed,
. With the notation
the jth component of
,
. Table provides an example of
, where a check mark indicates an observed datum and a question mark indicates a nonresponse.
Table 1. Example of when k = 3 and n = 30.
For each fixed h, we consider
(7)
(7)
where L = p + q + k−1,
is the L-dimensional vector whose first p + q components are the same as those of
in (Equation5
(5)
(5) ), the rest k−1 components are
,
, and
is the hth component of
. To see why the function
in (Equation7
(7)
(7) ) can be used in estimation equation, note that
where the second equality follows from the independence between z and
conditioned on
and the last equality follows from the fact that
under (A1)–(A2).
Note that the key difference between in (Equation5
(5)
(5) ) and
in (Equation7
(7)
(7) ) is that the components of
other than the hth component are used as ‘covariates’ and included in the vector
in (Equation7
(7)
(7) ). In this way, we have more estimating functions and does not need to have the restriction q>k in the case of (Equation6
(6)
(6) ), because
is easily satisfied as long as
.
If we apply the GMM algorithm with in (Equation5
(5)
(5) ) replaced by
in (Equation7
(7)
(7) ), we can obtain a GMM estimator
for every h. Our proposed final GMM estimator of θ is then the weighted average estimator
where
is the number of units in
.
When has continuous components, we can apply the method by discretising
into q categories or use moments of
as components of
.
Under the same regularity conditions assumed in Wang et al. (Citation2014), consistency and asymptotic normality of the estimator can be established and details are omitted. For a point estimator such as
defined in (Equation4
(4)
(4) ), its consistency and asymptotic normality can also be established but its asymptotic variance does not have a simple explicit form such as the one for
given in (Equation2
(2)
(2) ). The complication comes from the estimation of
, the correlation between
and
in (Equation4
(4)
(4) ), and the estimation of θ that produces
correlated with
and all
's in (Equation4
(4)
(4) ).
Thus we do not try to obtain an explicit form of the asymptotic variance of defined by (Equation4
(4)
(4) ). Instead, we recommend the bootstrap method for variance estimation or inference. Since our point estimators are all functions of averages and GMM estimators, the general bootstrap theory ensures that the bootstrap variance estimators are consistent and can be effectively applied to avoid the complicated derivation of asymptotic variances of estimators such as
in (Equation4
(4)
(4) ), at the expense of a large amount of computations. In Section 3, the performance of bootstrap variance estimators is evaluated by simulations.
2.4. Estimation for multivariate outcomes
In Sections 2.2 and 2.3, we consider the situation where components of have the same distribution and the population parameter such as the mean μ of a component of
can be estimated using the observed values from all components within each group under assumption (A1) to compensate the missing components. We now consider a multivariate outcome
whose components have different distributions, and we need to estimate population parameters of the jth component
of
,
. To illustrate, we focus on the estimation of population mean
with a fixed
.
To handle the nonignorable nonresponse under assumption (A1), we still group data according to the value of Δ, the number of observed components in , as described in Section 2.1. However, we cannot make use of observations from different components of
within each group; instead, to estimate
we can only use observed values from the fixed jth component. Assuming that
is known, an analog of
in (Equation2
(2)
(2) ) is
(8)
(8)
where
is the sample mean of observed values of the jth component of
within group
. The number of observations used for
,
, is smaller than the number of observations
used for
in (Equation1
(1)
(1) ). Hence,
in (Equation8
(8)
(8) ) may be not stable when the sample size n is not very large. To overcome this difficulty, we consider making use of the always observed covariate
to improve the estimation efficiency.
If a correct parametric model between and
is imposed, then covariate information can be effectively utilised through the model. Although a linear or parametric relationship between
and
for the whole dataset without nonresponse might be possible, it is unrealistic to expect such relationship still exists between
and
in each group with
. A purely nonparametric regression between
and
in each group may be applied, but a nonparametric method may be inefficient and suffers from the well-known curse of dimensionality.
A popular approach in sample surveys for improving efficiency without relying on any model between and
is the Generalised Regression (GREG) method. The GREG is first discussed in Cassel et al. (Citation1976) and studied extensively in the literature; for example, Sarndal et al. (Citation2003) and J. Shao and S. Wang (Citation2014). Since this approach is model-assisted but not model-based, i.e., a model is used to derive efficient estimators that are still asymptotically valid even if the model is incorrect, it suits our purpose of utilising covariates without modelling within each group.
For each d and j, let be the sample mean of observed values of the jth component of
within group
,
be the sample mean vector of
values corresponding to the observed values used in computing
within group
,
be the sample mean of
values based on all units in group
, and
(9)
(9)
which is a least squares estimator based on observed data from jth component of
and
within group
. Assuming that
is known, our proposed GREG estimator of population mean
is
(10)
(10)
The following theorem summarises the asymptotic behaviour of the proposed GREG estimator
in (Equation10
(10)
(10) ), for each fixed
. Note that no model assumption is imposed on the relationship between
and
.
Theorem 2.2.
Assume (A1) and that, for each , the second-order moments of
and
are finite, where
is the jth component of
. Assume also that, for every
,
, the conditional variance of
given
, is positive definite. Then, as
,
(11)
(11)
where
,
(12)
(12)
is the number of observed
's within group
,
,
,
is the conditional covariance between
and
given
,
, and
and
are defined to be 0. In addition, result (Equation11
(11)
(11) ) holds with
replaced by
in (Equation8
(8)
(8) ) and
in (Equation12
(12)
(12) ) replaced by 0.
As indicated by Theorem 2.2, the GREG estimator is always asymptotically more efficient than
unless
for all
. It can also be seen that
when d = k, the group with all completely observed response vectors. This means that the GREG approach does not help in the group
.
Note that we still need to estimate for each fixed j. But this can be done using the same approach we discussed in Sections 2.2 and 2.3. Also, the final estimator of
(after replacing
in (Equation10
(10)
(10) ) by its estimator) can be shown to be consistency and asymptotically normal under the same regularity conditions assumed in Wang et al. (Citation2014), but its asymptotic variance does not have a simple explicit form such as the one given in Theorem 2.2. Thus we do not try to obtain an explicit form of the asymptotic variance of the GREG estimator of
. Instead, we recommend the bootstrap method for variance estimation, as we discussed in the end of Section 2.3.
3. Simulation results
In this section, simulation results are presented to investigate the finite sample performance of our proposed estimators developed in Section 2. We consider some different settings. In all simulation studies, the proposed GMM estimator is calculated using the MATLAB function fminsearch with initial value
.
3.1. Results for a single covariate 
and 
with identically distributed components
We first present simulation results under situations where k = 3, , components
's are identically distributed, and there is only a single covariate
satisfying (A2), i.e., there is no covariate
. Our interest is to estimate the marginal population mean μ of a component of
, without applying GREG.
For comparison, in addition to the proposed estimator in (Equation4
(4)
(4) ), we also include the naive estimate
, the sample mean of observed
-values, and
, the sample mean when there is no nonresponse, used as a benchmark.
In the first simulation study, z is discrete with q = 2 categories, and
. Conditional on z, k = 3 components of
are independently generated from
. Note that components of
are conditionally independent, but are dependent unconditionally, and have the same distribution with unconditional mean
. Given the generated data, the nonrespondents are generated according to the propensity
(13)
(13)
where
with value
in case I and value
in case II. These values of θ are chosen so that β's have different signs and the unconditional nonresponse probability is approximately between 30% and 40%.
The population in cases III and IV is the same except that z has q = 3 categories with ,
, and
, the unconditional population mean is 41, and
and
in cases III and IV, respectively.
Table reports simulation results for n = 2000 with 1000 simulation runs. The reported quantities are values of estimate, bias in percentage, and standard deviation (SD) for the estimators of μ and parameters in the propensity, based on 1000 simulations. For the estimation of μ, we also calculate the simulation average of the standard error (SE) and coverage probability (CP) of the approximate 95% confidence interval, using the bootstrap variance estimator with bootstrap sample size 100. We do not compute SE and CP for estimators of α and β's as parameters in propensity are not the main parameters of interest.
Table 2. Simulation results for a single discrete covariate and
with identically distributed components (n = 2000 with 1000 simulations).
The results in Table show that the GMM estimator and
in (Equation4
(4)
(4) ) work well for all cases, in terms of estimation bias, SD, and CP. In addition, the bootstrap SE performs well. The naive estimator
has a serious positive bias when β's are negative (larger y has smaller nonresponse probability) and has a negative bias when β's are positive (larger y has larger nonresponse probability). Although
may have a small SD, its bias have a serious effect on inference as its related CP is far from the nominal level 95%.
We next turn to a continuous and compare different ways to use z in estimation equations in (Equation7
(7)
(7) ). Conditional on z, components of
are independent and identically distributed as
, which gives the unconditional mean
. Given the generated data, the nonrespondents are generated according to (Equation13
(13)
(13) ) with
. For the continuous z, we consider three ways of using z in the GMM estimation of θ. In case V, z is discretised into q = 2 categories according to the median of z. In case VI, z is discretised into q = 3 categories according to the 33% and 66% percentiles of z. In case VII, we use a moment of z, i.e., the vector
in (Equation7
(7)
(7) ) has its first two components as
. Results for n = 2000 with 1000 simulation runs are given in Table , with the same quantities in Table .
Table 3. Simulation results for a single continuous covariate and
with identically distributed components (n = 2000 with 1000 simulations).
From the results in Table , we can see that cutting z into three categories results in a smaller SD compared with that for discretising z into two categories. Using for
with a continuous z results in the most efficient estimators of μ among the three ways of using z in (Equation7
(7)
(7) ).
3.2. Results for 
and 
with identically distributed components
We now add a covariate u into the cases in Section 3.1 and consider with a univariate continuous u and a categorical z. We consider four cases. In cases VIII–IX, z is a discrete covariate having q = 2 categories,
, and
. Given z,
. Given z = 1 and u, components of
are independent and identically distributed as
; given z = 2 and u, components of
are independent and identically distributed as
. The unconditional mean μ is 24. The propensity is
(14)
(14)
where
in case VIII and
in case IX. These values are chosen so that γ has different signs and the unconditional nonresponse probability is approximately between 30% and 40%.
In cases X–XI, z has q = 3 categories, ,
and
. Given z,
. Given z = 1 and u, components of
are independent and identically distributed as
; given z = 2 and u, components of
are independent and identically distributed as
; given z = 3 and u, components of
are independent and identically distributed as
. The unconditional mean μ is 32.5. The propensity is given by (Equation14
(14)
(14) ) with
in case X and
in case XI.
Results for n = 2000 with 1000 simulation runs are given in Table . Conclusions for results in Table are similar to those in Tables and .
Table 4. Simulation results for with a categorical z and a continuous u (n = 2000 with 1000 simulations).
3.3. Results for a multivariate outcome 

In this section, we present simulation results under situations where k = 3, components of have different distributions, and our interest is to estimate each marginal population mean
,
. We consider the proposed GREG estimator
as well as the estimator
without applying GREG,
. The naive estimator
, the sample mean of observed values of
, and
, the sample mean of
when there is no nonresponse, are also included.
We consider with independent u and z, where u is continuous and distributed as
. In cases XII–XIII, z is continuous and distributed as
and given z and u,
,
,
and
's are independent. The unconditional mean vector
is
. In cases XIV–XV, z is discrete with q = 3 categories,
,
and
; given z and u,
,
,
, and
's are independent. The unconditional mean μ is
. The propensity is given by (Equation14
(14)
(14) ) with
in cases XII and XIV and
in cases XIII and XV. These values are chosen so that γ has different signs and the unconditional nonresponse probability is approximately between 30% and 40%.
Results for n = 2000 with 1000 simulation runs are given in Table . The results show that both proposed estimators and
perform well for each component of
under all cases with coverage probabilities close to the nominal level 0.95. They are much better compared with the naive biased estimator
. Also, the estimator
with GREG has a respectable improvement in standard deviation compared with
without GREG.
Table 5. Simulation results for and multivariate
(n = 2000 with 1000 simulations); SDimp(%)
SD of
SD of
.
4. Real data examples
We apply our proposed estimators to two real data sets from the National Longitudinal Survey of Mature and Young Women (NLSW) and the National Health and Nutrition Examination Survey (NHANES). The proposed estimation approach introduced in Section 2.2 is applied on the NLSW survey data since components of the outcome we choose from the dataset can be treated as from the same distribution. The proposed estimation method introduced in Section 2.4 with or without the GREG is applied on the NHANES data since the outcome we choose from the dataset is multivariate.
We present the estimated values and standard error (SE) under bootstrap method of the marginal means as well as the estimated values of the parameters in the nonresponse propensity. Our results and conclusions are based on assumptions (A1)–(A2) which, unfortunately, cannot be checked using available data. The assumption that components of are conditionally independent and identically distributed given
seems reasonable from the specific problems under investigation.
4.1. Application to NLSW data
The NLSW started in the mid-1960s because the U.S. Department of Labor was interested in studying the employment patterns of non-institutionalised civilian women in the United States. We focus on the survey of mature women cohort with ages from 30s to early 40s. A detailed description of this survey can be found at https://www.bls.gov/nls/original-cohorts/mature-and-young-women.htm.
Among many topics, we focus on the variable of women's weight in pounds (ERNYR-P) from heath topic as our example. More specifically, we consider the outcome
, where
's are weights (in lbs) of respondent in 1997, 1999 and 2001, respectively. The outcome values are self-reported in roughly every 2 years. Since the participants are matured women, the three components of
have almost the same distribution. We are interested in estimating the overall population mean μ of the weight using the proposed method in Section 2.2. We use the age of participant when she joined the NLSW survey as the nonresponse instrument z.
In the dataset, each of three components of has about 29% nonresponse probability while the covariate has no nonresponse. The number of observed values in each nonresponse pattern for the outcome
is shown in Table .
Table 6. The number of observed values in each nonresponse pattern
We computed the proposed estimator in Sections 2.2 and 2.3. Since the covariate
‘age of respondent when joining the survey’ is univariate and continuous, we treat
and use the moments of z directly in the GMM algorithm. The results are given in Table and the SE is computed as the squared root of the bootstrap variance estimate with bootstrap size 100.
Table 7. Estimation based on NLSW data.
For comparison, we include the naive estimator , the sample mean of observed
values. We can see that our proposed estimator
has a significant difference from the naive estimate
.
4.2. Application to NHANES data
The NHANES is a major program of the National Center for Health Statistics, which is a part of the Centers for Disease Control and Prevention responsible for producing vital and health statistics for the United States. The NHANES is a program designed to assess the health and nutritional status of adults and children in the non-institutionalised civilian resident population of the United States. A description of this survey can be found at https://www.cdc.gov/nchs/nhanes/about_nhanes.htm.
The NHANES program began in the early 1960s and had been conducted as a series of surveys focusing on different population groups or health topics. In 1999, the survey became a continuous program that has a changing focus on a variety of health and nutrition measurements to meet emerging needs. The survey is unique in that it combines interviews and physical examinations. The home-interview part collects answers from demographic, socioeconomic, dietary, and health-related questions. The examination component conducted in a mobile examination centre consists of medical, dental, and physiological measurements, as well as laboratory tests administered by highly trained medical personnel.
The data set we focused on is for 2013–2014 consisting of 9100 persons who completed both interview and examination. We consider a multivariate outcome with k = 3 and two demographic covariates from the dataset. The three components of
are the total cholesterol (mg/dL), ‘LBXSCH’, the first reading of systolic blood pressure (mm Hg), ‘BPXSY1’, and the average sagittal abdominal diameter (cm), ‘BMDAVSAD’. The two covariates are the age in years of the household reference person, ‘DMDHRAGE’, and the total household income (reported as a range value in dollars), ‘INDHHIN2’.
Each of the three components of has about 28% missing values while two covariates have no missing value. The number of observed values in each of nonresponse pattern for
is shown in Table .
Table 8. The number of observed values in each of nonresponse pattern.
In this example, the three components of have different distributions and we are interested in estimating the population mean for each
. Therefore, we apply our proposed estimator in Section 2.4 with GREG, denoted by
, and the estimator without generalised regression, denoted by
. For comparison, we also include the naive estimate
, the sample mean of observed
values.
Since is two dimensional, we try two scenarios,
DMDHRAGE and
INDHHIN2 in case 1, and
INDHHIN2 and
DMDHRAGE in case 2. The propensity model we used is given by (Equation14
(14)
(14) ).
The results for two cases are given in Table , where SE is computed as the squared root of the bootstrap variance estimate with bootstrap size 100.
Table 9. Estimation based on NHANES data.
From both cases, we can see that estimators and
are very similar but are significantly different from the naive estimator
, indicating that the naive estimator is biased according to our theory and empirical results. The fact that different ways of defining z in (A2) result in very similar estimates of
's indicates that both covariates DMDHRAGE and INDHHIN2 are suitable to be used as z in (A2), although different z's produce different estimates of parameters in propensity. In this example, covariates may not help very much in estimating the marginal population means, although they are very helpful in handling nonignorable nonresponse.
5. Technical proofs
Proof of Theorem 2.1
The asymptotic normality result (Equation3(3)
(3) ) follows from the Central Limit Theorem. Hence, it remains to show that the asymptotic mean and variance are of the given form. Let
. From conditioning,
so that the mean of
is 0. To derive the asymptotic variance, we calculate
where the last equality follows from the fact that the vector
follows a multinomial distribution so that
and
for any
. Then, the result follows from
Lemma 5.1.
Under the conditions of Theorem 2.2, for each and each
,
in probability as
, where
is defined in (Equation9
(9)
(9) ) and
.
Proof of Lemma 5.1
For fixed j and d, by (A1), the weak law of large numbers for independent random variables, and Lemma 2.1 in Section 2.1, as ,
in probability. Therefore, as
,
Similarly, it can be shown that
The proof is completed by combining the results and using the definitions of
and
.
Proof of Theorem 2.2
From (Equation10(10)
(10) ),
where
By Lemma 5.1,
is asymptotically negligible compared with
and
. Hence, to prove (Equation11
(11)
(11) ), it suffices to show that
converges in distribution to the limiting normal distribution in (Equation11
(11)
(11) ). Consider
first. Note that
Then
From the Central Limit Theorem,
We now turn to
. Let
. Conditioned on
,
where the last equality follows from
and
as given
,
and
values in group
are exchangeable. It follows from the Central Limit Theorem that, conditioned on
,
Then, unconditionally,
To complete the proof, it remains to show two items. One is
given in (Equation12
(12)
(12) ); the other is that
. The latter follows from
where the second equality follows from the fact that
is a function of
so that
almost surely. To calculate
, note that, for any fixed d,
where
Since observations in
and
are not overlapped, conditioned on
,
This shows the desired result and completes the proof.
Acknowledgments
We would like to thank the two referees for comments. The authors' research was partially supported by the National Natural Science Foundation of China grant 11831008 and the U.S. National Science Foundation grant DMS-1914411.
Disclosure statement
No potential conflict of interest was reported by the author(s).
Additional information
Funding
Notes on contributors
Sijing Li
Dr. Sijing Li holds a Ph.D. in statistics from University of Wisconsin-Madison. She is now a statistician at Roche in Shanghai, China. Her research interest is in missing data.
Jun Shao
Dr. Jun Shao holds a PhD in statistics from the University of Wisconsin-Madison. He is a Professor of Statistics at the University of Wisconsin-Madison. His research interests include variable selection and inference with high dimensional data, sample surveys, and missing data problems.
References
- Cassel, C. M., Sarndal, C. E., & Wretman, J. H. (1976). Some results on generalized difference estimation and generalized regression estimation for finite populations. Biometrika, 63, 615–620. https://doi.org/https://doi.org/10.1093/biomet/63.3.615
- Chen, S. X., Leung, D. H., & Qin, J. (2008). Improving semiparametric estimation by using surrogate data. Journal of the Royal Statistical Society: Series B (Statistical Methodology), 70, 803–823. https://doi.org/https://doi.org/10.1111/rssb.2008.70.issue-4
- Greenlees, J. S., Reece, W. S., & Zieschang, K. D. (1982). Imputation of missing values when the probability of response depends on the variable being imputed. Journal of the American Statistical Association, 77, 251–261. https://doi.org/https://doi.org/10.1080/01621459.1982.10477793
- Hall, A. R. (2005). Generalized method of moments. Oxford University Press.
- Hansen, L. (1982). Large sample properties of generalized method of moments estimators. Econometrica, 50, 1029–1054. https://doi.org/https://doi.org/10.2307/1912775
- Little, R. J. A., & Rubin, D. B. (2002). Statistical analysis with missing data ( 2nd ed.). Wiley.
- Robins, J. M., & Rotiv, Y. (1997). Toward a curse of dimensionality appropriate (CODA) asymptotic theory for semiparametric models. Statistics in Medicine, 16, 285–319. https://doi.org/https://doi.org/10.1002/(ISSN)1097-0258
- Rotnitzky, A., & Robins, J. M. (1997). Analysis of semiparametric regression models with nonignorable nonresponse. Statistics in Medicine, 16, 81–102. https://doi.org/https://doi.org/10.1002/(ISSN)1097-0258
- Rubin, D. B. (1976). Inference and missing data. Biometrika, 63, 581–592. https://doi.org/https://doi.org/10.1093/biomet/63.3.581
- Sarndal, C. E., Swensson, B., & Wretman, J. (2003). Model assisted survey sampling. Springer-Verlag.
- Shao, J., & Wang, L. (2016). Semiparametric inverse propensity weighting for nonignorable missing data. Biometrika, 103, 175–187. https://doi.org/https://doi.org/10.1093/biomet/asv071
- Shao, J., & Wang, S. (2014). Efficiency of model-assisted regression estimators in sample surveys. Statistica Sinica, 24, 395–414.
- Shao, J., & Zhang, J. (2015). A transformation approach in linear mixed-effect models with informative missing responses. Biometrika, 102, 107–119. https://doi.org/https://doi.org/10.1093/biomet/asu069
- Tang, G., Little, R. J. A., & Raghunathan, T. E. (2003). Analysis of multivariate missing data with nonignorable nonresponse. Biometrika, 90, 747–764. https://doi.org/https://doi.org/10.1093/biomet/90.4.747
- Wang, S., Shao, J., & Kim, J. K. (2014). An instrumental variable approach for identification and estimation with nonignorable nonresponse. Statistica Sinica, 24, 1097–1116.
- Wu, M. C., & Carroll, R. J. (1988). Estimation and comparison of changes in the presence of informative right censoring by modeling the censoring process. Biometrics, 44, 175–188. https://doi.org/https://doi.org/10.2307/2531905
- Xu, L., & Shao, J. (2009). Estimation in longitudinal or panel data models with random-effect-based missing responses. Biometrics, 65, 1175–1183. https://doi.org/https://doi.org/10.1111/j.1541-0420.2009.01195.x
- Zhao, J., & Shao, J. (2015). Semiparametric pseudo likelihoods in generalized linear models with nonignorable missing data. Journal of the American Statistical Association, 110, 1577–1590. https://doi.org/https://doi.org/10.1080/01621459.2014.983234