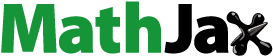
I would like to take this opportunity to congratulate Zhengjun for his continuing contribution to extreme-value statistics in recent years. In this review paper, some fundamental theories on univariate extremes and multivariate extremes are introduced, and recent developments on extremes from some structured stochastic processes are also given. The results in the latter sections of the paper are largely due to Zhengjun and his coauthors. The paper provides some insights for future challenges on extremes and can help young researchers follow the contemporary research topics.
Below I offer some comments on univariate extreme-value statistics. Although the theory for univariate extremes is quite complete, the statistical methods such as the estimation and inference procedures are far from perfect. Set and
in the definition (2.6) in the paper and write
where
is the tail index of the generalised extreme-value distribution H. Various estimates on ξ have been proposed in the literature, and under certain sense each of them may have its merit over other estimates. In general statistics, the method of maximum likelihood estimation is a widely accepted method because the maximum likelihood estimates (MLEs) are asymptotically efficient. It seems quite different in the estimation for extremes. In addition to the references cited in the review paper, I would like to offer the following three papers related to the maximum likelihood method for the tail index:
Hall, P. (1982). On estimating the endpoint of a distribution. Ann. Statist. 10, 556–568.
Peng, L. and Qi, Y. (2009). Maximum likelihood estimation of extreme value index for irregular cases. J. Statist. Plann. Inference 139, 3361–3376.
Wang, F., Peng, L., Qi, Y. and Xu, M. (2019). Maximum penalised likelihood estimation for the endpoint and exponent of a distribution. Statist. Sin. 29, 203–224.
In the first two papers, the MLEs for the tail index and endpoint of an underlying distribution F in the maximum domain of attraction of the generalised extreme-value distribution H with are discussed. Usually, only the cases with
are considered because the likelihood function is irregular when
. The method fails when
since the MLEs do not exist. In the range
, the likelihood score functions can be very messy in the sense that there may be more than one solution to ξ or no solution at all. In the paper by Wang et al. (2019), a maximum penalised likelihood (MPL) method is proposed for the entire range
, and a unique solution to ξ can be obtained from the method with probability one. Above all, the MPL estimate for ξ achieves the smallest asymptotic variance among those from commonly used methods in the literature. It might be possible to extend the MPL method to the range
.