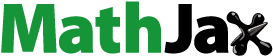
Abstract
In the era of big data, divide-and-conquer, parallel, and distributed inference methods have become increasingly popular. How to effectively use the calibration information from each machine in parallel computation has become a challenging task for statisticians and computer scientists. Many newly developed methods have roots in traditional statistical approaches that make use of calibration information. In this paper, we first review some classical statistical methods for using calibration information, including simple meta-analysis methods, parametric likelihood, empirical likelihood, and the generalized method of moments. We further investigate how these methods incorporate summarized or auxiliary information from previous studies, related studies, or populations. We find that the methods based on summarized data usually have little or nearly no efficiency loss compared with the corresponding methods based on all-individual data. Finally, we review some recently developed big data analysis methods including communication-efficient distributed approaches, renewal estimation, and incremental inference as examples of the latest developments in methods using calibration information.
1. Introduction
Statistical inference with big data can be extremely challenging owing to the high volume and large variety of observed quantities. Currently, one of the most popular approaches to this problem in statistics and computer science is the divide-and-conquer paradigm. The basic idea of this method is to break down a problem recursively into two or more sub-problems of the same or related type, such that each sub-problem becomes simple enough to be solved easily. The solution to the original problem is the optimal combination of the solutions to the sub-problems. A closely related statistical method is called parallel and distributed inference. In essence, large amounts of observed data are stored in different machines in a distributed manner. The computation is often relatively inexpensive in each machine. Then, communication is essential to enable assembly of the available results from all machines. Many related references can be found in, for example, Jordan et al. (Citation2019). Although many new statistical methods have been developed for big data analysis, most of them have roots in traditional statistical methods of combining auxiliary information.
Combining information from similar studies has been and will continue to be an extremely important strategy in statistical inference. The most popular example of such methods is meta-analysis, in which the published results of multiple similar scientific studies are pooled to produce an enhanced estimate without using the raw individual data from each study. We refer to Borenstein et al. (Citation2009) for a comprehensive introduction to meta-analysis. For various reasons such as privacy or capacity of computer storage, in massive data inference, only summarized data rather than the original individual data may be available. This poses a very challenging problem: how to conduct efficient updated inference by making full use of the summarized data? In recent years, many methods of combining information have been developed in economic studies, machine learning, and distributed statistical inference. The goal of this paper is to selectively review a few popular methods that are able to integrate information in different disciplines.
Utilizing external summary data or auxiliary information to obtain more accurate inference is an old and effective method in survey sampling. Owing to restrictions such as cost effectiveness or convenience, the variable of interest Y may be available for only a small portion of individuals. However, the explanatory variable X associated with Y may readily be available for all individuals. Cochran (Citation1977) presented a comprehensive discussion on regression-type estimators making use of the summarized information from X. Chen and Qin (Citation1993), Chen et al. (Citation2002), and Wu and Sitter (Citation2001) used empirical likelihood (EL; Owen, Citation1988) to incorporate such information in finite populations.
With advances in technology, many summarized statistical results have become available in public domains. For example, many aggregated demographic and socioeconomic status data are provided in the US census reports. The Surveillance, Epidemiology, and End Results (SEER) programme of the National Cancer Institute provides population-based cancer survival statistics such as covariate-specific survival probabilities. Imbens and Lancaster (Citation1994) combined micro and macro data in economic studies through the generalized method of moments (GMM). Chaudhuri et al. (Citation2008) showed that inclusion of population-level information could reduce bias and increase the efficiency of the parameter estimates in a generalized linear model setup. Wu and Thompson (Citation2020) published an excellent monograph on combining auxiliary information in survey sampling.
In this paper, we consider two situations. In the first, the summarized information from different studies was derived using the same statistical model. Second, the summarized information was derived using statistical models that were similar but not exactly the same. In general, combining information in the former case is easier. The latter case is more complex, as one has to take into consideration the heterogeneity among different studies.
The rest of this paper is organized as follows. In Section 2, we briefly review two simple and popular meta-analysis methods for combining similar results. In Section 3, we review Owen's (Citation1988) EL method and Qin and Lawless's (Citation1994) over-identified parameter problem as examples of general tools for synthesizing information from summarized data. In particular, we present a new way of deriving the lower information bound for the over-identified parameter problem. Section 4 discusses enhanced inference by utilizing auxiliary information. Section 5 presents results on more flexible meta-analyses where information on different covariates are available in similar studies. Calibration of information from previous studies is described in Section 6. We discuss methods of using disease prevalence information for more efficient estimation in case–control studies in Section 7. The popular communication-efficient distributed statistical inference method used in machine learning is discussed in Section 8. Renewal estimation and incremental inference are briefly presented in Section 9. Finally, some further discussion is presented in Section 10.
2. Two simple information-combining methods
2.1. Convex combination
Suppose that and
are two asymptotically unbiased estimators for θ from two independent studies, and that they satisfy
. The most straightforward way of combining
and
is a convex combination,
The asymptotic variance of
is
which takes its minimum at
. This suggests combining
and
by
an inverse-variance weighting estimator. In general,
and
are unknown; we may replace them by their estimators
and
, respectively, which leads to
As an alternative method, we may use the maximum likelihood method to argue that this is the best estimator. We can treat
as a direct observation from
, i = 1, 2. Then, the log-likelihood (regarding
and
as known constants) is
Maximizing this likelihood with respect to θ or setting the score function to be zero, we end up with the same inverse-variance weighting estimator.
2.2. Random-effect meta-analysis
Dersimonian and Laird (Citation1986) proposed a moment-based estimation method using a random-effect model for meta-analysis. Let be an estimator of
from the i-th study,
. For example,
could be the estimated mean response from the i-th study. When the sample size
in the i-th study is reasonably large, we may assume that
where the
s are treated as known. Although the normal models hold to be true approximately, we assume that they are all true for ease of theoretical development. The goal here is to better estimate θ by combining the results from all the studies.
Unconditionally, we have Consider the following inverse-variance weighting estimator for θ:
with variance
Define
We can easily check that
which implies that a natural estimator of
is
For small sample sizes, there is no guarantee that this estimator is non-negative; one may replace it by
Alternatively, we may estimate τ using the likelihood approach. The joint likelihood based on the s is
Maximizing ℓ with respect to θ and
gives their maximum likelihood estimators (MLEs).
Lin and Zeng (Citation2010) compared the relative efficiency of using summary statistics versus individual-level data in meta-analysis. They found that in general there was no information loss when using the summarized information compared with inference based on the original individual data when available.
3. Empirical likelihood and general estimating equations
In this section we briefly review Owen's (Citation1988) EL and Qin and Lawless' (Citation1994) estimating equations approaches, as those methods represent general tools for assembly of information from different sources. The maximum likelihood method for regular parametric models is among the most popular methods in statistical inference, as it has many nice properties. However, model mis-specification is a major concern, as a mis-specified model may lead to biased results. For the case when the underlying distribution is multinomial, Hartely and Rao (Citation1968) proposed a mean constrained estimator for the population total in survey sampling problems. To mimic the parametric likelihood but discard parametric model assumptions, Owen (Citation1988) and Owen (Citation1990) proposed the EL method, which is a natural generalization of the multinomial likelihood when the number of categories is equal to the sample size. The EL approach can be thought of as a bootstrap that does not resample, or as a likelihood without parametric assumptions (Owen, Citation2001).
3.1. Definition of empirical likelihood
Suppose that are n independent and identically distributed observations from X, with cumulative distribution F. For convenience, we assume there are no ties, i.e., any two observations are unequal to each other. The techniques developed below can be easily adapted to handle ties. Let
be the jumps of
at the observed data points. The nonparametric likelihood is
It is clear that if any
, then
, and if
, then
, where
. According to the likelihood principle (that parameters with larger likelihoods are preferable), one need only consider the distribution functions
with
and
.
If we maximize the log-likelihood
(1)
(1)
subject to the constraints
(2)
(2)
then we obtain
Therefore, the maximum EL estimator of F is
This is why the empirical distribution is called the nonparametric MLE of
.
Suppose we are interested in constructing a confidence interval for , the mean of X. Since we have discretized F at each of the observed data points, the integral becomes
. Next, we maximize the nonparametric log-likelihood subject to an extra constraint:
(3)
(3)
Maximizing the log-likelihood (Equation1
(1)
(1) ) subject to constraints (Equation2
(2)
(2) ) and (Equation3
(3)
(3) ), the Lagrange multiplier method gives the profile log-likelihood of μ,
(4)
(4)
where λ is the solution to
We can treat
as a parametric likelihood of μ. Based on this likelihood, the maximum EL estimator of μ is
, which is exactly the sample mean. We define the likelihood ratio function as
Under the regularity conditions specified in Owen (Citation1988) and Owen (Citation1990), as n goes to infinity,
converges to the
distribution with p degrees of freedom, where p is the dimension of μ, and
is the true value of μ.
3.2. General estimating equations
The original EL was mainly used to make inference for linear functionals of the underlying population distribution such as the population mean (Owen, Citation1988, Citation1990). Qin and Lawless (Citation1994) applied this method to general estimating models, which greatly broadened its applications. Specifically, suppose the population of interest satisfies a general estimating equation
(5)
(5)
for a
vector-valued function g and some θ, which is a
parameter to be estimated. We assume
as otherwise the true parameter value of θ would be undefined.
For general estimating equations with r>p or over-identified models, Hansen (Citation1982) proposed the celebrated GMM, which has become one of the most popular methods in the econometric community. In essence, the GMM minimizes
with respect to θ, where Σ is the variance matrix of the estimating equation
. If Σ is unknown, we may replace it by the sample variance
where
is an initial and consistent estimate of θ.
Instead of GMM, Qin and Lawless (Citation1994) used the EL to make inferences for parameters defined by a general estimating equation. For discretized satisfying (Equation2
(2)
(2) ), Equation (Equation5
(5)
(5) ) becomes
(6)
(6)
Maximizing the log-likelihood (Equation1
(1)
(1) ) subject to (Equation2
(2)
(2) ) and (Equation6
(6)
(6) ), we have the following profile log-likelihood of θ (up to a constant):
where λ is the Lagrange multiplier determined by
We then estimate θ by the maximizer
, whose limiting distribution is established in the following theorem. Hereafter, we use
to denote the differentiation operator with respect to θ.
Theorem 3.1
Qin & Lawless, Citation1994
Denote and
. Suppose that (1)
is positive definite, (2)
is continuous in a neighbourhood of
, (3)
and
can be bounded by some integrable function
in this neighbourhood, and (4)
is of full rank. Then, as
,
where
means ‘convergence in distribution’ and
(7)
(7)
3.3. Calculation of the information bound
Assuming that the parameter of interest satisfies the general estimating equation , we next consider how well we can estimate θ based on this model, and whether the maximum EL estimator is optimal. To answer these questions, we consider an ideal situation, where the probability function X has a parametric form
, which is known up to θ. We define
implicitly assuming that
Clearly,
is an enlarged parametric model of
as it reduces to
when
. As the parametric form
is unknown in practice, we anticipate that any estimator based on the moment constraints
should have a variance that is no less than that of the MLE derived from the enlarged model. We show that even if the form of
is available, the MLE of θ based on
has the same asymptotic variance as the maximum EL estimator.
With the parametric model h, we can estimate θ by maximizing with respect to
. We denote the resulting MLE by
. We show in Section 3.4 that under some regularity conditions on h (see, e.g., Theorems 14 and 23 of van de Vaart (Citation2000)), as
,
(8)
(8)
where V is defined in (Equation7
(7)
(7) ). In general, the parametric form
is unknown; hence, we expect that the best estimator of θ should have an asymptotic variance at least as large as V. As the maximum EL estimator of θ of Qin and Lawless (Citation1994) has asymptotic variance V, we conclude that it achieves the lower information bound.
Remark 3.1
If is an unbounded function of x for each θ, we may construct a new density
where
with
. Clearly, ψ is bounded. We may go through the same derivations to get the same conclusion.
Remark 3.2
Back and Brown (Citation1992) established a similar result by constructing an exponential family. In particular, they defined where
and
is determined implicitly by the following conditions:
,
,
and
In Back & Brown's approach,
is determined implicitly by the above constraint equation, whereas in our new approach, η is an independent parameter.
3.4. A sketched proof of (8)
The log-likelihood based on the enlarged model is , where
If
satisfies the conditions of Theorem 14 of van de Vaart (Citation2000) on
, then
is consistent with
.
Result (Equation8(8)
(8) ) follows from Theorem 23 of van de Vaart (Citation2000). With tedious algebra, we find that
Under some mild assumptions, such as that
holds for θ in a neighbourhood of
, differentiating both sides with respect to θ leads to
which means
As
is consistent with
, by Theorem 5.23 of van de Vaart (Citation2000), we have
(9)
(9)
This, together with the fact that
as n goes to infinity, implies (Equation8
(8)
(8) ).
3.5. Empirical entropy family
Again we assume that the available information is given by the estimating equation . The enlarged parametric model
satisfies
only if
. Naturally, one may require
to satisfy
It is often too restrictive to assume a known underlying parametric model
in the construction of the enlarged parametric model
. We may replace the cumulative distribution function
by the empirical distribution
. In this situation,
is the solution to
Let . For fixed parameter values
, the jump of H at
is
and the likelihood becomes
In fact, this is equivalent to the EL
, where the
s minimize the Kullback–Leibler divergence (up to a constant) or minus the exponential titling likelihood
subject to the constraints
,
, and
See Susanne (Citation2007) for more details. We call this the empirical entropy family induced by the estimating equation
.
4. Enhancing efficiency using auxiliary information
In this section, we discuss methods of incorporating auxiliary information to enhance estimation efficiency. This aspect was also investigated by Qin (Citation2000). We assume a parametric model for the conditional density function of Y given X and leave the marginal distribution
of X unspecified. We wish to make inferences for β when some auxiliary information is summarized through an estimating equation
For example, if we know the mean μ of Y, then we can construct an estimating equation
We can take
Furthermore, we allow that the response Y may have missing values. Let D be the non-missingness indicator, which takes the value 1 if Y is available, and 0 otherwise. We assume a missing-at-random model
where
depends only on x. We denote the observed data by
(
) and
. The likelihood of
is
We can maximize this likelihood subject to the constraints
As
is not a function of β, the profile hybrid empirical log-likelihood (up to a constant) is
(10)
(10)
where λ is the Lagrange multiplier determined by
(11)
(11)
For the special case where data are missing completely at random, i.e.,
is a constant function of x, Qin (Citation2000) established the following theorem.
Theorem 4.1
Let be the true parameter value, let
be the maximum hybrid EL estimator, i.e., the maximizer of (Equation10
(10)
(10) ), and let
be the corresponding Lagrange multiplier. Denote
,
and
Under some regularity conditions, when n goes to infinity, we have
where
with
(12)
(12)
Remark 4.1
Imbens and Lancaster (Citation1994) studied the same problem using GMM. In particular, they directly combined the conditional score estimating equation and
. Even though the first-order large-sample results are the same, the hybrid EL based approach is more appealing as it respects the parametric conditional likelihood and replaces only the marginal likelihood with the EL. See Qin (Citation2000) for numerical comparisons of results of the two methods.
5. Combining summary information: a more flexible method for meta-analysis
Developing systematic methods for combining published information is one of the main goals of meta-analysis, which has become increasingly popular since little extra cost is needed. The main restriction in meta-analysis is that all studies must include the same variables in their analyses. The only difference allowed is in the sample sizes. Thus, studies must be discarded if they contain different variables from those in other studies.
Summarized information is often available from publications such as census reports and results of national health studies. For reasons including confidentiality, it is typically not possible to gain access to the original data, only the summarized reports. Suppose we are interested in conducting a new study that may contain some new variables of interest that are not available in the summarized information, for example, a genetic study involving newly discovered biomarkers or genes. Below we discuss a more flexible method that could be used to combine published information and individual study data for enhanced inference in such cases. Chatterjee et al. (Citation2016) discussed a related problem on the utilization of auxiliary information. As Han and Lawless (Citation2016) pointed out, however, their methodology and theoretical results had already been developed by Imbens and Lancaster (Citation1994) and Qin (Citation2000) in the absence of selection bias in sampling.
We consider two cases. (I) The sample size for the summarized information is much larger than that of the new study. (II) Sample sizes from the two data sources are comparable. In Case I, we can treat the summarized information as known, i.e., the variation in the summarized data is negligible compared with the variation in the new study. In Case II, we have to take the variation in the summarized information into consideration as it is comparable to the variation in the new study. We focus on Case I in this section and study Case II in Section 6.
5.1. Setup and solution
Suppose that the summarized results were obtained from statistical analyses of response Y and covariate variables X (although the original data are not available), and that the new study includes an extra covariate Z in addition to . We are interested in fitting a parametric model
for the conditional density function of Y given X and Z. Let
be the historic data even though they are unavailable. The published information can be summarized in two ways:
is known; and
is the solution of an estimating equation
where the function
is known up to γ.
Let be observed data from the new study. The basic assumption is that
, and
have the same distribution. To utilize the summarized information, we can define estimating functions
in Scenario (I), and
in Scenario (II). We consider only the situation where
. In other words, the variation in the auxiliary information is negligible.
The EL approach amounts to maximizing subject to the constraint
According to Qin and Lawless (Citation1994), the asymptotic variance of the maximum EL estimator
based on estimating equation g is
where
,
, and
is the true value of β. We denote
Equivalently, the asymptotic variance can be written as
or
where
is Fisher's information matrix.
In the above approach, the estimating equation does not involve the parameter β. However, there are ways to achieve higher efficiency. For example, we define
with
Then,
If we combine the empirical log-likelihood based on the estimating equation
and the log-likelihood
as in the previous section (see Equation (Equation12
(12)
(12) )), then the asymptotic variance of the resulting MLE
is given by
In general, this approach can achieve better efficiency.
5.2. A comparison
Given two pairs of estimation functions, and
, we may wonder combining which pair leads to a better estimator if we directly compare their asymptotic variance formulae. Alternatively, we may enquire whether we should combine all three constraints
together. Write
,
, and
Using results from Qin and Lawless (Citation1994) and
with
, we find that the asymptotic variance of
obtained by combining the three estimating equations and
is
It can be shown that
and
Immediately, we have
which implies that the asymptotic variance in the case where
,
, and
are combined is the same as that in the case where
and
only are combined. This indicates that taking
into account leads to no efficiency gain in the estimation of β.
The method of combining and the parametric likelihood
is better than that of combining
,
, and the parametric likelihood. To see this, recall that the asymptotical variances for the MLEs of β with the two methods are
and
It suffices to show that
, namely,
is non-negative definite.
5.3. Proof of 

For convenience, we assume that . As
and
, it suffices to show that
(13)
(13)
Let
and
denote
and
, respectively. As
and
, it follows that
Multiplying both sides by
from the left and by
from the right, we arrive at
that is, inequality (Equation13
(13)
(13) ) holds, which implies
.
6. Calibration of information from previous studies
We consider calibration of information using parametric likelihood, EL (Owen, Citation1988), and GMM (Hansen, Citation1982). When only summary information from previous studies is available, these three well-known methods can be used to calibrate such summary information and to make inferences about the unknown parameters of interest. We may wonder whether doing so results in efficiency loss compared with inferences based on the pooled data if they were all available. Zeng and Lin (Citation2015) found that parametric-likelihood-based meta-analysis of summarized information retained first-order asymptotic efficiency compared with analysis based on individual data. We show here that EL and GMM also possess this property. This is extremely important, as individual data may involve privacy issues, whereas summarized information does not.
6.1. Efficiency comparison
Suppose that (
) are independent observations from the same population. We consider two scenarios according to the model's assumption about the population.
The conditional probability function (i.e., the probability density/mass function of a continuous/discrete random variable) of Y given X has a parametric form
.
The population satisfies
Here, β is a finite-dimensional unknown parameter, and is its true value. Assume that data are available batch by batch, and that
, where
. For the i-th batch (
) of data:
under assumption (I), the parametric log-likelihood function of β is
under assumption (II), we define an empirical log-likelihood function
where
satisfies
;
under assumption (II), we define the objective function of the GMM method (GMM log-likelihood for short) as
where
and
is the true value of β. In practice,
is generally replaced by a consistent estimator of β in the expression for Ω. Using the true value
of β does not affect the theoretical analysis presented in this section.
Let ,
, or
. Under certain regularity conditions, it can be verified that for
,
(14)
(14)
In Case (a),
In Case (b)
where
In Case (c),
We denote the MLE of β based on the i-th batch of data by
The above approximation implies that
When the K-th batch of individual data are available, we no longer have access to the individual data of the previous K−1 batches but only have summarized information
, where
is the MLE based on the i-th batch of data and
, and we can define an augmented log-likelihood
and the corresponding MLE
For
, using the approximation in (Equation14
(14)
(14) ), we have
where the constant C differs in different equations.
For comparison, based on the pooled data, in Case (a) we define the parametric log-likelihood as
in Case (b) we define the empirical log-likelihood function as
where λ satisfies
; and in Case (c) we define the GMM log-likelihood as
Let the log-likelihood based on the pooled data be
,
, and
in Cases (a), (b), and (c), respectively. Then, it can be shown that
for some constant C. Let
. By comparing
and
, we obtain
and
This indicates that compared with the methods, including parametric likelihood, EL, and GMM, based on all individual data, the calibration method based on the last batch of individual data and all summary results of the previous batches has no efficiency loss.
6.2. When nuisance parameters are present
For batch i, assume that the data (
) satisfy either
or
where β is common but
is a batch-specific parameter. We define
in the same way as
. Let
be the MLE of
based on the i-th batch of data, and assume that approximately
with
.
We have two ways of combining information from previous studies. If we use all the previous summary information, we can define
(15)
(15)
As
where
using only this summary information, we can define
Below we show that the MLEs of β based on these two likelihoods are actually equal to each other. In other words, there is no efficiency loss when estimating β based on
instead of
.
To see this, it suffices to show that
(16)
(16)
We denote the inverse matrix of
by
where
It can be seen that
Setting
(
) gives
Putting this back into
gives
where we used the definition of
in the last equation. We arrive at Equation (Equation16
(16)
(16) ) after comparing this with the definition of
.
7. Using covariate-specific disease prevalent information
As discussed in the previous section, summarized statistics from previous studies can sometimes be utilized to enhance the estimation efficiency in a current study. This is especially important in the big data era, when many types of information can be found through the internet. More specifically, suppose the prevalence of a disease is known at various levels of a known risk factor X. In this section, we combine this type of information in a case–control biased sampling setup.
7.1. Induced estimating equations under case–control sampling
Case–control sampling is among the most popular methods in cancer epidemiological studies. This is mainly because it is the most convenient, economic, and effective method. In the study of rare diseases in particular, one has to collect large samples in order to get a reasonable number of cases by using prospective sampling, which may not be practical. Using case–control sampling, a pre-specified number of cases () and controls (
) are collected retrospectively from case and control populations, respectively. Typically, this can be accomplished by sampling cases from hospitals and controls from the general disease-free population.
For a given risk factor X, let for i = 0, 1. Given X in a range
, the disease prevalence is
where
is known. Using Bayes' formula, we have
with
It follows that
or
where
and
denote the expectation operators with respect to
and
, respectively.
We assume that given covariates X and Y, the underlying disease model is given by the conventional logistic regression
(17)
(17)
Let
with
. It can be shown (see Qin, Citation2017) that this is equivalent to the exponential tilting model
where
. As a consequence,
or
(18)
(18)
We denote
and the summarized auxiliary information equations as
with
. Then
, where
7.2. Empirical likelihood approach
The log-likelihood is
(19)
(19)
where
, and the constraints are
The profile log-likelihood is
where the Lagrange multiplier λ is determined by
Finally, the underlying parameters can be obtained by maximizing ℓ.
If the overall disease prevalence probability is known, then
is known. On the other hand, if it is unknown but
, then π is identifiable. If I>1, then we have an over-identified equation problem. This can be treated as a generalization of the EL method for estimating functions (Qin & Lawless, Citation1994) for biased sampling problems. Qin et al. (Citation2015) considered the case where η is unknown and
.
Let and let
be its maximum EL estimator. As the first estimating function
corrects biased sampling in a case–control study, the remaining estimating functions
are used for improving efficiency. When n goes to infinity, it can be shown that the limit of λ is a
-dimensional vector where the first component is
and the remainder are all zero. Qin et al. (Citation2015) showed that if
remains constant as
and
, then under suitable regularity conditions
is asymptotically normally distributed with mean zero. Moreover, the estimation of the logistic regression parameters
improves as the number I of estimating functions increases. This means that a richer set of auxiliary information leads to better estimators. In practice, however, this consideration must be balanced with the numerical difficulty of solving a larger number of equations.
Notably, auxiliary information is informative for estimating β and ξ but not for estimating γ. This can be observed through the following equations:
As the underlying distribution
is unspecified, we can treat
as a new underlying distribution
. With
profiled out, the auxiliary information equation does not involve γ if
. Hence, even if
, the information for γ is minimal as γ and ξ cannot be separated.
7.3. Generalizations
The simulation results of Qin et al. (Citation2015) indicate that when covariate-specific auxiliary information is employed, the estimator of the coefficient β of X has the maximum variance reduction, whereas the variance reductions for other coefficients are small. If the auxiliary information
is also available, we can combine them through estimating equations
It would be more informative if the auxiliary information
is available.
7.4. More on the use of auxiliary information
Under a logistic regression model, the case and control densities are linked by the exponential tilting model
(20)
(20)
Suppose that for the general population
,
, and
are all known, and
is known or can be estimated using external data. Under the exponential tilting model (Equation20
(20)
(20) ), the density
in the general population and the density
in the control population are linked by
As a consequence
where
is an expectation with respect to
. Let
with known
,
, and
. The log-likelihood under case–control data is still (Equation19
(19)
(19) ), where the
s satisfy the following constraints:
More generally, any information in the general population such as
can be converted to an equation for the control population,
Therefore, the results developed by Qin et al. (Citation2015) can be applied. The results of Chatterjee et al. (Citation2016) for case–control data can be considered as a special case of Qin et al. (Citation2015).
8. Communication-efficient distributed inference
In the era of big data, it is commonplace for data analyses to run on hundreds or thousands of machines, with the data distributed across those machines and no longer available in a single central location. Recently, parallel and distributed inference has become popular in the statistical literature in both frequentist and Bayesian settings. In essence, the data-parallel procedures are intended to break the overall dataset into subsets that are processed independently. To the extent that communication-avoiding procedures have been discussed explicitly, the focus has been on one-shot or embarrassingly parallel approaches that use only one round of communication in which estimators or posterior samples are first obtained in parallel on local machines, then communicated to a centre node, and finally combined to form a global estimator or approximation to the posterior distribution (Lee et al., Citation2017; Neiswanger et al., Citation2015; Wang & Dunson, Citation2015; Zhang et al., Citation2013). In the frequentist setting, most one-shot approaches rely on averaging (Zhang et al., Citation2013), where the global estimator is the average of the local estimators. Lee et al. (Citation2017) extend this idea to high-dimensional sparse linear regression by combining local debiased Lasso estimates (van de Geer et al., Citation2014). Recent work by Duchi et al. (Citation2015) shows that under certain conditions, these averaging estimators can attain the information-theoretic complexity lower bound for linear regression, and at least bits must be communicated in order to attain the minimax rate of parameter estimation, where d is the dimension of the parameter and k is the number of machines. This result holds even in the sparse setting (Braverman et al., Citation2016).
The method of Jordan et al. (Citation2019) proceeds as follows. Suppose the big data consists of N observations and there are k machines. For the convenience of presentation, we assume that each machine has n observations, i.e., N = nk. Denote the full-data likelihood by
where
is the log-likelihood based on the data from the j-th machine. For θ near its target value
,
where
and
are remainders. Observing that
, define a surrogate log-likelihood
Ignoring the constant terms, the surrogate log-likelihood is
The score equation based on the surrogate likelihood is
Let
be the solution. Expanding it at
and using the fact that
we can easily show that if
then
If we let
be the MLE based on
, the surrogate log-likelihood can be simplified to
because
.
If the dimension of θ is high, one may add a penalty function in the surrogate log-likelihood and estimate θ by where
is the
-norm of θ. Similarly, Bayesian inference can be adapted to the surrogate likelihood as well.
Duan et al. (Citation2020) proposed distributed algorithms that account for heterogeneous distributions by allowing site-specific nuisance parameters. The proposed methods extend the surrogate likelihood approach (Jordan et al., Citation2019; Wang et al., Citation2017) to the heterogeneous setting by applying a novel density ratio tilting method to the efficient score function. Asymptotically, the approach described in Section 6.2 on nuisance parameters is equivalent to that of Duan et al. (Citation2020).
9. Renewal estimation and incremental inference
Let be a score function of β based on some objective function
from the first batch of data, where M can be either the log-likelihood
or a pseudo log-likelihood.
Let be the solution to
when only the first batch of data
is available. Let
denote the second batch of data. If both of them are available, we let
be the solution to the pooled score equation,
Clearly,
is the most efficient estimator of β when
and
are both available.
If is available but
is not, with only some summary information
and
in its place, how can we utilize the summary information efficiently? It is not feasible to estimate β by directly solving
which involves the individual data of the unavailable
. Luo (Citation2020) considers expanding
at
, i.e.,
for β close to
. As
, it follows that
Luo (Citation2020) proposes obtaining an updated estimator of β by solving
(21)
(21)
Alternatively, we may understand this renewal estimation strategy in the manner of Zhang et al. (Citation2020), who propose estimating β by maximizing
(22)
(22)
where
is the Fisher information. If both batches are available, the score for β is
After recording
and Σ, we no longer have the raw data
. As
differentiating (Equation22
(22)
(22) ) with respect to β gives
Here, we have assumed that
. This indicates that estimating β by maximizing (Equation22
(22)
(22) ) results in no efficiency loss asymptotically compared with the MLE based on all individual data, where the latter is infeasible in the current situation.
10. Concluding remarks
Rapid growth in hardware technology has made data collection much easier and more effective. In many applications, data often arrive in streams and chunks, which leads to batch-by-batch data or streaming data. For example, web sites served by widely distributed web servers may need to coordinate many distributed clickstream analyses, e.g., to track heavily accessed web pages as part of their real-time performance monitoring. Other examples include financial applications, network monitoring, security, telecommunications data management, manufacturing, and sensor networks (Babcock et al., Citation2002; Nguyen et al., Citation2021). The continuous arrival of such data in multiple, rapid, time-varying, possibly unpredictable and unbounded streams not only yields many fundamentally new research problems but provides contains various forms of auxiliary information.
Assembling information from different data sources has become indispensable in big data and artificial intelligence research. Statistical tools play an essential part in updating information. In this paper, we have presented a selective review of several traditional statistical methods, including meta-analysis, calibration information methods in survey sampling, and EL together with over-identified estimating equations and GMM. We have also briefly reviewed some recently developed statistical methods, including communication-efficient distributed statistical inference and renewal estimation and incremental inference, which can be regarded as the latest developments of calibration information methods in the era of big data. Although these methods were developed in different fields and in different statistical frameworks, in principle, they are asymptotically equivalent to well-known methods developed for meta-analysis. These methods result in almost no or little information loss compared with the case when full data are available.
Finally, we apologize to people whose work has inadvertently have been left out of our reference list.
Acknowledgments
The authors thank the editor and two referees for constructive comments and suggestions that led to significant improvements in this paper.
Correction Statement
This article has been republished with minor changes. These changes do not impact the academic content of the article.
Additional information
Funding
References
- Babcock, B., Babu, S., Datar, M., Motwani, R., & Widom, J. (2002). Models and issues in data stream systems. In Proceedings of the 21 ACM SIGMOD-SIGACT-SIGART symposium on principles of database systems (pp. 1–16). ACM.
- Back, K., & Brown, D. P. (1992). GMM, maximum likelihood, and nonparametric efficiency. Economics Letters, 39(1), 23–28. https://doi.org/10.1016/0165-1765(92)90095-G
- Braverman, M., Garg, A., Ma, T., Nguyen, H., & Woodruff, D. (2016). Communication lower bounds for statistical estimation problems via a distributed data processing inequality. In Proceedings of the 48th annual ACM symposium on theory of computing (pp. 1011–1020). ACM.
- Borenstein, M., Hedges, L. V., Higgins, J. P. T., & Rothstein, H. (2009). Introduction to meta-analysis. Wiley.
- Chatterjee, N., Chen, Y.-H., Maas, P., & Carroll, R. J. (2016). Constrained maximum likelihood estimation for model calibration using summary-level information from external big data sources. Journal of the American Statistical Association, 111(513), 107–117. https://doi.org/10.1080/01621459.2015.1123157
- Chaudhuri, S., Handcock, M. S., & Rendall, M. S. (2008). Generalized linear models incorporating population level information: an empirical likelihood based approach. Journal of the Royal Statistical Society: Series B, 70(2), 311–328. https://doi.org/10.1111/rssb.2008.70.issue-2
- Chen, J., & Qin, J. (1993). Empirical likelihood estimation for finite populations and the effective usage of auxiliary information. Biometrika, 80(1), 107–116. https://doi.org/10.1093/biomet/80.1.107
- Chen, J., Sitter, R., & Wu, C. (2002). Using empirical likelihood methods to obtain range restricted weights in regression estimators for surveys. Biometrika, 89(1), 230–237. https://doi.org/10.1093/biomet/89.1.230
- Cochran, W. G. (1977). Sampling techniques (3rd ed.). Wiley.
- Dersimonian, R., & Laird, N. (1986). Meta-analysis in clinical trials. Controlled Clinical Trials, 7(3), 177–188. https://doi.org/10.1016/0197-2456(86)90046-2
- Duan, R., Ning, Y., & Chen, Y. (2020). Heterogeneity-aware and communication-efficient distributed statistical inference. arXiv:1912.09623v1.
- Duchi, J., Jordan, M., Wainwright, M., & Zhang, Y. (2015). Optimality guarantees for distributed statistical estimation. arXiv:1405.0782.
- Han, P., & Lawless, J. (2016). Comment. Journal of the American Statistical Association, 111(513), 118–121. https://doi.org/10.1080/01621459.2016.1149399
- Hansen, L. P. (1982). Large sample properties of generalized method of moments estimators. Econometrica, 50(4), 1029–1054. https://doi.org/10.2307/1912775
- Hartely, H. O., & Rao, J. N. K. (1968). A new estimation theory for sample surveys. Biometrika, 55(3), 547–557. https://doi.org/10.1093/biomet/55.3.547
- Imbens, G., & Lancaster, T. (1994). Combining micro and macro data in microeconometric models. Review of Economic Studies, 61(4), 655–680. https://doi.org/10.2307/2297913
- Jordan, M. I., Lee, J. D., & Yang, Y. (2019). Communication-efficient distribution statistical inference. Journal of the American Statistical Association, 114(526), 668–681. https://doi.org/10.1080/01621459.2018.1429274
- Lee, J., Liu, Q., Sun, Y., & Taylor, J. (2017). Communication-efficient sparse regression. Journal of Machine Learning Research, 18, 1–30. http://jmlr.org/papers/v18/16-002.html
- Lin, D. Y., & Zeng, D. (2010). On the relative efficiency of using summary statistics versus individual-level data in meta-analysis. Biometrika, 97(2), 321–332. https://doi.org/10.1093/biomet/asq006
- Luo, L. (2020). Renewable estimation and incremental inference in generalized linear models with streaming data sets. Journal of the Royal Statistical Society, Series B, 82(1), 69–97. https://doi.org/10.1111/rssb.12352
- Neiswanger, W., Wang, C., & Xing, E. (2015). Asymptotically exact, embarrassingly parallel MCMC. In Proceedings of the 30th conference on uncertainty in artificial intelligence (pp. 623–632). AUAI Press.
- Nguyen, T. D., Shih, M. H., Srivastava, D., Tirthapura, S., & Xu, B. (2021). Stratified random sampling from streaming and stored data. Distributed and Parallel Databases, 39(3), 665–710. https://doi.org/10.1007/s10619-020-07315-w
- Owen, A. B. (1988). Empirical likelihood ratio confidence intervals for a single functional. Biometrika, 75(2), 237–249. https://doi.org/10.1093/biomet/75.2.237
- Owen, A. B. (1990). Empirical likelihood ratio confidence regions. Annals of Statistics, 18(1), 90–120. https://doi.org/10.1214/aos/1176347494
- Owen, A. B. (2001). Empirical likelihood. CRC.
- Qin, J. (2000). Combining parametric and empirical likelihoods. Biometrika, 87(2), 484–490. https://doi.org/10.1093/biomet/87.2.484
- Qin, J. (2017). Biased sampling, over-identified parameter problems and beyond. Springer.
- Qin, J., & Lawless, J. (1994). Empirical likelihood and general equations. Annals of Statistics, 22(1), 300–325. https://doi.org/10.1214/aos/1176325370
- Qin, J., Zhang, H., Li, P., Albanes, D., & Yu, K. (2015). Using covariate specific disease prevalence information to increase the power of case-control study. Biometrika, 102(1), 169–180. https://doi.org/10.1093/biomet/asu048
- Susanne, M. S. (2007). Point estimation with exponentially tilted empirical likelihood. Annals of Statistics, 35(2), 634–672. https://doi.org/10.1214/009053606000001208
- Tian, L., & Gu, Q. (2016). Communication-efficient distributed sparse linear discriminant analysis. arXiv:1610.04798.
- van de Geer, S., Buhlmann, P., Ritov, Y., & Dezeure, R. (2014). On asymptotically optimal confidence regions and tests for high dimensional models. Annals of Statistics, 42(3), 1166–1202. https://doi.org/10.1214/14-AOS1221
- van de Vaart, V. W. (2000). Asymptotic statistics. Cambridge University Press.
- Wang, X., & Dunson, D. (2015). Parallelizing MCMC via Weierstrass sampler. arXiv:1312.4605.
- Wang, J., Kolar, M., Srebro, N., & Zhang, T. (2017). Efficient distributed learning with sparsity. In Proceedings of the 34th international conference on machine learning, Sydney, Australia, PMLR 70 (pp. 3636–3645).
- Wu, C., & Sitter, R. R. (2001). A model-calibration approach to using complete auxiliary information from survey data. Journal of the American Statistical Association, 96(453), 185–193. https://doi.org/10.1198/016214501750333054
- Wu, C., & Thompson, M. E. (2020). Sampling theory and practice. Springer.
- Zeng, D. & Lin, D. Y. (2015). On random-effects meta-analysis. Biometrika, 102(2), 281–294.
- Zhang, Y., Duchi, J., & Wainwright, M. (2013). Communication-efficient algorithms for statistical optimization. Journal of Machine Learning Research, 14, 3321–3363.
- Zhang, H., Deng, L., Schiffman, M., Qin, J., & Yu, K. (2020). Generalized integration model for improved statistical inference by leveraging external summary data. Biometrika, 107(3), 689–703. https://doi.org/10.1093/biomet/asaa014