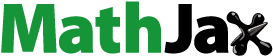
Abstract
In two-level fractional factorial designs, conditional main effects can provide insights by which to analyze factorial effects and facilitate the de-aliasing of fully aliased two-factor interactions. Conditional main effects are of particular interest in situations where some factors are nested within others. Most of the relevant literature has focused on the development of data analysis tools that use conditional main effects, while the issue of optimal factorial design for a given linear model involving conditional main effects has been largely overlooked. Mukerjee, Wu and Chang [Statist. Sinica 27 (2017) 997–1016] established a framework by which to optimize designs under a conditional effect model. Although theoretically sound, their results were limited to a single pair of conditional and conditioning factors. In this paper, we extend the applicability of their framework to double pairs of conditional and conditioning factors by providing the corresponding parameterization and effect hierarchy. We propose a minimum contamination-based criterion by which to evaluate designs and develop a complementary set theory to facilitate the search of minimum contamination designs. The catalogues of 16- and 32-run minimum contamination designs are provided. For five to twelve factors, we show that all 16-run minimum contamination designs under the conditional effect model are also minimum aberration according to Fries and Hunter [Technometrics 22 (1980) 601–608].
1. Introduction
Factorial designs have been widely used in industry and academia in the past decades. Two-level fractional factorial designs have proven particularly effective in situations in which the purpose of experiments is to screen out inactive factors. Researchers have dedicated considerable effort to the evaluation of two-level fractional factorial designs. Fries and Hunter (Citation1980) proposed a model robust criterion named minimum aberration tailored specifically to two-level regular fractional factorial designs. The minimum aberration criterion minimizes the wordlength patterns of two-level regular designs in a sequential manner from lower-order factorial effects to higher-order ones. It is established for design selection under the assumption that lower-order effects are more important than higher-order effects and effects of the same order are equally important. The minimum aberration criterion has since been adaptive to accommodate nonregular fractional factorial designs (Cheng et al., Citation2002; Tang & Deng, Citation1999; Xu & Wu, Citation2001) and multi-stratum factorial designs (Chang, Citation2022; Chang & Cheng, Citation2018). All the aforementioned minimum aberration criteria were developed under an orthogonal parameterization of factorial effects (Cheng, Citation2014, chapter 6). Accordingly, a defining word of length four, say , creates three pairs of fully aliased two-factor interactions as follows:
,
and
. The two-factor interactions in each pair are completely mixed up and cannot be estimated simultaneously. Interested readers may refer to Mukerjee and Wu (Citation2006), Cheng (Citation2014) and Wu and Hamada (Citation2021) for further details.
In some practical situations, it is preferable to investigate a two-factor interaction via two conditional main effects, with each one conditionally defined according to another factor of a specific level. For example, the two-factor interaction can be decomposed as the difference between a conditional main effect
conditioned on the low level of
and that conditioned on the high level of
. Sliding level experiments in engineering are structured in this way (Wu & Hamada, Citation2021, p. 343), where the interest is on the conditional main effects conditioned on the slid factors. Mukerjee et al. (Citation2017) reported on an industrial experiment involving motor and speed as two factors. The objective of the experiment was to assess the comparison of the motors separately at each speed; therefore, the conditional main effects of the motors conditioned on each level of speed are of particular interest. Other intriguing examples pertaining to the use of conditional main effects are outlined in Wu (Citation2015) and Wu (Citation2018).
It is known, also mentioned in Wu (Citation2015), that for 2 two-level factors and
, the main effect
in conjunction with the interaction
spans the same vector space as that spanned by the conditional main effects
respectively conditioned on the two levels of
. Mukerjee et al. (Citation2017) referred to
and
as a pair of conditional and conditioning factors. To deal with a single pair of conditional and conditioning factors, Mukerjee et al. (Citation2017) proposed a model in which the main effect of
and the two-factor interaction
are replaced with the two associated conditional main effects. This can be viewed as an alternative to the aforementioned orthogonal parameterization, under which the defining relation
produces partially aliased effects (neither fully aliased nor orthogonal). In this paper, the model proposed by Mukerjee et al. (Citation2017) is referred to as a conditional effect model. The fact that the parameterization of a single-pair conditional effect model differs from that of a model tailored to the minimum aberration necessitates a new aberration criterion applicable to conditional effect models. Mukerjee et al. (Citation2017) proposed a minimum aberration criterion as well as a strategy by which to search for designs under a single-pair conditional effect model. Apart from Mukerjee et al. (Citation2017), an alternative approach by the use of the indicator function in Pistone and Wynn (Citation1996) is given by Sabbaghi (Citation2020), who developed an algebra for conditional effect models. From the data analysis aspect, Mak and Wu (Citation2019) proposed a bi-level variable selection of conditional main effects in observational data using a penalty function with two layers: the outer one controlling between-group selection, and the inner one controlling within-group selection.
It is important to consider that the parameterization under a condition effect model destroys the fully aliased relationship between two-factor interactions. Thus, one application of conditional main effects involves the de-aliasing of two-factor interactions in regular fractional factorial designs of resolution four. This involves identifying significant but aliased two-factor interactions and then transforming the model to the corresponding conditional effect model. Afterwards, the de-aliasing strategies in Wu (Citation2015), Su and Wu (Citation2017), Chang (Citation2019) and Lawson (Citation2020) can then be used to facilitate subsequent data analysis.
In the current study, we consider two-level factorial designs in conjunction with conditional effect models involving two pairs of conditional and conditioned factors. The remaining factors, not involving the two pairs of conditional and conditioning factors, are called traditional factors. Wu and Hamada (Citation2021, p. 347) described an experiment involving the sealing of a light bulb, in which the outcomes were determined mainly by two pairs of conditional and conditioning factors and
, respectively. The corresponding linear model comprised the conditional main effects of H and J respectively conditioned on G and I. In the current study, we extend the work of Mukerjee et al. (Citation2017) to double-pair conditional effect models. The minimum aberration was based on the effect hierarchy principle (Wu & Hamada, Citation2021, p. 168); however, it is difficult to artificially argue an order among the effects in a conditional effect model. In accordance with Mukerjee et al. (Citation2017), we adopt the Bayesian approach proposed in Mitchell et al. (Citation1995), later popularized by Kerr (Citation2001), Joseph (Citation2006), Joseph and Delaney (Citation2007), Ai et al. (Citation2009), Joseph et al. (Citation2009), Kang and Joseph (Citation2009), Chang and Cheng (Citation2018) and Chang (Citation2019, Citation2022), to derive an order of factorial effects based on their prior variances. Once this order has been established, we then define a criterion for design evaluation by sequentially minimizing the bias (contamination) caused by lower-order interactions to higher-order interactions. We refer to the proposed criterion as the minimum contamination criterion. We provide the catalogues of 16-run and 32-run minimum contamination designs for various factor numbers at the end of this paper.
The remainder of this paper is organized as follows. Section 2 introduces the parametrization and a Bayesian-inspired hierarchical order of effects under a double-pair conditional effect model. Some sufficient conditions for a design to be universally optimal under the conditional effect model involving only main effects are given in Section 3. Section 4 presents a new minimum contamination criterion defined according to the Bayesian-inspired hierarchical order. In addition, we develop a complementary set theory to guide the search for minimum contamination designs involving a large number of factors. An efficient computational procedure is developed in Section 5 to search for minimum contamination regular/nonregular designs for an arbitrary number of factors. Numerical examples and a real experiment are discussed. Conclusions are drawn in Section 6.
2. Conditional effect model and Bayesian-inspired effect hierarchy
We give the details regarding the parameterization under a double-pair conditional effect model. We adopt the Bayesian approach in Mitchell et al. (Citation1995) to derive an effect order, which serves as the building block for the minimum contamination criterion introduced in Section 4.
2.1. Conditional effect model
A double-pair conditional effect model is a linear model with reparameterization using two pairs of conditional and conditioning factors. Consider a full factorial design with n (
) factors
, each at levels 0 and 1. Without loss of generality, let
be one pair of conditional and conditioned factors and
be the other pair. The main effect and interaction effects involving
(respectively,
) are defined conditionally on each fixed level of
(respectively,
). Define Ω as the set of
binary n-tuples representing the
treatment combinations of the full factorial design. For
, let
be the treatment effect of treatment combination
. Under a linear model,
is the expectation of the response measured at the treatment combination
. Similarly, we write
for the factorial effect
using the conventional orthogonal parameterization (Cheng, Citation2014, chapter 6) when
is nonnull, and
for the grand mean. With n = 3 for illustration,
separately represent the main effect of
, the two-factor interaction of
and
, and the three-factor interaction of
. Each
can be interpreted using the mean responses measured at different levels of factors. For example, the main effect
is the average of the difference between the mean responses measured at the levels 1 and 0 of
. Let
and
be
vectors with elements
and
arranged in the lexicographic order, respectively, where for n = 3, we have
Then, the linear model using the orthogonal parameterization under the full factorial design is given by
(1)
(1) where ⊗ represents the Kronecker product and
denotes the n-fold Kronecker product of
a Hadamard matrix of order two. When interpreted under the linear model, the columns of
respectively correspond to the grand mean and a contrast of the mean responses measured at the two levels of the factor. Since a
full factorial design has a cross-product structure, the associated matrix of the grand mean and contrasts can be obtained via the n-fold Kronecker product of
as in (Equation1
(1)
(1) ). We refer to the model in (Equation1
(1)
(1) ) as a traditional model under the full factorial design.
Let and
be the top and bottom rows of
, respectively. Emphasizing
and
, we express Equation (Equation1
(1)
(1) ) as
(2)
(2) Let
be the factorial effect
under a conditional effect model with two pairs conditional and conditioning factors
and
, respectively. Denote the vector with the ν elements
's by
in the same lexicographic order as
. Under the double-pair conditional effect model, the factorial effects involving
and
are defined conditionally on the levels of
and
, respectively. Thus in (Equation2
(2)
(2) ),
is replaced with
whenever
precedes it. We can reparametrize
by
with
(3)
(3) where
in which
is the identity matrix of order two. The model in (Equation3
(3)
(3) ) is called a double-pair conditional effect model in this paper. The factorial effects involving
and
are referred to as conditional (factorial) effects, while those not involving them are referred to as unconditional (factorial) effects. We cluster
into various groups of vectors representing unconditional and conditional factorial effects. Define
where
. It is apparent that
if
. Let
be the vector with elements
, where
. Later we will see that the effects associated with the same
have the same importance using the Bayesian-inspired effect hierarchy introduced in the next subsection.
2.2. Bayesian-inspired effect hierarchy
Chipman et al. (Citation1997) proposed a Bayesian variable selection for designed experiments with complex aliasing. Rather than independence prior distribution on the factorial effects, Chipman et al. (Citation1997) used a hierarchical prior that is consistent with the effect heredity principle (Wu & Hamada, Citation2021, p. 169). A basic idea of their approach is to assign a larger prior variance to a more important factorial effect. This idea is compatible with the Bayesian (functional) prior distribution derived by Mitchell et al. (Citation1995), Kerr (Citation2001), Joseph (Citation2006), Joseph and Delaney (Citation2007), Ai et al. (Citation2009), Joseph et al. (Citation2009), Kang and Joseph (Citation2009), Chang and Cheng (Citation2018) and Chang (Citation2019, Citation2022). With the derived prior variances, one can readily define an effect hierarchical order and an aberration-like criterion for design evaluation.
In this paper, we adopt the Bayesian approach in Mitchell et al. (Citation1995), who set up a functional prior by regarding as a realization of a stationary Gaussian random function. Then the prior distribution of factorial effects is induced by the relation in (Equation1
(1)
(1) ). When applied to (Equation3
(3)
(3) ), the Bayesian approach induces an effect hierarchy of the
's via a prior specification on
in terms of a zero-mean Gaussian random function such that
, where
and the
matrix
has diagonal elements 1 and off-diagonal elements ρ,
. This covariance structure is equivalent to Equation (4) of Joseph (Citation2006). It follows by expanding
that the correlation of
and
is
which is equal to
and only depends on the total number of components where
and
differ. Thus, such a covariance structure can be interpreted as treating every factor equally, which is reasonable since there is usually no knowledge about the importance of the factors at the experimentation stage. In conjunction with (Equation3
(3)
(3) ), the prior covariance matrix of
is given by
The following result gives the variances of the
's.
Theorem 2.1
For a , we have
Proof.
By the identity , one can easily verify that
,
and
is a
matrix, which can be regarded as a
block-matrix with each block being a
matrix. Denote the
th block-matrix of
by
. Then by calculation, we get
when
; for i = j, we obtain
,
,
and
.
The diagonal elements of , denoted by
, can be obtained as
. We also have
,
and
. Thus, we get
,
,
and
.
Since the variances of 's are only related to the diagonal elements of
, one can easily check for a
,
based on the above calculation.
Let for
. From Theorem 2.1, it is clear that
for
. Because
for all
, we have
(4)
(4) In view of (Equation4
(4)
(4) ), we define the following effect hierarchy under the conditional effect model (Equation3
(3)
(3) ) as follows. The unconditional main effects have the largest variance
and are the most important, while the conditional main effects with variance
are positioned next; then come the unconditional two-factor interactions (
), followed by the one-pair conditional two-factor interactions (
), then two-pair conditional two-factor interactions (
), and so on. This effect hierarchy order is not surprising since a conditional main effect is proportional to the average of a unconditional main effect and two-factor interaction, resulting in a variance in-between.
3. Universally optimal designs for main effect model
As mentioned in Mukerjee et al. (Citation2017), a justifiable criterion for design evaluation is to identify a class of designs which ensure optimal inference on the 's corresponding to
and
in the absence of all interactions. Then, in order to possess model robustness, among these designs we find one which sequentially minimizes a suitably defined measure of bias caused by successive interactions in the effect hierarchy. In this section, we connect the conditional effect model with the traditional model. Then we provide some sufficient conditions for a design to be universally optimal under a main-effect conditional effect model.
The connection between conditional effects and traditional factorial effects
can be established by (Equation1
(1)
(1) ) and (Equation3
(3)
(3) ) as follows:
By using the fact
and
we obtain
which implies
because
. This yields
where
. In view of
, the first of above identities shows that
of the
's remain unconditional effects, while the other 3/4 of the
's involve
and
and hence are a combination of the conditional effects. These equations uncover the connection between factorial effects under the traditional model and under the conditional effect model. Take n = 5 for example. We have
. Recall that
and
are the conditional main effects of
conditioned on levels 0 and 1 of
, respectively. Thus, the unconditional main effect of
is proportional to the average of the two conditional main effects of
. Likewise, we have
, which means that the unconditional two-factor interaction of
and
is proportional to the difference between the two conditional main effects of
.
Consider an N-run design represented by the design matrix
with elements 1 (high level) and
(low level). Denote the corresponding
full model matrix under (Equation1
(1)
(1) ) by
, each column corresponding to one
. Note that
can be obtained by deleting the rows of
that are not in
. With the vector of responses
, we have
. Each column of
is represented by
with
. The connection between
's and
's suggests
where
. Let
and
consist of the
's and
's respectively, where
. Then the conditional effect model under
can be represented by
(5)
(5) We follow the convention that the random observational errors are uncorrelated and homogeneous with equal variance.
3.1. Universally optimal designs
If all interactions are absent, then the model (Equation5(5)
(5) ) reduces to
(6)
(6) consisting of only unconditional and conditional main effects. In the following, we present a theorem which gives some requirements for a design to be universally optimal under model (Equation6
(6)
(6) ).
Theorem 3.1
Suppose an N-run design satisfies
(i) |
| ||||
(ii) | all eight triples of symbols occur equally often when | ||||
(iii) | all eight triples of symbols occur equally often when | ||||
(iv) | all sixteen triples of symbols occur equally often when |
Proof.
Let . Denote the information matrix of
and
under model (Equation6
(6)
(6) ) by
. Note that
can be obtained by the Schur complement
which can be simplified as
because
. Because
is nonnegative definite, we have
(7)
(7) for every N-run design. Under the conditions (i),…,(iv), it is easy to verify that
and
. Thus
and
reaches the upper bound in (Equation7
(7)
(7) ). The result now follows from Kiefer (Citation1975).
By Theorem 3.1, a necessary condition for universally optimal designs is . Therefore, if n = 4, the model (Equation5
(5)
(5) ) can only involve the two pairs of conditional and conditioning factors
and
. Then the universally optimal design is exactly the
full factorial design. Thus, to avoid trivialities, we let
in the discussion of design selection in the next section.
4. Minimum contamination and complementary set theory
The designs meeting the conditions (i),…,(iv) of Theorem 3.1 are universally optimal under model (Equation6(6)
(6) ). In addition,
is the best linear unbiased estimator of
, h = 0, 1. However, nonnegligible interactions may exist and
is no longer unbiased in this case. We revert back to the model (Equation5
(5)
(5) ), which includes all interactions, to assess the impact of possible presence of interactions on
. Under model (Equation5
(5)
(5) ),
has bias
. The matrix
is referred to as an alias matrix in Wu and Hamada (Citation2021, p. 419). A reasonable measure of the bias in
caused by the interactions, as in Tang and Deng (Citation1999), is
where the last equality holds because
is an orthogonal transform of
. Based on the effect hierarchy in (Equation4
(4)
(4) ), one should minimize the bias successively in order of priority. Thus, we define a minimum contamination design as the one which minimizes the terms of
(8)
(8) in a sequential manner from left to right. In (Equation8
(8)
(8) ),
appears before
because the contamination or bias in
is deemed more severe than in
. The concept of minimizing contamination due to the existence of interactions is not new. We note that Cheng and Tang (Citation2005) used this idea to develop a general theory for minimum aberration.
The minimum contamination criterion in (Equation8(8)
(8) ) induces a ranking of designs of the same run size. It is time consuming, however, to find the minimum contamination design via complete search using (Equation8
(8)
(8) ) if n is large. A useful technique of design construction is via complementary designs. Tang and Wu (Citation1996) provided identities related to the wordlength pattern of a regular two-level design to that of its complementary design. Suen et al. (Citation1997) extended these identities to regular
designs. Cheng (Citation2014, p. 179) reviewed the design construction using complementary designs.
We now focus on regular designs under the conditional effect model due to their nice properties and popularity among practitioners. Let be the set of nonnull
binary vectors. All operations with these vectors are over the finite field GF(2). Regarding the notation, we do not apply bold font style to these binary vectors to distinguish them from the vectors with the elements belonging to real numbers. A regular design in
(r<n) runs is given by n distinct vectors
from
such that the matrix
has full row rank. The design consists of the N treatment combinations of the form
, where
.
In the following, we define some useful quantities to represent . Let
be the number of ways of choosing l out of
such that the sum of the chosen l equals 0;
be the number of ways of choosing l out of
such that the sum of the chosen l is in the set
;
be the number of ways of choosing l out of
such that the sum of the chosen l is in the set
;
;
be the number of ways of choosing l out of
such that the sum of the chosen l is in the set
;
be the number of ways of choosing l out of
such that the sum of the chosen l is in the set
;
;
be the number of ways of choosing l out of
such that the sum of the chosen l is in the set
;
be the number of ways of choosing l out of
such that the sum of the chosen l is in the set
;
be the number of ways of choosing l out of
such that the sum of the chosen l is in the set
;
be the number of ways of choosing l out of
such that the sum of the chosen l is in the set
;
be the number of ways of choosing l out of
such that the sum of the chosen l is in the set
. The next result, with the proof deferred to the appendix, gives expressions for
in terms of the quantities just introduced.
Theorem 4.1
For , we have
(a) |
| ||||
(b) |
| ||||
(c) |
| ||||
(d) |
| ||||
(e) |
| ||||
(f) |
|
In view of Theorem 4.1, sequential minimization of K is equivalent to that of the terms of , which is reduced to
because
form a complete factorial, implying
.
We now develop a complementary set theory for the first four terms in the sequence A. Let be the complement of
in
;
be the number of ways of choosing l members of
such that the sum of the chosen l equals 0. Let
;
;
be the number of ways of choosing l members of
such that the sum of the chosen l is in
;
be the number of ways of choosing l members of
such that the sum of the chosen l is in
.
Theorem 4.2
Let ,
, be constants irrelevant to designs and
. Define
as Equation (2) in Mukerjee and Wu (Citation2001). We have
(a) |
| ||||
(b) |
| ||||
(c) |
| ||||
(d) |
|
Proof.
Parts (a) and (b) are evident from Tang and Wu (Citation1996).
For (c), note that , which can be simplified as
by Lemmas 1 and 3 in Mukerjee and Wu (Citation2001), where c is a constant for every design. Because the design is an orthogonal array of strength two, we have
and
. Hence
. Similarly,
, where
is a constant for every design. Therefore, we have
by letting
.
For (d), note that . Let
. If
for
, then
. If
for some
, then
by Lemmas 1 and 3 in Mukerjee and Wu (Citation2001), where
is a constant for every design. Since
for some
, we have F = T and
. Thus
. Similarly, we have
,
and
. So,
.
Theorem 4.2 provides a way to evaluate designs using the sequence A, and equivalently sequence K, with the number of factors , where
is the cardinality of
. Once
is constructed, one can quickly get
by the identity
, and construct the design
. Constructing
with minimum contamination given a large
is usually time-consuming. It is more practical to find a small
with minimum contamination, leading to a large n. Thus, Theorem 4.2 helps find large minimum contamination designs. For example, consider
. The set
can be verified to have maximal
, where
are four linearly independent vectors from
. Moreover, assigning
,
,
and
results in minimal
. Thus, we have
and construct a minimum contamination design with the number of factors N−6, which equals 10, 26, 58 for 16-, 32- and 64-run designs.
5. Efficient design search and examples
Finding minimum contamination designs using (Equation8(8)
(8) ) is a daunting task for even moderate run size and number of factors. This section presents an extension of a searching procedure given by Mukerjee et al. (Citation2017) to the current setting and provides examples for illustration.
5.1. A procedure for efficient design search
The minimum contamination criterion (Equation8(8)
(8) ) can be applied to regular and nonregular designs, but requires heavy computation of
. By noting that
is reminiscent of minimum moment aberration in Xu (Citation2003), Mukerjee et al. (Citation2017) developed an efficient computational procedure for
. We now extend this procedure for computing
to double-pair conditional effect models. For
, let
,
, and
(9)
(9) where
. Write
for the subarray given by the last n−4 columns of
(i.e. only consisting of traditional factors). For
, let
be the number of positions where the uth and wth rows of
have the same entry, and
be the
th element of
. Denote the
th element of
by
. Then the following result holds.
Theorem 5.1
For and
, we have
(a) |
| ||||
(b) |
| ||||
(c) |
|
Proof.
For , let
be the sum over binary tuples
such that l of
equal 1. We have
where
. Similarly, we have
The result will follow if
. It is clear that
and
. It remains to show
satisfies the recursion relation (Equation9
(9)
(9) ).
Let and let
be the lth derivative of
. Note that
. Differentiation of
yields
that is,
. Differentiating this l−1 and taking
, we get
This leads to (Equation9
(9)
(9) ) by using
.
With the help of Theorem 5.1 and suggested by Mukerjee et al. (Citation2017), an algorithm is provided as follows.
Search for minimum contamination designs by first listing of all nonisomorphic regular designs for given run size
and number of factors
.
For each nonisomorphic regular design, permute its columns such that the resulting design satisfies the conditions in Theorem 3.1. Let the first four columns represent the two pairs of conditional and conditioned factors, that is,
and
.
Calculate the criterion in (Equation8
(8)
(8) ) by using Theorem 5.1, and hence find a minimum contamination design.
This procedure mostly consumes affordable computational time. For N = 16 and n = 10, for example, it takes around 3.94 minutes to find a minimum contamination design on a desktop with 3.8 GHz CPU and 64 GB of RAM.
5.2. Examples
We apply the algorithm presented in Section 5.1 to 16- and 32-run designs. Afterwards, the light bulb experiment (Wu & Hamada, Citation2021, p. 347) mentioned in Section 1 is revisited.
For N = 16 and 32, a list of all nonisomorphic regular designs are given in the catalogues in Chen et al. (Citation1993). Table 1 exhibits the results for N = 16 and . In the table, the numbers 1,2,4,8 represent basic factors in a design. The other numbers represent added factors. For example, for n = 5, if the five factors are denoted by A, B, C, D, E, then the minimum contamination design is the one with the defining relation E = ABCD because 15 = 1 + 2 + 4 + 8. We can see that all minimum contamination designs under conditional effect models are also minimum aberration under traditional models. The finding supports using minimum aberration designs under traditional models to perform subsequent de-aliasing analysis in Su and Wu (Citation2017). Table 2 exhibits the results for N = 32 and
. Same as Table 1, the numbers 1,2,4,8,16 represent basic factors in a design. The other numbers represent added factors. For example, for n = 6, if the five factors are denoted by A, B, C, D, E, F, then the minimum contamination design is the one with the defining relation F = ABCDE because 31 = 1 + 2 + 4 + 8 + 16. The R codes for generating these designs are attached to the supplementary material.
Table 2. Regular minimum contamination designs for N = 32.
6. Concluding remarks
This paper extends the work of Mukerjee et al. (Citation2017) to two pairs of conditional and conditioning factors. The conditional effect model in (Equation3(3)
(3) ) is an orthogonal reparameterization of the traditional model in (Equation1
(1)
(1) ). Such a reparameterization introduces a new effect hierarchy order of factorial effects, resulting in a different design evaluation from the minimum aberration due to Fries and Hunter (Citation1980). We note that alternative reparameterization of (Equation1
(1)
(1) ) is required if the topic of interest does not depend on conditional effects. For example, Yang and Speed (Citation2002) proposed to define the factorial effects with reference to natural baseline levels of the factors, referred to as baseline parameterization. Later, Mukerjee and Tang (Citation2012), Mukerjee and Huda (Citation2016) and Sun and Tang (Citation2022) discussed design optimality and construction under the baseline parameterization.
Table 1. Regular minimum contamination designs for N = 16.
Table 3. Sixteen-run minimum contamination design with ten factors for the light bulb experiment.
Mukerjee et al. (Citation2017) claimed that, in practice, the number of conditional and conditioned pairs seldom exceeds two. On the other hand, the effect hierarchy order in (Equation4(4)
(4) ) is only irrelevant to the value of r when the number of pairs is not greater than two. When dealing with more than two pairs, the effect hierarchy order is a nontrivial function of r and deriving useful results can be exceedingly difficult. Alternatively, we note that the minimum contamination criterion in (Equation8
(8)
(8) ) can be applied to regular as well as nonregular designs; however, this paper focuses on regular designs owing to their popularity and theoretical underpinnings. The application of this framework to nonregular designs is left for future research. Another interesting future direction suggested by a reviewer is to involve qualitative four-level conditional/conditioning factors. It is known that a qualitative four-level factor can be decomposed into three orthogonal main effect components with two levels each (Wu & Hamada, Citation2021, chapter 7). However, our theory cannot be directly applied since the three two-level main effect components do not share the same properties as a real two-level factor. The covariance structure for the main effect components is different from that for two-level factors; see Joseph et al. (Citation2009) for a discussion. The resulting effect hierarchy order of conditional factorial effects may be complicated with vague interpretations. Since this topic requires a nontrivial extension, we leave it for future research.
Supplemental Material
Download (6.5 KB)Acknowledgments
We thank the Editor and two Reviewers for their constructive comments and suggestions, which have helped us to improve the article.
Disclosure statement
No potential conflict of interest was reported by the author(s).
Additional information
Funding
References
- Ai, M. Y., Kang, L., & Joseph, V. R. (2009). Bayesian optimal blocking of factorial designs. Journal of Statistical Planning Inference, 139(9), 3319–3328. https://doi.org/10.1016/j.jspi.2009.03.008
- Chang, M. C. (2019). De-aliasing in two-level factorial designs: A Bayesian approach. Journal of Statistical Planning and Inference, 203, 82–90. https://doi.org/10.1016/j.jspi.2019.03.002
- Chang, M. C. (2022). A unified framework for minimum aberration. Statistica Sinica, 32(1), 251–270.
- Chang, M. C., & Cheng, C. S. (2018). A Bayesian approach to the selection of two-level multi-stratum factorial designs. Annals of Statistics, 46(4), 1779–1806.
- Chen, J., Sun, D. X., & Wu, C. F. J. (1993). A catalogue of two-level and three-level fractional factorial designs with small runs. International Statistical Review, 61(1), 131–145. https://doi.org/10.2307/1403599
- Cheng, C. S. (2014). Theory of factorial design: Single- and multi-stratum experiments. CRC Press.
- Cheng, C. S., Deng, L. Y., & Tang, B. (2002). Generalized minimum aberration and design efficiency for nonregular fractional factorial designs. Statistica Sinica, 12(4), 991–1000.
- Cheng, C. S., & Tang, B. (2005). A general theory of minimum aberration and its applications. Annals of Statistics, 33(2), 944–958. https://doi.org/10.1214/009053604000001228
- Chipman, H., Hamada, M., & Wu, C. F. J. (1997). A bayesian variable-selection approach for analyzing designed experiments with complex aliasing. Technometrics, 39(4), 372–381. https://doi.org/10.1080/00401706.1997.10485156
- Fries, A., & Hunter, W. G. (1980). Minimum aberration 2k−p designs. Technometrics, 22(4), 601–608.
- Joseph, V. R. (2006). A Bayesian approach to the design and analysis of fractionated experiments. Technometrics, 48(2), 219–229. https://doi.org/10.1198/004017005000000652
- Joseph, V. R., Ai, M., & Wu, C. F. J. (2009). Bayesian-inspired minimum aberration two- and four-level designs. Biometrika, 96(1), 95–106. https://doi.org/10.1093/biomet/asn062
- Joseph, V. R., & Delaney, J. D. (2007). Functionally induced priors for the analysis of experiments. Technometrics, 49(1), 1–11. https://doi.org/10.1198/004017006000000372
- Kang, L., & Joseph, V. R. (2009). Bayesian optimal single arrays for robust parameter design. Technometrics, 51(3), 250–261. https://doi.org/10.1198/tech.2009.08057
- Kerr, M. K. (2001). Bayesian optimal fractional factorials. Statistica Sinica, 11(3), 605–630.
- Kiefer, J. (1975). Construction and optimality of generalized Youden designs. In A survey of statistical design and linear models (pp. 333–353). North-Holland.
- Lawson, J. (2020). Comparison of conditional main effects analysis to the analysis of follow-up experiments for separating confounded two-factor interaction effects in 2IVk−p fractional factorial experiments. Quality and Reliability Engineering International, 36(4), 1454–1472. https://doi.org/10.1002/qre.v36.4
- Mak, S., & Wu, C. F. J. (2019). cmenet: A new method for bi-level variable selection of conditional main effects. Journal of the American Statistical Association, 114(526), 844–856. https://doi.org/10.1080/01621459.2018.1448828
- Mitchell, T. J., Morris, M. D., & Ylvisaker, D. (1995). Two-level fractional factorials and Bayesian prediction. Statistica Sinica, 15(2), 559–573.
- Mukerjee, R., & Huda, S. (2016). Approximate theory-aided robust efficient factorial fractions under baseline parameterization. Annals of the Institute of Statistical Mathematics, 68(4), 787–803. https://doi.org/10.1007/s10463-015-0509-x
- Mukerjee, R., & Tang, B. (2012). Optimal fractions of two-level factorials under a baseline parametrization. Biometrika, 99(1), 71–84. https://doi.org/10.1093/biomet/asr071
- Mukerjee, R., & Wu, C. F. J. (2001). Minimum aberration designs for mixed factorials in terms of complementary sets. Statistica Sinica, 11(1), 225–239.
- Mukerjee, R., & Wu, C. F. J. (2006). A modern theory of factorial designs. Springer.
- Mukerjee, R., Wu, C. F. J., & Chang, M. C. (2017). Two-level minimum aberration designs under a conditional model with a pair of conditional and conditioning factors. Statistica Sinica, 27(3), 997–1016.
- Pistone, G., & Wynn, H. P. (1996). Generalised confounding with gröbner bases. Biometrika, 83(3), 653–666. https://doi.org/10.1093/biomet/83.3.653
- Sabbaghi, A. (2020). An algebra for the conditional main effect parameterization. Statistica Sinica, 30(2), 903–924.
- Su, H., & Wu, C. F. J. (2017). CME analysis: A new method for unraveling aliased effects in two-level fractional factorial experiments. Journal of Quality Technology, 49(1), 1–10. https://doi.org/10.1080/00224065.2017.11918181
- Suen, C. Y., Chen, H., & Wu, C. F. J. (1997). Some identities on qn−m designs with application to minimum aberration designs. Annals of Statistics, 25(3), 1176–1188. https://doi.org/10.1214/aos/1069362743
- Sun, C. Y., & Tang, B. (2022). Relationship between orthogonal and baseline parameterizations and its applications to design constructions. Statistica Sinica, 32(1), 239–250.
- Taguchi, G. (1987). System of experimental design: Engineering methods to optimize quality and minimize costs. UNIPUB/Kraus International Publications.
- Tang, B., & Deng, L. Y. (1999). Minimum G2-aberration for nonregular fractional factorial designs. Annals of Statistics, 27(6), 1914–1926.
- Tang, B., & Wu, C. F. J. (1996). Characterization of minimum aberration 2n−k designs in terms of their complementary designs. Annals of Statistics, 27(6), 1914–1926.
- Wu, C. F. J. (2015). Post-Fisherian experimentation: From physical to virtual. Journal of the American Statistical Association, 110(510), 612–620. https://doi.org/10.1080/01621459.2014.914441
- Wu, C. F. J. (2018). A fresh look at effect aliasing and interactions: Some new wine in old bottles. Annals of the Institute of Statistical Mathematics, 70(2), 249–268. https://doi.org/10.1007/s10463-018-0646-0
- Wu, C. F. J., & Hamada, M. (2021). Experiments: Planning, analysis, and optimization (3rd ed.). Wiley Series.
- Xu, H. (2003). Minimum moment aberration for nonregular designs and supersaturated designs. Statistica Sinica, 13(3), 691–708.
- Xu, H., & Wu, C. F. J. (2001). Generalized minimum aberration for asymmetrical fractional factorial designs. Annals of Statistics, 29(2), 1066–1077.
- Yang, Y. H., & Speed, T. P. (2002). Design issues for cDNA microarray experiments. Nature Reviews Genetics, 3(8), 579–588. https://doi.org/10.1038/nrg863
Appendix. Proof of Theorem 4.1
In this proof, a traditional factorial effect is represented by a word, i.e. a subset of . For two words
and
, we define
to be
. Note that
, which is the sum of squared entries of
. Because the design is regular, each squared entry is either one or zero according to whether the corresponding effects are aliased.
Part (a) is evident from Tang and Deng (Citation1999) except that the number of factors considered in the computation is n−2 (exclude and
). So we have
.
For (b), let be the set of all words of length l not containing any word involving 1 and 3. Let
be a subset of
and 2 belongs to each word in
. Then
,
, is of the form
, where
is of length l−1. Similarly,
,
, is of the form
, where W is of length l. Similar argument can be made when the roles of
and
,
and
are interchanged, respectively. By the definition of
and
, we obtain
.
For (c), first consider and
, where W runs through all the words not involving
and has length l−1. It is equivalent to consider
and
for such W's. This yields
in
. Next we consider
and
, where
and W runs through all the words not involving
and has length l−1. By Tang and Deng (Citation1999), this yields
in
. Similar argument can be made when the roles of
and
,
and
are interchanged, respectively. By the definition of
and
, we obtain
.
For (d), first consider ,
,
and
, where W runs through all the words not involving
and has length l−1. It is equivalent to consider W,
,
and W for such W's. This yields
in
. Next consider
,
,
and
, where W runs through all the words not involving
and has length l−1. For such W's,
and
yield
in
;
and
yield
in
. Similar argument can be made when the roles of
and
,
and
are interchanged, respectively. By the definition of
, we obtain
.
For (e), first consider ,
,
,
and
,
,
,
, where W runs through all the words not involving
and has length l−2. This yields
in
. Next consider
,
,
,
for
. By Tang and Deng (Citation1999), this yields
. So we obtain
.
For (f), consider ,
,
,
for j = 1, 3, and
,
,
,
for
, where W runs through all the words not involving
and has length l−2. This yields
. So we obtain
.