Abstract
The last decade has witnessed a debate about the disruptive role of emerging digital technologies. At the heart of this debate is the issue of big data, which underpins the operations of these digital technologies but creates a series of new risks and issues for society and governments. New policy problems concerning data require formulators of new governance strategies and innovative policy designs. In this introduction to the special issue Data Policy and Governance, we examine scholarship on data governance and data policy, with a particular focus on emerging contributions on these topics. We also present the six articles that make up this special issue and indicate trends and future research directions from the discussion. This review demonstrates how data governance and policy are central to the digital world and require new designs and dynamics to deal with instruments, mixes, practices, and regulatory mechanisms.
1. Introduction – a journey to data governance and policy
Contemporary societies live in a context of hyper-connection to the Internet. The hyperconnection changes the way information and knowledge affect social environments, creating new governance dilemmas related to the digital world. And while these dilemmas may seem large, the possibility of using data to open various aspects of governments, society, and the economy through the use of big data and emerging digital technologies such as artificial intelligence, the Internet of Things (IoT), blockchain and platforms (Kitchin Citation2013; Mayer-Schönberger and Cukier Citation2013; Ekbia et al. Citation2014) draws attention at large scale. The pull of data-driven decision-making is almost too strong as societies have witnessed a process of re-engineering their institutions, both in the public and private sectors (Almeida, Filgueiras, and Mendonça Citation2022). At the heart of these social re-engineering are digital technologies and the commodification of massive data. As more and more entities subscribe to the commodification process, new social dilemmas and policy problems emerge, many of which directly affect people and their daily lives (Frischmann and Selinger Citation2018).
The movement to data-driven decision-making and data-driven instrumentation provides for increasing automation and customization of public services, changes in the cost-and-benefit ratio for governments and businesses, development of analytical capacities with the potential to improve policies and businesses, improve communication with society, and a variety of applications in different policy domains as health, social welfare, public security, education, among others (Filgueiras and Almeida Citation2021; Dunleavy and Margetts Citation2013). However, these digital technologies assume a disruptive character, changing the entire way of doing policy formulation (Giest Citation2017), organizing tasks and market decisions (Pasquale Citation2015), modifying all economic transactions, and creating new forms of political power (Culpepper and Thelen Citation2020). As such, these disruptive technologies bring a series of new risks to society, such as problems related to privacy (Bennett and Raab Citation2017, Sanfilippo et al., Citation2020), the possibility of new patterns of algorithmic injustice (Benjamin Citation2019; Eubanks Citation2018; Noble Citation2018), problems related to cybersecurity (Shackelford Citation2020).
Moreover, the emergence of digital disruptive technologies reshapes institutional aspects of governments, industries, and markets, implying a race for the development and application of these technologies (Filgueiras Citation2021). Disruptive digital technologies provide productivity gains and process optimization for organizations and convenience and personalization for consumers. The emerging digital world takes data as a central resource for the application of digital technologies as instruments to reengineering society (Frischmann and Selinger Citation2018). The development of these information and communication technologies depends on large volumes of data so that they can be applied and enable these new knowledge modes.
Technologies such as these produce innovations that result in a different way of doing and living. That is, they can have a disruptive potential when they produce economic, social, political, and cultural changes, implying consequences for human organizations. Disruptive technologies are those that produce radically different ways of doing and living (Christensen Citation1997). Disruptions imply diverse social consequences, changing many aspects of social capital and its structure (Fukuyama Citation2017).
Because disruptive technologies provide radical changes in ways of doing and living, it is essential that they disrupt public policies. Disruptions in public policy change the way problems or solutions are conceived, in turn shifting attention within a policy-making domain – mainly due to external shocks, but also changes in power alignments, perhaps through the cause of new ideas or actors (Jones and Baumgartner Citation2005). These disruptions are needed to create a broader framework for technology governance so that consequences and impacts can be controlled or minimized. Regulating disruptive technologies is not a simple activity. Technology regulation is based on various uncertainties and ambiguities, creating a governance pattern with difficulties in implementation and effectiveness (Taeihagh, Ramesh, and Howlett Citation2021).
Disruptive technologies – such as autonomous vehicles, autonomous weapons systems, blockchain technology, autonomous systems, cloud computing, and the Internet of Things (IoT) – have triggered changes that threaten existing socio-economic systems. The rapid beat of technological innovation poses serious challenges for governments, which must deal with the disruptive speed and scope of transformations taking place in many domains. While these technologies offer opportunities for improvements in economic efficiency and quality of life, they also generate many unintended consequences and pose new forms of risk (Li, Taeihagh, and De Jong Citation2018; Taeihagh and Lim Citation2019). In the entire architecture of governance and regulatory policies are the massive volumes of data, to identify organizational patterns necessary to collect, store, process and share data among different actors in society. As soon as different legal instruments have emerged with the aim of regulating the process of collecting, storing, processing, and sharing data, such as the European GDPR, the California Consumer Privacy Act (CCPA) in the United States, the General Data Protection Act of Brazil (LGPD). Other countries such as Canada, New Zealand and Argentina have well-established data protection laws since the early 2000s.
Government responses to these technologies must consider safety, privacy, and well-being, as well as protecting their livelihoods and health. However, regulating and governing these technologies is challenging due to the high levels of risk, ambiguities, and uncertainty associated with them (Li et al. Citation2018; Tan and Taeihagh Citation2021) and that often the beneficiaries of these technologies – the investors, producers, and users – they transfer risks to society or governments. In addition, the problem or lag between state regulatory agencies in responding to the challenges of these technologies further aggravates the situation (Marchant Citation2011). The convenience created by these technologies for consumers creates an informal alliance that establishes enormous political power for large technology companies, creating difficulties for governments to respond effectively to the risks of emerging technologies (Culpepper and Thelen Citation2020).
The regulation of emerging technologies is based on information asymmetries, political uncertainties, power dynamics, and failures in the policy design and government responses (Taeihagh, Ramesh, and Howlett Citation2021). For digital technologies, the collection, storage, processing and sharing of data is a necessary condition for the development and deployment and to produce public value. Digital technologies can improve processes, reduce costs, and expand society’s well-being. However, big data structures create a series of new governance dilemmas and new risks for society, similarly requiring disruption with respect to the data policy and new institutional frameworks for data governance.
Data policy and governance is an emerging field of study, with an important institutional innovation process to be addressed. Data governance is about authority and institutional designs with different instruments for dealing with data policy, taking on a dual objective: (1) how to protect citizens’ privacy and reduce risks for governments and companies regarding data management? (2) how, at the same time, to provide adequate mechanisms to guarantee data sharing and accelerate technological development? Data policy is linked to a tradeoff between the risks. On the one hand, the risk to citizen’s privacy, data leaks, problems with cybersecurity, for example. In the other hand, data is a strategic asset to governments and industry deploy economic and social development. Restricting access to data can hinder or inhibit economic development. It is due to this tradeoff that the data policy requires institutional frameworks that enable data governance, considering that data represent, in the contemporary world, a strategic resource for governments and companies.
This special issue we now present aims to answer some of these questions. What designs are required to protect the privacy and secure data? What governance arrangements are adequate to address contemporary dilemmas regarding big data? What can innovations in institutional and policy designs be produced to deal with the various issues concerning big data? In this first section, we make a brief overview about data governance and policy scholarship. The second section overviews the articles that make up this special issue. In the final section, we address trends and future research questions that emerge with changes in data governance.
2. State of data governance and policy scholarship
Data governance and data policy are emerging fields, soon marked by great attempts. The aim of this section is not to conduct a systematic review of the literature, but to review how scholarship on data governance and policy has been developed.
Consulting the Scopus database, articles with the term data governance in the title represent 453 articles published in different journals in different areas. Constituting the knowledge network composed of these articles, considering the keywords, we compose a network, as shown in below. The nodes of these networks are composed of the keywords information management, information systems, data quality management, and humans. Looking at , two questions are essential. First is the expected dominance of the Computer Science area in producing knowledge on data governance. Second, the variety of subjects around these nodes essentially deals with the constitution of data management systems and processes related to different topics and policy domains, such as health, justice, organizational models, decision-making, and agricultural production. In general, data governance is superimposed on information management processes.
Figure 1. Scientific knowledge network on data governance. Source: Scopus, consultation completed on November 20, 2022.
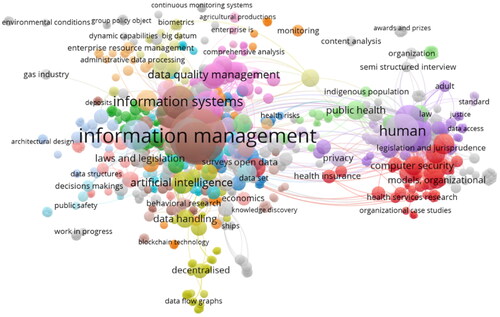
However, we need to consider how problems relating to data governance go beyond the specialized domain of Computer Science, requiring expertise in public policy and law. This interdisciplinary nature of data governance requires policy designs that provide practitioners with instruments, mixes, rules, norms, and adequate strategies to deal with the problem. Moreover, recent advances in data governance and data policy do not occur within issues related to available technologies but on what policies and institutional designs need to be tailored to face emerging problems with disruptive digital technologies. In summary, we need to produce disruptions in public policies and new institutional frameworks to deal with the wide range of problems in the digital world.
Returning to the Scopus database, when we use the expression data policy as a search factor, we can create a visualization that shows another type of network with a grid volume of points and nodes defined by the keywords open data, human or humans, one on data policy (). In the 167 articles in the Scopus base, some insights can be produced in this knowledge network. The first node concerns the problem of open data. Open data immediately connects to open governments, comprising policies that underpin transparency and accountability, availability of data to society, and the possibility for it to participate in the decision-making process. The second node concerns the policymaking keyword. This node connects to the decision-making process, public servants’ role, and technologies’ social effects. The third node concerns humans, data collection, publishing, and information dissemination. Finally, the fourth node is related to the data policy, containing issues such as data collection, data sharing, data access, data infrastructure, and data semantics. This fourth node is a point of deep interest. It is constitutive in the knowledge network that data policy is related to problems of data sharing, information management, and data access.
Figure 2. Scientific knowledge network on data policy. Source: Scopus, consultation completed on November 20, 2022.
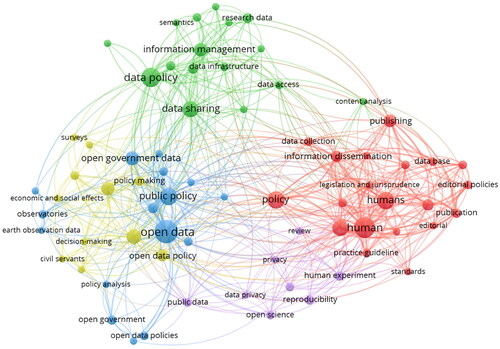
Data policy involves designs capable of connecting procedures, strategies, and rules in different legislations with instruments and mixes that make it possible to achieve policy objectives. Data policy is a policy domain that deals with the commodification of knowledge provided by public and private organizations in their business models. Data policy is about collective choices, focusing on general rules and principles that guide the actors in collecting, storing, processing, and sharing data, ensuring the appropriate use of data and information assets. This concept of data policy stems from the seminal work of Elinor Ostrom and Charlotte Hess, who detected this data commodification process as the central point for policies dealing with data and information (Hess and Ostrom Citation2007). Data policy includes actions for data quality, access, security, privacy, and usage, and possibilities to design technological applications that focus the data usage on the application in policy and services.
These knowledge networks reveal how we deal with two broad dimensions. Data governance implies distributing and redistributing resources and operational norms, creating responsibilities, and compliance with principles and norms. Data governance implies institutional frameworks that shape data policy. The data policy, on the other hand, means a set of actions prescribed to the actors to ensure the protection of data as resources, protect the citizens’ privacy and constitute adequate instruments to guide the actors’ behavior in the collection, processing and sharing data.
The purpose of these two graphs is not to produce a systematic review but to illustrate how data governance and data policy are diffuse themes based on different approaches, problems, and technological innovations. Data governance is about allocating authority and control over data and exercising such authority through decision-making in data-related matters. Data governance, therefore, is a disputed object that receives contributions from different areas, with the support of innovations shaped by governments, industry, and markets. Different institutional designs for data governance compose a set of very different organizational practices and strategies.
The design of data governance involves recognizing these different principles since the nature of the policy is regulatory and with clear procedures for data stewardship (Dawes Citation2010). Applying these principles can take place in different emerging institutional frameworks, such as data sharing pools, data cooperatives, public data trusts, and personal data sovereignty (Micheli et al. Citation2020). As applied by the city of Barcelona, for example, data pools are the constitution of platforms that expand access to data by bringing together data from different sources (Grossman et al. Citation2016). Data public trust is the institutional model of data governance based on the fiduciary duty of organizations that collect data and share it. This fiduciary duty organizes the relationship between the individual who has the personal data collected and the collectors (Delacroix and Lawrence Citation2019). Data cooperatives address societal challenges and produce data policy focusing on justice and fairness conditions to value production (Borkin Citation2019). Finally, personal data sovereignty is focused on data subjects’ self-determination (Ilves and Osimo Citation2019). These data governance models frame different action situations that shape data policy and organize power relations (Micheli et al. Citation2020).
From different modes of governance, policies are formulated and implemented concerning different issues and problems. For example, open data policies imply different challenges for transparency and privacy. Big open linked data enables governments to analyze individual behaviors, expand possibilities for designing policies expanding control, directly affecting citizens’ privacy, and creating new policy problems. Nevertheless, on the other hand, big open-linked data enables governments to increase transparency and openness (Janssen and Van den Hoven Citation2015).
Data policy is toward ambiguous issues, depending on the advancement of technologies or institutional governance designs. The gap in the literature lies in identifying policy designs that may reflect institutional governance parameters for different data-based technologies. This connection is essential and, in many ways, involves a trial-and-error approach to more adaptive institutions (Kuhlmann, Stegmaier, and Konrad Citation2019). Identifying these policy designs in practical cases of digital technologies can add different approaches to problems that emerge with disruptions in technological development. As an emerging field of public policy, data governance and policy can benefit from the analysis of different policy designs and alternatives found to deal with data and the diversity of problems surrounding it.
3. Special issue overview
The articles that compose this special issue have a fascinating thematic diversity, which deals with different topics related to data governance and data policy. The special issue comprises a variety of possibilities for the use of data, as well as governance issues and challenges. The special issue’s focus is to produce practical knowledge about the various challenges involved in data policy and governance design, considering the link between these two dimensions.
The first article, written by Andrew B. Withford and Jeff Yates, discusses an essential element built into many data governance initiatives, and disruptions. Disruptions increasingly involve the challenge for governments, industry, and various market actors to access, gather, analyze, and employ information about citizens. The emergence of different designs for protecting citizens’ data and privacy requires consensus from citizens so that governments, industry, and markets can process their data. Consensus is a fundamental practice for actors to modulate their actions based on emerging citizenship rights. Notice and choice pose many challenges for practitioners, who must reconcile these citizenship rights with the competing interests of actors. Thus, notice and choice emerge as essential instrument in data protection and privacy design. However, it challenges practitioners because it implies mechanisms of nudging and coevolving technological business. Accompanying these co-evolutions implies challenging designs to meet privacy and data protection objectives in the contemporary world. The article by Withford and Yates enables the construction of different insights for designs that deal with the challenge of coevolving technologies and policy disruptions.
The second article, by Zhizhao Li, Yuqing Guo, Masaru Yarime, Xun Wu, analyzes the regulatory challenges implicit in disruptive technologies based on the case of the use of facial recognition technologies (FRTs) in China. These challenges imply policy designs that focus on safeguarding privacy and data security. These policy designs that emerge from the objective of data privacy and security require care in dealing with disruptive technologies, demanding innovative forms of regulatory governance. Challenges such as safeguarding privacy and data security require more flexible and adaptive forms of governance, considering innovative institutional frameworks. Among these innovations, adopting regulatory sandboxes that can anticipate problems and test solutions is an essential key to the adaptive governance of emerging technologies. The regulatory sandbox is an innovative approach to regulation to obtain first-hand information regarding cutting-edge technologies and how to intervene more effectively at key technological stages with regulatory actions. Regulatory sandboxes are adopted from a more adaptive perspective of governance. Sandboxes establish forms of engagement for different stakeholders and new policy mixes that are more adapted to solve different problems.
The third article, by Fernando Filgueiras and Lizandro Lui, deals with the institutional construction of data governance, taking the case of Brazil. Internally to the Brazilian federal government, there are institutional challenges for the design of data policies. Brazil has different characteristics. The Brazilian government is one of the most prominent collectors of data in society, which are stored in different repositories, relying on public companies that store and process data for different federal government organizations. To design technological development strategies, the Brazilian federal government sharing data with different governmental and private actors is essential. In addition, there is a framework for data protection and privacy in Brazil, implemented with the General Data Protection Act (LGPD) and another set of specific laws. These institutional frameworks shape the process of collecting, storing, processing, and sharing data. The Brazilian government set up a Central Data Governance Committee, bringing together representatives from the center of government to formulate the data policy, covering the entire data sharing process between different government organizations and between the government and the private sector. The article analyzes policy design dynamics within the Brazilian federal government, showing how many decisions taken in the Central Data Governance Committee tend to be path dependent. Path dependence materializes in creating barriers to data sharing, regardless of the authorizations inscribed in rules such as the LGPD.
The fourth article by Si Ying Tan, Araz Taeihagh, and Devyani Pande analyzes the barriers to data sharing by taking the case of Singapore. The emergence of autonomous systems requires deepening data sharing for them to carry out their different operations. Therefore, data sharing is essential for developing disruptive technologies with varied applications in the public and private sectors. According to Taeihagh, there are six barriers to data sharing. First, technical barriers arise from the unavailability of capabilities to facilitate data sharing. Second, motivational barriers are related to the beliefs of people dealing with data sharing. Third, economic barriers are related to the lack of technical resources and economic damage. Fourth, the political barriers concerning distrust between users and providers restricting sharing. Fifth is the legal barriers imposed on data ownership, privacy protection, and copyrights. Finally, ethical barriers are related to the principles that organize the digital world and its practices. Therefore, the development of data sharing initiatives requires policy designs that allow overcoming the barriers, as is the case in Singapore. These policy designs must consider the practice of regulatory sandboxes for data sharing, the establishment of partnerships between governments and the private sector, and analytical capabilities that allow advancing the development of disruptive digital technologies. The involvement of the private sector is essential to overcome the motivational barriers of the actors, as well as the constitution of analytical capacities that allow a framework of knowledge that consolidates ethical and legal analyzes to overcoming these barriers.
In the fifth article by Gleb Papyshev and Masaru Yarime, they analyze 31 national strategies for artificial intelligence around the globe. Through textual analysis, based on qualitative content analysis and Latent Dirichlet Allocation (LDA) topic modeling, the authors build a typology of national strategies for artificial intelligence, comprising three strategic models. First, a development model, typical in East Asian countries, considers designs related to the state’s direct involvement in the development of AI innovation. The state assumes the developer role and deploys policy designs that enable more direct support to human capital formation, research and development, financing structures, regulation, data sharing, and support to the private sector. The second type relates to control strategies typical of European Union countries. Control involves developing AI regulations that enable designs to establish a more direct role for the state in technological development and regulatory governance strategies. Finally, the third type is related to a promotion perspective. This third type is typical in the United States, United Kingdom, and Ireland, where the state creates in its strategies a governance arrangement aimed at promoting the private sector in technological development. These three types of national strategies for artificial intelligence comprise different designs, where policy objectives and policy mixes vary depending on broader understandings of the state’s role in AI innovation.
Finally, the sixth article, by Mansi Babbar, Shruti Agrawal, Dilshad Hossain, and M Mustahid Husain, analyzes how the lack of accountable digital regulation in India and Bangladesh regarding the adoption of public health-related digital technologies during the COVID-19 pandemic produced institutional void. Institutional void is how the state removes forms of control and privacy protection considered unnecessary to face crises, such as the case of the COVID-19 pandemic. The pandemic has enabled, as in the cases of India and Bangladesh, the emergence of different digital technologies that reinforce the surveillance process and, in turn, governmentality. The article brings insights into how crises can imply new power relations based on algorithmic governmentality without regulations. The article presents a series of recommendations for practitioners to deal with the challenge of regulating disruptive technologies, focusing on privacy protection and data safeguarding.
All papers in this special issue deal with challenges related to the design of data policy and governance. Governments face the challenge of producing answers to the problems that emerge with disruptive digital technologies. In particular, the challenge of data governance and the design of policies are central in the digital world. The policy designs aimed at a set of mixes, approaches, institutional frameworks, and grammars that operate different mechanisms which make it possible to govern data as essential resources of twenty-first-century societies. Practical recommendations can be extracted and shared in all papers, with the aim of steering policies focused on data and its contemporary challenges.
4. Challenges and trends in design data governance and policy – future research directions
Considering the lessons learned from this special issue, governments should monitor the institutional formats by which data governance is shaped and the policies related to the collection, storage, processing, and sharing of data. In addition, governments must understand the designs involved in different issues related to data protection and citizen privacy and define strategies to deal with emerging technologies, such as artificial intelligence.
In all these situations, governments must promote design dynamics that are more adaptive and flexible concerning technological development. For example, dealing with the issue of data concerning the Internet of Things is essential to think about the problems related to the collection and sharing facilitated by this technology. As for artificial intelligence, the central problem is the qualification of data, elimination of biases, and design and validation of algorithms. Data governance and policy design dynamics thus require adaptive capacities to engage stakeholders, strengthen transparency and accountability mechanisms, and coordinate mechanisms capable of managing conflicts and promoting forms of collaboration and cooperation (Filgueiras and Almeida Citation2021).
Adaptive capacities are defined as “the ability of a resource governance system to first alter processes and if required to convert structural elements as [a] response to experienced or expected changes in [the] societal or natural environment” (Pahl-Wostl Citation2009, 355). Adaptive capacities are enhanced when organizations can (1) provide information; (2) deal with conflict; (3) induce rule compliance; (4) provide infrastructure; and (5) be prepared for change (Dietz, Ostrom, and Stern Citation2003). In many situations, according to Dietz, Ostrom, and Stern (Dietz, Ostrom, and Stern Citation2003), adaptive governance concerns situations of rapid change, demanding designs that encourage experimentation, learning, and policy change. In this case, adaptive capacity implemented in data governance and policy requires partnerships between governments, industry, and the market, to solidify an institutional design in which, despite the advancement of technologies, institutions easily adapt to the new context, generating stability and policy robustness.
Future research agenda on data governance and policy demands this process of experimentation and learning, in which practitioners can design different solutions to problems that emerge at the same speed as technological disruptions. Thus, the policy design for emerging digital technologies, with data as central resources to be explored in the digital world, demands adaptation processes and institutional and organizational flexibility, while it requires robustness and effectiveness to generate knowledge and learning. Therefore, data governance and policy research must document different initiatives and institutional designs, considering the diversity of competing interests of actors and requirements of principles that should guide digital development.
Supplemental Material
Download Zip (380 KB)Disclosure statement
No potential conflict of interest was reported by the author(s).
Additional information
Funding
References
- Almeida, V., F. Filgueiras, and R. Mendonça. 2022. “Algorithms and Institutions: How Social Sciences Can Contribute to Governance of Algorithms.” IEEE Internet Computing 26 (2): 42–46. doi:10.1109/MIC.2022.3147923.
- Bennett, C. J., and C. D. Raab. 2017. The Governance of Privacy: Policy Instruments in Global Perspective. Cambridge: MIT Press.
- Benjamin, R. 2019. “Assessing Risk, Automating Racism.” Science 366 (6464): 421–422. doi:10.1126/science.aaz3873.
- Borkin, S. 2019. Platform Cooperatives. Solving the Capital Conundrum. Report, NESTA and Co-Operatives. London, UK: Nesta and Co-operatives UK.
- Christensen, C. M. 1997. The Innovator’s Dilemma: When New Technologies Cause Great Firms to Fail. Boston, MA: Harvard Business School Press.
- Culpepper, P., and K. Thelen. 2020. “Are We All Amazon Primed? Consumer and Politics of Platform Power.” Comparative Political Studies 53 (2): 288–318. doi:10.1177/0010414019852687.
- Dawes, S.S. 2010. “Stewardship and Usefulness: Policy Principles for Information-Based Transparency.” Government Information Quarterly, 27 (4): 377–383. doi:10.1016/j.giq.2010.07.001
- Delacroix, S., and N. D. Lawrence. 2019. “Bottom-up Data Trusts: Disturbing the ‘One Size Fits All’ Approach to Data Governance.” International Data Privacy Law 9 (4): 236–252. doi:10.1093/idpl/ipz014.
- Dietz, T., E. Ostrom, and P. C. Stern. 2003. “The Struggle to Govern the Commons.” Science 302 (5652): 1907–1912. doi:10.1126/science.1091015.
- Dunleavy, P., and H. Margetts. 2013. “The Second Wave of Digital-Era Governance: A Quasi-Paradigm for Government on the Web.” Philosophical Transactions of the Real Society 371 (1987): 1–17. doi:10.1098/rsta.2012.0382.
- Ekbia, H. M., Mattioli, I., Kouper, G., Arave, A., Ghazinejad A., Bowman, V. R., Suri, A., et al. 2014. “Big Data, Bigger Dilemmas: A Critical Review.” Advances in Information Science 66 (8): 1523–1545. doi:10.1002/asi.23294.
- Eubanks, V. 2018. Automating Inequality. How High-Tech Tools Profile, Police, and Punish the Poor. New York: St. Martin’s Press.
- Filgueiras, F., and V. Almeida. 2021. Governance for the Digital World: Neither More State Nor More Market. London: Palgrave.
- Filgueiras, F. 2021. “Artificial intelligence policy regimes: Comparing Politics and Policy to National Strategies for Artificial Intelligence”. Global Perspectives, 3 (1): 3236. doi:10.1525/gp.2022.32362
- Frischmann, B., and E. Selinger. 2018. Re-Engineering Humanity. Cambridge: Cambridge University Press.
- Fukuyama, F. 2017. The Great Disruption. Human Nature and the Reconstitution of Social Order. New York: Free Press.
- Giest, S. 2017. “Big Data for Policymaking: Fad or FastTrack?” Policy Sciences 50 (3): 367–382. doi:10.1007/s11077-017-9293-1.
- Grossman, R., A. Heath, M. Murphy, M. Patterson, and W. Wells. 2016. “A Case for Data Commons: Toward Data Science as a Service.” Computing in Science & Engineering 18 (5): 10–20. doi:10.1109/MCSE.2016.92.
- Hess, C., and E. Ostrom. 2007. Understanding Knowledge as a Commons. Cambridge: MIT Press.
- Ilves, L. K., & Osimo, D. 2019. A roadmap for a fair data economy. Helsinki, Finland: Policy Brief, Sitra and the Lisbon Council.
- Janssen, M., and J. Van den Hoven. 2015. “Big and Open Linked Data (BOLD) in Government: A Challenge to Transparency and Privacy?” Government Information Quarterly 32 (4): 363–368. doi:10.1016/j.giq.2015.11.007.
- Jones, B. D., and F. R. Baumgartner. 2005. The Politics of Attention: How Government Prioritizes Problems. Chicago: The University of Chicago Press.
- Kitchin, R. 2013. “Big Data and Human Geography: Opportunities, Challenges, and Risks.” Dialogues in Human Geography 3 (3): 262–267. doi:10.1177/2043820613513388.
- Kuhlmann, S., P. Stegmaier, and K. Konrad. 2019. “The Tentative Governance of Emerging Science and Technology – A Conceptual Introduction.” Research Policy 48 (5): 1091–1097. doi:10.1016/j.respol.2019.01.006.
- Li, Y., A. Taeihagh, and M. De Jong. 2018. “The Governance of Risks in Ridesharing: A Revelatory Case from Singapore.” Energies 11 (5): 1277. doi:10.3390/en11051277.
- Marchant, G. E. 2011. “Addressing the Pacing Problem.” The Growing Gap between Emerging Technologies and Legal-Ethical Oversight. Dordrecht: Springer.
- Mayer-Schönberger, V., and K. Cukier. 2013. Big Data: A Revolution That Will Transform How We Live, Work and Think. Boston: Eamon Dolan/Houghton Mifflin Harcourt.
- Micheli, M., M. Ponti, M. Craglia, and A. B. Suman. 2020. “Emerging Models of Data Governance in the Age of Datafication.” Big Data & Society 7 (2): 205395172094808–205395172094815. doi:10.1177/2053951720948087.
- Noble, S. U. 2018. Algorithms of Oppression. How Search Engines Reinforce Racism. New York: New York University Press.
- Pahl-Wostl, C. 2009. “A Conceptual Framework for Analysing Adaptative Capacity and Multi-Level Learning Process in Resource Governance Regimes.” Global Environmental Change 19 (3): 354–365. doi:10.1016/j.gloenvcha.2009.06.001.
- Pasquale, F. 2015. “The Black Box Society.” The Secret Algorithms That Control Money and Information. Cambridge: Harvard University Press.
- Sanfilippo, M. R., Y. Shvartzshnaider, I. Reyes, H. Nissenbaum, and S. Egelman. 2020. “Disaster Privacy/Privacy Disaster.” Journal of the Association for Information Science and Technology 71 (9): 1002–1014. doi:10.1002/asi.24353.
- Shackelford, S. 2020. Governing New Frontiers in the Information Age: Toward a Cyber Peace. Cambridge, UK: Cambridge University Press.
- Taeihagh, A., M. Ramesh, and M. Howlett. 2021. “Assessing the Regulatory Challenges of Emerging Disruptive Technologies.” Regulation & Governance 15 (4): 1009–1019. doi:10.1111/rego.12392.
- Taeihagh, A. and Lim H.S.M. (2019). “Governing Autonomous Vehicles: Emerging Responses for Safety, Liability, Privacy, Cybersecurity, and Industry Risks.” Transport Reviews, 39 (1): 103–128. doi:10.1080/01441647.2018.149464
- Tan, S. Y., and A. Taeihagh. 2021. “Governing the Adoption of Robotics and Autonomous Systems in Long-Term Care in Singapore.” Policy and Society 40 (2): 211–231. doi:10.1080/14494035.2020.1782627.