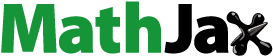
Abstract
In this study, we define the fractional random variable. The concept of convergence in fractional probability, almost surely convergence and some related theorems and examples are studied with the purpose of expanding the fractional probability theory parallel to the classical one. It is shown that almost surely convergence in the fractional probability space does not lead to the convergence in fractional probability. And, some valuable features related to fractional probability theory such as Cauchy function in fractional probability are discussed. We proved that a fractional random variable converges in fractional probability if it is Cauchy in fractional probability. Finally, the well-known 0-1 Kolmogorov theorem is proved in a fractional probability space.
PUBLIC INTEREST STATEMENT
Fractional calculus including the subjects, derivative, integration and Taylor’s series of fractional order plays an essential role in a number of fields of application such as the stochastic mechanics and fractional differential and integral equations. Probability density of fractional order has been raised by Guy Jumarie in 2007, which has application in the stochastic differential equation. Thus, expanding the mentioned concept by Guy Jumarie to the other fractional probability concepts such as fractional probability space, measure and convergence was so interesting. In this paper, we discussed some important definitions and theorems of the fractional probability theory and compared them with the ones of the classical probability theory.
1. Introduction
We try to continue the concept of fractional probability calculus, based on the study by Jumarie (Citation2007) which defines probability dens ity of fractional order and fractional moments by using fractional calculus (Jumarie, Citation2007). Our paper is in the continuation of the paper by Mostafaei and Ahmadi Ghotbi (Citation2010) in which the fractional probability space , the fractional probability measure
,
and invalidity of the classical probability measure continuity theorem,
for the fractional probability measure
have been explained (Mostafaei & Ahmadi Ghotbi, Citation2010). There is a great literature on fractional calculus, fractional derivatives and fractional integration (Jumarie, Citation2006; Kober, Citation1940; Miller & Ross, Citation1973; Nishimoto, Citation1989; Oldham & Spanier, Citation1974; Ross, Citation1974; Samko, Kilbas, & Marichev, Citation1987). Fractional probability distribution is defined by the measure
. Combining this with the definition of the fractional Taylor`s series
, obtained by modified fractional Riemann–Liouville derivatives, leads to the definition of the probability density of fractional order
. Using fractional calculus, Jumarie (Citation2007) defined the probability density of fractional order
,
as the following:
Definition 1 (Probability density of fractional order ).
Let denote a real-valued random variable with the probability density
, where
.
is referred to as a random variable with fractional probability density of order
,
, whenever one has
with normalizing condition . Also, he introduced the kth fractional moment as (Jumarie, Citation2007)
These definitions can be considered as the first step in expanding a fractional probability theory. Furthermore, Mostafaei and Ahmadi Ghotbi (Citation2010) introduced the fractional probability measure and the fractional probability space
as the following:
Definition 2 (Fractional probability principles).
Given a sample space and an associated
-field
, the fractional probability measure of order
,
, is a set function
that satisfies
1.
2.
3.
Definition 3 (Fractional probability space).
A fractional probability space is a triple where
is the sample space corresponding to outcomes of some of the experiments.
is the
-algebra of subsets of
. These subsets are called events.
is a fractional probability measure.
Theorem 1 (Fractional probability measure properties).
Let be a fractional probability space, then one has
(a)
(b) If are two events that
, then
(c) (Mostafaei & Ahmadi Ghotbi, Citation2010).
Now, according to the difference between fractional and classical probability space, it would be interesting to know what happen to the essential probabilistic concepts such as convergence. It is desirable to know whether major theorems in classical probability theory are satisfied in fractional probability space. These would be first steps to expand a fractional probability theory which has application in the fractional statistical mechanics and the fractional diffusion equation (Liang & Chen, Citation2015; Liang et al., Citation2017; Liang, Chen, & Magin, Citation2016), parallel to the classical probability theory.
In this paper, the definition of fractional random variable is denoted and some well-known convergence theorems or lemmas such as almost surely convergence are verified in fractional probability space. Furthermore, regarding the classical definition of the convergence in probability
the convergence in fractional probability
is defined. Also, the relation between the almost surely convergence
in fractional probability space and the convergence in fractional probability
is verified. Finally, the validity of the important 0-1 Kolmogorov theorem in the fractional probability space
is proved.
2. Fractional random variable
A random variable is a measurable function that forms the sample space
to
;
That is the inverse image of any Borel set -measurable (Pasha, Citation2007; Resnik, Citation1998):
In other words, random variable is a function by which inverse projection of any Borel set is an event. But, being random variable is not a natural feature of functions, so a fractional random variable is only expressed when a fractional probability measure is defined. That is, after defining fractional probability measure for a random variable, that random variable is called a fractional random variable. Properties of random variables are satisfied for fractional random variables. For example, let denote two fractional random variables on
. Then, by using transformation of fractional probability density, fractional probability density function of the random variable
is obtained. Therefore,
is a fractional random variable.
3. Almost surely convergence in fractional probability space
Assume that is a specific property of sample space. However, there is a possibility that all members of sample space
do not have this property. Then, it is said that this property is almost surely satisfied when, first, those points that do not have the property are events, and second, the probability of the event is zero. In other words, we can state that
is almost surely satisfied if
. [9, 10]
Now we can claim that in the fractional case is almost surely satisfied if
. That is, fractional probability of the event
(the points that do not have the property
) is zero. For example, suppose we are given a
and
,
.
Now, if fractional random variable on
is set as
Then, the fractional random variable in all points of
, except the point
, is zero. According to the mathematical analysis concepts, we cannot say the function
is zero. On the other hand, fractional probability of the point, in which the function
is not zero, is zero,
. Then
. Since fractional random variables are a specific branch of the classical random variables and they are classified as functions, therefore, we can discuss their convergence.
Definition 5: pointwise convergence.
Suppose that is a sequence of fractional random variable on fractional probability space
. The sequence
of random variables is called pointwise convergence when numerical sequence
is convergence for any
. Certainly, value of
depends on
, so the value of
, for any
, is obtained in
. Therefore, we have a function such as
from
to
(
) that is defined in the point
by the relation
, which itself is a fractional random variable.
If is the pointwise limit of
, then we have
. Or,
. So, the definition of pointwise convergence for fractional random variables is exactly the same as the classical one in the probability theory. If for the set of points in which
(a sequence of fractional random variables on fractional probability space
) is not convergent, the fractional probability is zero, then we can state that
is almost surely convergent to
and we have
or
. As we already know, if
, then
, hence, almost surely convergence is also called convergence with a probability of one. Now in fractional probability space if
, then
. Therefore, almost surely convergence, in a fractional probability space, is also called convergence with fractional probability one, because as we know
, so if we define
, then
where .
So,
According to the first condition of fractional probability principle,
So,
Therefore,
Remark 1.Suppose that we are given the fractional probability space . If
, then
.
Example 2.Let be a fractional probability space in which fractional probability function is defined by the expression
where is the uniform probability density of fractional order
on
. The sequence of functions
on
is defined as
For any ,
is a random variable and also it is a fractional random variable. Because this random variable is defined in the fractional probability space
, we have
Because does not tend to zero only in
and the fractional probability of this point is zero, so
.
In the classical probability calculus, it has been proved as a lemma that if and only if for any
,
. Now by giving an example it is illustrated that this lemma is not satisfied in the fractional probability calculus. That means we cannot claim that
if and only if for any
,
.
Example 3.Suppose is a fractional probability space and we have
. The sequence of functions
on
is defined as follows:
So for ,
,
is a fractional random variable and we have
Since only in
does not tend to zero and the fractional probability of this point is zero,
and
.
Now we prove that , for any
. As we know
, so
According to the fractional probability function ,
,
is obtained as follows:
Whereas variation amplitude of is the interval
,
varies between 0 and
. That is
. So, for obtaining
, for any
, we have
and so on. Therefore, we have
Therefore,
According to the continuity of fractional probability functions, the equality is not satisfied. So, in spite of
, we cannot claim that
Therefore, it is concluded that the lemma, if and only if for any
,
is not satisfied in fractional probability space.
4. Convergence in fractional probability
Definition 6.The sequence of random
variables converges to a random
variable
in fractional probability, written as
, if for any
Almost sure convergence in fractional probability space does not imply convergence in fractional probability. By giving the following example we demonstrate that in the fractional probability space we cannot prove that if , then
.
Example 4. Suppose is a fractional probability space and
The sequence of functions on
is defined as the following:
So that for ,
,
is a random
variable and we have
Now we need to show that . By using fractional calculus and transformation of fractional probability density, the fractional probability density of
is obtained as the following. The sequence of functions
on
is set as
. For any
,
is a fractional random variable or random
variable, as follows:
Based on the fractional probability density of ,
and using transformation of fractional probability density, fractional probability function (fractional probability density) of the random
variable
,
is calculated as below:
Since , one has
So, , which leads to
.
So, almost sure convergence in does not imply convergence in fractional probability.
To show that the convergence in fractional probability does not imply almost sure convergence in fractional probability space we have the following example.
Example 5.Suppose ,
and
is the fractional probability measure that is denoted by
. The sequence of random variables
,
is defined as
And the sequence of random variables is defined as
It is going to be outlined that in fractional probability is convergent to zero (
) but
is not almost surely convergent. First it is noticed that
s have the values zero or one. So suppose that
is arbitrary then
on one of the subintervals
is
. Therefore, for any
there is a point in the sample space in which
. So
and
. However, the fractional probability of this point in which
is not zero but is
.
On the other hand, ’s index is in the form of
that
. If
, then
. As a result,
Therefore,
So, we have . Thus, convergence in fractional probability does not imply almost sure convergence in
.
Theorem 2.Suppose that are random
variables.
converges in fractional probability if
is Cauchy in fractional probability. Cauchy in fractional probability means
or more precisely, given any
, there exists
such that for all
we have
Proof.It is illustrated that if then
is Cauchy in fractional probability. For any
,
Thus, taking fractional probabilities, we have
If , for any
. Then,
, for any
.
5. 0-1 Kolmogorov theorem in fractional probability space
Suppose is a sequence of fractional random variables. We define the following
algebras
Theorem 3 (0-1 Kolmogorov theorem in fractional probability space). Suppose is a sequence of independent variables. So, for any
, we have
Or
.
Proof. Let for any
. So, for any
and for any
and for any
, we have
. This equality is also satisfied for any
and for any
and for any
. We assume
. So, according to the classical probability theory,
has the following properties:
is closed under finite intersection,
Precisely
consists of
.
D also has another class of properties as following
Consists of
.
is closed under difference operation.
is closed under increasing and countable union of their members.
According to these properties and some theorems of classical probability theory, it is proved that . So, for any
and for any
, we have
But, . Therefore, by assuming
in above equality, we would have
And, it is concluded that any member of has the probability equal to zero or one.
6. Concluding remarks
In this study we have proposed a new section in fractional probability theory named as “convergences in the fractional probability space ” to expand a probability theory of fractional order completely parallel to the classical probability theory. It would also be of interest to study some other probabilistic concepts and theorems in the fractional probability space such as Hilbert space and
convergence for the fractional probability measure
.
Additional information
Funding
Notes on contributors
Ahmad Zendehdel
Ahmad Zendedel received his BSc in Statistics from Ferdowsi University, Mashhad, Iran in 1991 and MSc in Statistics from the Shahid Beheshti University of Tehran, Iran in 1995 and PhD in Applied Statistics from Islamic Azad University, Tehran, Iran in 2004. Since 1995, he is working as a member of Full-time Mathematics and Statistics Department, Neyshabour, Iran. At present, he is an assistant professor in Islamic Azad University (IAU). He is interested in the Applied Statistics, especially: Financial Statistics.
Parisa Ahmadi Ghotbi
Parisa Ahmadi Ghotbi has Bachelor of Science in Statistics from Azad University of Mashhad, Iran in 2005 and Masters of Science in Statistics from Azad University of Tehran, Iran in 2009. At present, she is studying PhD of Science in Probability at International Unit of Shiraz University, Iran from 2011. Her Doctoral Thesis is “Steiltjes sample characteristic function estimation procedure”. And, her second research area is probability calculus of fractional order.
References
- Jumarie, G. (2006). Modified Riemann-Liouville derivative and fractional Taylor series of on-differentiable functions further results. Computers & Mathematics with Applications, 51, 1367–1376. doi:10.1016/j.camwa.2006.02.001
- Jumarie, G. (2007). Probability calculus of fractional order and fractional Taylor’s series application to Fokker-Planck equation and information of non-random functions. Chaos: Solitons and Fractals.
- Kober, H. (1940). On fractional integrals and derivatives. Quart Journal Mathematical Oxford, 11, 193–211.
- Liang, Y., & Chen, W. (2015). A cumulative entropy method for distribution recognition of model error. Physica A: Statistical Mechanics and Its Applications, 419, 729. doi:10.1016/j.physa.2014.10.077
- Liang, Y., Chen, W., Akpa, B. S., Neuberger, T., Webb, A. G., & Magin, R. L. (2017). Using spectral and cumulative spectral entropy to classify anomalous diffusion in Sephadex™ gels. Computers & Mathematics with Applications, 73(5), 765–774. doi:10.1016/j.camwa.2016.12.028
- Liang, Y., Chen, W., & Magin, R. L. (2016). Connecting complexity with spectral entropy using the Laplace transformed solution to the fractional diffusion equation. Physica A: Statistical Mechanics and Its Applications, 453, 327. doi:10.1016/j.physa.2016.02.056
- Miller, K. S., & Ross, B. (1973). An introduction to the fractional calculus and fractional differential equations. New York, NY: Wily.
- Mostafaei, H., & Ahmadi Ghotbi, P. (2010). Fractional probability measure and its properties. Journal of Sciences, Islamic Republic of Iran, 21(3), 259-264.
- Nishimoto, K. (1989). Fractional calculus. Koroyama: Descartes Press.
- Oldham, K. B., & Spanier, J. (1974). The fractional calculus. Theory and application of differentiation and integration to arbitrary order. New York, NY: Acadenic Press.
- Pasha, E. (2007). Probability theory, Persian language. Tehran: Publisher Teacher Training University Iran.
- Resnik, S. I. (1998). A probability path. Boston: Birkhauser.
- Ross, B. (1974). Fractional calculus and its applications. Lecture notes in mathematics, Vol. 457. Berlin: Springer.
- Samko, S. G., Kilbas, A. A., & Marichev, O. I. (1987). Fractional integrals and derivatives. Theory and applications. London: Gordon and Breach Science Publishers.