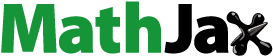
Abstract
In this article, we have derived a new distribution named as Rayleigh–Rayleigh distribution (RRD) motivated by the transformed transformer technique by Alzaatreh, Lee, and Famoye (2013). The statistical properties of RRD, comprising of explicit expressions for quantile function, moments, moment generating function, mean deviation, skewness, kurtosis, reliability measures, measures of uncertainty, distributions of order statistics and L moments have been derived. Parameter estimation is carried out using method of maximum-likelihood estimation and Fisher information matrix is derived. The flexibility of the new distribution is assessed by applying it to four real data sets. The comparative behavior of RRD with Rayleigh distribution, Generalized Rayleigh distribution, Exponentiated Rayleigh distribution, Weibull Rayleigh distribution and Alpha Power Rayleigh distribution provided the evidence that it outperforms the other competing distributions.
PUBLIC INTEREST STATEMENT
One of the basic tools of statistics is to modeling the real-life phenomenon in the form of statistical distributions. Adding parameters in the existing distribution and combining two or more existing distributions are two common techniques used for the generalization of a distribution. These generalized distributions accommodate the changing circumstances and complexities of real life. In this study, we have extended the Rayleigh distribution by adding the parameter of the other Rayleigh distribution. The resultant distribution contained two parameters and is called Rayleigh–Rayleigh distribution (RRD). Simulation study revealed that estimators of the parameters are asymptotically unbiased and efficient. The application of RRD to four real data sets exhibited the more flexibility of RRD as compared to some other life time distributions. It is hoped that the proposed distribution will attract the attention of the researcher in the fields of life sciences, physical sciences and social sciences.
1. Introduction
Uncertainties and risks are two main realities of real-life phenomena. Probability theory is used to handle these uncertainties and modeling of these real-life phenomena. Due to variation, complexities and diversities in real life, a large number of statistical distributions are derived. Still there are many important problems where the real-life data do not follow any standard probability distributions. This leads to the extensions and development of generalized statistical distributions.
In literature numerous generalized distributions have been developed with common feature of having more parameters. Induction of parameters in existing distribution improves the goodness of fit of the distribution under study and tail properties of a distribution increases.
Rayleigh distribution (RD) introduced by Lord Rayleigh in 1880 plays a crucial role in modelling and analyzing life time data such as project effort loadings modelling, life testing experiments, reliability analysis, communication theory, physical sciences, engineering, medical imaging science, applied statistics and clinical studies. Let the random variable X has RD with scale parameter, i.e. X
RD (x;
. Then the probability density function (PDF) and the cumulative density function (CDF) of RD are defined as
Due to the importance of Raleigh distribution in a variety of fields, a wide range of extensions of Raleigh Distribution has been established. Kundu and Raqab (Citation2005) proposed the generalized RD and its unknown parameters are estimated by using different estimation methods. Abd Elfattah, Hassan, and Ziedan (Citation2006) studied the estimation of unknown parameter of RD in the presence of different censoring sampling schemes. Voda (Citation2007) proposed the new generalization of RD by using conservability approach. In this technique the PDF of generalized distribution can be obtained as, where
and
are PDF and finite mean of positive continuous random variable. Dey (Citation2009) derived the Bayes estimators for the parameter of RD by using square error and LINEX loss functions. Merovci (Citation2013) developed the transmuted RD by using quadratic rank transmutation technique. Merovci (Citation2014) proposed the transmuted generalized RD and describe its mathematical properties. Merovci and Elbatal (Citation2015) studied the Weibull RD. Mahmoud and Ghazal (Citation2017) deliberated the parameters estimation of exponentiated Rayleigh based on type II censored data.
In this article we have derived the generalization of the RD named as Rayleigh–Rayleigh distribution (RRD) using Transformed Transformer technique proposed by Alzaatreh, Lee, and Famoye (Citation2013). Main motivation of this study is to generate a new distribution by adding scale parameter in the RD, so that the performance of generalized distribution becomes better than the original one.
According to Transformed Transformer method the function form of CDF of a random variable is used to transform PDF of other random variable into a new distribution. Let T be a continuous random variable with PDF and CDF and
, respectively, for
. Let X is another random variable with PDF
and CDF
. W [
] is a functional form of CDF of the random variable X defined on the support of random variable T, it is differentiable and monotonically non- decreasing function and when
then
and when
then
. The PDF of random variable T is transformed into the function of random variable X through the transformer W [
]. The new generalized family of distribution is called the T-X family of distribution. Let
be the CDF and PDF of generalized family of distributions, respectively, then
By taking different functional forms of , large number of generalized families of distributions can be formed. For discrete random variable X, discrete families of distributions can be derived. T-Geometric family of discrete distributions is proposed by Alzaatreh, Lee, and Famoye (Citation2012).
Consider the support of random variable T is [0,), then the functional form of
may be defined as (-log [1-
]) which satisfied all the above three conditions. The CDF of new generalized distribution is defined as
and the PDF is
In the literature, a large number of generalized families of distributions has been derived and studied by using Transformed-Transformer technique, e.g. T-geometric family of discrete distributions by Alzaatreh et al. (Citation2012), T-normal family by Alzaatreh, Lee, and Famoye (Citation2014), Logistic-X by Tahir, Cordeiro, Alzaatreh, Mansoor, and Zubair (Citation2016), Weibull-G by Tahir et al. (Citation2016), Gompertz-G family of distribution by Alizadeh, Cordeiro, Pinho, and Ghosh (Citation2017), etc.
The rest of the paper is organized as follows. Section 2 presents the derivation of RRD along with shape of its PDF and CDF. Reliability analysis is studied with the shapes of survival and hazard rate function in Section 3. In Section 4, statistical properties like quantile function, moments and moment generating function, skewness and kurtosis are investigated. Section 5 present the Shannon and Re’nyi entropies. Order statistics and L-moments are derived in Section 6. Maximum-likelihood estimators and information matrix are defined in Section 7. In Section 8 simulation study is carried out to examine performance of the maximum-likelihood estimators of parameters of RRD. In Section 9, four real-life data sets are considered to examine the application of RRD in real-life phenomena and comparison of proposed distribution with parent and other existing distributions. Finally, the study is concluded in Section 10.
2. Rayleigh–Rayleigh distribution
In this Section, an RRD is derived. Let random variable T follows RD having PDF given in expression (1) with scale parameter. The functional form of
is defined as (–log [1–
]) depending upon the support of Rayleigh random variate T. Then the PDF of Rayleigh-X family of distributions is given as
and the corresponding CDF is defined as
By taking different distributions of random variable X, a large number of Rayleigh-X distributions can be obtained such as Rayleigh–Gamma, Raleigh–Pareto, Rayleigh–Gumbel, Rayleigh-Exponential, etc. In our study, we assumed that X is another Rayleigh variate with scale parameter i.e. X~ RD (x;
. By substituting the value of
(x) and
define in (1) and (2) having parameter
in expression (5) the PDF of the RRD is obtained as
The CDF of RRD is
By adding a scale parameter in the base line distribution, the generalized distribution is expected to be more flexible to model complicated real-life phenomena than the original one.
Figures and illustrate some of the possible shapes of the PDF and CDF of RRD for some selected values of the parameters β and σ. The PDF plots in Figure reveal that the RRD is unimodel, increasing and decreasing.
3. Reliability analysis
The reliability function focuses the probability of an event for a specific time without failing the event. CDF and
are reverse of each other. The
for RRD is defined as
For various values of and
, the reliability function is monotonically decreasing which is shown in Figure .
The ratio of PDF and reliability function is hazard rate function. That is another specification in reliability analysis. The hazard rate function for the NGR distribution is given as
The plots of hazard rate function are exponentially increasing which is shown in Figure .
4. Statistical properties
In this section, we have derived the statistical properties of the RRD, specifically quantile function, random number generator, moments, moment generating function, skewness, kurtosis and mean deviation.
4.1. Quantile function and simulation
Here and hereafter let the random variable “X” follows RRD with parametersand
i.e. X
RRD (x;
. The quantile function corresponding to the CDF of RRD is
Median, first and third quartiles of RRD can conveniently derived by substituting , respectively.
The RR random variate can easily be simulated by taking U as a uniform random variate on the unit interval. By using the technique proposed by Alzaatreh et al. (Citation2013) the random X is generated as
4.2. Moments and moment generating function
Moments are necessary and important in any statistical analysis, especially in applications. It can be used to study the most important features and characteristics of the distribution e.g. central tendency, dispersion, skewness and kurtosis.
4.2.1. rth Moment
By definition the rth moment about origin of “X” is
For, 2, 3 and 4, the first four non-central moments of NGR distribution are specified as
4.2.2. Negative moments
The negative moment generating function is defined as
For the random variable of RRD
4.2.3. Moment generating function
The moment generating function of “X” is
4.2.4. Characteristic function
The characteristic function of random variable X is defined as
4.3. Skewness and kurtosis
The coefficient of Skewness and kurtosis of RRD is given as
Hence, RRD is platykurtic and negatively skewed distribution.
4.4. Mean deviation
The mean deviation of “X” is
5. Measure of uncertainty
Entropy measures the dynamical uncertainty of the probability distribution, unpredictability of the state or disorder of a system … …
5.1. Shannon entropy
Shannon (1948) proposed the idea of entropy. The Shannon entropy of “X” is defined as
Where is the Euler constant and its value is 0.5772.
5.2. Renyi Entropy
The generalized form of Shannon entropy is Rnyi entropy, proposed by R
nyi (1961). The R
nyi Entropy of “X”, denoted by
can be defined as
6. Order statistics
For the sake of data analysis relating to quality control, reliability, hydrological and extreme values, order statistics and moments of order statistics play a starring role. In this Section, we have derived the PDFs of the kth order, maximum and minimum order statistics from the RRD.
6.1. The PDF of the smallest order statistic
Let is the first order statistics from random sample
from RRD. The PDF of
is defined as
6.2. The PDF of the largest order statistic
For the order statistics of the sample drawn from RRD, the PDF of the largest order statistics is given as
;
6.3. The joint PDF of ith and jth order statistics
Let the joint pdf of the and
order statistics is denoted by
then using the standard formula this can be derived as
6.4. L-moments
In statistics, conventional moments have a great importance to describe the shape of a distribution but provide inadequate performance in case of extreme values due to the sensitivity to extreme observations. Moreover, the conventional moments are asymptotically inefficient for fat tails distributions. In such a situation many empirical studies shows that the L-moments, the linear combination of ordered statistics outperform the conventional moments. Like the conventional moments, the estimation process using the population L-moments and sample L-moments of a distribution can be carried out. The measures of skewness and kurtosis derived in term of L-moments are named as L-skewness and L-kurtosis, respectively.
In this study, the L-moments of X have been derived through the probability weighted moments (PWM) and this method was introduced by Hosking (Citation1990). The PWM denoted by are given below
; r = 0, 1, 2, 3 …
The rth L-Moment denoted by is the linear combination of PW moments. The first four L-moments of X are
=
Consequently the L-Skewness of RRD is
and the L-Kurtosis of RRD is
7. Maximum-likelihood estimation and Fisher information matrix
Due to possessing the asymptotic properties of normality and efficiency, the maximum-likelihood estimators have greater importance in statistical inference. In this Section, maximum-likelihood estimators of the parameters of RRD have been derived. Let X1, X2, X3, …, Xn be a random sample from XRRD(x;
. Then the likelihood function of the observed sample is given as
and the corresponding log likelihood function is
By applying the rule of maximum-likelihood estimation, expression (17) is partially differentiated with respect to and
and equating to zero, the corresponding normal equations are given as
The expression (18) and (19) cannot be solved analytically. R package is used to solve them numerically by using Newton–Raphson method.
To obtain the Fisher’s information matrix (FIM), the second derivatives of the log likelihood function are derived as
As the MLE are asymptotically unbiased and normally distributed with its variance covariance matrix obtained from the inverse of FIM. Hence, the interval estimation and hypothesis testing of the model parameters can easily be applied.
8. Simulation study
In this Section, simulation study has been carried out to check the performance of the estimators. Using the R statistical package 5,000 replications of sample sizes n = 50, 100, 150, 200, 300 and 400 have been generated from RRD by using the random number generator given in expression (9). Four different combinations of values of the actual parameters are taken. The average ML estimates (MLEAV.) of the parameters along with average bias (BIASAV.) and average root mean square error ( are reported in Tables –.
Table 1. MLE’s, average bias and average root mean square error at β = 1.5, 2, 2.5, 3 and σ = 1.5
Table 2. MLE’s, average bias and average root mean square error σ = 1, 1.25, 1.5, 1.75 and β = 2
Table 3. MLE’s, average bias and average root mean square error for σ = 2.5, 3, 3.5, 4 and β = 1.5, 2, 2.5, 3
Table 4. MLE’s, average bias and average root mean square error σ = 1.5, 2.5, 3.5, 4.5 and β = 1.5, 2.5, 3.5, 4.5
The results showing in Tables – for all the combinations of four sets of values of parameters, mentioned above, demonstrate that average bias for both the parameters appear negative which indicates that the estimators are under estimated and value of bias approaches to zero by increasing sample size. Hence, estimators of the parameters of RRD are asymptotically unbiased. These maximum-likelihood estimates remains under estimated by varying the values of both parameters. Values of average root mean square error decrease by increasing the sample size indicating that the estimators are asymptotically efficient. There is no effect on value of root mean square error by increasing or decreasing the values of parameters. Both of the evidences show that maximum-likelihood estimators of the parameters of RRD perform well and estimates are precise and accurate.
9. Real-life applications
In this Section, we have explored the comparative performance of RRD with five existing distributions: RD by Lord Rayleigh (1880); Generalized Rayleigh distribution (GRD) by Kundu and Raqab (Citation2005); Exponentiated Rayleigh distribution (ERD) by (Mahmoud and Ghazal (Citation2017); Weibull Rayleigh distribution (WRD) by Merovci and Elbatal (Citation2015); Alpha Power Rayleigh distribution (APRD) by Malik and Ahmed (2017).
The comparison is carried out by taking the following four real data sets:
Lifetime data set of the 46 patients survival times (in years) to given treatment of chemotherapy already used by Bekker, Roux, and Mosteit (Citation2000) and Fundi, Njenga, and Keitany (Citation2017)
Data set about the strengths of 1.5 cm glass fibers that is measured at the National Physical Laboratory in England used by Smith and Naylor (Citation1987).
Real-life data set about the breaking stress of carbon fibers of 50 length (GPA) already used by Cordeiro and Lemonte (Cordeiro & Lemonte, Citation2011), Al-Aqtash, Lee (Al-Aqtash, Lee, & Famoye, Citation2014) .
Real-life data set about the wind speed of Elanora Heights. In November 2007, average wind speeds (in meter/sec) is used by Best, Rayner, & Thas (Citation2010).
The data sets and their sources can be seen in the respective references. Hereafter we will call the above data sets as Dataset1, Dataset 2, Dataset 3 and Dataset 4, respectively.
For the comparison of the distributions, the goodness of fit criteria used are −2lnL, Akaike information criterion (AIC) by Akaike (Citation1974), Consistent Akaike information criterion (CAIC) by Bozdogan (Citation1987), and Bayesian information criterion (BIC) by Schwarz (Citation1978) and Hannan-Quinn Information Criterion (HQIC) by Hannan and Quinn (Citation1979). AIC estimates the performance of a model while comparing with other models. CAIC provide a consistent and asymptotically unbiased estimate of order of the true model. HQIC is a consistent model selection criterion. The distribution with smaller values of −2lnL, AIC, BIC, CAIC and HQIC is considered as the best distribution. The specifications of these criteria are as follows:
,
,
where k = number of estimated parameters in the distribution
= maximized log likelihood of the distribution under consideration
n = total number of observations
The estimated parameter of the distributions under consideration along with the values of goodness fit criteria for Dataset1, Dataset2, Dataset3 and Dataset4 are given in Table –, respectively.
Table 5. ML estimates with goodness-of-fit criteria for Dataset 1
Table 6. ML estimates with goodness-of-fit criteria for Dataset2
Table 7. ML estimates with goodness-of-fit criteria for Dataset3
Table 8. ML estimates with goodness-of-fit criteria for Dataset4
The results given in Table – show that the values of −2lnL, AIC, BIC, CAIC and HQIC are smallest for NGRD as compared to the other distributions under consideration. The above results strongly lead to recommend that our proposed distribution outperforms the RD, GRD, ERD, WRD and APRD for the selected data sets.
Hence, for given data sets RRD is chosen as the best fitted model than the competitors models.
10. Conclusion
In this study, RD is successfully generalized by adding one-scale parameter from other RD. Explicit expression of probability density and cumulative distribution function are derived. Behavior of parameters is checked by PDF and CDF plots. Comprehensive studies of the statistical properties of the new distribution have been presented. The reliability behavior of RRD is investigated by varying the values of the parameters. Order statistics, distribution of the order statistics and L-moments are also derived. The estimation of the parameters is performed through maximum-likelihood approach. Results of simulation study shows that maximum-likelihood estimators of proposed model are asymptotically unbiased and root mean square error reduces by increasing the sample size. The application of the suggested distribution to four real-life data exhibited that RRD outperformed some other existing distributions. In all the four datasets proposed RRD performs better than original RD. Hence, the induction of one or more parameter improves the performance of a distribution. It is hoped that the proposed distribution will attract the attention of researchers and practitioners in the fields of physical sciences, biological sciences, actuarial studies and social sciences.
Additional information
Funding
Notes on contributors
Kahkashan Ateeq
Kahkashan Ateeq is an assistant professor in the Department of Statistics, The Women University Multan and Ph.D. scholar at Bahauddin Zakariya University Multan. Her area of interest is distribution theory and Bayesian analysis.
Tahira Bano Qasim
Dr Tahira Bano Qasim is assistant professor in the Department of Statistics, The Women University Multan. She earned her M.Sc., M.phil and Ph.D. degrees in Statistics from Bahauddin Zakariya University, Multan. Her research interests lie in the field of distribution theory and time series modeling with the issue of Conditional Heteroscedasticity. She has three publications in different journals.
Ayesha Rehman Alvi
Ayesha Rehman Alvi is an M.Phil Student at The Women University Multan. Her research interests lie in the field of Distribution Theory and Applications. Present paper is a portion of her M.Phil thesis under the supervision of Dr. Tahira Bano Qasim and Co-Supervision of Kahkashan Ateeq.
References
- Abd Elfattah, A., Hassan, A. S., & Ziedan, D. (2006). Efficiency of maximum likelihood estimators under different censored sampling schemes for Rayleigh distribution. Interstat.
- Akaike, H. (1974). A new look at the statistical model identification. Selected Papers of Hirotugu Akaike: Springer, 215–16.
- Al-Aqtash, R., Lee, C., & Famoye, F. (2014). Gumbel-Weibull distribution: Properties and applications. Journal of Modern Applied Statistical Methods, 13, 11. doi:10.22237/jmasm/1414815000
- Alizadeh, M., Cordeiro, G. M., Pinho, L. G. B., & Ghosh, I. (2017). The Gompertz-G family of distributions. Journal of Statistical Theory and Practice, 11, 179–207. doi:10.1080/15598608.2016.1267668
- Alzaatreh, A., Lee, C., & Famoye, F. (2012). On the discrete analogues of continuous distributions. Statistical Methodology, 9, 589–603. doi:10.1016/j.stamet.2012.03.003
- Alzaatreh, A., Lee, C., & Famoye, F. (2013). A new method for generating families of continuous distributions. Metron, 71, 63–79. doi:10.1007/s40300-013-0007-y
- Alzaatreh, A., Lee, C., & Famoye, F. (2014). T-normal family of distributions: A new approach to generalize the normal distribution. Journal of Statistical Distributions and Applications, 1, 16. doi:10.1186/2195-5832-1-16
- Bekker, A., Roux, J., & Mosteit, P. (2000). A generalization of the compound Rayleigh distribution: Using a Bayesian method on cancer survival times. Communications in Statistics-Theory and Methods, 29, 1419–1433. doi:10.1080/03610920008832554
- Best, D. J., Rayner, J. C., & Thas, O. (2010). Easily applied tests of fit for the Rayleigh distribution. Sankhya B, 72, 254–263. doi:10.1007/s13571-011-0011-2
- Bozdogan, H. (1987). Model selection and Akaike’s information criterion (AIC): The general theory and its analytical extensions. Psychometrika, 52, 345–370. doi:10.1007/BF02294361
- Cordeiro, G. M., & Lemonte, A. J. (2011). The β-Birnbaum–Saunders distribution: An improved distribution for fatigue life modeling. Computational Statistics & Data Analysis, 55, 1445–1461. doi:10.1016/j.csda.2010.10.007
- Dey, S. (2009). Comparison of Bayes estimators of the parameter and reliability function for Rayleigh distribution under different loss functions. Malaysian Journal of Mathematical Sciences, 3.
- Fundi, M. D., Njenga, E. G., & Keitany, K. G. (2017). Estimation of parameters of the two-parameter Rayleigh distribution based on progressive Type-II censoring using maximum likelihood method via the NR and the EM algorithms. American Journal of Theoretical and Applied Statistics, 6, 1–9. doi:10.11648/j.ajtas.20170601.11
- Hannan, E. J., & Quinn, B. G. (1979). The determination of the order of an autoregression. Journal of the Royal Statistical Society: Series B (Methodological), 41, 190–195.
- Hosking, J. R. (1990). L-moments: Analysis and estimation of distributions using linear combinations of order statistics. Journal of the Royal Statistical Society Series B (Methodological), 52, 105–124. doi:10.1111/rssb.1990.52.issue-1
- Kundu, D., & Raqab, M. Z. (2005). Generalized Rayleigh distribution: Different methods of estimations. Computational Statistics & Data Analysis, 49, 187–200. doi:10.1016/j.csda.2004.05.008
- Mahmoud, M., & Ghazal, M. (2017). Estimations from the exponentiated Rayleigh distribution based on generalized Type-II hybrid censored data. Journal of the Egyptian Mathematical Society, 25, 71–78. doi:10.1016/j.joems.2016.06.008
- Merovci, F. (2013). Transmuted Rayleigh distribution. Austrian Journal of Statistics, 42, 21–31. doi:10.17713/ajs.v42i1.163
- Merovci, F. (2014). Transmuted generalized Rayleigh distribution. Journal of Statistics Applications & Probability, 3, 9. doi:10.18576/jsap/030102
- Merovci, F., & Elbatal, I. (2015). Weibull Rayleigh distribution: Theory and applications. Applied Mathematics & Information Sciences, 9, 2127.
- Schwarz, G. (1978). Estimating the dimension of a model. The Annals of Statistics, 6, 461–464. doi:10.1214/aos/1176344136
- Smith, R. L., & Naylor, J. (1987). A comparison of maximum likelihood and Bayesian estimators for the three-parameter Weibull distribution. Applied Statistics, 358–369. doi:10.2307/2347795
- Tahir, M., Cordeiro, G. M., Alzaatreh, A., Mansoor, M., & Zubair, M. (2016). The Logistic-X family of distributions and its applications. Communications in Statistics-Theory and Methods, 45, 7326–7349. doi:10.1080/03610926.2014.980516
- Tahir, M., Zubair, M., Mansoor, M., Cordeiro, G. M., Alizadeh, M., & Hamedani, G. (2016). A new Weibull-G family of distributions. Hacettepe Journal of Mathematics and Statistics.
- Voda, V. G. (2007). A new generalization of Rayleigh distribution. Reliability: Theory & Applications, 2.