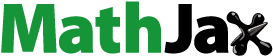
Abstract
The continuous wavelet transform of Schwartz tempered distributions is investigated and derive the corresponding wavelet inversion formula (valid modulo a constant-tempered distribution) interpreting convergence in . But uniqueness theorem for the present wavelet inversion formula is valid for the space
obtained by filtering (deleting) (i) all non-zero constant distributions from the space
, (ii) all non-zero constants that appear with a distribution as a union. As an example, in considering the distribution
we would omit 1 and retain only
. The wavelet kernel under consideration for determining the wavelet transform are those wavelets whose all the moments are non-zero. As an example,
is such a wavelet.
is an arbitrary constant. There exist many other classes of such wavelets. In our analysis, we do not use a wavelet kernel having any of its moments zero.
Mathematics Subject Classification:
PUBLIC INTEREST STATEMENT
In the present work, the authors studied the continuous wavelet transform to Schwartz tempered distributions and found its inversion formula. This theory is useful for many researchers who are doing research work in image processing and signal processing. Researchers will be advantageous, who are using different types of integral transforms. The aforesaid theory is applicable, where many differential equations can be solved by exploiting the theory of Fourier transform. This work can be played an important role to study different types of integral equations. So the approach of this research paper is multidisciplinary in nature, which are applicable in mathematics, physics, and engineerings.
1. Background results
The Schwartz testing function space of rapid descent consists of infinitely differentiable functions
defined on
such that
for each .
The topology on is generated by two-parameter family of separating collection of seminorms
The topology of can as well be generated by the separating collection of the one-parameter family of seminorms
It has been proved by Zemanian (Citation1965, p. 111) that the topology generated by the sequence of seminorms (1.1) on is the same as that generated by the sequence of seminorms (1.2). It has also been proved by him that
(Zemanian, Citation1965, pp. 111–112), i.e.
So a sequence of functions in
converges to a function
if and only if
for each . For example, the sequence of functions
as
in the topology of
. Here
stands for the identically zero function in
.
We say that a sequence in
is a Cauchy sequence in
if
as
for each
. The topology on
is defined by the countable set of pseudonorms given by (1.2) and with respect to this topology,
is sequentially complete (Yosida, Citation1995; Zemanian, Citation1965, Citation1968).
The result stated in the following paragraph is well known and can be found in many books (Gelfand & Shilov, Citation1968, pp. 21–23; Robewicz, Citation1972), p.21. We however state these facts to make the reading of this paper easy and interesting for many readers who may not know this result.
The space is obviously metricize by the metric
defined by
The fact that is a metric is proved by using the fact that the function
, is an increasing function of
. It is well known that the topology generated by the metric
on
is the same as that generated by the sequence of seminorms (1.2). Since the locally convex topological vector space
is complete and metrizable, it is a Frechet space.
Definition 1.
A function is said to be a window function if
(Boggess & Narcowich, Citation2001; Chui, Citation1992).
It is proved in (Chui, Citation1992) that this window function also belongs to
. A more general result in
-dimensions is proved in (Pandey & Upadhyay, Citation2015).
(1.4)
Definition 2. A function is called a basic wavelet if the following admissibility condition is satisfied
from (Chui, Citation1992; Daubechies, Citation1990; Lebedeva & Postinikov, Citation2014; Postnikov, Lebedeva, & Lavrova, Citation2016).
We denote the constant defined in (1.4) by . Here,
is the Fourier transform of
which is given as
From Definition 2 it follows that the function is a basic wavelet belonging to
. This is because
is the Fourier transform of
Therefore,
, which is bounded. The theorem stated below helps us in constructing wavelets in various testing function spaces very simply.
Theorem 1.1. A window function is a basic wavelet if and only if
. A more general theorem in
-dimensions,
is proved in (Pandey & Upadhyay, Citation2015). Since
is a window function, an element
is a basic wavelet if and only if
So
is a basic wavelet in . For a similar reason, a function
is a basic wavelet in
if and only if
So the function
is a basic wavelet in
. We have already verified this fact by direct calculation in the paragraph preceding Theorem 1.1.
2. Introduction
Let be the Schwartz testing function space of rapid descent and let
be a subspace of
so that every element
satisfies
, i.e., every element of
is a basic wavelet. The subspace
of
is equipped with the topology induced by
on
. One can verify that the restriction of
to
is in
and, therefore, in the following discussion the wavelet inversion formula that is valid for
restricted to
modulo a constant distribution, is also valid for elements of
restricted to
. More clearly, we have
We extend the continuous wavelet transform to the Schwartz tempered distribution space , exploiting the structure formula
Here and
, and
is a function belonging to
depending upon
and not on
. The structure formula
follows from the boundedness property of
, i.e., for
there exists a nonnegative integer m and a constant
such that
This is derived by virtue of the fact that and the method of contradiction; using (2.2) we get for a non-negative integer
satisfying
Therefore,
Now, using the Hahn Banach theorem (Yosida, Citation1995, p. 102, 105, 106), can be extended to
. Since
is dense in
, this extension is unique.
By Riesz theorem, the dual of is homeomorphic to
we get a function
such that
from (Akhiezer & Glazman, Citation1961, p. 33). This justifies the structure formula (2.1).
(2.3) Fact 1: If is a wavelet belonging to
, the continuous wavelet transform
of
in view of the relation
can be proved to be
Using the classical wavelet inversion formula for functions as proved in (Boggess & Narcowich, Citation2001; Chui, Citation1992; Daubechies, Citation1990; Lebedeva & Postinikov, Citation2014), we will prove in the next section that
in the weak topology of , i.e.,
Since two tempered distributions having the same continuous wavelet transform may differ by a constant distribution, our inversion formula will be valid modulo a constant distribution.The structure formula for reduces a functional analytic problem to a classical problem of analysis, i.e. a
function theory.
Pathak (Pathak, Citation2004) extended the wavelet transform to Schwartz tempered distributions in the year 2004 using the method of adjoints, i.e.
but he did not prove an inversion formula. Here stands for the generalized wavelet transform of
the dual of
(Pathak, Citation2004, Citation2009, Chapter III).
He defined the test function space containing the Schwartz testing function space
whose topology is generated by a sequence of semi-norms
,
and
, (Pathak, Citation2004, p. 413).
From (2.3) it follows that the wavelet transform of a constant distribution is zero as the wavelet belonging to the space
satisfies the condition
Therefore, the wavelet transform of a constant distribution is
Thus, two wavelets having the same wavelet transform may differ by a constant.
Pathak (Citation2004) was motivated to give the definition (2.3) for the wavelet transform of tempered distribution by the Parseval’s type of relation for the wavelet transform
He strengthened his result (definition) 2.2 (Pathak, Citation2004) further by proving some continuity results and boundedness property; but he did not derive the corresponding wavelet inversion formula. Since the wavelet transform of a constant distribution is zero the uniqueness theorem for the wavelet inversion formula will not be true; it will be valid modulo a constant distribution. In order that the uniqueness theorem may be valid we have to delete all non-zero constants distribution from the space . In addition, we have to delete a non-zero constant distribution from a tempered distribution which is contained in it as a sum or difference. For example, in considering the distribution
We delete the constant 1 and retain the tempered distribution only.
The space filtered this way is represented by the symbol
, then the uniqueness theorem for the wavelet inversion formula will be valid for this space
.
During the last five years several good results on the continuous wavelet transform of functions appeared. Notable amongst them is the work of Postnikov et al. (Citation2016), Lebedeva & Postinikov (Citation2014), who proved the wavelet inversion formula for functions in the year 2016 without a requirement of the admissibility condition.
Weisz (Citation2013) proved the norm and a.e. convergence of inversion formula in and Wiener amalgam spaces. In 2014 he proved the inverse wavelet transform to summability means of Fourier transforms and obtained norm and almost everywhere convergence of the inversion formula for functions from the
and Wiener amalgam spaces (Weisz, Citation2014). In 2015, Weisz (Citation2015) also proved, using the summability methods of Fourier transform, norm convergence and convergence at Lebesgue points of the inverse wavelet transform for functions from the
and Wiener amalgam spaces.
Our objective is to extend the continuous wavelet transform to Schwartz space and prove an inversion formula modulo a constant distribution and then extend the uniqueness theorem for the continuous wavelet transform of distributions to the space
; the space
is a subspace of the space
.
Our spaces and
are big spaces and they contain the spaces
,
as considered by these authors.
The wavelets that we use as a kernel of the wavelet transform will not be any element of will be those elements of
whose moments of any order will be non-zero. An example of one such wavelet is
. Many more such wavelets can be constructed by assigning arbitrary values to the constant
in the expression
. Another set of such wavelet kernels can be constructed by assigning appropriate values to the constants
and
in the expression
. We first select
such that
. The number
will be independent of
and therefore
can be assigned arbitarary real values, thereby proving the existence of wavelet kernels in
whose any moment will be non-zero. Many more such wavelets (unaccountably many of them) can be constructed.
Our reason to avoid wavelets whose every moment is zero is that wavelet transform of every polynomial function will be zero, and our inversion formula will break. This situation is already dealt with by Holschneider (Citation1995). He quotients out the space of tempered distributions by the space of all polylnomials.
2.1. Comparison of our results with that of Pathak
Pathak (Citation2004) followed the method of adjoints, whereas we have followed the method of embedding to define the wavelet transform of tempered distributions; but he did not prove the inversion formula.
Pathak took
whereas we took
,
, a more general result in this sense.
We have proven the inversion formula for the wavelet transform of distributions giving the situation where our inversion formula has unique results and where it does not.
Calculation of the wavelet transform is far easier by our method whereas calculation of the wavelet transform by Pathak’s method is not quite as easy.
We have proven the uniqueness theorem for the inversion formula for the wavelet transform for the space
,
and the result can be extended for
.
Our objective is to prove the wavelet inversion formula for tempered distributions in the weak distributional sense and this will be accomplished in Chapter 3.
3. An integral wavelet transforms of schwartz tempered distributions in 
and its inversion
3.1. Integral wavelet transform
In this section we will use three symbols ,
and F; the symbols
and F stand for the Fourier transform of functions of
and
respectively, and the symbol
stands for the admissibility constant which is defined in (1.4).
We require that be finite as in the derivation of the wavelet inversion formula. The expression
appears in the denominator of the related expression, that is useful in our derivation of the inversion formula using the Fourier transform technique. But there exists an alternative reconstruction formula for the continuous wavelet transform, which is applicable even if the admissibility condition is violated see (Holschneider, Citation1995; Postnikov et al., Citation2016).
Fact 2: Let be a subspace of
such that
implies
and so
is a basic wavelet. It is a simple exercise to show that
also belongs to
as a function of
for fixed
and
. Therefore, for
, the integral wavelet transform
of
is defined as
Since any constant distribution in can be identified as a zero distribution, the uniqueness theorem for the wavelet inversion formula in
is valid.
Theorem 3.1. Let be a subspace of the Schwartz testing function space
of rapid descent such that every
satisfies the condition
Then is a basic wavelet, i.e., it satisfies the conditions
Proof. Note that (i) is trivially satisfied and (ii) follows as by taking
in (Pandey & Upadhyay, Citation2015, Theorem 3.1.).
Theorem 3.2. Let and
where
and
are spaces of functions as defined in Theorem 3.1. Then
Proof. In (Pandey & Upadhyay, Citation2015, Theorem 3.1) the proof of above theorem is given.
Theorem 3.3. Let , and
then
where denotes the admissibility constant (1.4).
Proof. It is a special case of (Pandey & Upadhyay, Citation2015, Theorem 3.1) see also (Daubechies, Citation1990).
Theorem 3.4. For , define the continuous wavelet transform or integral wavelet transform
of
as
Then ,
, as a function of
, belongs to
.
Proof. Using (2.1) for and
we have
where . Now,
for fixed and
;
. Therefore, replacing
by
in (3.1), we have
Now, by Plancherel’s formula we get
The function . Hence the expression in the above integral which is in curly bracket is bounded. Therefore, the coefficient of
in the integrand in the above integral belongs to
as a function of
; which implies that the above integral as a function of
belongs to
. Similarly, we can show that
as a function of and is infinitely differentiable with respect to
and each of its derivatives also belongs to
.
Therefore, as a function of
,
. The form of the structure formula we have chosen is valid for
. In fact, the structure formula for
when
can also be derived from (2.1) by setting
.
Corollary. Let be the function defined in theorem 3.4, then
belong to
as a function of
for each
Theorem 3.5. [Inversion formula]: For and
, define the Wavelet transform
of
as defined in Theorem 3.4. Then
(i)
(ii).
(iii) ,
and
are uniformly bounded in a compact neighbourhood of the point
,
.
(iv) is a continuous function of
everywhere on
except possibly at
(the -axis).
(3.2) Proof. Using the structure formula (2.1) for we have
Therefore
Therefore, using a standard result in analysis we have
A similar result for differentiation with respect to can be proved. Therefore, we obtain
The results (3.3 and 3.4) are valid for .
(iii) .
Using the boundedness property of we get,
where is a polynomial of degree
. The non-negative integer
is the least possible value conforming to the boundedness property of
. These polynomials will be uniformly bounded in a compact neighborhood of
. Since
,
will be also finite. Therefore,
is bounded in a compact neighborhood of
,
. Similar bounds can be established for the first partial derivatives of
with respect to
and
.
(iv) To prove (iv), we assume (i) and (ii) for , and (iii). Now
This is valid if is real-valued. If it is complex-valued, then we apply the mean value theorem of differential calculus separately for the real and imaginary part of
.
The first partial derivatives of are bounded in a compact neighborhood of
where
and
lies in the neighborhood of
during the limiting process. Therefore, from (3.6)
Note that differentiality results proved by us apply to the wavelet transform of tempered distributions, whereas Pathak’s differentiality results apply to the wavelet transform of functions. Our results are a lot more general (Pathak, Citation2004).
(3.7) Theorem 3.6 [Inversion Formula]: Let be a tempered distribution belonging to
and
, and define
of
with respect to the wavelet
by
Then following inversion formula holds
Above limit is interpreted in the weak distributional sense, i.e. in .
or
When we use a structure formula for , the distributional problem is converted into the classical one and so all lower limits and upper limits of the integral will be
and
, respectively.
(3.9) Proof. From (2.1), (3.7) can be written as
Our aim to find the inversion formula
interpreting convergence in the weak topology of , i.e., as in (3.8)
For the sake of convenience, let us represent
In the following integrations we delete the region and make changes in the order of integration and after that let
. Therefore, in view of (3.6) and Theorems 3.4 and 3.5, the integral in (3.11) is meaningful (it exists) and now when operated against
, (3.11) becomes:
[The above angular brackets represent integration with respect to in
].
Therefore, we have
as
so that
using integration by parts, note that the integral of the terms enclosed in the curly brackets with respect to as a function of
is infinitely differentiable and belongs to
for each
. So to evaluate the integral term we use integration by parts with the limit terms being zero.
In Equations (3.11)–(3.13) we could have taken finite limits of integration ,
and then after integration by parts let
; the same results as shown above would have been obtained. Thus, there is no error involved in setting the lower and upper limits of the foregoing integrals as
and
. We also make use of the fact that
Finally by distributional differentiation, (3.13) and (3.14) become
If we express the expressions in (3.15) as a fourfold iterated integral by removing the angular brackets, the two expressions will be fourfold iterated integrals in the order . We wish to express them in the order
by switching the order of integrations. We cannot apply the Fubini-Tonelli theorem (Yosida, Citation1995), p.18 at this stage as none of the above iterated integrals is absolutely convergent. We therefore proceed as follows to apply Fubini’s theorem to switch the order of integration. We number the above integrands in (3.15)
and
Let K be a compact set of the “ XBAT-space” given by
Then the fourfold iterated integrals of the integrands (3.16) and (3.17) by (Yosida, Citation1995), p.18, with respect to the measure are absolutely convergent over compact set
and so are integrable. Therefore, switches in the order of integration over
can be done in 4! ways and all these 4! iterated integrals of integrands (3.16) and (3.17) are equal in view of Fubini’s theorem. Our concern for the time being is the equality of the fourfold iterated integrals
and
of the above mentioned integrands over the compact set
, which is valid in view of Fubini’s theorem. We now let
and
all tend to
and then we let
; the fact that the fourfold iterated infinite integrals
of integrands (3.16) and (3.17) are now convergent is proved by using the Plancherel theorem with respect to the Fourier transform
(Boggess & Narcowich, Citation2001, p. 107; Pandey & Upadhyay, Citation2015). Hence,
Therefore,
Now using the wavelet inversion formula the triple integrals
and
converge to
,
, respectively,
(i) in
(ii) pointwise
(iii) uniformly for all .
The results (i), (ii) and (iii) are all proved in (Pandey, Jha, & Singh, Citation2016), but the proofs of (i) and (ii) can also be found in (Boggess & Narcowich, Citation2001, p.258). This implies (in view of (iii)) that
using the structure formula (2.1) for .
We have proven that
As explained earlier, this inversion formula is valid uniquely if . If
then
where
and
is a constant. Therefore, the wavelet transform of
is
Hence
and so, using (3.19) we get
This explains the ambiguity in our inversion formula. For the validity of the uniqueness in our inversion formula must belong to
.
4. Conclusion
In this present paper authors introduced the continuous wavelet transform on Schwartz tempered distributions and proved the corresponding wavelet inversion formula (valid modulo a constant distribution) interpreting convergence in the weak topology of .
We have observed that our aforesaid investigations are true when the wavelet kernel under cosideration for determining the wavelet transform are those wavelets whose all the moments are non-zero. Our entire results and facts are stated and proved as Lemmas and Theorems.
Acknowledgements
1. Authors express their gratefulness to referees for their constructive criticism and for many good suggestions for the improvement in the presentation.
2. They also express their gratefulness to Professor Angelo Mingarelli, and Professor Lucy Campbell of the School of Mathematics and Statistics, Carleton University, Ottawa, for suggesting improvements to our presentation in the manuscript.
Additional information
Funding
Notes on contributors
J.N. Pandey
The Continuous wavelet transform on Schwartz tempered distributions was firstly introduced by Holschneider in 1995. Pathak(2004) studied the various properties of contiuous wavelet transform on Schwartz tempered distributions. Pandey and Upadhyay(2015) introduced the continuous wavelet transform using the concept of window functions. Motivated from the above results, the authors extend the continuous wavelet transform to Schwartz tempered distributions and investigate the corresponding wavelet inversion formula (valid modulo a constant tempered distribution) interpreting convergence in the weak distributional sense. This theory is true, when the wavelet kernel under consideration for determining the wavelet transform are those wavelets, whose all the moments are non-zero. The example of such types of wavelet kernel is also given in this work.
References
- Akhiezer, N. I., & Glazman, I. M. (1961). Theory of linear operators in Hilbert space (Vol. I.). New York, NY: Ungar Publishing Company.
- Boggess, A., & Narcowich, F. J. (2001). A first course in wavelets with fourier analysis. New Jersey: Prentice-Hall, Inc.
- Chui, C. K. (1992). Introduction to Wavelets. New York, NY: Academic Press Inc.
- Daubechies, I. (1990). Ten lectures on wavelets. Massachusetts: Mathematics Department, University of Lowell.
- Gelfand, I. M., & Shilov, G. E. (1968). Generalized functions (Vol. 2). New York and London: Academic Press.
- Holschneider, M. (1995). Wavelets, An Analysis Tool. New York: The Clarendon Press, Oxford University Press.
- Lebedeva, E. A., & Postinikov, E. B. (2014). On alternative wavelet reconstruction formula: A case study of approximate wavelets. Royal Society Open Science, 1(2), 1140124. doi:10.1098/rs05.140124
- Pandey, J. N., Jha, N. K., & Singh, O. P. (2016). The continuous wavelet transform in n-dimensions. International Journal of Wavelets, Multiresolution and Information Processing, 14(5). doi:10.1142/S0219691316500375
- Pandey, J. N., & Upadhyay, S. K. (2015). The continuous Wavelet transform and window functions. Proceedings of the American Mathematical Society, 143(11), 4759–15. doi:10.1090/proc/2015-143-11
- Pathak, R. S. (2004). The wavelet transform of distributions. Tohoku Mathematical Journal, 56, 411–421. doi:10.2748/tmj/1113246676
- Pathak, R. S. (2009). The wavelet transform. Amsterdam and Paris: Atlantis Press World Scientific.
- Postnikov, E. B., Lebedeva, E. A., & Lavrova, A. F. (2016). Computational implementation of the inverse continuous wavelet transform without a requirement of the admissibility condition. Applied Mathematics and Computation, 282, 128–136. doi:10.1016/j.amc.2016.02.013
- Robewicz, S. (1972). Metric linear spaces. Warsaw: PWN-Polish Scientific Publishers.
- Weisz, F. (2013). Inversion formula for the continuous wavelet transform. Acta Mathematica Hungarica, 138(3), 237–258. doi:10.1007/s10474-012-0263-y
- Weisz, F. (2014). Inverse continuous wavelet transform, in Pringsheim’s sense on Wiener Amalgam spaces. Acta Mathematica Hungarica. doi:10.1007/s10474-015-0492-y,
- Weisz, G. (2015). Convergence of the inverse continuous wavelet transform in Wiener amalgam spaces. Analysis, 35(1), 33–46. doi:10.1515/anly-2014-1267
- Yosida, K. (1995). Functional analysis. Berlin Heidelberg: Springer Verlag.
- Zemanian, A. H. (1965). Distribution theory and transform analysis. New York, NY: McGraw-Hill Book Company.
- Zemanian, A. H. (1968). Generalized integral transformation. New York, NY: Inter Science Publishers, John Wiley and Sons Inc.