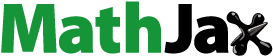
Abstract
The quest to generate distributions with more desirable and flexible properties for the modeling of data has led to an intense focus on the development of new families that are generalizations of existing distributions by researchers. A new family of distributions called the chen generated family is developed in this study. Its statistical properties such as the quantile, moments, incomplete moments, stochastic ordering and order statistics are derived by using the method of maximum likelihood, estimators for the parameters of the new family are developed. Three special distributions, Chen Burr III, Chen Kumaraswamy and Chen Weibull, are proposed from the new family, though it can generalize other distributions. A demonstration of the usefulness of the new family is performed using real dataset.
PUBLIC INTEREST STATEMENT
Modeling of natural phenomena such as earthquakes, rainfall, tsunami and so on mostly involves the use of statistical distributions. Since the accuracy of the results largely depends on how well the distribution fits the dataset, the study develops a new family of distributions which is to improve the flexibility of existing distributions.
Conflict of Interest
The authors declare that there are no conflicts of interest regarding the publication of this article.
1. Introduction
The accuracy of parametric statistical inference and modeling of datasets largely depends on how well the probability distribution fits the given dataset once it has met all distributional assumptions. Several studies have been carried out on statistical distributions in the quest to generate distributions with more desirable and flexible properties that can model real-life datasets of varying shapes of density and failure rate functions. Currently, most studies are focused on developing new families that are generalizations of existing distributions to provide better fit to the modeling of data. These families of distributions are constructed by either compounding two or more distributions or adding one or more parameters to the baseline model. Many authors have extensively reviewed the various families of distributions (Hamedani, Yousof, Rasekhi, Alizadeh, & Najibi, Citation2018; Lee, Famoye, & Alzaatreh, Citation2013; Nasiru, Citation2018; Nasiru, Mwita, & Ngesa, Citation2018; Zubair, Citation2018).
In this study a new class of distributions is developed and proposed using the T-X approach (Alzaatreh, Lee, & Famoye, Citation2013). The Chen generated (CG) family of distributions is obtained by compounding the two-parameter Chen distribution (Chen, Citation2000) and an arbitrary baseline cumulative distribution function (cdf) of a continuous random variable. The main motivation for developing this family is to improve the flexibility of the existing classical distributions, thus to enabling them to provide a better fit to real data sets than other candidate distributions with the same number of parameters and model different kinds of failure rate (monotonic and non-monotonic).
The remaining sections of the paper follow this order: the Chen generated (CG) family of distributions is defined in section 2. The mixture representation of the probability density function (pdf) is presented in section 3. Some statistical properties of the family of distributions are derived in section 4. The estimators for the parameters of the family are developed in section 5. Some special distributions from the CG family of distributions are proposed and discussed in section 6. Simulations to examine the properties of estimators of parameters of the special distributions are carried out in section 7. Real-life data set is used to demonstrate the application of the special distributions in section 8. Concluding remarks of the study are captured in section 9.
2. Chen generated a family of distributions
Let be a Chen distributed continuous random variable, its cdf denoted by
is given by
(Chen, Citation2000). Also, let
and
be the respective cdf and pdf of an arbitrary continuous random variable
. The cdf of the CG family is defined as;
where is a normalizing constant,
and
are scale and shape parameters, respectively. The pdf
of the family is given by;
The survival function, of the CG family is;
The failure rate or hazard function, of the family is obtained as follows:
3. Mixture representation of distribution
Mixture representation plays a useful role in the derivation of the statistical properties of the new family of distributions. Hence, the mixture representation of the pdf of the CG family of distributions is derived in this section.
By applying Taylor series expansion, the pdf of the CG family in EquationEquation (2)(2)
(2) is expressed as
EquationEquation (5)(5)
(5) can be rewritten as;
is further expanded using the binomial series expansion
for any real non-integer
as follows:
Assuming an integer in the binomial expansion,
where
From EquationEquation (6)(6)
(6) , the CG family’s density is expressed as a product of the parameters and the sum of the product of the pdf and weighted power series of the baseline distribution function
.
Alternatively, EquationEquation (6)(6)
(6) can further be written in terms of the exponentiated-G (expo-G) density function as
where and
is the expo-G density function with the power parameter
.
4. Statistical properties
This section discusses some of the statistical properties of the CG family of distributions. These include: quantile function, non-central moments, moments, generating functions, inequality measures, entropies, residual life, stochastic ordering and order statistics.
4.1. Quantile function
Proposition 1. The quantile function for CG family of distributions is given by
Proof. The quantile function of a random variable
is defined as
. Replacing
with
in EquationEquation (1)
(1)
(1) , equating
to
and making
the subject yields the quantile function. The median of the family is obtained by substituting
in EquationEquation (8)
(8)
(8) .
4.2. Moments, moment generating functions and incomplete moments
Moments are very essential in statistical analysis as they can be used to study important features (such as tendencies, variation, skewness, kurtosis and so on) of a distribution.
4.2.1. Non-central moments
Proposition 2. The non-central moment of the CG family is given by
is the probability weighted moment of the baseline distribution
Proof. The non-central moment is defined as
, thus using the mixture form of the density, the
non-central moment of the CG family is given by
Alternatively, the non-central moment of the CG family can be described in terms of the quantile function as follows;
. From EquationEquation (9)
(9)
(9) ,
4.2.2. Moment generating functions
Proposition 3. The moment generating function of the CG family is given by
Proof. By definition, the moment generating function is given by , expanding
using Taylor series,
.
But from EquationEquation (9)(9)
(9) ,
hence the proof.
Alternatively, letting , the moment generating function can be expressed in terms of quantile functions as;
4.2.3. Incomplete moments
Proposition 4. The incomplete moment of the CG family of distribution is given by
Proof. The incomplete moment is defined as
Substituting the mixture representation of the density function into the definition of the
incomplete moments completes the proof.
Alternatively, letting , the incomplete moments can be expressed in terms of the quantile function as;
4.3. Inequality measures
Lorenz and Bonferroni curves are applied in so many fields such as econometrics, demography, reliability, medicine and insurance. They are generally used in studying inequality measures like income and poverty.
4.3.1. Lorenz curve
The Lorenz curve for incomplete moments is defined as
for the CG family, it is given by;
Alternatively, letting ,
can be expressed in terms of the quantile functions as;
4.3.2. Bonferroni curve
Bonferroni curve is defined as
, hence for the CG family it is given by;
4.4. Mean residual life
The mean residual life of a component (which is the average survival time of the component after it has exceeded a specific time) is defined as
Proposition 5. The mean residual life of a CG random variable is given by
Proof. The mean residual life is defined as . Substituting
in EquationEquation (6)
(6)
(6) into
gives the mean residual life.
4.5. Entropy
Entropy is a measure of variation or uncertainty of a random variable. Its application spans across probability theory, engineering and science in general.
4.5.1. Rényi’s entropy
The Rényi’s entropy (Rényi, Citation1961) for a random variable with pdf , is defined as;
Proposition 5. Renyi’s entropy for the CG random variable is given by;
where
Proof. From EquationEquation (2)(2)
(2) ,
Adopting similar concept for expanding the density, becomes
where . Substituting
into
completes the proof.
4.6. Stochastic ordering
Ordering mechanism in data can easily be shown using stochastic ordering. Let and
be random variables with cdfs
and
respectively.
is less than
in likelihood ratio order
, if the function
.
Proposition 6. Let and
, where
is a
vector of parameters associated with the baseline distribution.
is less than
in likelihood ratio order
if
.
Proof. The ratio of their pdfs is given by , which is a decreasing function if
.
4.7. Order statistics
The pdf for the order statistic
, of an independent identically distributed random sample
of size
,
, is given by;
Expanding using binomial expansion,
. Substituting into the density of the
order statistic yields,
where .
Hence, the pdf for the order statistic is given by;
Employing a similar concept of expanding the density of the CG family, a mixture representation of the pdf of the order statistic is defined as;
where
4.7.1. Moments of order statistics
The non-central moment of the
order statistic is given by
. Substituting EquationEquation (21)
(21)
(21) into
the
non-central moment of the
order statistic of the CG random variable is given by,
where is the probability weighted moment of the baseline distribution.
5. Parameter estimation
Maximum likelihood estimation method was used in estimating the parameters for the family of distribution for similar reasons as stated in Nasiru et al. (Nasiru et al., Citation2018). Given a random sample of size
from the CG family of distributions, the total log-likelihood function is given by
where is a
vector of parameters associated with the baseline distribution.
The parameters are then estimated by partially differentiating the total log-likelihood function with respect to the parameters of the CG family as follows.
and
where and
.
Equating the score functions to zero and numerically solving the system of equations using techniques such as the quasi Newton-Raphson method, gives the maximum likelihood estimates for the parameters. The interval estimates of the parameters are obtained by first finding the observed information matrix given by
(for
and
), whose elements can be numerically computed. Under the regularity conditions, as
,
, where
is the observed information matrix evaluated at
The approximate
confidence intervals (where
is the significance level) can be constructed using the asymptotic normal distribution.
6. Some special distributions
The CG family of distributions can be used to extend many distributions to create more flexibility in their applications. In this section some special distributions were developed.
6.1. Chen Burr III distribution
Suppose that the baseline distribution is Burr III (Burr, Citation1942), it’s cdf and pdf are given by and
,
respectively. The cdf of Chen Burr III (CB) is given by
Its corresponding density and hazard functions are, respectively
and
Plots of the density and hazard rate functions of the CB distribution are displayed in Figure . The density plot exhibit varying shapes including unimodal with different degrees of kurtosis, right skewed and reversed J shapes. The hazard rate function for some selected values exhibited upside down bathtub, decreasing and increasing failure rates.
The CB distribution’s quantile function is given by;
6.2. Chen Kumaraswamy distribution
The Chen Kumaraswamy (CK) distribution uses the Kumaraswamy distribution (Kumaraswamy, Citation1980) with pdf and cdf respectively given by and
,
as the baseline distribution. The cdf of CK distribution is given by
with its corresponding density and hazard rate functions, respectively, given by
and
Plots of the density and hazard rate functions of the CK distribution are displayed in Figure . The plot of the density shows shapes such as; the reversed J, left skewed, right skewed and unimodal shapes among others. The hazard rate plot for some selected values exhibits increasing and decreasing failure rates, unimodal and bathtub shapes.
The quantile function is obtained as.
6.3. Chen Weibull distribution
Chen Weibull (CW) distribution is obtained using Weibull distribution (Weibull, Citation1951) with cdf and pdf, respectively, given by and
as baseline distribution. The cdf and pdf of the CW distribution are, respectively, given by
and
The hazard rate function is given by
The CW distribution’s plots of its density exhibit; right skewed, left skewed, unimodal and reversed J shapes among others as shown in Figure . The hazard rate plot of the CW distribution for some selected values exhibits varying shapes such as increasing and decreasing failure rates, right and left skewed unimodal shapes and upside down bathtub shape.
The quantile function of the CW distribution is given by
7. Simulations
Monte Carlo simulations were performed in this section to investigate the behavior of the maximum likelihood estimators of the parameters. For illustration purposes, the simulation experiments were undertaken using the Chen Weibull distribution. The experiments were replicated for times using sample size
and parameter values
and
. The average bias (AB), root-mean-square error (RMSE) and coverage probability (CP) of the
confidence intervals for the estimators of the parameters were estimated. From Table , the ABs and RMSEs for the estimators generally decrease to zero as the sample size increases. This implies that as the sample size increases the accuracy and consistency of the maximum likelihood estimators are achieved. Also, the CPs for most of the estimators are quite close to the nominal value of 0.95. Thus, we can say that the maximum likelihood technique works very well to estimate the parameters of the Chen Weibull distribution.
Table 1. Monte Carlo simulation results
8. Applications
In this section the performance of the CW distribution in providing good parametric fits to real-life datasets is demonstrated. Its goodness of fit measures are compared with competing models such as; exponentiated Chen (EC) (Chaubey & Zhang, Citation2015), extended Weibull (EW) (Xie, Tang, & Goh, Citation2002) and Kumaraswamy exponentiated Chen (KEC) (Khan, King, & Hudson, Citation2018) distributions. The information criteria and goodness of fit measures used are; Akaike information criteria (AIC), Bayesian information criteria (BIC), consistent Akaike information criteria (CAIC), HQ information Criteria (HQIC), Kolmogorov–Smirnov statistic(KS),Cramer-von misses distance values (CM) and Anderson Darling statistic (AD). In obtaining the maximum likelihood estimates for the parameters, the log-likelihood function of the models were maximized using the bbmle package’s subroutine mle2 in R (Bolker, Citation2014). The maximum likelihood estimates with the largest maxima were chosen after using a wide range of initial values.
For illustration, the first dataset (data1) consists of the fatigue times of 6061-T6 aluminum coupons cut parallel with the direction of rolling and oscillated at 18 cycles per second found in Birnbaum & Saunders (Birnbaum & Saunders, Citation1969), whilst the second dataset (data2) represents survival times of guinea pigs injected with different amounts of tubercle bacilli studied by Bjerkedal (Bjerkedal, Citation1960). These datasets are given in Tables and .
Table 2. Fatigue time of 101 6061-T6 aluminum coupons
Table 3. Survival times of guinea pigs injected with different amounts of tubercle bacilli
A preliminary exploration of the datasets on the shapes of the hazard rate functions showed that data1 has an increasing hazard rate function whilst data two have a unimodal hazard rate function as shown in Figure .
The maximum likelihood estimates and the corresponding standard errors of the parameters of the fitted distributions for both datasets and their goodness of fit measures are displayed in Tables and respectively. The parameters of all the distributions were significant at 5% significance level, with the exception of CW and KEC distributions which had only one of their parameters (and
respectively) significant at 15% significance level.
Table 4. Maximum likelihood estimates and standard errors of parameters in brackets
Table 5. Goodness-of-fit statistics and information criteria
Compared to the competing models, the CW distribution with its four parameters provides a better fit for the datasets as it has the smallest value for all the goodness of fit measures used as shown in Table .
This is further confirmed by the plots of densities and cdfs of the empirical and fitted distributions as shown in Figures and . From the fitted plot, it is observed that the CW provides a reasonable fit to the density.
The P-P plots also indicates the CW distribution provides a better fit for both datasets in comparison with KEC, EC and EW distributions as shown in Figures and .
The profile likelihoods of the estimated parameters of the CW distribution for the datasets are shown in Figures and . From the plots, it is observed that the estimated values for the parameters are the maxima.
9. Conclusion
The focus of most researchers is geared towards developing new families of distributions for generalizing existing distributions to provide better fit for the modeling of life data. A new family of distribution called the CG family is developed and studied. Its statistical properties such as the quantile, moments, incomplete moments, generating function, entropies, stochastic ordering and order statistics are derived. Estimators for the parameters of the new family were developed using the method of maximum likelihood. A demonstration of the application of the special distribution developed from the family was carried out using two-real datasets. A comparison of the results with that of other existing distributions showed that the special distribution developed from the CG family provide a better parametric fit to these datasets.
Additional information
Funding
Notes on contributors
Lea Anzagra
Lea Anzagra is a doctoral candidate with the Department of Statistics, University for Development Studies, Ghana. Her research interest is in distribution theory, probability theory and survival analysis.
Solomon Sarpong
Solomon Sarpong is a Senior Lecturer with the Department of Statistics, University for Development Studies, Ghana. His research interest is in distribution theory and time series analysis.
Suleman Nasiru
Suleman Nasiru is a Senior Lecturer with the Department of Statistics, University for Development Studies, Ghana. His research interest is in distribution theory, quality control and time series analysis.
References
- Alzaatreh, A., Lee, C., & Famoye, F. (2013). A new method for generating families of continuous distributions. Metron, 71(1), 63–20. doi:10.1007/s40300-013-0007-y
- Birnbaum, Z. W., & Saunders, S. C. (1969). Estimation for a family of life distributions with applications to fatigue. Journal of Applied Probability, 6, 328–347. doi:10.2307/3212004
- Bjerkedal, T. (1960). Acquisition of resistance in guinea pies infected with different doses of virulent tubercle bacilli. American Journal of Hygeine, 72(1), 130–148.
- Bolker, B. (2014). Tools for general maximum likelihood estimation. R development core team.
- Burr, I. W. (1942). Cumulative frequency functions. Annals of Mathematical Statistics, 13, 215–232. doi:10.1214/aoms/1177731607
- Chaubey, Y. P., & Zhang, R. (2015). An extension of Chen’s family of survival distributions with bathtub shape or increasing hazard rate function. Communications in Statistics - Theory and Methods, 44(19), 4049–4064. doi:10.1080/03610926.2014.997357
- Chen, Z. (2000). A new two-parameter lifetime distribution with bathtub shape or increasing failure rate function. Statistics & Probability Letters, 49, 155–161. doi:10.1016/S0167-7152(00)00044-4
- Cordeiro, G. M., & de Castro, M. (2011). A new family of generalized distributions. Journal of Statistical Computation and Simulation, 81(7), 883–898. doi:10.1080/00949650903530745
- Hamedani, G. G., Yousof, H. M., Rasekhi, M., Alizadeh, M., & Najibi, S. M. (2018). Type I general exponential class of distributions. Pakistan Journal of Statistics and Operation Research, 14(1), 39–55. doi:10.18187/pjsor.v14i1.2193
- Khan, M. S., King, R., & Hudson, I. L. (2018). Kumaraswamy exponentiated Chen distribution for modelling lifetime data. Applied Mathematics and Information Sciences, 12(3), 617–623. doi:10.18576/amis/120317
- Kumaraswamy, P. (1980). Generalized probability density-function for double-bounded random-processes. Journal of Hydrology, 462, 79–88. doi:10.1016/0022-1694(80)90036-0
- Lee, C., Famoye, F., & Alzaatreh, A. Y. (2013). Methods for generating families of univariate continuous distributions in the recent decades. WIREs Computational Statistics, 5(3), 219–238. doi:10.1002/wics.1255
- Nasiru, S. (2018). Extended Odd Fréchet-G family of distributions. Journal of Probability and Statistics, 2018, 1–12. doi:10.1155/2018/2931326
- Nasiru, S., Mwita, P. N., & Ngesa, O. (2018). Exponentiated generalized power series family of distributions. Annals of Data Science, 6(3), 463–489. doi:10.1007/s40745-018-0170-3
- Rényi, A. (1961). On measures of entropy and information. Proceedings of the Fourth Berkeley Symposium on Mathematical Statistics and Probability, Contributions to the Theory of Statistics, 1, 547–561.
- Weibull, W. (1951). A statistical distribution function of wide applicability. Journal of Applied Mechanics, 18, 293–296.
- Xie, M., Tang, Y., & Goh, T. N. (2002). A modified Weibull extension with bathtub-shaped failure rate function. Reliability Engineering and System Safety, 76(3), 279–285. doi:10.1016/S0951-8320(02)00022-4
- Zubair, A. (2018). The Zubair-G family of distributions: Properties and applications. Annals of Data Science. doi:10.1007/s40745-018-0169-9