Abstract
Early diagnosis and timely therapeutic intervention are clinical challenges of rheumatoid arthritis (RA), especially for treatment-resistant or difficult-to-treat patients. Little is known about the immunological mechanisms involved in refractory RA. In this review, we summarize previous research findings on the immunological mechanisms of treatment-resistant RA. Genetic prediction of treatment-resistant RA is challenging. Patients with and without anti-cyclic citrullinated peptide autoantibodies are considered part of distinct subgroups, especially regarding long-term clinical prognosis and treatment responses. B cells, T cells and other immune cells and fibroblasts are of pathophysiological importance and are associated with treatment responses. Finally, we propose a new hypothesis that stratifies patients with RA into two subgroups with distinct immunological pathologies based on our recent immunomics analysis of RA. One RA subgroup with a favorable prognosis is characterized by increased interferon signaling. Another subgroup with a worse prognosis is characterized by enhanced acquired immune responses. Increases in dendritic cell precursors and diversified autoreactive anti-modified protein antibodies may have pathophysiological roles, especially in the latter subgroup. These findings that improve treatment response predictions might contribute to future precision medicine for RA.
1. Introduction
Rheumatoid arthritis (RA) is a common chronic autoimmune inflammatory disease characterized by persistent synovitis and/or joint destruction. Although RA is currently challenging to cure, early diagnosis and timely therapeutic intervention are important to suppress disease activity and prevent irreversible joint destruction [Citation1]. The ‘treat-to-target (T2T)‘ strategy has been adopted for tight control of the disease by defining the aim of treatment and using appropriate combinations of disease-modifying anti-rheumatic drugs (DMARDs), including biologics and targeted synthetic (ts) DMARDs [Citation2]. Difficult-to-treat (D2T) RA is emerging as a serious problem in the clinical setting [Citation3–6]. An estimated 5–20% of patients have D2T RA [Citation3–5]. Even when biological DMARDs and/or tsDMARDs are used, an improvement of at least 50% (ACR50) is currently only obtained in around half of patients [Citation7,Citation8]. In 2020, the European Alliance of Associations for Rheumatology (EULAR) issued a definition of D2T RA [Citation9]. For the treatment of RA, a stratified approach that incorporates treatment response predictions is strongly desired, and research progress in this area is anticipated. The stratification of patients and early identification and appropriate treatment of treatment-resistant patients are considered part of the goals of next-generation therapies.
It has been identified that longer disease duration, female sex, prior DMARDs use, higher disease activity and delay of initial treatment are important clinical predictors of refractory RA [Citation5,Citation10]. Early RA has a so-called ‘window of opportunity‘ in which the treatment response is generally good [Citation11]. Therefore, it is crucial for patients with RA to be treated with the most suitable DMARDs as a first-line therapy and to suppress disease activity during this window of opportunity.
Precision medicine in rheumatic diseases is in the early stages of development. For example, patients with psoriatic arthritis have been classified according to peripheral lymphocyte phenotypes measured by flow cytometry. Differential drug administration based on these phenotypes was associated with a >90% reduction in non-responders compared with conventional treatment with biologics [Citation12,Citation13]. In RA, similarly, many studies have been conducted to allow the future realization of precision medicine.
Not only genome, transcriptome and proteome data but also splicing [Citation14] and epigenome [Citation15] data can be associated with mechanisms of treatment resistance. Recent studies aiming to predict treatment response have been conducted by integrating these omics data with data from research on the synovium (as reviewed below) or microbiome [Citation16–18]. For example, multi-omics research testing the association between drug response and the peripheral blood transcriptome, proteome and immunophenotyping identified molecular signatures related to treatment resistance, which could be largely explained by an imbalance of neutrophils, monocytes and lymphocytes [Citation19].
When aiming for precision medicine, it is essential to understand the overall picture of immunological mechanisms leading to D2T RA. In this review, we first summarize research findings on the immunological mechanisms of D2T RA, followed by a new hypothesis we propose for RA stratification.
2. Genomics
Genetic research on RA is essential to understand the mechanism involved in RA. Previous genome-wide association studies (GWAS) have identified >100 genetic regions and single-nucleotide polymorphisms (SNPs) associated with RA susceptibility [Citation20–23]. Genetic variants of PTPN22, PADI4 (encoding PAD4 enzyme that citrullinate proteins) and CTLA4 have more impact on seropositive RA than seronegative RA [Citation23].
These SNPs associated with RA susceptibility can potentially predict treatment responses to drugs or disease progression. For example, SNPs in the TRAF1 gene can predict clinical response to tumor necrosis factor (TNF) inhibitor treatment in patients with RA [Citation24]. HLA–DRB1 and non-HLA gene alleles, such as PADI4 and C5orf3, are associated with radiographic progression [Citation25,Citation26]. Another GWAS study identified the influence of the LINC02549 and LRRC55 loci in the response to TNF inhibitors [Citation27]. However, further GWAS studies assessing the treatment response to TNF inhibitors revealed that genetic variants only provide a small contribution to the treatment response [Citation28,Citation29].
The polygenic risk score (PRS) is an estimate of an individual’s genetic risk to a trait or disease, calculated according to their genotype profile and relevant GWAS data [Citation30,Citation31], enabling the prediction of an individual’s disease risk from genomic data. For example, PRS can predict radiographic progression in RA, especially in patients who were younger at disease onset [Citation32]. PRS may have the potential to predict treatment responses.
Most of the GWAS-identified genetic variants in RA are located in non-coding enhancer regions of the genome [Citation33,Citation34], and their biological function remains unclear. Expression quantitative trait locus (eQTL) analysis seeks to identify genetic variants that affect the expression of genes and is one of the focuses of functional genetic research in the post-GWAS era. Importantly, eQTL effects vary by immune cell type [Citation35]. Various eQTL studies have been performed in multiple tissues and cell types [Citation36–38]. We have recently constructed an atlas for the comprehensive study of detailed gene expression profiles of 28 peripheral blood immune cell types in patients with immune-mediated diseases (the Immune Cell Gene Expression Atlas from the University of Tokyo, or ImmuNexUT) [Citation39]. Polygenic analysis of ImmuNexUT and GWAS results reconfirmed previous findings with epigenome annotation, including the association between regulatory T cells (Fr. II eTreg) and RA [Citation40,Citation41].
3. Autoantibodies
The primary characteristic of RA is the production of autoantibodies, such as rheumatoid factor (RF) and anti-modified protein antibodies (AMPAs), such as anti-cyclic citrullinated peptide autoantibodies (ACPAs), anti-carbamylated protein antibodies and anti-acetylated protein antibodies. These antibodies are formed to eliminate the modified proteins by targeting antigens in the synovium and other parts of the body in patients with RA. RF and ACPA may precede the onset of RA by several years [Citation42,Citation43], and are well-known indicators of disease severity [Citation44–46]. However, the validity of ACPA for short-term clinical prognosis in RA has not been established: ACPA has been reported to predict more severe radiographic damage after several years of follow-up [Citation47–51]. On the other hand, studies of patients with RA presenting within three months and two years of symptom onset suggested no differences in the disease activity based on ACPA status [Citation52,Citation53].
Amino acid sequences common to RA-sensitive HLA-DRs (e.g., DRB1*0401, DRB1*0405) are termed shared epitope (SE). HLA-DRB1 alleles encoding SE are associated with ACPA-positive RA, and ACPA-positive and ACPA-negative patients are considered to have distinct phenotypes [Citation54,Citation55]. A positive autoantibody status is associated with good responses to rituximab (RTX), tocilizumab (TCZ) or abatacept (ABT) [Citation56–58].
Positivity for both RF and ACPA or multiple AMPAs has been shown to represent a clinically more severe phenotype [Citation45,Citation59–61]. In addition, a prospective study showed that in patients with RA who were in remission, autoantibodies against various antigens were associated with an increased risk of relapse [Citation62]. Non-response to RTX was associated with incomplete depletion of the B cell receptor repertoire in the first month of treatment [Citation63]. On the other hand, another randomized controlled trial showed that a baseline broad autoantibody profile was associated with early treatment response but not long-term outcomes [Citation64]. Positivity for multiple antibodies may have an amplifying effect in the long term and may worsen treatment response.
Synovial tissue of RA harbors dominant B cell and plasma cell clones associated with autoreactivity [Citation65]. In synovial tissue and fluid, ACPA-positive patients had higher levels of infiltrating lymphocytes [Citation66] and T cell-derived and pro-inflammatory cytokines than ACPA-negative patients [Citation67]. Recently, a single-cell RNA-sequencing (scRNA-seq) study revealed that ACPA titers differed in RA with distinct synovial cell type abundance phenotypes [Citation68]. Another scRNA-seq study of the synovium showed upregulation of CCL13, CCL18 (which inhibits CCR1 mediated chemotaxis [Citation69]) and MMP3 in myeloid cell subsets, together with poor B cell and T cell responses, were salient immune features in the synovium of ACPA-negative patients [Citation70].
It is highly likely that differences in autoantibody-associated pathogenicity may explain mechanisms behind treatment resistance and lead to personalized treatment in the near future.
4. Cell subsets
4.1. B cells
As mentioned above, autoantibodies such as RF or ACPA are characteristic of RA, and the efficacy of B cell depletion therapy further indicates the pathogenic significance of B cells in RA [Citation71–73]. RA results from a T cell-driven inflammation, and T cell activation in the rheumatoid synovium is dependent on B cells in germinal center-like structures [Citation74,Citation75]. Epidermal growth factor ligand amphiregulin (AREG), which was identified to be produced in all B cells in patients with RA, led to increased migration and proliferation of fibroblast-like synoviocytes and, in combination with ACPA, led to the increased differentiation of osteoclasts [Citation76].
B cells play an essential role in the autoimmune process of RA. A higher frequency of activated memory (CD21− double-negative) and activated naïve B cells in non-responders was observed both before and after treatment, although most B cell subsets in peripheral blood did not change in response to anti-TNF treatment [Citation77]. In contrast, the number of transitional B cells, a putative regulatory subset, and Tregs were lower in non-responders [Citation77]. On the other hand, other reports suggested increases or decreases in cell subsets with treatment. For example, the use of TNF inhibitors and/or TCZ treatment was shown to affect double-negative memory B cells in the periphery of patients with longer disease duration [Citation78]. Decreased unswitched memory B cells were observed in responders [Citation79]. Longer disease duration is known to affect the frequency of memory B cells [Citation80], so further research is necessary to assess whether treatment responses can be predicted using B cell proportions.
RNA-seq adds more information to phenotype data. A longitudinal RNA-seq study assessing the peripheral blood of patients with RA revealed that naïve B cells, which are known to be activated in RA [Citation81], are activated several weeks before relapse, and this results in an increase in pre-inflammation mesenchymal cells (PRIME cells). These data suggest that this mechanism could potentially be used to predict RA relapse [Citation82].
Synovial RNA-seq data analysis of early treatment-naive patients revealed that plasma cell gene expression was associated with ACPA positivity and predicted future progressive joint damage on X-rays [Citation83], which was consistent with the fact that local plasma cell differentiation was associated with a high ACPA titer [Citation84,Citation85]. Another synovial RNA-seq study revealed that TCZ is more effective than RTX in patients with a lower lineage expression signature of B cells [Citation86].
4.2. T cells
T cells can recognize antigens and act as a command center for the immune response. When antigen-presenting cells are activated, they present HLA class II and antigens to helper T cells, which activate them. Activated T cells induce the differentiation of B cells into plasma cells, resulting in the production of autoantibodies. An HLA-DRB1 genotype is the strongest genetic risk factor for RA. Since GWAS RA-susceptible genes correspond to CD4+ T cell enhancers, CD4+ T cells are suggested to be involved in the development of RA [Citation22,Citation33]. Previously, we have compared the eQTL catalog of five peripheral blood fractions (CD4+ T cells, CD8+ T cells, B cells, NK cells and monocytes) with RA GWAS data, and revealed that the activation of the TNF pathway in CD4+ T cells is involved in the development of RA [Citation35]. As mentioned above, ImmuNexUT and GWAS results suggested the association of Fr. II eTreg with RA [Citation39].
Cytotoxic T lymphocyte-associated protein 4 (CTLA-4) binds to CD80/CD86 on antigen-presenting cells and suppresses co-stimulation by binding to CD28 at the T cell surface. Tregs are known to permanently express CTLA-4. ABT is a fusion protein that combines the extracellular portion of CTLA-4 with the IgG-Fc portion (CTLA-Ig), and the inhibition of T cell activation by ABT suggests a T cell contribution in RA.
In addition, in our RNA-seq analysis of CD4+ T cell subsets from the peripheral blood of patients with RA, the T cell receptor signaling pathways were upregulated in RA, and ABT therapy induced improvement of the dysregulation [Citation87].
T follicular helper (Tfh) cells are a CD4+ T cell subset located in the locus coeruleus germinal center of secondary lymphoid tissues that play an important role in T cell-dependent antibody production. Another study identified that ABT treatment resulted in a marked decrease in activated Tfh cells and that an increase in baseline Tfh cell levels was a significant predictor of treatment resistance to ABT [Citation88]. A subset of CXCR5–PD1+CD4+ T cells, termed T peripheral helper (Tph) cells, present in the peripheral blood and synovial tissue of RA and exhibit a transcriptional profile that overlaps with Tfh cells and have the potential to induce autoantibody production in B cells [Citation89]. While there is currently no demonstrated association between Tph and treatment resistance in RA, there is a possibility that Tph-induced antibody production in B cells may contribute to treatment resistance.
Prediction of treatment resistance was attempted with a proportion of T cells. Ponchel et al. identified a new subset of CD4+ T cells, called ‘inflammation-related cells (IRC)‘ in patients with RA [Citation90]. In ACPA-positive patients, dysregulation of T cell subsets predicted the risk of faster progression to arthritis: higher naïve T cell and Treg frequencies were found to have a protective effect, while higher IRC frequencies were associated with an increased risk of progression [Citation91]. The mechanism of treatment resistance related to these three subsets may be complicated, as these subsets are generated through different pathways. Of these three cells, increased naïve T cells in early RA were found to predict the response to methotrexate (MTX) [Citation92].
4.3. Monocytes
Monocytes are bone marrow-derived cells that mediate essential regulatory and effector functions in innate and adaptative immunity [Citation93]. Circulating peripheral blood monocytes may migrate into tissues and differentiate into different effector cells, such as macrophages, dendritic cells (DCs) and osteoclasts. In particular, HBEGF+ macrophages in RA induce fibroblast invasiveness [Citation94].
Some reports have suggested relationships between treatment response and myeloid cells. A higher pre-treatment number of circulating monocytes was found to predict a reduced clinical response to MTX in untreated patients with RA [Citation95]. Responders to adalimumab plus MTX treatment showed a significant normalization of the number of circulating monocytes [Citation96].
Among biological DMARDs, anti-TNF treatments exert the most prominent activity on monocytes [Citation97]. In innate immune cells, including monocytes, activation requires transcription of NLRP3. In monocytes, SNPs in NLRP3 were associated with response to infliximab (IFX) [Citation98]. Differences in monocyte gene expression in TNF inhibitor responders or non-responders suggest differential interferon (IFN) pathway activation [Citation99].
Signal transducer and activator of transcription (STAT) 6 pathway activation in circulating monocytes at baseline was a predictor of treatment response to synthetic DMARDs [Citation100]. High Janus kinase (JAK) 3 phosphorylation and low JAK2 phosphorylation in CD14+ monocytes have been found to correlate significantly with remission following treatment with synthetic DMARDs [Citation101]. The treatment response to baricitinib is correlated with STAT1 phosphorylation in monocytes [Citation102]. Therefore, activation of the JAK-STAT pathway in monocytes may be related to treatment response.
Interactions with other cells may also be related to treatment response. The binding of platelets has a modulatory effect, accentuated by the increased binding of platelets to monocytes in response to interleukin (IL)-6 inhibitors [Citation103].
4.4. Dendritic cells
Conventional (c)DC and plasmacytoid (p)DC constitute major DC subsets. cDCs are activated by pathogen stimulation and migrate to lymph nodes. There, cDCs activate naive T cells by presenting pathogen-derived antigens. cDCs are classified into CD141+ cDC (cDC1) and CD1c+ cDC (cDC2). pDCs have a morphology similar to plasma cells and are responsible for the immune response in the early stages of infection by recognizing viral nucleic acids and producing large amounts of type I IFNs.
An increase of cDCs in peripheral blood is correlated with treatment response to a TNF inhibitor, IFX [Citation104]. cDCs have also been shown to promote joint inflammation in RA. Transient depletion of cDCs reduced arthritis in CD11cDTR transgenic mice [Citation105]. Synovial cDC1s were also reported to be increased in patients with RA and induced higher levels of CD4+ and CD8+ T cell activation compared with their peripheral blood counterparts [Citation106]. As for cDC2, the same group reported that, in patients with inflammatory arthritis, cDC2s in the synovium were increased and more maturated compared to cDC2s in the peripheral blood [Citation107].
Another synovial RNA-seq study revealed that a more inflammatory synovial phenotype at baseline, including many modules of monocytes and chemokines and modules of DCs and antigen presentation, correlated with treatment response to six months of DMARD treatment [Citation83].
Dendritic cell precursors (pre-DC) are a recently identified subpopulation of DCs and differentiate into cDC1 and cDC2 [Citation108]. The known function of pre-DCs is IL-12 production and naive CD4+ T cell stimulation. Using peripheral blood RNA-seq data of RA, we recently reported that an increase in pre-DC in peripheral blood can predict RA treatment resistance [Citation109].
4.5. Neutrophils
Neutrophils are the most abundant circulating leucocyte subset in humans and travel to inflamed synovium in response to chemokines. A high baseline neutrophil-to-lymphocyte ratio (NLR) in the blood is associated with treatment resistance to TNF inhibitors or TCZ [Citation110,Citation111]. A more than 20% reduction of neutrophil count one month after initiating TCZ predicts clinical remission within one year at an early treatment phase [Citation112]. Indeed, RNA-seq data analysis revealed that 23 neutrophil transcripts in the blood predicted response to therapy with TNF inhibitors in RA [Citation113].
Neutrophils are typically the most abundant leucocyte in the synovial fluid of arthritis. Synovial neutrophils produce pro-inflammatory factors such as IL-1, citrullinated peptides, neutrophil extracellular traps, leukotriene B4 and reactive oxygen species [Citation114–118]. The difference between neutrophils in active arthritis and healthy controls is indistinguishable in the peripheral blood. However, the transcriptome of neutrophils in inflamed joints is different from that of healthy controls, indicating that aging and the IFNγ response drive the phenotype of neutrophils in the inflamed joint [Citation119]. Few reported biomarkers of treatment resistance relating to synovial neutrophils have been described.
4.6. Synovium including fibroblast-like synoviocytes/fibroblasts
The synovium is a tissue mainly consisting of fibroblasts, with a lining and sublining surrounding the joints. NOTCH3 signaling contributes to the differentiation of THY1-expressing sublining fibroblasts [Citation120], and the expansion of sublining fibroblasts leads to the development of inflammatory arthritis [Citation121–123].
Recently, exhaustive synovial research in RA has progressed rapidly. There are two major cohorts of synovial RNA-seq data: the large European cohort of blood and synovium obtained from early treatment-naive patients, namely, the Pathobiology of Early Arthritis Cohort (PEAC) [Citation124], and the data in Accelerating Medicines Partnership (AMP) program in the United States [Citation68,Citation121,Citation125]. Other synovial data are being actively collected and analyzed [Citation70].
In 2019, cellular and molecular analyses of synovial tissue in the PEAC cohort demonstrated the presence of three pathology groups in early RA [Citation83,Citation126]. These analyses also showed that elevation of myeloid- and lymphoid-associated gene expression at baseline strongly correlated with DMARD response at six months and that elevation of osteoclast-targeting genes predicted radiographic joint damage progression at 12 months [Citation126].
As for the response to each drug, TCZ was found to be more effective than RTX in patients with low or absent B-cell lineage expression signatures and in patients with higher macrophages/monocytes and myeloid dendritic cells (mDC) in synovial tissue [Citation86,Citation127]. Poor response to multiple biologics was associated with an increase in baseline CD34+ sublining fibroblasts and DKK3+ sublining fibroblasts [Citation127]. The fibroid/pauci-immune synovial pathotype has been known to predict treatment resistance to TNF inhibitors [Citation128], and it also appeared to be predictive of treatment resistance to multiple other drugs [Citation127]. The treatment response may depend on the cellular composition of the synovium, so the era when we select treatment in accordance with synovial phenotype may be arriving soon.
5. Type 1 interferon
Type I IFNs, which consist of IFNα and IFNβ, are ubiquitously expressed in various cells and mediate innate immune responses against viruses. It has been reported that around 33% of patients with RA exhibit increased type I IFN signaling [Citation129]. In one study, there were twice as many patients with a positive interferon-stimulated gene signature (IGS) among those with early RA than those with established RA [Citation130].
To date, the type I IFN gene signature or activity has been proposed as a predictive factor of treatment response [Citation109,Citation131–133], while other reports found that IFN is a factor that predicts a poor prognosis [Citation130,Citation134,Citation135]. As for RTX, a good clinical response was associated with baseline levels of low type I IFN-response genes and a selective drug-induced increase in type I IFN-response activity in patients with RA [Citation136–138]. The role of IFN in the pathology of RA remains, therefore, unclear but these data indicate that the type I IFN signature has the potential to differentiate patients who might respond to a certain medication.
6. Possible stratification of RA into IFN and acquired immune subgroups
Our new hypothesis is that a subgroup of patients with RA with a relatively poor prognosis can be characterized by increased pre-DCs and a diverse AMPA repertoire (). Meanwhile, patients with RA with a relatively favorable prognosis characterized by increased IFN signaling may represent a different subgroup with distinct immunological pathologies.
Figure 1. One hypothesis of stratifying patients with RA for treatment response. Patients with RA may be classified into two subgroups based on type I IFN, pre-DCs and AMPA repertoires. (1) Subgroup 1: patients with increased type I IFN signaling have fewer pre-DCs in the peripheral blood, reduced stimulation of CD4+ T cells and B cells, and thus a better response to therapy. This may explain why early RA is more responsive to therapy. (2) Subgroup 2: in cases of type I IFN deficiency, there is an increase in pre-DCs in the peripheral blood. This increase in pre-DCs, either as pre-DC-like cells themselves or after further differentiation into inflammatory cDC2s, stimulates antigen-specific CD4+ T cells, leading to diversity in the AMPA repertoire, which may contribute to treatment resistance. AMPA: anti-modified protein antibodies; DC: dendritic cell; IFN; interferon; RA: rheumatoid arthritis.
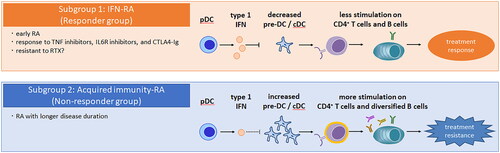
The type I IFN gene signature or activity is a predictive factor of treatment response [Citation131–133]. In our recent study of RA peripheral blood, we revealed that a subgroup of RA with shorter disease duration and a relatively favorable prognosis is characterized by increased IFN signaling (subgroup 1) [Citation109]. Consistently, it is reported that type I IFN increases in early RA [Citation130] and that it is a good prognostic factor in early RA [Citation11]. From these data, it is hypothesized that early RA has increased type I IFN and is more responsive to therapy.
On the other hand, as for RTX, good clinical responses in RA were associated with low baseline levels of type I IFN response genes [Citation136–138]. Higher IFN activity can be associated with lower dependence on B cells or be protective from the depletion of pathogenic B cells. Alternatively, high IFN levels can have some effects on B cell differentiation, as it is known that type I IFN induces the differentiation of CD40– activated B cells into non-immunoglobulin-secreting plasmablasts [Citation139].
Another subgroup is associated with longer disease duration and treatment resistance (subgroup 2). In our data, an increase of pre-DCs in the peripheral blood was characteristic of this subgroup, regardless of treatment history [Citation109]. Type I IFN signaling was negatively correlated to pre-DC gene expression, consistent with a report that suggests that type I IFN signaling suppresses cDC development [Citation140]. In our synovial single-cell transcriptome profiling analysis, we identified pre-DC-like DCs occupying around a third of the synovial DC population, related to pro-inflammatory cDC2Bs helping CD4+ T cell responses [Citation141]. In treatment-resistant patients, an increase in pre-DCs may affect the synovial acquired immune response in RA by stimulating antigen-specific CD4+ T cells, either as pre-DC-like cells themselves or after further differentiation into pro-inflammatory cDC2s. JAK inhibitors (tofacitinib, baricitinib and ruxolitinib) inhibit the T cell stimulation by DC [Citation142–144], suggesting that JAK inhibitors could be a key treatment for subgroup 2.
Interestingly, we have observed a more diverse plasmablast activation through B cell receptor repertoire analysis in this treatment-resistant group compared to the treatment-response group (Nagafuchi et al. unpublished). As a diverse AMPA repertoire is related to worse prognosis in RA [Citation62], this subgroup of RA could have diversified ‘acquired immunity‘ responses. Importantly, our data showed no significant differences in ACPA positivity in the treatment response and treatment-resistant groups. Therefore, these immunological subgroups of RA are independent of conventional ACPA seropositivity subgroups.
The reasons for the differential expression of type 1 IFN based on the duration of disease and the mechanisms underlying the negative correlation between type 1 IFN and pre-DC are still unknown, making them important areas for future research. Additional data from prospective studies based on our new stratification will also be awaited in the future.
As a limitation to this hypothesis, the original study [Citation109] was retrospective, and therefore, it was not possible to completely eliminate potential confounding factors between the two groups. Another limitation was that our study was limited to the Japanese population only, and it is desirable to conduct further studies involving multiple racial groups in the future.
7. Conclusions
In this review, we have discussed various immunological mechanisms, including the composition of various cells and the activation of specific pathways, which contribute to the treatment resistance of RA in a complex manner. Moreover, the mechanisms behind treatment resistance can change with each disease phase, as treatment resistance is related to longer disease duration [Citation5,Citation10]. Therefore, it is not straightforward to stratify patients and realize precision medicine in RA. Stratifying patients with RA into two subgroups, as we proposed above, may be a good starting point. We may also need to combine various other data of the patients, such as autoantibody profiles, immune cell phenotypes and various omics data to realize personalized medicine in RA.
Author contributions
SY and YN designed the study and contributed to writing the draft manuscript. KF supervised and contributed to the critical revision of the manuscript. All authors approved the final version to be published.
Disclosure statement
YN belongs to the Social Cooperation Program, Department of Functional Genomics and Immunological Diseases, supported by Chugai Pharmaceutical. The other authors declare no conflict of interest.
References
- Goekoop-Ruiterman YP, de Vries-Bouwstra JK, Allaart CF, et al. Comparison of treatment strategies in early rheumatoid arthritis: a randomized trial. Ann Intern Med. 2007;146(6):406–415. doi: 10.7326/0003-4819-146-6-200703200-00005.
- Smolen JS, Aletaha D, Bijlsma JW, et al. Treating rheumatoid arthritis to target: recommendations of an International Task Force. Ann Rheum Dis. 2010;69(4):631–637. doi: 10.1136/ard.2009.123919.
- Kearsley-Fleet L, Davies R, De Cock D, et al. Biologic refractory disease in rheumatoid arthritis: results from the British Society for Rheumatology Biologics Register for Rheumatoid Arthritis. Ann Rheum Dis. 2018;77(10):1405–1412. doi: 10.1136/annrheumdis-2018-213378.
- de Hair MJH, Jacobs JWG, Schoneveld JLM, et al. Difficult-to-treat rheumatoid arthritis: an area of unmet clinical need. Rheumatology. 2018;57(7):1135–1144.
- Bécède M, Alasti F, Gessl I, et al. Risk profiling for a refractory course of rheumatoid arthritis. Semin Arthritis Rheum. 2019;49(2):211–217. doi: 10.1016/j.semarthrit.2019.02.004.
- Buch MH. Defining refractory rheumatoid arthritis. Ann Rheum Dis. 2018;77(7):966–969. doi: 10.1136/annrheumdis-2017-212862.
- Hazlewood GS, Barnabe C, Tomlinson G, et al. Methotrexate monotherapy and methotrexate combination therapy with traditional and biologic disease modifying antirheumatic drugs for rheumatoid arthritis: abridged Cochrane systematic review and network meta-analysis. BMJ. 2016;353:i1777. doi: 10.1136/bmj.i1777.
- Choy EH. Clinical significance of Janus kinase inhibitor selectivity. Rheumatology. 2019;58(6):1122. doi: 10.1093/rheumatology/kez002.
- Nagy G, Roodenrijs NM, Welsing PM, et al. EULAR definition of difficult-to-treat rheumatoid arthritis. Ann Rheum Dis. 2021;80(1):31–35. doi: 10.1136/annrheumdis-2020-217344.
- Anderson JJ, Wells G, Verhoeven AC, et al. Factors predicting response to treatment in rheumatoid arthritis: the importance of disease duration. Arthritis Rheum. 2000;43(1):22–29. doi: 10.1002/1529-0131(200001)43:1<22::AID-ANR4>3.0.CO;2-9.
- Boers M. Understanding the window of opportunity concept in early rheumatoid arthritis. Arthritis Rheum. 2003;48(7):1771–1774. doi: 10.1002/art.11156.
- Genovese MC, Kalunian K, Gottenberg JE, et al. Effect of filgotinib vs placebo on clinical response in patients with moderate to severe rheumatoid arthritis refractory to disease-modifying antirheumatic drug therapy: the FINCH 2 randomized clinical trial. JAMA. 2019;322(4):315–325. doi: 10.1001/jama.2019.9055.
- Tanaka Y, Kubo S, Miyagawa I, et al. Lymphocyte phenotype and its application to precision medicine in systemic autoimmune diseases. Semin Arthritis Rheum. 2019;48(6):1146–1150. doi: 10.1016/j.semarthrit.2019.04.007.
- Ibáñez-Costa A, Perez-Sanchez C, Patiño-Trives AM, et al. Splicing machinery is impaired in rheumatoid arthritis, associated with disease activity and modulated by anti-TNF therapy. Ann Rheum Dis. 2022;81(1):56–67. doi: 10.1136/annrheumdis-2021-220308.
- Tao W, Concepcion AN, Vianen M, et al. Multiomics and machine learning accurately predict clinical response to adalimumab and etanercept therapy in patients with rheumatoid arthritis. Arthritis Rheumatol. 2021;73(2):212–222. doi: 10.1002/art.41516.
- Gupta VK, Cunningham KY, Hur B, et al. Gut microbial determinants of clinically important improvement in patients with rheumatoid arthritis. Genome Med. 2021;13(1):149. doi: 10.1186/s13073-021-00957-0.
- Qiao J, Zhang SX, Chang MJ, et al. Specific enterotype of gut microbiota predicted clinical effect of methotrexate in patients with rheumatoid arthritis. Rheumatology. 2023;62(3):1087–1096. doi: 10.1093/rheumatology/keac458.
- Artacho A, Isaac S, Nayak R, et al. The pretreatment gut microbiome is associated with lack of response to methotrexate in new-onset rheumatoid arthritis. Arthritis Rheumatol. 2021;73(6):931–942. doi: 10.1002/art.41622.
- Tasaki S, Suzuki K, Kassai Y, et al. Multi-omics monitoring of drug response in rheumatoid arthritis in pursuit of molecular remission. Nat Commun. 2018;9(1):2755. doi: 10.1038/s41467-018-05044-4.
- Okada Y, Terao C, Ikari K, et al. Meta-analysis identifies nine new loci associated with rheumatoid arthritis in the Japanese population. Nat Genet. 2012;44(5):511–516. doi: 10.1038/ng.2231.
- Eyre S, Bowes J, Diogo D, et al. High-density genetic mapping identifies new susceptibility loci for rheumatoid arthritis. Nat Genet. 2012;44(12):1336–1340. doi: 10.1038/ng.2462.
- Okada Y, Wu D, Trynka G, et al. Genetics of rheumatoid arthritis contributes to biology and drug discovery. Nature. 2014;506(7488):376–381. doi: 10.1038/nature12873.
- Ishigaki K, Sakaue S, Terao C, et al. Multi-ancestry genome-wide association analyses identify novel genetic mechanisms in rheumatoid arthritis. Nat Genet. 2022;54(11):1640–1651. doi: 10.1038/s41588-022-01213-w.
- Nishimoto T, Seta N, Anan R, et al. A single nucleotide polymorphism of TRAF1 predicts the clinical response to anti-TNF treatment in Japanese patients with rheumatoid arthritis. Clin Exp Rheumatol. 2014;32(2):211–217.
- Suzuki T, Ikari K, Yano K, et al. PADI4 and HLA-DRB1 are genetic risks for radiographic progression in RA patients, independent of ACPA status: results from the IORRA cohort study. PLOS One. 2013;8(4):e61045. doi: 10.1371/journal.pone.0061045.
- Muthana M, Hawtree S, Wilshaw A, et al. C5orf30 is a negative regulator of tissue damage in rheumatoid arthritis. Proc Natl Acad Sci U S A. 2015;112(37):11618–11623. doi: 10.1073/pnas.1501947112.
- Sánchez-Maldonado JM, Cáliz R, López-Nevot M, et al. Validation of GWAS-identified variants for anti-TNF drug response in rheumatoid arthritis: a meta-analysis of two large cohorts. Front Immunol. 2021;12:672255. doi: 10.3389/fimmu.2021.672255.
- Sieberts SK, Zhu F, García-García J, et al. Crowdsourced assessment of common genetic contribution to predicting anti-TNF treatment response in rheumatoid arthritis. Nat Commun. 2016;7:12460. doi: 10.1038/ncomms12460.
- Spiliopoulou A, Colombo M, Plant D, et al. Association of response to TNF inhibitors in rheumatoid arthritis with quantitative trait loci for. Ann Rheum Dis. 2019;78(8):1055–1061. doi: 10.1136/annrheumdis-2018-214877.
- Choi SW, Mak TS, O’Reilly PF. Tutorial: a guide to performing polygenic risk score analyses. Nat Protoc. 2020;15(9):2759–2772. doi: 10.1038/s41596-020-0353-1.
- Khera AV, Chaffin M, Aragam KG, et al. Genome-wide polygenic scores for common diseases identify individuals with risk equivalent to monogenic mutations. Nat Genet. 2018;50(9):1219–1224. doi: 10.1038/s41588-018-0183-z.
- Honda S, Ikari K, Yano K, et al. Association of polygenic risk scores with radiographic progression in patients with rheumatoid arthritis. Arthritis Rheumatol. 2022;74(5):791–800. doi: 10.1002/art.42051.
- Farh KK, Marson A, Zhu J, et al. Genetic and epigenetic fine mapping of causal autoimmune disease variants. Nature. 2015;518(7539):337–343. doi: 10.1038/nature13835.
- Finucane HK, Bulik-Sullivan B, Gusev A, et al. Partitioning heritability by functional annotation using genome-wide association summary statistics. Nat Genet. 2015;47(11):1228–1235. doi: 10.1038/ng.3404.
- Ishigaki K, Kochi Y, Suzuki A, et al. Polygenic burdens on cell-specific pathways underlie the risk of rheumatoid arthritis. Nat Genet. 2017;49(7):1120–1125. doi: 10.1038/ng.3885.
- Chen L, Ge B, Casale FP, et al. Genetic drivers of epigenetic and transcriptional variation in human immune cells. Cell. 2016;167(5):1398–1414.e24. doi: 10.1016/j.cell.2016.10.026.
- Schmiedel BJ, Singh D, Madrigal A, et al. Impact of genetic polymorphisms on human immune cell gene expression. Cell. 2018;175(6):1701–1715.e16. doi: 10.1016/j.cell.2018.10.022.
- GTEx Consortium. The GTEx Consortium atlas of genetic regulatory effects across human tissues. Science. 2020;369(6509):1318–1330. doi: 10.1126/science.aaz1776.
- Ota M, Nagafuchi Y, Hatano H, et al. Dynamic landscape of immune cell-specific gene regulation in immune-mediated diseases. Cell. 2021;184(11):3006–3021.e17. doi: 10.1016/j.cell.2021.03.056.
- Kundaje A, Meuleman W, Ernst J, et al. Integrative analysis of 111 reference human epigenomes. Nature. 2015;518(7539):317–330. doi: 10.1038/nature14248.
- Trynka G, Sandor C, Han B, et al. Chromatin marks identify critical cell types for fine mapping complex trait variants. Nat Genet. 2013;45(2):124–130. doi: 10.1038/ng.2504.
- Nielen MM, van Schaardenburg D, Reesink HW, et al. Specific autoantibodies precede the symptoms of rheumatoid arthritis: a study of serial measurements in blood donors. Arthritis Rheum. 2004;50(2):380–386. doi: 10.1002/art.20018.
- Rantapää-Dahlqvist S, de Jong BA, Berglin E, et al. Antibodies against cyclic citrullinated peptide and IgA rheumatoid factor predict the development of rheumatoid arthritis. Arthritis Rheum. 2003;48(10):2741–2749. doi: 10.1002/art.11223.
- Machold KP, Stamm TA, Nell VP, et al. Very recent onset rheumatoid arthritis: clinical and serological patient characteristics associated with radiographic progression over the first years of disease. Rheumatology. 2007;46(2):342–349. doi: 10.1093/rheumatology/kel237.
- Aletaha D, Alasti F, Smolen JS. Rheumatoid factor, not antibodies against citrullinated proteins, is associated with baseline disease activity in rheumatoid arthritis clinical trials. Arthritis Res Ther. 2015;17(1):229. doi: 10.1186/s13075-015-0736-9.
- Gonzalez A, Icen M, Kremers HM, et al. Mortality trends in rheumatoid arthritis: the role of rheumatoid factor. J Rheumatol. 2008;35(6):1009–1014.
- van Gaalen FA, van Aken J, Huizinga TW, et al. Association between HLA class II genes and autoantibodies to cyclic citrullinated peptides (CCPs) influences the severity of rheumatoid arthritis. Arthritis Rheum. 2004;50(7):2113–2121. doi: 10.1002/art.20316.
- Meyer O, Labarre C, Dougados M, et al. Anticitrullinated protein/peptide antibody assays in early rheumatoid arthritis for predicting five year radiographic damage. Ann Rheum Dis. 2003;62(2):120–126. doi: 10.1136/ard.62.2.120.
- van Jaarsveld CH, ter Borg EJ, Jacobs JW, et al. The prognostic value of the antiperinuclear factor, anti-citrullinated peptide antibodies and rheumatoid factor in early rheumatoid arthritis. Clin Exp Rheumatol. 1999;17(6):689–697.
- Westgeest AA, Boerbooms AM, Jongmans M, et al. Antiperinuclear factor: indicator of more severe disease in seronegative rheumatoid arthritis. J Rheumatol. 1987;14(5):893–897.
- Kroot EJ, de Jong BA, van Leeuwen MA, et al. The prognostic value of anti-cyclic citrullinated peptide antibody in patients with recent-onset rheumatoid arthritis. Arthritis Rheum. 2000;43(8):1831–1835. doi: 10.1002/1529-0131(200008)43:8<1831::AID-ANR19>3.0.CO;2-6.
- Cader MZ, Filer AD, Buckley CD, et al. The relationship between the presence of anti-cyclic citrullinated peptide antibodies and clinical phenotype in very early rheumatoid arthritis. BMC Musculoskelet Disord. 2010;11:187. doi: 10.1186/1471-2474-11-187.
- van der Helm-van Mil AH, Verpoort KN, Breedveld FC, et al. Antibodies to citrullinated proteins and differences in clinical progression of rheumatoid arthritis. Arthritis Res Ther. 2005;7(5):R949–R958. doi: 10.1186/ar1767.
- van der Helm-van Mil AH, Huizinga TW. Advances in the genetics of rheumatoid arthritis point to subclassification into distinct disease subsets. Arthritis Res Ther. 2008;10(2):205. doi: 10.1186/ar2384.
- Huizinga TW, Amos CI, van der Helm-van Mil AH, et al. Refining the complex rheumatoid arthritis phenotype based on specificity of the HLA-DRB1 shared epitope for antibodies to citrullinated proteins. Arthritis Rheum. 2005;52(11):3433–3438. doi: 10.1002/art.21385.
- Isaacs JD, Cohen SB, Emery P, et al. Effect of baseline rheumatoid factor and anticitrullinated peptide antibody serotype on rituximab clinical response: a meta-analysis. Ann Rheum Dis. 2013;72(3):329–336. doi: 10.1136/annrheumdis-2011-201117.
- Maneiro RJ, Salgado E, Carmona L, et al. Rheumatoid factor as predictor of response to abatacept, rituximab and tocilizumab in rheumatoid arthritis: systematic review and meta-analysis. Semin Arthritis Rheum. 2013;43(1):9–17. doi: 10.1016/j.semarthrit.2012.11.007.
- Gottenberg JE, Ravaud P, Cantagrel A, et al. Positivity for anti-cyclic citrullinated peptide is associated with a better response to abatacept: data from the ‘Orencia and Rheumatoid Arthritis’ registry. Ann Rheum Dis. 2012;71(11):1815–1819. doi: 10.1136/annrheumdis-2011-201109.
- Mouterde G, Lukas C, Goupille P, et al. Association of anticyclic citrullinated peptide antibodies and/or rheumatoid factor status and clinical presentation in early arthritis: results from the ESPOIR cohort. J Rheumatol. 2014;41(8):1614–1622. doi: 10.3899/jrheum.130884.
- Sokolove J, Johnson DS, Lahey LJ, et al. Rheumatoid factor as a potentiator of anti-citrullinated protein antibody-mediated inflammation in rheumatoid arthritis. Arthritis Rheumatol. 2014;66(4):813–821. doi: 10.1002/art.38307.
- Nijjar JS, Morton FR, Bang H, et al. The impact of autoantibodies against citrullinated, carbamylated, and acetylated peptides on radiographic progression in patients with new-onset rheumatoid arthritis: an observational cohort study. Lancet Rheumatol. 2021;3(4):e284–e293. doi: 10.1016/S2665-9913(20)30381-7.
- Figueiredo CP, Bang H, Cobra JF, et al. Antimodified protein antibody response pattern influences the risk for disease relapse in patients with rheumatoid arthritis tapering disease modifying antirheumatic drugs. Ann Rheum Dis. 2017;76(2):399–407. doi: 10.1136/annrheumdis-2016-209297.
- Pollastro S, Klarenbeek PL, Doorenspleet ME, et al. Non-response to rituximab therapy in rheumatoid arthritis is associated with incomplete disruption of the B cell receptor repertoire. Ann Rheum Dis. 2019;78(10):1339–1345. doi: 10.1136/annrheumdis-2018-214898.
- de Moel EC, Derksen VFAM, Stoeken G, et al. Baseline autoantibody profile in rheumatoid arthritis is associated with early treatment response but not long-term outcomes. Arthritis Res Ther. 2018;20(1):33. doi: 10.1186/s13075-018-1520-4.
- Doorenspleet ME, Klarenbeek PL, de Hair MJ, et al. Rheumatoid arthritis synovial tissue harbours dominant B-cell and plasma-cell clones associated with autoreactivity. Ann Rheum Dis. 2014;73(4):756–762. doi: 10.1136/annrheumdis-2012-202861.
- van Oosterhout M, Bajema I, Levarht EW, et al. Differences in synovial tissue infiltrates between anti-cyclic citrullinated peptide-positive rheumatoid arthritis and anti-cyclic citrullinated peptide-negative rheumatoid arthritis. Arthritis Rheum. 2008;58(1):53–60. doi: 10.1002/art.23148.
- Gómez-Puerta JA, Celis R, Hernández MV, et al. Differences in synovial fluid cytokine levels but not in synovial tissue cell infiltrate between anti-citrullinated peptide/protein antibody-positive and -negative rheumatoid arthritis patients. Arthritis Res Ther. 2013;15(6):R182. doi: 10.1186/ar4372.
- Zhang F, Jonsson AH, Nathan A, et al. Cellular deconstruction of inflamed synovium defines diverse inflammatory phenotypes in rheumatoid arthritis. bioRxiv; 2022. doi: 10.1101/2022.02.25.481990.
- Krohn SC, Bonvin P, Proudfoot AE. CCL18 exhibits a regulatory role through inhibition of receptor and glycosaminoglycan binding. PLOS One. 2013;8(8):e72321. doi: 10.1371/journal.pone.0072321.
- Wu X, Liu Y, Jin S, et al. Single-cell sequencing of immune cells from anticitrullinated peptide antibody positive and negative rheumatoid arthritis. Nat Commun. 2021;12(1):4977. doi: 10.1038/s41467-021-25246-7.
- Anolik JH, Barnard J, Cappione A, et al. Rituximab improves peripheral B cell abnormalities in human systemic lupus erythematosus. Arthritis Rheum. 2004;50(11):3580–3590. doi: 10.1002/art.20592.
- Edwards JC, Cambridge G. Sustained improvement in rheumatoid arthritis following a protocol designed to deplete B lymphocytes. Rheumatology. 2001;40(2):205–211. doi: 10.1093/rheumatology/40.2.205.
- Edwards JC, Szczepanski L, Szechinski J, et al. Efficacy of B-cell-targeted therapy with rituximab in patients with rheumatoid arthritis. N Engl J Med. 2004;350(25):2572–2581. doi: 10.1056/NEJMoa032534.
- Takemura S, Klimiuk PA, Braun A, et al. T cell activation in rheumatoid synovium is B cell dependent. J Immunol. 2001;167(8):4710–4718. doi: 10.4049/jimmunol.167.8.4710.
- Manzo A, Bombardieri M, Humby F, et al. Secondary and ectopic lymphoid tissue responses in rheumatoid arthritis: from inflammation to autoimmunity and tissue damage/remodeling. Immunol Rev. 2010;233(1):267–285. doi: 10.1111/j.0105-2896.2009.00861.x.
- Mahendra A, Yang X, Abnouf S, et al. Beyond autoantibodies: biologic roles of human autoreactive B cells in rheumatoid arthritis revealed by RNA-sequencing. Arthritis Rheumatol. 2019;71(4):529–541. doi: 10.1002/art.40772.
- Meednu N, Barnard J, Callahan K, et al. Activated peripheral blood B cells in rheumatoid arthritis and their relationship to anti-tumor necrosis factor treatment and response: a randomized clinical trial of the effects of anti-tumor necrosis factor on B cells. Arthritis Rheumatol. 2022;74(2):200–211. doi: 10.1002/art.41941.
- Moura RA, Quaresma C, Vieira AR, et al. B-cell phenotype and IgD-CD27- memory B cells are affected by TNF-inhibitors and tocilizumab treatment in rheumatoid arthritis. PLOS One. 2017;12(9):e0182927. doi: 10.1371/journal.pone.0182927.
- Hu F, Zhang W, Shi L, et al. Impaired CD27. Front Immunol. 2018;9:626. doi: 10.3389/fimmu.2018.00626.
- Souto-Carneiro MM, Mahadevan V, Takada K, et al. Alterations in peripheral blood memory B cells in patients with active rheumatoid arthritis are dependent on the action of tumour necrosis factor. Arthritis Res Ther. 2009;11(3):R84. doi: 10.1186/ar2718.
- Lu DR, McDavid AN, Kongpachith S, et al. T cell-dependent affinity maturation and innate immune pathways differentially drive autoreactive B cell responses in rheumatoid arthritis. Arthritis Rheumatol. 2018;70(11):1732–1744. doi: 10.1002/art.40578.
- Orange DE, Yao V, Sawicka K, et al. RNA identification of PRIME cells predicting rheumatoid arthritis flares. N Engl J Med. 2020;383(3):218–228. doi: 10.1056/NEJMoa2004114.
- Lewis MJ, Barnes MR, Blighe K, et al. Molecular portraits of early rheumatoid arthritis identify clinical and treatment response phenotypes. Cell Rep. 2019;28(9):2455–2470.e5. doi: 10.1016/j.celrep.2019.07.091.
- Corsiero E, Bombardieri M, Carlotti E, et al. Single cell cloning and recombinant monoclonal antibodies generation from RA synovial B cells reveal frequent targeting of citrullinated histones of NETs. Ann Rheum Dis. 2016;75(10):1866–1875. doi: 10.1136/annrheumdis-2015-208356.
- Teng YK, Levarht EW, Hashemi M, et al. Immunohistochemical analysis as a means to predict responsiveness to rituximab treatment. Arthritis Rheum. 2007;56(12):3909–3918. doi: 10.1002/art.22967.
- Humby F, Durez P, Buch MH, et al. Rituximab versus tocilizumab in anti-TNF inadequate responder patients with rheumatoid arthritis (R4RA): 16-week outcomes of a stratified, biopsy-driven, multicentre, open-label, phase 4 randomised controlled trial. Lancet. 2021;397(10271):305–317. doi: 10.1016/S0140-6736(20)32341-2.
- Sumitomo S, Nagafuchi Y, Tsuchida Y, et al. A gene module associated with dysregulated TCR signaling pathways in CD4. J Autoimmun. 2018;89:21–29. doi: 10.1016/j.jaut.2017.11.001.
- Nakayamada S, Kubo S, Yoshikawa M, et al. Differential effects of biological DMARDs on peripheral immune cell phenotypes in patients with rheumatoid arthritis. Rheumatology. 2018;57(1):164–174. doi: 10.1093/rheumatology/kex012.
- Rao DA, Gurish MF, Marshall JL, et al. Pathologically expanded peripheral T helper cell subset drives B cells in rheumatoid arthritis. Nature. 2017;542(7639):110–114. doi: 10.1038/nature20810.
- Ponchel F, Morgan AW, Bingham SJ, et al. Dysregulated lymphocyte proliferation and differentiation in patients with rheumatoid arthritis. Blood. 2002;100(13):4550–4556. doi: 10.1182/blood-2002-03-0671.
- Hunt L, Hensor EM, Nam J, et al. T cell subsets: an immunological biomarker to predict progression to clinical arthritis in ACPA-positive individuals. Ann Rheum Dis. 2016;75(10):1884–1889. doi: 10.1136/annrheumdis-2015-207991.
- Ponchel F, Goëb V, Parmar R, et al. An immunological biomarker to predict MTX response in early RA. Ann Rheum Dis. 2014;73(11):2047–2053. doi: 10.1136/annrheumdis-2013-203566.
- Auffray C, Sieweke MH, Geissmann F. Blood monocytes: development, heterogeneity, and relationship with dendritic cells. Annu Rev Immunol. 2009;27:669–692. doi: 10.1146/annurev.immunol.021908.132557.
- Kuo D, Ding J, Cohn IS, et al. HBEGF+ macrophages in rheumatoid arthritis induce fibroblast invasiveness. Sci Transl Med. 2019;11(491):eaau8587. doi: 10.1126/scitranslmed.aau8587.
- Chara L, Sánchez-Atrio A, Pérez A, et al. The number of circulating monocytes as biomarkers of the clinical response to methotrexate in untreated patients with rheumatoid arthritis. J Transl Med. 2015;13:2. doi: 10.1186/s12967-014-0375-y.
- Chara L, Sánchez-Atrio A, Pérez A, et al. Monocyte populations as markers of response to adalimumab plus MTX in rheumatoid arthritis. Arthritis Res Ther. 2012;14(4):R175. doi: 10.1186/ar3928.
- Talmon M, Percio M, Obeng JA, et al. Transcriptomic profile comparison of monocytes from rheumatoid arthritis patients in treatment with methotrexate, anti-TNFa, abatacept or tocilizumab. PLOS One. 2023;18(3):e0282564. doi: 10.1371/journal.pone.0282564.
- Mathews RJ, Robinson JI, Battellino M, et al. Evidence of NLRP3-inflammasome activation in rheumatoid arthritis (RA); genetic variants within the NLRP3-inflammasome complex in relation to susceptibility to RA and response to anti-TNF treatment. Ann Rheum Dis. 2014;73(6):1202–1210. doi: 10.1136/annrheumdis-2013-203276.
- Wampler Muskardin TL, Fan W, Jin Z, et al. Distinct single cell gene expression in peripheral blood monocytes correlates with tumor necrosis factor inhibitor treatment response groups defined by type I interferon in rheumatoid arthritis. Front Immunol. 2020;11:1384. doi: 10.3389/fimmu.2020.01384.
- Kuuliala K, Kuuliala A, Koivuniemi R, et al. STAT6 and STAT1 pathway activation in circulating lymphocytes and monocytes as predictor of treatment response in rheumatoid arthritis. PLOS One. 2016;11(12):e0167975. doi: 10.1371/journal.pone.0167975.
- Kuuliala K, Kuuliala A, Koivuniemi R, et al. Baseline JAK phosphorylation profile of peripheral blood leukocytes, studied by whole blood phosphospecific flow cytometry, is associated with 1-year treatment response in early rheumatoid arthritis. Arthritis Res Ther. 2017;19(1):75. doi: 10.1186/s13075-017-1278-0.
- Tucci G, Garufi C, Pacella I, et al. Baricitinib therapy response in rheumatoid arthritis patients associates to STAT1 phosphorylation in monocytes. Front Immunol. 2022;13:932240. doi: 10.3389/fimmu.2022.932240.
- Mariscal A, Zamora C, Díaz-Torné C, et al. Increase of circulating monocyte–platelet conjugates in rheumatoid arthritis responders to IL-6 blockage. Int J Mol Sci. 2022;23(10):5748.
- Richez C, Schaeverbeke T, Dumoulin C, et al. Myeloid dendritic cells correlate with clinical response whereas plasmacytoid dendritic cells impact autoantibody development in rheumatoid arthritis patients treated with infliximab. Arthritis Res Ther. 2009;11(3):R100. doi: 10.1186/ar2746.
- Benson RA, Patakas A, Conigliaro P, et al. Identifying the cells breaching self-tolerance in autoimmunity. J Immunol. 2010;184(11):6378–6385. doi: 10.4049/jimmunol.0903951.
- Canavan M, Walsh AM, Bhargava V, et al. Enriched Cd141+ DCs in the joint are transcriptionally distinct, activated, and contribute to joint pathogenesis. JCI Insight. 2018;3(23):e95228. doi: 10.1172/jci.insight.95228.
- Canavan M, Marzaioli V, Bhargava V, et al. Functionally mature CD1c. Front Immunol. 2021;12:745226. doi: 10.3389/fimmu.2021.745226.
- See P, Dutertre CA, Chen J, et al. Mapping the human DC lineage through the integration of high-dimensional techniques. Science. 2017;356(6342):eaag3009. doi: 10.1126/science.aag3009.
- Yamada S, Nagafuchi Y, Wang M, et al. Immunomics analysis of rheumatoid arthritis identified precursor dendritic cells as a key cell subset of treatment resistance. Ann Rheum Dis. 2023;82(6):809–819.
- Lee HN, Kim YK, Kim GT, et al. Neutrophil-to-lymphocyte and platelet-to-lymphocyte ratio as predictors of 12-week treatment response and drug persistence of anti-tumor necrosis factor-α agents in patients with rheumatoid arthritis: a retrospective chart review analysis. Rheumatol Int. 2019;39(5):859–868. doi: 10.1007/s00296-019-04276-x.
- Ghang B, Kwon O, Hong S, et al. Neutrophil-to-lymphocyte ratio is a reliable marker of treatment response in rheumatoid arthritis patients during tocilizumab therapy. Mod Rheumatol. 2017;27(3):405–410. doi: 10.1080/14397595.2016.1214340.
- Nakajima T, Watanabe R, Hashimoto M, et al. Neutrophil count reduction 1 month after initiating tocilizumab can predict clinical remission within 1 year in rheumatoid arthritis patients. Rheumatol Int. 2022;42(11):1983–1991. doi: 10.1007/s00296-021-04944-x.
- Wright HL, Cox T, Moots RJ, et al. Neutrophil biomarkers predict response to therapy with tumor necrosis factor inhibitors in rheumatoid arthritis. J Leukoc Biol. 2017;101(3):785–795. doi: 10.1189/jlb.5A0616-258R.
- Miyabe Y, Miyabe C, Murooka TT, et al. Complement C5a receptor is the key initiator of neutrophil adhesion igniting immune complex-induced arthritis. Sci Immunol. 2017;2(7):eaaj2195. doi: 10.1126/sciimmunol.aaj2195.
- Romero V, Fert-Bober J, Nigrovic PA, et al. Immune-mediated pore-forming pathways induce cellular hypercitrullination and generate citrullinated autoantigens in rheumatoid arthritis. Sci Transl Med. 2013;5(209):209ra150. doi: 10.1126/scitranslmed.3006869.
- Khandpur R, Carmona-Rivera C, Vivekanandan-Giri A, et al. NETs are a source of citrullinated autoantigens and stimulate inflammatory responses in rheumatoid arthritis. Sci Transl Med. 2013;5(178):178ra40. doi: 10.1126/scitranslmed.3005580.
- Chen M, Lam BK, Kanaoka Y, et al. Neutrophil-derived leukotriene B4 is required for inflammatory arthritis. J Exp Med. 2006;203(4):837–842. doi: 10.1084/jem.20052371.
- Wright HL, Lyon M, Chapman EA, et al. Rheumatoid arthritis synovial fluid neutrophils drive inflammation through production of chemokines, reactive oxygen species, and neutrophil extracellular traps. Front Immunol. 2020;11:584116. doi: 10.3389/fimmu.2020.584116.
- Grieshaber-Bouyer R, Exner T, Hackert NS, et al. Ageing and interferon gamma response drive the phenotype of neutrophils in the inflamed joint. Ann Rheum Dis. 2022;81(6):805–814. doi: 10.1136/annrheumdis-2021-221866.
- Wei K, Korsunsky I, Marshall JL, et al. Notch signalling drives synovial fibroblast identity and arthritis pathology. Nature. 2020;582(7811):259–264. doi: 10.1038/s41586-020-2222-z.
- Zhang F, Wei K, Slowikowski K, et al. Defining inflammatory cell states in rheumatoid arthritis joint synovial tissues by integrating single-cell transcriptomics and mass cytometry. Nat Immunol. 2019;20(7):928–942. doi: 10.1038/s41590-019-0378-1.
- Mizoguchi F, Slowikowski K, Wei K, et al. Functionally distinct disease-associated fibroblast subsets in rheumatoid arthritis. Nat Commun. 2018;9(1):789. doi: 10.1038/s41467-018-02892-y.
- Croft AP, Campos J, Jansen K, et al. Distinct fibroblast subsets drive inflammation and damage in arthritis. Nature. 2019;570(7760):246–251. doi: 10.1038/s41586-019-1263-7.
- PEAC RNA-seq Data. Available at: https://peac.hpc.qmul.ac.uk/
- Accelerating Medicines Partnership (AMP). National Institutes of Health (NIH). Available at: https://www.nih.gov/research-training/accelerating-medicines-partnership-amp
- Humby F, Lewis M, Ramamoorthi N, et al. Synovial cellular and molecular signatures stratify clinical response to csDMARD therapy and predict radiographic progression in early rheumatoid arthritis patients. Ann Rheum Dis. 2019;78(6):761–772. doi: 10.1136/annrheumdis-2018-214539.
- Rivellese F, Surace AEA, Goldmann K, et al. Rituximab versus tocilizumab in rheumatoid arthritis: synovial biopsy-based biomarker analysis of the phase 4 R4RA randomized trial. Nat Med. 2022;28(6):1256–1268. doi: 10.1038/s41591-022-01789-0.
- Nerviani A, Di Cicco M, Mahto A, et al. A pauci-immune synovial pathotype predicts inadequate response to TNFα-blockade in rheumatoid arthritis patients. Front Immunol. 2020;11:845. doi: 10.3389/fimmu.2020.00845.
- Higgs BW, Liu Z, White B, et al. Patients with systemic lupus erythematosus, myositis, rheumatoid arthritis and scleroderma share activation of a common type I interferon pathway. Ann Rheum Dis. 2011;70(11):2029–2036. doi: 10.1136/ard.2011.150326.
- Cooles FAH, Anderson AE, Lendrem DW, et al. The interferon gene signature is increased in patients with early treatment-naive rheumatoid arthritis and predicts a poorer response to initial therapy. J Allergy Clin Immunol. 2018;141(1):445–448.e4. doi: 10.1016/j.jaci.2017.08.026.
- Sanayama Y, Ikeda K, Saito Y, et al. Prediction of therapeutic responses to tocilizumab in patients with rheumatoid arthritis: biomarkers identified by analysis of gene expression in peripheral blood mononuclear cells using genome-wide DNA microarray. Arthritis Rheumatol. 2014;66(6):1421–1431. doi: 10.1002/art.38400.
- Mavragani CP, La DT, Stohl W, et al. Association of the response to tumor necrosis factor antagonists with plasma type I interferon activity and interferon-beta/alpha ratios in rheumatoid arthritis patients: a post hoc analysis of a predominantly Hispanic cohort. Arthritis Rheum. 2010;62(2):392–401. doi: 10.1002/art.27226.
- van Baarsen LG, Wijbrandts CA, Rustenburg F, et al. Regulation of IFN response gene activity during infliximab treatment in rheumatoid arthritis is associated with clinical response to treatment. Arthritis Res Ther. 2010;12(1):R11. doi: 10.1186/ar2912.
- Lübbers J, Brink M, van de Stadt LA, et al. The type I IFN signature as a biomarker of preclinical rheumatoid arthritis. Ann Rheum Dis. 2013;72(5):776–780. doi: 10.1136/annrheumdis-2012-202753.
- Iwasaki T, Watanabe R, Ito H, et al. Dynamics of type I and type II interferon signature determines responsiveness to anti-TNF therapy in rheumatoid arthritis. Front Immunol. 2022;13:901437. doi: 10.3389/fimmu.2022.901437.
- Vosslamber S, Raterman HG, van der Pouw Kraan TC, et al. Pharmacological induction of interferon type I activity following treatment with rituximab determines clinical response in rheumatoid arthritis. Ann Rheum Dis. 2011;70(6):1153–1159. doi: 10.1136/ard.2010.147199.
- Raterman HG, Vosslamber S, de Ridder S, et al. The interferon type I signature towards prediction of non-response to rituximab in rheumatoid arthritis patients. Arthritis Res Ther. 2012;14(2):R95. doi: 10.1186/ar3819.
- de Jong TD, Vosslamber S, Blits M, et al. Effect of prednisone on type I interferon signature in rheumatoid arthritis: consequences for response prediction to rituximab. Arthritis Res Ther. 2015;17(1):78. doi: 10.1186/s13075-015-0564-y.
- Jego G, Palucka AK, Blanck JP, et al. Plasmacytoid dendritic cells induce plasma cell differentiation through type I interferon and interleukin 6. Immunity. 2003;19(2):225–234. doi: 10.1016/s1074-7613(03)00208-5.
- Lutz K, Musumeci A, Sie C, et al. Ly6D+Siglec-H+ precursors contribute to conventional dendritic cells via a Zbtb46+Ly6D+ intermediary stage. Nat Commun. 2022;13(1):3456. doi: 10.1038/s41467-022-31054-4.
- Brown CC, Gudjonson H, Pritykin Y, et al. Transcriptional basis of mouse and human dendritic cell heterogeneity. Cell. 2019;179(4):846–863.e24. doi: 10.1016/j.cell.2019.09.035.
- Kubo S, Yamaoka K, Kondo M, et al. The JAK inhibitor, tofacitinib, reduces the T cell stimulatory capacity of human monocyte-derived dendritic cells. Ann Rheum Dis. 2014;73(12):2192–2198. doi: 10.1136/annrheumdis-2013-203756.
- Kubo S, Nakayamada S, Sakata K, et al. Janus kinase inhibitor baricitinib modulates human innate and adaptive immune system. Front Immunol. 2018;9:1510. doi: 10.3389/fimmu.2018.01510.
- Heine A, Held SA, Daecke SN, et al. The JAK-inhibitor ruxolitinib impairs dendritic cell function in vitro and in vivo. Blood. 2013;122(7):1192–1202. doi: 10.1182/blood-2013-03-484642.