ABSTRACT
Dissolved organic matter (DOM) plays a crucial role in various aquatic environments, while comprehensive insights into DOM and its relations with heavy metals in drinking water sources are still lacking. Here, we choose the drinking water sources of Anhui province (China) as a typical case, exploring the occurrence of DOM and its relationship with heavy metals in 159 major drinking water sources. Results indicate the occurrence levels of DOM and three identified fluorescent components in the surface water (rivers, lakes, reservoirs) are significantly higher than that in the groundwater, while there was no significant difference in the occurrence levels of the chromophoric dissolved organic matter (CDOM) among the drinking water sources. Significant correlations between the DOM and heavy metals indicate that DOM could be used as a promising indicator for evaluating the occurrence and environmental behavior of some heavy metals in drinking water sources.
1. Introduction
DOM constitutes a complex and diverse amalgamation of organic molecules found extensively in various aquatic environments, including rivers, lakes, reservoirs and groundwater [Citation1, Citation2]. Operating as the most dynamic element of the carbon cycle, DOM actively participates in numerous biogeochemical cycles within the aquatic environment, forging a vital link between the carbon cycle and nutrient output across terrestrial and freshwater ecosystems [Citation3, Citation4]. In addition, due to the dual effects of DOM on nutrient cycling and pollutant environmental behavior in the water environment, there has been a surge of attention to DOM research in aquatic ecosystems in recent years [Citation5,Citation6]. Nevertheless, the current research on DOM in raw drinking water is still insufficient.
Concurrently, in raw water containing elevated levels of DOM, the presence of a substantial quantity of organic matter enhances particle stability, impeding precipitation. The effective removal of these suspended particles necessitates the application of a sufficient amount of flocculant, thereby amplifying the complexity of the water treatment process [Citation7]. Additionally, DOM interacts with disinfectants like chlorine during the water purification process. This interaction has the potential to produce carcinogenic compounds as by-products, posing a potential threat to both drinking water quality and human health [Citation8]. Overall, the chemical composition and bioavailability of DOM are intricately linked to the odor and taste of drinking water, disinfectant requirements, membrane contamination, and carcinogenic disinfection byproducts [Citation9]. Hence, a comprehensive investigation of the occurrence and distribution of DOM in drinking water sources is helpful to ensure drinking water quality.
Additionally, there are some complex pollutants present in drinking water bodies, which can pose potential risks to both aquatic environments and human health [Citation10, Citation11]. This paper focuses on the distribution of heavy metals in water bodies, a prevalent issue in drinking water sources within Anhui province. Heavy metal residue in drinking water sources has garnered global attention due to its high toxicity, persistence, and bioaccumulation [Citation12, Citation13]. Extensive research has demonstrated their potential to pose serious ecological risks in drinking water bodies across various regions worldwide [Citation14–16]. The global average content of certain heavy metals in the water environment surpasses allowable limits recommended by the World Health Organization (WHO) and the United States Environmental Protection Agency (USEPA), with concentrations in Asia generally exceeding those in Europe and North America [Citation17, Citation18]. Nevertheless, it was well-known that the high cost associated with determining heavy metals and the challenge of achieving a comprehensive range of determinations, recent studies have found that numerous spectral indices were closely related to some heavy metals [Citation19]. For instance, the fluorescence index (FI), humification index (HIX) and spectral slope ratio (SR) are related to heavy metals to some extent [Citation20, Citation21]. This indicates that DOM could be utilized to some extent as a characterization agent for the occurrence of heavy metal. Importantly, the DOM spectral analysis method is more cost-effective than the ICP-MS method used in the mainstream analysis of heavy metal content [Citation22]. However, it is worth noting that most studies on DOM and heavy metals focus on raw water from a single water source, while systematic and synchronous studies on raw water from multiple water sources are still insufficient, which limits the comprehensive understanding of the relationship between DOM and heavy metals in raw water from different types of water sources [Citation20,Citation23].
To address these gaps in knowledge, we chose 159 drinking water sources (Anhui province, China) including rivers, lakes, reservoirs and groundwaters as a representative case. Technically, ultraviolet-visible (UV-vis) absorption spectroscopy and three-dimensional fluorescence spectroscopy (3D-EEM) were used to explore the spectral characteristics of DOM, and inductively coupled plasma mass spectrometry (ICP-MS) was adopted to determine the occurrence of heavy metals. Based on these, our study aims to comprehensively illustrate the occurrence characteristics of DOM and its relationship with heavy metals in different types of drinking water sources. This will contribute to a more systematic understanding of DOM in drinking water sources and its indicative significance for typical pollutants.
2. Materials and methods
2.1. Study area and sampling
The research sites are situated in Anhui Province, within the Yangtze River Delta region of East China. Its geospatial coordinates range from 114°54’to 119°37’ east longitude and 29°41’to 34°38’ north latitude [Citation24]. In the winter of 2022, water samples were collected from the 159 representative drinking water sources, comprising 41 reservoirs, 13 lakes, 74 rivers, and 31 groundwater (). These sites are geographically associated with the Yangtze River, Huai River, and Xin’an River basins. Specific names and locations are listed in Table S1. Surface water samples (rivers, lakes and reservoirs) were collected 0.5-1 m below the surface and transferred in triplicates to 5 L sample drums. Thirty-one samples of shallow groundwater (5 to 10 m) were collected from the pumping well using a groundwater sampler, which was also transferred in t triplicates to a 5 L sample barrel. To maintain the integrity of the samples, they were carefully stored in ice packs at 4°C before being transported to the laboratory. The water sample is then filtered using a 0.45 µm aqueous filter membrane in the filter for subsequent fractionation.
2.2. Spectral detection
The UV-vis absorption spectrometry was conducted using the Cary 60 instrument from Agilent Company in the United States. Milli-Q water served as the blank, and a 1 cm quartz colorimetric plate was utilized for scanning within the 200–800 nm range at 1 nm intervals. For 3D-EEM, a Japan Hitachi F-4600 fluorescence spectrometer equipped with a 150 W ozone-free xenon lamp (PMT voltage = 400 V) was employed. Analysis of the 3D-EEM data utilized the Parallel Factor Analysis (PARAFAC) method in conjunction with the Dreemflour toolbox [Citation25]. The analytical process involved blank deduction, internal filtration effect, Raman correction, and subsequent disassembly analysis to derive the component diagram and the maximum fluorescence intensity for each component [Citation26]. Furthermore, UV spectral indices were computed using three representative parameters: a (350), SR, and SUVA254. Fluorescence spectral indices comprised the HIX, FI, and BIX [Citation27]. Refer to the supplementary information for detailed calculations of the spectral indices.
2.3. Chemical analysis
Water quality measurements and essential environmental factors were systematically conducted in both laboratory and field settings. In brief, on-site pH values were directly analyzed. Laboratory parameters, including NH3-N, potassium permanganate (CODMn), nitrate, volatile phenol (VOPs), fluoride, chloride, and sulfide, were assessed according to the standard methods (State Environmental Protection Administration of China, 2002). The determination of DOC was also determined by Multi N/C3000. All chemical reagents utilized in this study were of analytical grade and sourced from China Sinopharm Chemical Reagents Co., LTD.
2.4. Heavy metal(loid) detection
A minute quantity of ultra-pure HNO3 control solution with a pH below 2 was introduced into the water sample in advance, followed by the commencement of heavy metal detection. Fifteen heavy metals (V, Cr, Mn, Co, Ni, Cu, Zn, As, Se, Mo, Cd, Sb, Ba, TI and Pb) were specifically chosen as representative metal pollutants in water environmental systems. The metal content in the aqueous solution was determined through inductively coupled plasma mass spectrometry (ICP-MS) (Thermo Scientific, U.S.A.), following the standard method outlined in HJ 700–2014. Refer to supplementary material for specific determination methods.
2.5. Data analysis
Parallel Factor Analysis (PARAFAC) for the fluorescent dissolved organic matter (FDOM) was specifically executed using Matlab 2022a. Correlation analysis, one-way Kruskal-Wallis ANOVA test (K-W test) and visualization were performed using SPSS 21.0 and OriginPro 2021. Principal Component Analysis (PCA) and Redundancy Analysis (RDA) were conducted based on Canoco 5. Furthermore, the ‘vegan’ package within the R Studio platform was utilized to analyze structural composition between different groups. All the results with the p level of 0.05 were considered to have statistical significance.
3. Results and discussion
3.1. Spectral characteristics of DOM in the various drinking water sources
The DOC content varied across different drinking water sources, ranging from 1.60 to 43.9 mg/L in rivers, 8.10 to 27.8 mg/L in lakes, 3.60 to 70.8 mg/L in reservoirs, and 0.30 to 10.70 mg/L in groundwater. For surface water, the mean concentration of lakes was significantly higher than that of rivers and reservoirs (K-W test, p < 0.01) () Among these, rivers exhibited the lowest mean concentration, possibly attributed to the enrichment of DOM in static water bodies such as lakes and reservoirs [Citation28]. In addition, the water bodies of lakes exhibited a greater degree of perturbation attributable to human activities compared to reservoirs, thereby resulting in elevated levels of DOC content [Citation29]. The reservoirs serving as the drinking water source in Anhui are predominantly situated in towns and villages, characterized by smaller populations and lower levels of human activity in their vicinity. Conversely, rivers and lakes located mostly within urban areas experience urbanization and urban development, leading to shifts in land use and the introduction of numerous human activities and pollution sources [Citation30, Citation31]. These may include industrial and municipal sewage, as well as waste generated from human activities [Citation32, Citation33]. Additionally, overfishing activities are common in Anhui’s drinking water source lakes [Citation34]. All these human activities contribute to fluctuations in DOC content in lake water. At the same time, it is observed that the DOC content in the three manifestations of surface water exceeds that found in the groundwater (K-W test, p < 0.01). Broadly speaking, DOC serves as an indicative measure reflecting the prevalence of DOM in the effluent environment to a certain extent [Citation35]. In simpler terms, the occurrence level of DOM in the surface water of the drinking water sources significantly surpasses that in groundwater. Differences in organic matter content between surface water and groundwater affect water quality, impacting biodiversity, ecological function, and aquatic ecosystem stability in Anhui Province’s drinking water sources [Citation36]. Higher organic content in surface water fosters plant and plankton growth, increasing eutrophication risks and disrupting the ecological balance in areas reliant on surface water for drinking [Citation37].
Figure 2. The distribution of ultraviolet spectral index (a) and fluorescence spectral (b) in various drinking water sources.
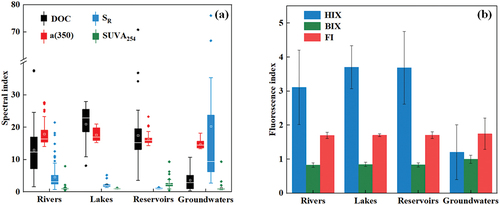
In addition, it is discernible that the concentration of absorption coefficient at 350 nm (a (350)) and specific UV absorption at 254 nm (SUVA254) exhibited no significant variations across diverse water sources () (K-W test, p > 0.05). This suggests that the distribution of CDOM occurrence levels and the degree of DOM aromatization are relatively uniform in drinking water sources [Citation38], with minimal disparity between surface water and groundwater. In contrast, high SR content determined that groundwater had a lower DOM molecular weight [Citation39], while surface water had a higher DOM molecular weight (K-W test, p < 0.01). Simultaneously, substantial differences are observed among the three forms of surface water (K-W test, p < 0.01), with the molecular weight of DOM in the river being the lowest (). These phenomena may be attributed to the fact that groundwater undergoes filtration through the soil and geological layers compared to the other water sources, resulting in a lower CDOM occurrence level in groundwater [Citation40]. Lakes and rivers, being more influenced by external environmental factors, and reservoirs, largely static water bodies, potentially contribute to an increase in DOM molecular weight [Citation41].
The DOM fluorescence index of various water sources was also examined. The HIX value of the groundwater was significantly lower than surface water (K-W test, p < 0.01), indicating a low degree of DOM humification in groundwater (). Simultaneously, HIX values across the three categories of surface water displayed significant differences, mirroring the patterns observed in DOM molecular weight. Reservoirs and lakes exhibited a higher degree of humification, possibly attributable to their lower mobility and more straightforward static enrichment [Citation28]. On the contrary, the biological index (BIX) showed the highest content in groundwater (K-W test, p < 0.01), and specific sites registered values surpassing 1, indicating a higher autogenetic component in groundwater [Citation42]. In addition, a significant negative correlation was found between the HIX and BIX (R2 = −0.663, p < 0.01). This suggested that the lower the degree of DOM satisfying humification in the water environment, the higher the proportion of biogenic DOM [Citation43]. Notably, the values of BIX for the four types of water samples were higher than 0.6, with the fact that the BIX values of rivers, lakes, reservoirs and groundwater were in the ranges of 0.71–1.04, 0.79–1.02, 0.72–1.05 and 0.78–1.27 respectively. These results indicated that drinking water sources had strong autogenetic components. On the contrary, it can be seen that the average HIX of the four types of water sources is less than 4, which means that the degree of DOM humification is generally low and is mainly generated by biological activities [Citation44]. The mean value of FI is mainly between 1.6 and 1.9, with only a few points in groundwater exceeding 1.9. These results suggest that the humus source comprises both endogenous microbial activity and certain terrestrial input, with groundwater being more influenced by endogenous microbial activity [Citation45].
3.2. Major fluorescent DOM components and source apportionment
The fluorescent components were identified through EEM-PARAFAC analysis, yielding three distinct components (Fig. S1). These components comprise two humic acid-like components (C1 and C2) and one protein-like component (C3). C1 exhibits peaks at 260/475 nm, traditionally designated as peak C and recognized as a terrestrial humus-like component [Citation46]. Terrestrial humus is primarily introduced into water bodies through various pathways, including organic matter runoff from land due to soil erosion, rainfall, and human activities [Citation47]. C2 displays an Ex/Em peak at 250/390 nm, traditionally identified as an M peak, representing humus material influenced by specific microorganisms [Citation48]. Humus material processed by certain microorganisms primarily originates from plant residues and deceased organisms [Citation49]. Additionally, organic matter generated through the metabolic processes of phytoplankton, algae, bacteria, and other aquatic organisms within the water body undergoes degradation and transformation by microorganisms, contributing to the formation of this humus [Citation50]. C3 demonstrates an Ex/Em peak at 270/340 nm, comparable to the T peak, and is considered a protein-like substance. It represents polypeptide substances produced by microbial activities. Factors such as human activities, pollution, or algae eutrophication can elevate C3 components, a phenomenon commonly observed in various aquatic environments [Citation51].
Generally, the peak fluorescence intensities of C1, C2, and C3 in surface water were significantly higher than those in groundwater (K-W test, p < 0.01), suggesting a lower presence of protein and humic material in groundwater compared to surface water (). Groundwater typically exhibits fewer impurities than surface water due to its filtration through underground rock formations and soil [Citation52]. Essentially, rock and soil act as a filtration layer, adsorbing organic material and filtering it out, thereby reducing the organic matter content in groundwater. Elevated levels of C1, C2, and C3 in surface water can lead to increased turbidity, potentially impacting aquatic plant photosynthesis and promoting algae overgrowth, thus causing water eutrophication [Citation53, Citation54]. In contrast, groundwater typically contains lower levels of these components, resulting in better water quality [Citation55]. This discrepancy contributes to varying water quality changes and ecological dynamics across different water bodies, consequently affecting the overall water quality and ecological functions of Anhui Province’s drinking water sources. Currently, there is a push to prioritize the use of surface water over groundwater for drinking purposes to conserve groundwater resources, maintain regional ecological balance, and safeguard biodiversity and ecosystem health [Citation32]. To effectively implement this initiative, subsequent water management practices must further control the occurrence of DOM levels in surface water. Furthermore, concerning surface water, discernible variations in the forms of existence of C1, C2, and C3 components were evident among different water sources (K-W test, p < 0.01). Notably, both C1 and C2 exhibit the highest concentrations in lakes, particularly in terrestrial humus. This may be attributed to organic matter from surrounding soil and vegetation entering lake water bodies via surface runoff and forming humus with elevated content after microbial decomposition [Citation56]. Lakes, being static water bodies with prolonged hydraulic retention times compared to rivers, could accumulate higher organic matter content over time [Citation57]. Additionally, in contrast to reservoirs, most of the lakes included in the study are situated in urban areas and county towns with heightened human activities. These encompass diverse industrial and municipal discharges, as well as the accumulation of refuse and waste from anthropogenic sources, alongside intensified fishing practices in lake ecosystems [Citation32–34]. Increased human activities could contribute to higher organic matter inflow into lakes [Citation58]. At the same time, the maximum fluorescence intensity of the C3 component is higher in lakes and rivers but relatively lower in reservoirs (K-W, p < 0.01). As previously mentioned, human pollution or algae eutrophication can elevate C3 components [Citation51]. Given that rivers and lakes are more impacted by human activities, a resultant increase in C3 content is expected. Moreover, rivers and lakes, with their more intricate ecosystems, experience greater biological activity, potentially leading to higher protein content [Citation59]. Overall, lake water bodies exhibit higher levels of humus occurrence. Serving as a nutrient source for aquatic plants, humus stimulates their growth, consequently influencing the balance of the water ecosystem [Citation60]. Rivers and lakes tend to have relatively higher protein content, which can contribute to the excessive growth of aquatic plants, leading to water body eutrophication [Citation37]. In essence, fluctuations in DOM components impact the overall water quality and ecological dynamics of surface water bodies to a certain extent.
Figure 3. Fluorescence intensity (a) and principal component analysis (b) in various drinking water sources.
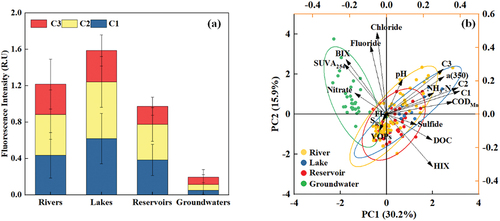
Simultaneously, the basic water quality of 159 drinking water sources was analyzed. The variations in basic water quality parameters of the four existing water sources are depicted in Fig.S2. Significant distinctions emerged between surface water and groundwater in CODMn, NH3-N, fluoride, chloride, and nitrate content (K-W test, p < 0.01). Notably, CODMn and NH3-N manifested variations within surface water (K-W test, p < 0.01), particularly with elevated levels in lakes. This occurrence could be attributed to the heightened susceptibility of lakes to eutrophication, resulting in increased NH3-N content [Citation61]. Moreover, CODMn levels might be associated with manganese oxidation in oxygen-rich lake environments [Citation62]. Groundwater exhibited the highest levels of fluoride, chloride, and nitrate (K-W test, p < 0.01), with the collection area primarily concentrated in the northern region of Anhui Province. Geological structures in this area likely influenced the dissolution and release of fluoride and chloride. The prevalence of intensive agriculture in these regions may have contributed to elevated nitrate nitrogen levels in groundwater [Citation63]. Furthermore, VOPs and sulfide content were predominantly below detection limits, indicating minimal presence in drinking water sources. The pH values of the four water sources remained relatively stable and within a neutral range (K-W test, p > 0.05). To further investigate the pollutant source for the drinking water sources, Principal Component Analysis (PCA) was used to analyze DOM-related indicators (DOC, a350, HIX, BIX, FI, SUVA254 and PARAFAC components) and water quality indicators (pH, CODMn, chloride, fluoride, VOPs and sulfide). PCA contributes to 46.1% of the variation in DOM (). The first principal component, PC1, explained 30.2% of the variation, while the second principal component, PC2, explained 15.9% of the variation. It can be seen that the composition of DOM varies significantly. PC1 is predominantly explained by microbial and terrestrial humus-like components, along with HIX and CODMn. Conversely, PC2 is influenced by chloride, fluoride, and BIX. Therefore, PC1 May be dominated by exotic DOM, representing more complex compounds. On the other hand, PC2 May be related to biological sources, encompassing local DOM from phytoplankton production and benthic flux. Four distinct clusters were identified, with groundwater primarily associated with PC2, whereas reservoirs, rivers, and lakes were linked to PC1 (p < 0.01). In other words, DOM in groundwater may be more self-generated and less affected by external activities than in reservoirs, rivers, and lakes.
3.3. The correlation between water quality and DOM
Simultaneously, the correlation between water quality parameters and DOM is analyzed, and Redundancy Analysis (RDA) is conducted for the four types of water sources. The RDA reveals that 31.1% of the variation in river DOM can be elucidated by input environmental parameters (). These parameters encompass three pivotal environmental variables: nitrate, CODMn, and VOPs. Specifically, nitrate explains 14.2% (p = 0.002), CODMn explains 8.2% (p = 0.004), and VOPs explain 5.4% (p = 0.016) of the observed variation. These outcomes underscore the substantial impact of these variables on the distribution of DOM in drinking water sources, with a particular emphasis on nitrate. Importantly, nitrate serves as an indicative parameter reflecting the eutrophication status of the water body, thereby influencing both the sourcing and degradation processes of organic matter [Citation64]. Building on this foundation, the strong correlation between nitrate and DOM has the potential to influence water eutrophication processes, posing a detrimental impact on the health of riverine aquatic organisms and ecosystems. Concurrently, nitrate in river water primarily originates from agricultural discharge and domestic sewage, among other exogenous inputs, while DOM in rivers is also largely influenced by external sources [Citation65] (). The robust correlation between nitrate and DOM suggests that the water body is impacted by pollution sources such as agricultural discharge and domestic sewage. In the case of lakes, fluoride and chloride emerge as the predominant variables, collectively accounting for 36.0% (p = 0.016) and 16.5% (p = 0.038), respectively, with fluoride exhibiting a more substantial effect (). It is imperative to note that while fluoride does not directly influence DOM, its presence in the water environment indirectly affects alterations in lake water DOM [Citation66]. Based on this, the correlation between fluoride and DOM may affect the overall environmental change of the lake water body, and then indirectly affect the health of the lake ecosystem. In addition, it can be seen from the above that the DOM of the lake water body is greatly influenced by exogenous factors, and fluoride mainly comes from external inputs [Citation67] (). Similar to river bodies, the high correlation suggests that lake bodies are also affected by exogenous inputs. Furthermore, RDA indicates that 31.5% of the variation in reservoir DOM is explicable by environmental parameters, with only one significant variable, NH3-N, elucidating 20.6% (p = 0.006) (). In essence, the strong correlation between DOM and NH3-N can influence the occurrence levels of NH3-N within reservoir water bodies, thereby impacting the overall environmental status and ecological health to some extent. Like other surface waters, DOM and NH3-N in reservoirs are primarily influenced by external inputs, suggesting indirect impacts of exogenous pollution on reservoir water bodies [Citation68] (). For groundwater, RDA highlights that 33.8% of DOM variations can be explained by environmental parameters, with chloride being the primary explanatory variable, elucidating 26% (p = 0.016) (). The relatively high chloride content in groundwater may lead to the formation of complexes with certain metals [Citation69]. The creation of these complexes can influence the interaction between metals and DOM, thereby indirectly affecting the distribution and stability of DOM. On this basis, these complexes may increase the solubility of metals, affect the migration of metals in groundwater, and increase the toxicity of metals to organisms in groundwater [Citation70]. This could have a detrimental impact on the overall ecosystem of groundwater. In addition, alterations in the interaction between DOM and metals may also result in the release or deposition of heavy metals, affecting the quality of groundwater [Citation71]. If the metal is released into groundwater, it can also lead to groundwater contamination and pose a threat to drinking water sources, thereby endangering human health [Citation72]. In summary, the key factors influencing DOM vary across different water sources. Nitrate, fluoride, and NH3-N are pivotal in rivers, lakes, and reservoirs, with significant positive correlations observed with most DOM parameters. Conversely, chloride, the primary environmental variable in groundwater, exhibited significant negative correlations with most DOM parameters. This underscores the disparities in the interaction between DOM and water quality in surface water and groundwater. Additionally, for surface water, nitrate and fluoride in rivers and lakes exhibited significant negative correlations with DOC, while NH3-N in reservoirs demonstrated a positive correlation. The heightened susceptibility of rivers and lakes to external influences, coupled with their more intricate sources, potentially explains the negative correlation between these environmental variables and DOC [Citation73]. In essence, the distinctive characteristics of different water sources significantly influence the interplay between DOM and water quality. Given the significant relationship between DOM and water quality parameters, it is imperative to implement regular monitoring of DOM occurrence and composition in subsequent water management practices. This approach allows for a deeper understanding of DOM sources, patterns of change, and its influence on water ecosystems. Moreover, such monitoring aids in assessing the quality of drinking water bodies to a certain extent. Simultaneously, in the realm of ecosystem protection, there is a pressing need to intensify efforts to safeguard the surrounding environments of water bodies. This includes reducing the input of organic matter and implementing measures to uphold the health of regional water quality and ecological balance.
3.4. Heavy metal contents in drinking water of different forms in Anhui Province
The distribution of heavy metals in each drinking water source is delineated (), presenting the specific contents of V, Cr, Mn, Co, Ni, Cu, Zn, As, Se, Mo, Cd, Sb, Ba, Ti, and Pb in Table S2. The total heavy metal content across rivers, lakes, reservoirs, and groundwater ranged from 4.55 to 142.28 mg/L (mean 64.03 mg/L), 13.58 to 135.92 mg/L (mean 75.72 mg/L), 8.41 to 465.02 mg/L (mean 70.69 mg/L), and 30.76 to 169.14 mg/L (mean 88.64 mg/L), respectively. Among the four types of drinking water sources, Ba content notably stands out as the highest, consistent with numerous studies indicating generally elevated Ba levels in water bodies [Citation74, Citation75]. Following this, As content emerges as the second-highest in rivers, while Mn content ranks as the second-highest in lakes and reservoirs. This divergence could be attributed to reduced water flow rates in lakes and reservoirs, allowing for prolonged accumulation and dissolution of Mn [Citation76, Citation77]. Moreover, the higher Mn and Mo content in groundwater may be associated with the geological structure [Citation78]. Furthermore, groundwater exhibited higher heavy metal content than surface water (K-W test, p < 0.01), a pattern observed in mangrove wetlands, the Pearl River Delta, and Nigeria [Citation79–81]. This discrepancy is likely influenced by soil and mineral deposits, where heavy metal elements enter groundwater through natural settling, resulting in localized heavy metal enrichment [Citation82]. Conversely, no significant differences were observed among the three types (K-W test, p > 0.05), indicating a more uniform distribution of heavy metals in surface water.
Figure 5. Distribution of heavy metals (a) and the structural composition (b) in various drinking water sources.
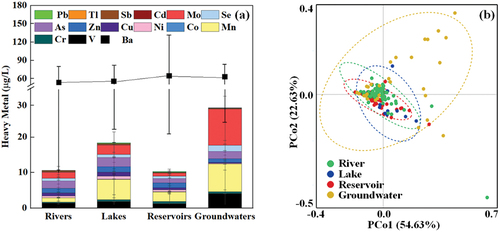
At the same time, an analysis of the structural composition characteristics of heavy metals in various water sources was undertaken. The percentage composition of each heavy metal content in the total heavy metal content was determined, and sequence analysis of reservoirs, lakes, rivers, and groundwater systems was conducted using Principal Coordinate Analysis (PCoA) (). The graphical representation indicates that the distribution of heavy metals in groundwater was notably more dispersed, whereas the distribution in surface water was denser. This observation suggests discernible differences in the structural composition of heavy metals between surface water and groundwater. Further statistical analysis using the One-Anosim test confirmed significant differences among the four systems (p < 0.01) (Fig.S3). Specifically, there were noteworthy distinctions in the distribution of heavy metals between reservoirs and rivers, reservoirs and groundwater, groundwater and lakes, and groundwater and rivers (p < 0.01). Despite the absence of a significant difference in heavy metal content between reservoirs and rivers, a significant difference was identified in their structural composition. Conversely, groundwater and surface water also show significant differences in the composition of heavy metals. Furthermore, the SIMPER test based on Bray-Curtis demonstrated that Pb (p = 0.005), Ba (p = 0.001), Mn (p = 0.006), and Ba (p = 0.01) contributed the most to the differences observed in the pairs of reservoir and river, reservoir and groundwater, groundwater and lake, and groundwater and river, respectively. These phenomena indicate that the distribution of these metals could alter the composition of heavy metals in the corresponding drinking water source to a certain extent.
3.5. The indicative role of DOM in heavy metals
The significance of DOM in influencing the presence and mobility of heavy metals in water bodies has been extensively documented [Citation83, Citation84]. Spearman correlation analyses were conducted to delve deeper into the intricate relationship between DOM and heavy metals across distinct water sources, integrating a variety of DOM compositions and heavy metal data. The findings revealed a notable correlation between heavy metal concentrations and DOM across the four types of drinking water sources (). Generally, a significant positive correlation between surface water and DOM was observed, while groundwater exhibited a negative correlation. This disparity can be attributed to surface water’s heightened vulnerability to pollution, stemming from direct atmospheric exposure, interaction with surrounding land, and the influx of pollutants from human activities [Citation85]. Soil erosion and sedimentation during such events may introduce organic matter, along with carried heavy metals, into rivers, lakes, and reservoirs, leading to the coexistence of DOM and heavy metals in water bodies [Citation86, Citation87]. Moreover, a notable positive association between SR and heavy metals was observed in both groundwater and surface water, suggesting that the molecular weight of DOM consistently influences heavy metal concentrations across various water sources. When analyzing surface water from rivers, lakes, and reservoirs, similarities and differences in the correlation between DOM and heavy metals were noted. Components C1, C2, and C3 exhibited a significant positive correlation with heavy metals in all three water sources, particularly with V, Ni, and As, highlighting the considerable influence of FDOM on the distribution of these heavy metals. Conversely, DOC and FI displayed a strong positive correlation with heavy metals only in reservoirs, indicating that heavy metals in reservoirs could be influenced by the DOC content and fluorescence index in the water body, underscoring variations in the influence of DOM on heavy metals across different water sources.
Figure 6. Correlation heat maps of heavy metals and DOM in rivers (a), lakes (b), reservoirs (c) and groundwaters (d).
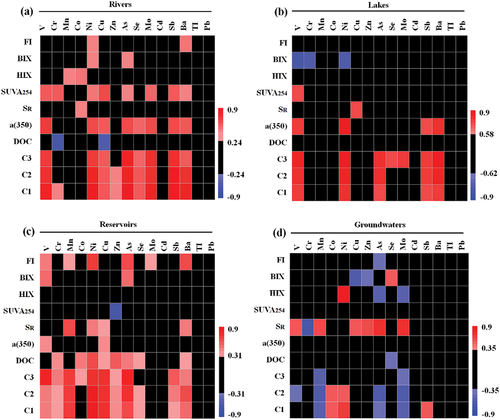
Furthermore, while ICP-MS equipment is efficacious, it is relatively costly, demanding substantial investments in equipment, personnel training, and ongoing maintenance [Citation88]. Its complex operational procedures and susceptibility to interfering substances or isotopes can impede the analysis process, necessitating considerable time and effort. In contrast, DOM spectroscopy emerges as a more straightforward and accessible alternative. The simplicity of its sample preparation and measurement procedures makes spectroscopy conducive to swift analysis and large-scale testing [Citation81]. Given that DOM spectroscopy can swiftly reveal the occurrence of heavy metals, it stands to significantly decrease costs. As discussed previously, a notable correlation existed between the content of heavy metals in both surface water and groundwater (). From an analytical chemistry perspective, DOM spectroscopy could reasonably reflect the content of heavy metals. Specifically, various spectral indices effectively indicated the presence of 13 different heavy metals in river water, with Ni, As, and Ba proving to be the most significant. Conversely, the elements Cd, Ti, and Pb were not reliably indicated by DOM, a consistent pattern observed across lakes, reservoirs, and groundwater. The extremely low concentrations of Cd, Ti, and Pb in all water sources may have hindered DOM recognition. In contrast to lakes and rivers, only a fraction of heavy metals could be effectively indicated, with Cr, Cu, Se, and Mo primarily influenced by a single spectral index, implying that the content of these heavy metals in lakes could be more accurately indicated by a singular spectral index. Similarly, various spectral indices effectively indicated 13 types of heavy metals in reservoirs, with Mn, Ni, As, and Ba proving to be the most significant. Unlike surface water, numerous indices in groundwater displayed a negative correlation with heavy metals. This implied that these indices could inversely indicate the content of heavy metals to a certain extent, with As and Mo being the most significant. Diverging from surface water, a (350) was unable to reflect the heavy metal content in groundwater. Additionally, DOM could not effectively indicate Ba content in groundwater, highlighting the divergent indicative meanings between surface water and groundwater. In summary, our findings affirmed that DOM served as a reliable indicator of heavy metal content in drinking water sources, whether in reservoirs, lakes, rivers, or groundwater. However, it should be noted that low levels of heavy metals might not have been as effectively indicated. While our study primarily focused on drinking water sources in Anhui Province, it holds indicative significance and establishes a foundation for further research on the intricate relationship between DOM and heavy metals in water sources.
4. Conclusion
Our study unveiled distinctions in DOM and heavy metals among four types of drinking water sources. Specifically, the concentrations of DOC in the surface water were higher than that in the groundwater. However, there was no significant difference in the occurrence of CDOM among different drinking water sources. Terrestrial humus-like components (C1), microbial humic-like (C2), and protein-like components (C3) were identified using the PARAFAC. The occurrence of C1, C2, and C3 in the groundwater was markedly lower than that in the surface water. Among the surface water, the occurrence of these components was the highest in the lakes. Additionally, nitrate, fluoride, NH3-N, and chloride influenced DOM distribution in rivers, lakes, reservoirs, and groundwater, respectively. Although the total concentration of heavy metals in the groundwater was significantly higher than that in the surface water, there were no differences in the total concentration of heavy metals among surface water. Spearman correlations indicated that DOM may potentially affect the environmental behavior of heavy metals, and DOM in drinking water sources could serve as a reliable indicator of heavy in various drinking water sources. Taken together, the occurrence and indicative roles of DOM in different drinking water sources exhibit diverse patterns, which need to be treated differently.
Authors’ contributions
Min Zhang and Yihan Chen conceived of the presented idea. Material preparation, data collection and analysis were performed by Chao Zhu, Ke Wu and Dongmei Xu. The first draft of the manuscript was written by Chao Zhu and Ke Wu. Xiaofei Tang, Yaqin Hu commented on previous versions of the manuscript. Md Tanbir Hassan was in charge of the language revision. All authors read and approved the final manuscript.
Supplemental Material
Download MS Word (405.4 KB)Disclosure statement
No potential conflict of interest was reported by the author(s).
Data availability statement
The datasets generated during and/or analyzed during the current study are available from the corresponding author on reasonable request.
All data generated or analyzed during this study are included in this published article (and its supplementary information files).
Supplementary material
Supplemental data for this article can be accessed online at https://doi.org/10.1080/26395940.2024.2367429
Additional information
Funding
References
- Huo P, Zhang W, Jia H, et al. Characteristics of optical properties of DOM and nutrients in rainwater of different ecological areas of a large reservoir in China. Chemosphere. 2023;342:140091. doi: 10.1016/j.chemosphere.2023.140091
- Liu Q, Jiang Y, Tian Y, et al. Impact of land use on the DOM composition in different seasons in a subtropical river flowing through a region undergoing rapid urbanization. J Clean Prod. 2019;212:1224–14. doi: 10.1016/j.jclepro.2018.12.030
- Liu D, Du Y, Yu S, et al. Human activities determine quantity and composition of dissolved organic matter in lakes along the Yangtze River. Water Res. 2020;168:115132. doi: 10.1016/j.watres.2019.115132
- Song X, Zhao M, Chen A, et al. Effects of input of terrestrial materials on photodegradation and biodegradation of DOM in rivers: the case of Heilongjiang River. J Hydrol. 2022;609:127792. doi: 10.1016/j.jhydrol.2022.127792
- Gucker B, Silva RCS, Graeber D, et al. Urbanization and agriculture increase exports and differentially alter elemental stoichiometry of dissolved organic matter (DOM) from tropical catchments. Sci Total Environ. 2016;550:785–792. doi: 10.1016/j.scitotenv.2016.01.158
- Zhu Y, Chen H, Jia Q, et al. Interactions of anthropogenic and terrestrial sources drive the varying trends in molecular chemodiversity profiles of DOM in urban storm runoff, compared to land use patterns. Sci Total Environ. 2022;817:152990. doi: 10.1016/j.scitotenv.2022.152990
- Wang K, Ren H, Yuan S, et al. Exploring the diversity of dissolved organic matter (DOM) properties and sources in different functional areas of a typical macrophyte - derived lake combined with optical spectroscopy and FT-ICR MS analysis. J Environ Sci. 2023;147:462–473. doi: 10.1016/j.jes.2023.11.027
- Chen Y, Yu K, Zhou Y, et al. Characterizing spatiotemporal variations of chromophoric dissolved organic matter in headwater catchment of a key drinking water source in China. Environ Sci Pollut Res. 2017a;24(36):27799–27812. doi: 10.1007/s11356-017-0307-5
- Zhou Y, Zhou L, Zhang Y, et al. Unraveling the role of anthropogenic and natural drivers in shaping the molecular composition and biolability of dissolved organic matter in non-pristine lakes. Environ Sci Technol. 2022;56(7):4655–4664. doi: 10.1021/acs.est.1c08003
- Fouda-Mbanga BG, Seyisi T, Nthwane YB, et al. A review on pollutants found in drinking water in Sub-Sahara African rural communities: detection and potential low-cost remediation methods. Ind Domestic Waste Manag. 2023;3(2):67–89. doi: 10.53623/idwm.v3i2.264
- New WX, Kristanti RA, Manik H, et al. Occurrence of microplastics in drinking water in South East Asia: a short review. Tropical Environ Biol Technol. 2023;1(1):14–24. doi: 10.53623/tebt.v1i1.221
- Azzam MA, Khan MR, Youssef HM. Drinking water as a substantial source of toxic alkali, alkaline and heavy metals: toxicity and their implications on human health. J King Saud Univ Sci. 2023;35(6):102761. doi: 10.1016/j.jksus.2023.102761
- Huang M, Zhou M, Li Z, et al. How do drying-wetting cycles influence availability of heavy metals in sediment? A perspective from DOM molecular composition. Water Res. 2022;220:118671. doi: 10.1016/j.watres.2022.118671
- Maharjan AK, Wong DRE, Rubiyatno R. Level and distribution of heavy metals in Miri River, Malaysia. Tropic Aquat Soil Pollut. 2021;1(2):74–86. doi: 10.53623/tasp.v1i2.20
- Yahaya T, Balogun K, Danlami MB, et al. Human safety evaluation of heavy metals, physicochemical parameters, and microorganisms in lagoon water at Ikorodu lighter terminal in Lagos, Nigeria. Tropic Aqua Soil Pollut. 2023;3(1):58–68. doi: 10.53623/tasp.v3i1.200
- Yahaya T, Chidi O, Abdulrahman S, et al. Health risk assessment of heavy metals, physicochemical properties and microbes in groundwater near Igando dumpsite in Lagos, Nigeria. Ind Domestic Waste Manag. 2024;4(1):1–13. doi: 10.53623/idwm.v4i1.375
- Kumar V, Parihar RD, Sharma A, et al. Global evaluation of heavy metal content in surface water bodies: a meta-analysis using heavy metal pollution indices and multivariate statistical analyses. Chemosphere. 2019;236:124364. doi: 10.1016/j.chemosphere.2019.124364
- Liang E, Li J, Li B, et al. Roles of dissolved organic matter (DOM) in shaping the distribution pattern of heavy metal in the Yangtze River. J Hazard Mater. 2023;460:132410. doi: 10.1016/j.jhazmat.2023.132410
- Geng C, Dong Z, Zhang T, et al. Advances in atmospheric pressure plasma-based optical emission spectrometry for the analysis of heavy metals. Talanta. 2024;270:125634. doi: 10.1016/j.talanta.2024.125634
- Li L, Cao X, Wu P, et al. Spatio-temporal characterization of dissolved organic matter in karst rivers disturbed by acid mine drainage and its correlation with metal ions. Sci Total Environ. 2023;897:165434. doi: 10.1016/j.scitotenv.2023.165434
- Wang Z, Han R, Muhammad A, et al. Correlative distribution of DOM and heavy metals in the soils of the Zhangxi watershed in Ningbo city, East of China. Environ Pollut. 2022;299:118811. doi: 10.1016/j.envpol.2022.118811
- Lee MH, Osburn CL, Shin KH, et al. New insight into the applicability of spectroscopic indices for dissolved organic matter (DOM) source discrimination in aquatic systems affected by biogeochemical processes. Water Res. 2018;147:164–176. doi: 10.1016/j.watres.2018.09.048
- Dong L, Zhang J, Guo Z, et al. Distributions and interactions of dissolved organic matter and heavy metals in shallow groundwater in Guanzhong basin of China. Environ Res. 2022;207:112099. doi: 10.1016/j.envres.2021.112099
- Xu Y, Hao S, Cui Y, et al. Analysis of the spatiotemporal expansion and pattern evolution of urban areas in Anhui Province, China, based on nighttime light data. Ecological Indicators. 2023;157:111283. doi: 10.1016/j.ecolind.2023.111283
- Stedmon CA, Bro R. Characterizing dissolved organic matter fluorescence with parallel factor American society of limnology and oceanography methods 6. Limnology Oceanography: Methods. 2008;6(11):572–579. doi: 10.4319/lom.2008.6.572b
- Aftab B, Hur J. Fast tracking the molecular weight changes of humic substances in coagulation/flocculation processes via fluorescence EEM-PARAFAC. Chemosphere. 2017;178:317–324. doi: 10.1016/j.chemosphere.2017.03.068
- Wu K, Cui K, Huang Y, et al. Degree of human activity exert differentiated influence on conventional and emerging pollutants in drinking water source. Environ Sci Pollut Res. 2023;31(5):1–13. doi: 10.1007/s11356-023-31440-5
- Liu Q, Jiang Y, Huang X, et al. Hydrological conditions can change the effects of major nutrients and dissolved organic matter on phytoplankton community dynamics in a eutrophic river. J Hydrol. 2024;628:130503. doi: 10.1016/j.jhydrol.2023.130503
- Zheng L, Jiang C, Chen X, et al. Combining hydrochemistry and hydrogen and oxygen stable isotopes to reveal the influence of human activities on surface water quality in Chaohu Lake Basin. J Environ Manage. 2022;312:114933. doi: 10.1016/j.jenvman.2022.114933
- Luo Z, Shao Q, Zuo Q, et al. Impact of land use and urbanization on river water quality and ecology in a dam dominated basin. J Hydrol. 2020;584:124655. doi: 10.1016/j.jhydrol.2020.124655
- Xie C, Huang X, Mu H, et al. Impacts of land-use changes on the lakes across the Yangtze floodplain in China. Environ Sci Technol. 2017;51(7):3669–3677. doi: 10.1021/acs.est.6b04260
- Chen K, Liu Q, Jiang Q, et al. Source apportionment of surface water pollution in North Anhui Plain, Eastern China, using APCS-MLR model combined with GIS approach and socioeconomic parameters. Ecologic Indicat. 2022;143:109324. doi: 10.1016/j.ecolind.2022.109324
- Xu W, Liu Z. Evaluation on the current situation of integrated treatment of rural sewage in China: a case study of Anhui Province. Water. 2023;15(3):415. doi: 10.3390/w15030415
- Zhang H, Kang M, Shen L, et al. Rapid change in Yangtze fisheries and its implications for global freshwater ecosystem management. Fish Fisher. 2020;21(3):601–620. doi: 10.1111/faf.12449
- Zhao Y. New insight into and characterization of DOC, DON and CDOM for urban waters in the lower reaches of the Yellow River, China. Sci Total Environ. 2024;914:169828. doi: 10.1016/j.scitotenv.2023.169828
- Cao X, Lu Y, Wang C, et al. Hydrogeochemistry and quality of surface water and groundwater in the drinking water source area of an urbanizing region. Ecotoxicol Environ Saf. 2019;186:109628. doi: 10.1016/j.ecoenv.2019.109628
- Siuda W, Grabowska K, Kaliński T, et al. Trophic state, eutrophication, and the threats for water quality of the great Mazurian Lake System. Polish River Basins And Lakes–Part I: Hydrology Hydrochemistry. 2020;86:231–260. doi: 10.1007/978-3-030-12123-5
- Bai Y, Zhang S, Mu E, et al. Characterizing the spatiotemporal distribution of dissolved organic matter (DOM) in the Yongding River Basin: insights from flow regulation. J Environ Manage. 2023;325:116476. doi: 10.1016/j.jenvman.2022.116476
- Tadeu C, Brandão L, Bezerra-Neto J, et al. Photodegradation of autochthonous and allochthonous dissolved organic matter in a natural tropical lake. Limnologica. 2021;87:125846. doi: 10.1016/j.limno.2020.125846
- Hu D, Indika S, Zhong H, et al. Fluorescence characteristics and source analysis of DOM in groundwater during the wet season in the CKDu zone of North Central Province, Sri Lanka. J Environ Manage. 2023;327:116877. doi: 10.1016/j.jenvman.2022.116877
- Kolding J, van Zwieten PA. Relative lake level fluctuations and their influence on productivity and resilience in tropical lakes and reservoirs. Fish Res. 2012;115:99–109. doi: 10.1016/j.fishres.2011.11.008
- Zhao Y, Lin J, Cheng S, et al. Linking soil dissolved organic matter characteristics and the temperature sensitivity of soil organic carbon decomposition in the riparian zone of the Three Gorges Reservoir. Ecological Indicators. 2023;154:110768. doi: 10.1016/j.ecolind.2023.110768
- Chen Y, Yu K, Zhou Y, et al. Characterizing spatiotemporal variations of chromophoric dissolved organic matter in headwater catchment of a key drinking water source in China. Environ Sci Pollut Res Int. 2017b;24(36):27799–27812. doi: 10.1007/s11356-017-0307-5
- Xie R, Qi J, Shi C, et al. Changes of dissolved organic matter following salinity invasion in different seasons in a nitrogen rich tidal reach. Sci Total Environ. 2023;880:163251. doi: 10.1016/j.scitotenv.2023.163251
- Lin Y, Hu E, Sun C, et al. Using fluorescence index (FI) of dissolved organic matter (DOM) to identify non-point source pollution: the difference in FI between soil extracts and wastewater reveals the principle. Sci Total Environ. 2023;862:160848. doi: 10.1016/j.scitotenv.2022.160848
- Harjung A, Ejarque E, Battin T, et al. Experimental evidence reveals impact of drought periods on dissolved organic matter quality and ecosystem metabolism in subalpine streams. Limnol Oceanogr. 2018;64(1):46–60. doi: 10.1002/lno.11018
- Cai X, Lei S, Li Y, et al. Humification levels of dissolved organic matter in the eastern plain lakes of China based on long-term satellite observations. Water Res. 2024;250:120991. doi: 10.1016/j.watres.2023.120991
- Murphy KR, Hambly A, Singh S, et al. Organic matter fluorescence in municipal water recycling schemes: toward a unified PARAFAC model. Environ Sci Technol. 2011;45(7):2909–2916. doi: 10.1021/es103015e
- Wu C, Wu X, Ge X, et al. Characteristics of dissolved organic matter in sediments of typical lakes in Southeastern Hubei Province, China. Int J Environ Res Public Health. 2022;19(12):7402. doi: 10.3390/ijerph19127402
- Liu Q, Tian Y, Liu Y, et al. Relationship between dissolved organic matter and phytoplankton community dynamics in a human-impacted subtropical river. J Clean Prod. 2021;289:125144. doi: 10.1016/j.jclepro.2020.125144
- Berggren M, Gudasz C, Guillemette F, et al. Systematic microbial production of optically active dissolved organic matter in subarctic lake water. Limnol Oceanography. 2019;65(5):951–961. doi: 10.1002/lno.11362
- Gatto B, Furlanetto D, Camporese M, et al. Quantifying groundwater recharge in the Venetian high plain between the Brenta and Piave Rivers through integrated surface–subsurface hydrological modeling. J Hydrology Regional Stud. 2023;50:101550. doi: 10.1016/j.ejrh.2023.101550
- Bai L, Zhang Q, Wang C, et al. Effects of natural dissolved organic matter on the complexation and biodegradation of 17α-ethinylestradiol in freshwater lakes. Environ Pollut. 2019;246:782–789. doi: 10.1016/j.envpol.2018.12.098
- Li D, Pan B, Zheng X, et al. CDOM in the source regions of the Yangtze and yellow rivers, China: optical properties, possible sources, and their relationships with environmental variables. Environ Sci Pollut Res. 2020;27(26):32856–32873. doi: 10.1007/s11356-020-09385-w
- Lyu L, Wen Z, Jacinthe P-A, et al. Absorption characteristics of CDOM in treated and non-treated urban lakes in Changchun, China. Environ Res. 2020;182:109084. doi: 10.1016/j.envres.2019.109084
- Yang B, Ljung K, Nielsen AB, et al. Impacts of long-term land use on terrestrial organic matter input to lakes based on lignin phenols in sediment records from a Swedish forest lake. Sci Total Environ. 2021;774:145517. doi: 10.1016/j.scitotenv.2021.145517
- An S, Chen F, Chen S, et al. In-lake processing counteracts the effect of allochthonous input on the composition of color dissolved organic matter in a deep lake. Sci Total Environ. 2023;856:158970. doi: 10.1016/j.scitotenv.2022.158970
- Jiang X, Liu D, Li J, et al. Eutrophication and salinization elevate the dissolved organic matter content in arid lakes. Environ Res. 2023;233:116471. doi: 10.1016/j.envres.2023.116471
- Ren H, Wang G, Ding W, et al. Response of dissolved organic matter (DOM) and microbial community to submerged macrophytes restoration in lakes: a review. Environ Res. 2023;231:116185. doi: 10.1016/j.envres.2023.116185
- Nardi S, Schiavon M, Francioso O. Chemical structure and biological activity of humic substances define their role as plant growth promoters. Molecules. 2021;26(8):2256. doi: 10.3390/molecules26082256
- Huaisong W, Rui G, Yibo T, et al. Arbuscular mycorrhizal fungi reduce NH3 emissions under different land-use types in agro-pastoral areas. pedosphere. 2023;34(2):497–507. doi: 10.1016/j.pedsph.2023.05.006
- Wan D, Kong Y, Wang X, et al. Effect of permanganate oxidation on the photoreactivity of dissolved organic matter for photodegradation of typical pharmaceuticals. Sci Total Environ. 2022;813:152647. doi: 10.1016/j.scitotenv.2021.152647
- Li J, Zhu D, Zhang S, et al. Application of the hydrochemistry, stable isotopes and MixSIAR model to identify nitrate sources and transformations in surface water and groundwater of an intensive agricultural karst wetland in Guilin, China. Ecotoxicol Environ Saf. 2022;231:113205. doi: 10.1016/j.ecoenv.2022.113205
- He D, Li P, He C, et al. Eutrophication and watershed characteristics shape changes in dissolved organic matter chemistry along two river-estuarine transects. Water Res. 2022;214:118196. doi: 10.1016/j.watres.2022.118196
- Xuan Y, Liu G, Zhang Y, et al. Factor affecting nitrate in a mixed land-use watershed of southern China based on dual nitrate isotopes, sources or transformations? J Hydrol. 2022;604:127220. doi: 10.1016/j.jhydrol.2021.127220
- Genxu W, Guodong C. Fluoride distribution in water and the governing factors of environment in arid north-west China. J Arid Environ. 2001;49(3):601–614. doi: 10.1006/jare.2001.0810
- Tian L, Zhu X, Wang L, et al. Distribution, occurrence mechanisms, and management of high fluoride levels in the water, sediment, and soil of Shahu Lake, China. Appl Geochem. 2021;126:104869. doi: 10.1016/j.apgeochem.2021.104869
- Dong G, Hu Z, Liu X, et al. Spatio-temporal variation of total nitrogen and ammonia nitrogen in the water source of the middle route of the south-to-north water diversion project. Water. 2020;12(9):2615. doi: 10.3390/w12092615
- Zhang H, Zhang S, Liu Y, et al. Effects of chloride on corrosion scale compositions and heavy metal release in drinking water distribution systems. J Hazard Mater. 2024a;465:133452. doi: 10.1016/j.jhazmat.2024.133452
- Borggaard OK, Holm PE, Strobel BW. Potential of dissolved organic matter (DOM) to extract As, Cd, Co, Cr, Cu, Ni, Pb and Zn from polluted soils: a review. Geoderma. 2019;343:235–246. doi: 10.1016/j.geoderma.2019.02.041
- Huang Y, Fu C, Li Z, et al. Effect of dissolved organic matters on adsorption and desorption behavior of heavy metals in a water-level-fluctuation zone of the Three Gorges Reservoir, China. Ecotoxicol Environ Saf. 2019;185:109695. doi: 10.1016/j.ecoenv.2019.109695
- Aftabtalab A, Rinklebe J, Shaheen SM, et al. Review on the interactions of arsenic, iron (oxy)(hydr) oxides, and dissolved organic matter in soils, sediments, and groundwater in a ternary system. Chemosphere. 2022;286:131790. doi: 10.1016/j.chemosphere.2021.131790
- Guo W, Yang H, Ma Y, et al. Comprehensive evaluation of the hydrological health evolution and its driving forces in the river-lake system. Ecol Inf. 2023;75:102117. doi: 10.1016/j.ecoinf.2023.102117
- Zhang K-F, Chang S, Tu X, et al. Heavy metals in centralized drinking water sources of the Yangtze River: a comprehensive study from a basin-wide perspective. J Hazard Mater. 2024b;469:133936. doi: 10.1016/j.jhazmat.2024.133936
- Zhang K, Chang S, Zhang Q, et al. Heavy metals in influent and effluent from 146 drinking water treatment plants across China: occurrence, explanatory factors, probabilistic health risk, and removal efficiency. J Hazard Mater. 2023;450:131003. doi: 10.1016/j.jhazmat.2023.131003
- Munger ZW, Carey CC, Gerling AB, et al. Effectiveness of hypolimnetic oxygenation for preventing accumulation of Fe and Mn in a drinking water reservoir. Water Res. 2016;106:1–14. doi: 10.1016/j.watres.2016.09.038
- Och LM, Müller B, Voegelin A, et al. New insights into the formation and burial of Fe/Mn accumulations in Lake Baikal sediments. Chem Geol. 2012;330:244–259. doi: 10.1016/j.chemgeo.2012.09.011
- Li J, Zhang C, Tan H, et al. Occurrence and influencing factors of high groundwater manganese in the oxbow lakes of the middle reaches of Yangtze River. J Hydrol. 2024;630:130713. doi: 10.1016/j.jhydrol.2024.130713
- Ayejoto DA, Egbueri JC. Human health risk assessment of nitrate and heavy metals in urban groundwater in Southeast Nigeria. Ecological Frontiers. 2024;44(1):60–72. doi: 10.1016/j.chnaes.2023.06.008
- Bi P, Liu R, Huang G, et al. Evaluating natural background levels of heavy metals in shallow groundwater of the pearl river delta via removal of contaminated groundwaters: comparison of three preselection related methods. Environ Pollut. 2023;335:122382. doi: 10.1016/j.envpol.2023.122382
- Chen Y, Zhang J, Liu J, et al. Predicting brown tide microalgae concentrations using reconstructed fluorescence spectroscopy combined with CNN. Microchem J. 2024;199:110071. doi: 10.1016/j.microc.2024.110071
- Saha P, Saikia KK, Kumar M, et al. Assessment of health risk and pollution load for heavy and toxic metal contamination from leachate in soil and groundwater in the vicinity of dumping site in Mid-Brahmaputra Valley, India. Total Environ Res Themes. 2023;8:100076. doi: 10.1016/j.totert.2023.100076
- Li Z, Huang M, Luo N, et al. Spectroscopic study of the effects of dissolved organic matter compositional changes on availability of cadmium in paddy soil under different water management practices. Chemosphere. 2019;225:414–423. doi: 10.1016/j.chemosphere.2019.03.059
- Zhao C, Gao S-J, Zhou L, et al. Dissolved organic matter in urban forestland soil and its interactions with typical heavy metals: a case of Daxing District, Beijing. Environ Sci Pollut Res. 2019;26(3):2960–2973. doi: 10.1007/s11356-018-3860-7
- Sun S, Konar M, Tang Q, et al. Tracing surface water pollution in China’s supply chain. J Hydrol. 2023;624:129960. doi: 10.1016/j.jhydrol.2023.129960
- Hossen MA, Mostafa M. Assessment of heavy metal pollution in surface water of Bangladesh. Environ Challenges. 2023;13:100783. doi: 10.1016/j.envc.2023.100783
- Ke Z, Tang J, Sun J, et al. Influence of watershed characteristics and human activities on the occurrence of organophosphate esters related to dissolved organic matter in estuarine surface water. Sci Total Environ. 2024;914:169956. doi: 10.1016/j.scitotenv.2024.169956
- Gao Y, Sturgeon RE, Mester Z, et al. On-line UV photochemical generation of volatile copper species and its analytical application. Microchem J. 2016;124:344–349. doi: 10.1016/j.microc.2015.09.020