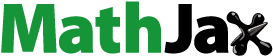
Abstract
In Ethiopia, chili (Capsicum annum L.) is an important dietary component. Chili is produced as a source of income for smallholder farmers covering a large share of the national as well as export market. A field experiment was conducted to study the association of green pod yield (FYD) and 11 yield-related traits in 29 chili genotypes under flood irrigation conditions in Ethiopia. The experiment was arranged in a randomized complete block design with three biological replicates. Phenotypic and genotypic correlations and path coefficients were analyzed for FYD and 11 yield-related traits. The genotypic and phenotypic correlation coefficients showed that all investigated traits, except days to 50% flowering and days to the first harvest, showed a positive and significant correlation with FYD. The phenotypic path coefficient analysis revealed that the number of flowers per plant (NFP, 0.4114), and fruit length (FL, 0.3678) showed a significant (p < 0.01) positive direct effect on FYD. The genotypic path coefficient showed a significant (p < 0.01) positive direct effect of NFP (0.7968), number of primary branches (0.3982), and FL (0.3982) on FYD. The traits with positive direct phenotypic and genetic effects on fruit yield indicate that improving these traits could increase fruit yield.
Introduction
Chili (Capsicum annum L.) is a diploid plant with a chromosome number of 2n = 24 belonging to the family Solanaceae (Kranthi Rekha Citation2015). The origin of chili is believed to be in Mexico with secondary centers in Guatemala and Bulgaria (Salvador Citation2002). It was introduced to Europe by Columbus in the fifteenth century and spread to the rest of the globe along the spice trading routes to Africa, India, China, and Japan (Kadwey et al. Citation2016).
In Ethiopia, chili is one of the most important vegetable cum spice crops valued for its aroma, taste, flavor, and pungency. Chili is an important dietary component for most Ethiopians that food without the presence of chili is tasteless (Mussema Citation2006). Cultivation of chili is the source of income for small farm holders, and it is covering a large share of the national as well as export market. Chili, in its powder form is a pungent product of indispensable uses; flavoring and and coloring ingredient in the common traditional sauce ‘Wot’, whereas the green pod is consumed as a vegetable with other food items. There is a general belief among Ethiopians that a person who frequently consumes hot pepper chili shall acquire/develop resistance against various diseases for fresh green chili is very rich in vitamin C (Kumar Citation2007; Swapan et al. Citation2017). The average daily consumption of hot pepper by an Ethiopian adult is estimated at 15 grams, which is higher than the consumption of tomatoes and most other vegetables (Delelegne et al. Citation2014).
The production of chili is increasing from time to time in many parts of Ethiopia (Gobie Citation2019; FAOSTAT Citation2020). According to FAOSTAT (Citation2020), the production of dry chilies and peppers in Ethiopia has increased from 158,546 tones in 2012–313,115.00 tones in 2019. However, productivity (yield) in Ethiopia is very low (17.94 quintals hectare−1) as compared to China (67.54 quintals hectare−1) for the year 2020 (FAOSTAT Citation2020).
Chili yield is a complex trait controlled by a large number of contributing characters and their interaction. It is not only influenced by several related characters which are governed by a few genes but is also influenced to a greater extent by the environment (Iqbal et al. Citation2015). In this regard, correlation coefficient analysis is helpful to select for yield using more than one character (Sharma et al. Citation2009). However, as yield is dependent on many component characters, the total correlation is insufficient to explain the true association between the characters (Jyothirmayi et al. Citation2008). Path coefficient analysis which gives a clearer picture of the relative magnitude of yield components is of paramount importance for an effective selection program. Path coefficient analysis help to sort out the total correlation into direct and indirect effects which is useful in selecting high-yielding genotypes (Vidya et al. Citation2018). Correlation and path coefficient analysis has been extensively used in crop breeding to determine the nature of associations between fruit yield and its contributing components and characterize the components with significant effects on yield to be used as selection standards (Aman et al. Citation2020). This field experiment was conducted to study the association of green pod yield and yield-related traits in chili genotypes in Ethiopia.
Materials and methods
Description of the study area
The study was carried out under flood irrigation conditions at Koga irrigation site of Adet Agricultural Research Center during the off-season period from December 2018 to June 2019. Koga irrigation site is located in Mecha District of West Gojjam Administrative Zone in Amhara Region about 540 km to the North of Addis Ababa, the capital of Ethiopia. Koga is located at 11.4°N latitude and 37°E longitudes, with an altitude of 1960m a.s.l, and receives a mean annual rainfall of 1480 mm. The mean maximum and minimum temperatures of the area are 26.5°C and 8.5°C, respectively. The topsoil of the experimental plots was heavy clay in texture with a pH of 4.5 (Denekew and Bekele Citation2009).
Experimental materials
For this study, four established chili varieties collected from Melkassa Agricultural Research Center (Table ) and twenty-five chili landraces collected from the Institute of Biodiversity Conservation and Research, Ethiopia (IBCR) (Table ) were used.
Table 1. The released chili varieties collected from Melkasa Agricultural Research center (MARC) and used in the study.
Table 2. A description of chili landraces/ accessions collected from the Ethiopian Institute of Biodiversity Conservation and Research (IBCR).
Experimental design
A Randomized Complete Block Design (RCBD) with three replications of twenty-nine chili genotypes were used for the experiment (Raghavarao Citation1983). In the study, chili seeds were sown on a seedbed of 1.5 × 8 m2 area. Seedlings were transplanted to the experimental plot after 60 days when the seedlings attained a height of 20-25 cm. Each experimental plot had an area of 8.4 m2 (2.8 m × 3 m) and contained 40 plants in four rows of ten plants each. The spacing between rows was 70 cm and between individual plants was 30 cm.
Data collection
The description of 12 different yield-related traits is given in Table . For the trait measurements, eight plants were randomly selected from the central rows of each plot and tagged/identified for data collection. Therefore, from the three replications, a total of 24 plants were measured for each trait in each genotype. The measurements were taken from those selected plants for all the characters.
Table 3. The chili traits measured and used for correlation and path coefficient analysis.
Data analysis
Correlation coefficient (r)
The correlation coefficients among all possible character combinations at phenotypic (rp) and genotypic (rg) levels were estimated employing the following formula (Al-Jibouri et al. Citation1958; Aman et al. Citation2020).
Where: rp and rg are phenotypic and genotypic correlation coefficients, respectively; pcov x·y and gcov x·y are phenotypic and genotypic covariances between variables x and y, respectively; δ2px and δ2gx are phenotypic and genotypic variances, respectively, for variable x; and δ2py and δ2gy are phenotypic and genotypic variances, respectively, for variable y.
The statistical significance of the correlation coefficient was tested using t-test as:
Where: n – number of treatments
Path coefficient analysis
Path coefficient analysis was estimated using the formula given below (Dewey and Lu Citation1959; Aman et al. Citation2020).
Where: rij is the association between independent character i (yield-related trait) and the dependent variable j (yield) as measured by the phenotypic and genotypic correlation coefficients; Pij refers to the components of direct effects of the independent character i on the dependent character j as measured by the path coefficients; ∑rik.Pjk refers to the summation of components of indirect effects of a given independent character i on a given dependent character j via all other independent characters k.
The contribution of the remaining unknown characters is measured as the residual as given by:
Results and Discussion
Phenotypic correlation
The phenotypic (rp) and genotypic (rg) correlations of 12 yield and yield-related traits of the 29 chili genotypes is presented in Table . The phenotypic correlation between FYD and other traits was positive and significant (at least p≤0.05), except with DFF, and DFH. FYD has the highest and significant phenotypic correlation with FPP (rp = 0.821; p≤0.01) followed by FL (rp = 0.768; p≤0.01). The positive and significant correlation of the traits with FYD implies the contribution of the traits for higher FYD productivity, and therefore, selection based on these traits could be considered for selective breeding. The result is in line with the work of other scholars (Sreelathakumary and Rajamony Citation2002; Usman et al. Citation2017) who observed that yield per plant revealed strong and highly significant associations with fruit length, fruit weight, number of fruits and plant height at harvest characters.
Table 4. Phenotypic (rp, above diagonal) and genotypic (gp, below diagonal) correlation coefficients of 12 yield and yield-related traits of 29 chili (Capsicum annum L.) genotypes from Ethiopia.
There was a positive and highly significant (rp = 0.955; p≤0.01) association between NFP and FPP which could be because of the direct association of the traits that most of the flowers mature to fruits (Sharma et al. Citation2010). The association between PB with SB (rp = 0.612) was also significant (p≤0.01). The NFP showed a significant positive association with FYD (rp = 0.821). This implies that plants bearing larger fruit number produce a higher yield. This is similar to previous reports (Ben-Chaim and Paran Citation2000; Rohini and Lakshmanan Citation2015). PH showed a positive significant (p≤0.01) correlation with FL, DFF, and FD. It is natural that taller plants require a longer time to mature, and produce longer and larger fruits.
However, significant negative correlations were also observed between DFH and NFP (rp = −0.427; p≤0.01), DFH and FPP (rp = −0.463; p≤0.01), FL and NFP (rp = −0.387; p≤0.01), FL and FPP (rp = −0.392; p≤0.01), FD and NFP (rp = −0.301; p≤0.01), SL and NFP (rp = −0.410; p≤0.01), SL and FPP (rp = −0.398; p≤0.01), FTP and NFP (rp = −0.307; p≤0.01) which implies that selection programs using these traits as a selection critiera requires caution.
Genotypic correlation
The observation on genotypic correlation coefficients showed that all the traits examined in this study are positively and significantly correlated with FYD, except DFF (rg = −0.227) and DFH (rg = −0.188). Traits that showed high genotypic correlations with FYD are NFP (rg = 0.636; p≤0.01), PB (rg = 0.541; p≤0.01), FPP (rg = 0.409; p≤0.01), and FL (rg = 0.404; p≤0.01). Similar findings were reported by Naik (Citation2008), who observed that the correlation of chili yield was significant (p≤0.01) and positive with the number of fruits per plant (0.725), fruit length (0.60), pericarp thickness (0.388), number of primary branches (0.357) and number of secondary branches (0.846).
The genotypic correlation coefficient revealed a positive and significant (p≤0.01) association of PH with DFF (rg = 0.360), FL (rg = 0.547), and SL (rg = 0.515). These findings were in line with Ukkund et al. (Citation2007), who observed that plant height has a positive and significant association with fruit length (0.452), stalk length (0.654), fruit number per plant (0.452), and fruit yield per plant (0.260). FL had a positively significant (p < 0.01) correlation with PH (rg = 0.547), SL (rg = 0.774), and FYD (rg = 0.404). This indicated that DFF, FL, and SL could be improved by improving plant height. The genotypic correlations were higher than the phenotypic correlation in most of the cases indicating the high heritable nature of the characters. Similarly, Rana et al. (Citation2015) reported that the magnitude of genotypic correlation coefficients, in general, was higher than the phenotypic correlation coefficients for traits studied on chili genotypes. Farwah et al. (Citation2020), reported a high estimate of the heritability of chili characters.
The FPP had a positive and significant correlation with NFP (rg = 0.972), FYD (rg = 0.409), and FPT (rg = 0.361). Correlation studies by Sharma et al. (Citation2009), showed that selection for harvest duration, the number of chili fruits per plant, fruit diameter, and fruit pericarp thickness will be effective for selecting plants with higher fruit yield. FL had a positive and significant correlation with PH (rg = 0.547), SL (rg = 0.774), and FYD (rg = 0.404). This indicates that selection for traits such as plant height and stalk length will improve fruit length.
FD had a positive and significant genetic correlation with FPT (rg = 0.503), SL (0.443), and FYD (rg = 0.309). This result revealed that selection based on FPT, SL, and FYD will be effective for screening plants with higher fruit diameters. The FPT had a positive and significant genetic correlation with FD (rg = 0.503), FPP (rg = 0.361), NFP (rg = 0.351), and FYD (rg = 0.292). Similar findings were reported elsewhere by Ibrahim et al. (Citation2001), that fruit pericarp thickness had a positive and significant correlation with fruit diameter and number of fruit per plant.
Path-coefficient analysis of fruit yield with other quantitative traits
Phenotypic path analysis
The results of phenotypic path coefficient analysis in Table revealed that all the characters studied had a positive direct effect on FYD except DFF, DFH, FPP, and SL. The highest direct positive effect on FYD was shown by the NFP (0.4114; rp = 0.8208, p≤0.01), followed by FL (0.3678; rp = 0.7682, p < 0.01). This implies that a higher NFP and FL lead to an increased FYD of chili. Similar findings were reported by Usman et al. (Citation2017) that fruit diameter, fruit pericarp thickness, and fruit length had a positive direct effect on fruit yield. Mishra et al. (Citation2002), also reported a high direct and positive correlation of fruit length and number of fruit per plant with fruit yield. The direct effect of these characters on fruit yield indicates that an improvement in these traits will increase fruit yield.
Table 5. Estimates of phenotypic direct effects (bold and diagonal) and indirect effects (off-diagonal) of traits via other independent traits on fresh fruit yield of 29 chili (Capsicum annum L.) genotypes.
In contrast, a negative direct effect was observed for DFF (−0.2492) and DFH (−0.1632), like their phenotypic correlations (−0.0269 and −0.0886, respectively). The direct effect of PL (0.0007) on FYD was small. However, it could have a cumulative effect through its indirect effect on the traits like FL, FPP, and FD. Therefore, combining plant height with other traits as a selection criterion may end up with high fruit-yielding genotypes.
FL, FD, and SL had a negative indirect effect through all characters except the number of fruit per plant and fruit pericarp thickness. The residual effect (0.286) indicates that all other characters which are not included in this study have a negative direct effect on yield or the traits included in the present investigation accounted for most of the variation (99.7%) present in the dependent variable that is fruit yield per plant.
Genotypic path analysis
The results of genotypic path coefficient analysis for direct and indirect effects (Table ) revealed that all the characters studied exerted positive direct effects on fruit yield, except DFF, DFH, and FPP. The NFP exerted the highest positive direct effect (0.7969; rg = 0.636, p ≤ 0.01) on FYD. The PB (0.3983, rg = 0.5408, p < 0.01), FL (0.3083, rg = 0.4041, p < 0.01) and PH (0.1035, rg = 0.3242, p < 0.5) has also a direct effect on FYD. In contrast, FPP (−0.9568, rg = 0.4092, p < 0.01), and DFF (−0.7370) had a negative direct genetic effect on FYD.
Table 6. Estimates of genotypic direct effects (bold and diagonal) and indirect effects (off-diagonal) of traits via other independent traits on fresh fruit yield of 29 chili (Capsicum annum L.) genotypes.
Similar findings were reported previously for the positive direct effect of traits except for days to 50% flowering, days to first harvest, the number of fruit per plant, and fruit diameter (Rohini and Lakshmanan Citation2015; Shumbulo et al. Citation2017). Other scholars (Ben-Chaim and Paran Citation2000; Srilakshmi Citation2006) reported a negative direct effect of days to 50% flowering and fruit diameter on fruit yield, and positive direct effects were reported for plant height, number of primary branches, number of the secondary branches, and fruit length. On the other hand, PB (0.256), SB (0.0057), DFH (0.0978), FD (0.0156), FPT (0.0119), and DFF (0.3757) exerted positive indirect effects. These results were in line with the finding of Aklilu et al. (Citation2016).
The higher positive direct effect of NFP on FYD implies that NFP can be used as either a direct or indirect selection criterion to improve fruit yield. Selecting the tallest plants and those with many branches, without considering other traits may lead to accessions with reduced fruit yield. Whereas selecting plants with a larger number of flowers bearing many branches will increase fruit yield. The residual (0.2136) indicated that characters included in these genotypic path analyses explained 99.79% of the total variation in fruit yield or all other characters which are not included in this study have a negative direct effect on fruit yield.
Conclusions
To improve chili fruit yield, selection considering yield-related traits is very important. In this study, genotypic and phenotypic correlations followed a similar trend for all the characters studied. The genotypic correlations were higher than the phenotypic correlation in most of the cases indicating the high heritable nature of the characters implying its importance in selective breeding. Fruit yield had a significant and positive genotypic association with plant height, number of primary branches, number of secondary branches, fruit diameter, fruit length, stalk length, number of flowers per plant, fruit pericarp thickness, and number of fruits per plant.
Genotypic path analysis for fruit yield revealed that the number of primary branches, number of secondary branches, number of flowers per plant, fruit length, stalk length, fruit pericarp thickness, and plant height had a positive direct effect on fruit yield. The phenotypic path for fruit yield revealed that plant height, number of primary branches, number of secondary branches, number of flowers per plant, fruit length, fruit diameter, and fruit pericarp thickness had a positive direct effect on fruit yield. Whereas days to 50 percent flowering, days to the first harvest, number of fruit per plant, and stalk length showed a negative direct effect on fruit yield. Generally, the traits with positive direct phenotypic and genetic effects on fruit yield indicate that the improvement of these traits could increase fruit yield.
Disclosure statement
No potential conflict of interest was reported by the author(s).
Data availability statement
The authors confirm that the data supporting the findings of this study are available within the article [and/or] its supplementary materials. The data can be accessed using the link: http://www.doi.org/10.11922/sciencedb.01406.
References
- Aklilu S, Abebie B, Wogari D. 2016. Genetic variability and association of characters in Ethiopian hot pepper (Capsicum annum L.) landraces. Journal of Agricultural Sciences, Belgrade. 61(1):19–36.
- Al-Jibouri H_A, Miller PA, Robinson HF. 1958. Genotypic and environmental variances and covariances in an upland Cotton cross of interspecific origin 1. Agron J. 50(10):633–636. doi:10.2134/agronj1958.00021962005000100020x.
- Aman J, Bantte K, Alamerew S, Sbhatu DB. 2020. Correlation and path coefficient analysis of yield and yield components of quality protein maize (Zea mays L.) hybrids at Jimma, western Ethiopia. International Journal of Agronomy. 2020:7. doi:10.1155/2020/9651537.
- Ben-Chaim A, Paran I. 2000. Genetic analysis of quantitative traits in pepper (Capsicum annuum). J Am Soc Hortic Sci. 125(1):66–70.
- Delelegne S, Belew D, Mohammed A, Getachew Y. 2014. Evaluation of elite Hot pepper varieties (Capsicum spp.) for growth, Dry Pod yield and quality under jimma condition, south west Ethiopia. International Journal of Agricultural Research. 9:364–374.
- Denekew A, Bekele S. 2009. Characterization and atlas of the Blue Nile basin and its sub basins. Improved water and land management in the Ethiopian highlands: its impact on downstream stakeholders dependent on the Blue Nile. intermediate results dissemination, workshop held at the International livestock Research Institute (ILRI), Addis Ababa, Ethiopia, 5-6 February 2009.
- Dewey DR, Lu KH. 1959. A Correlation and Path-Coefficient Analysis of Components of Crested Wheatgrass Seed Production. Agron J. 51(9):515–518. doi:10.2134/agronj1959.00021962005100090002x.
- FAOSTAT. 2020. http://www.fao.org/faostat/en/#data/QC/visualize.
- Farwah S, Hussain K, Rizvi S, Hussain SM, Rashid M, Saleem S. 2020. Genetic variability, heritability and genetic advance studies in chilli (Capsicum annuum L.) genotypes. IJCS. 8(3):1328–1331.
- Gobie W. 2019. A seminar review on red pepper (Capsicum) production and marketing in Ethiopia. Cogent Food & Agriculture. 5(1):1647593.
- Ibrahim M, Ganiger VM, Yenjerappa ST. 2001. Genetic variability, heritability, genetic advance and correlation studies in chilli. Karnataka Journal of Agricultural Sciences. 14(3):784–787.
- Iqbal S, Tak HI, Inam A, Inam A, Sahay S, Chalkoo S. 2015. Comparative effect of wastewater and groundwater irrigation along with nitrogenous fertilizer on growth, photosynthesis and productivity of Chilli (Capsicum annuum L.). J Plant Nutr. 38(7):1006–1021. doi:10.1080/01904167.2014.991032.
- Jyothirmayi T, Rao GN, Rao DG. 2008. Physicochemical changes during processing and storage of green chili (Capsicum annuum) powders. J Food Process Preserv. 32(5):868–880. doi:10.1111/j.1745-4549.2008.00219.x.
- Kadwey S, Dadiga A, Prajapati S. 2016. Genotypes performance and genetic variability studies in Hot chilli (Capsicum annum L.). Indian J Agric Res. 50(1):56–60.
- Kranthi Rekha G. 2015. Development of hybrids and their stability in chilli (Capsicum annuum L.) [Ph. D Thesis]. Dr. YSR Horticultural University, Venkataramannagudem, Andhra … .
- Kumar, HD Mohan. 2007. Genetic variability, correlation, morphological and molecular diversity in byadgi kaddi and byadgi dabbi chillies (capsicum annuum L.) accessions.” UAS, Dharwad.
- Mishra AC, Singh RV, Ram HH. 2002. Path coefficient analysis in sweet pepper (Capsicum annuum L.) genotypes under mid hills of uttaranchal. Vegetable Science. 29(1):71–74.
- Mussema R. 2006. Analysis of red pepper marketing: the case of alaba and siltie in SNNPRS of Ethiopia. Haramaya University, Dire Dawa, Ethiopia.
- Naik, V Rudra. 2008. “Assessment of genetic diversity in local chilli collections (Capsicum annuum L.)."MSc, UAS, Dharwad.
- Raghavarao D. 1983. Statistical techniques in agricultural and biological research: Oxford & IBH Pub.
- Rana M, Sharma R, Sharma P, Kumar S, Kumar D, Dogra RK. 2015. Correlation and path-coefficient analysis for yield and its contributing traits in capsicum, Capsicum annuum L. International Journal of Farm Sciences. 5(2):66–73.
- Rohini N, Lakshmanan V. 2015. Correlation and path coefficient analysis in chilli for yield and yield attributing traits. Journal of Applied and Natural Sciences. 4:25–32.
- Salvador MH. 2002. Genetic resources of chilli (Capsicum spp.) in Mexico. Proc. Of the 16th Int. pepper conf., tampico, tamaulips, Mexico.
- Sharma VK, Semwal CS, Uniyal SP. 2010. Genetic variability and character association analysis in bell pepper (Capsicum annuum L.). Journal of Horticulture and Forestry. 2(3):58–65.
- Sharma VK, Semwal CS, Unlyal SP. 2009. Genetic variability and character association analysis in bell pepper under rainfed mid hills situation of uttarakhand. Annals of Horticulture. 2(2):177–183.
- Shumbulo A, Nigussie M, Alamerew S. 2017. Correlation and path coefficient analysis of hot pepper (Capsicum annuum L.) genotypes for yield and its components in Ethiopia. Adv Crop Sci Tech. 5(3):1–5. doi:10.4172/2329-8863.1000277.
- Sreelathakumary I, Rajamony L. 2002. Variability, heritability and correlation studies in chilli (Capsicum spp.) under shade. Indian Journal of Horticulture. 59(1):77–83.
- Srilakshmi P. 2006. Genetic diversity for productivity and quality characters in chilli (Capsicum annuum L.).” UAS, Dharwad.
- Swapan C, Mominul Islam AKM, Aminul Islam AKM. 2017. Nutritional benefits and pharmaceutical potentialities of chili: a review. Fundamental and Applied Agriculture. 2(2):227–232.
- Ukkund KC, Patil MP, Madalageri MB, Ravindra M, Jagadeesh RC. 2007. Character association and path analysis studies in Green chilli for yield and yield attributes (Capsicum annuum L.). Karnataka Journal of Agricultural Sciences. 20(1):99–101.
- Usman MG, Rafii MY, Martini MY, Oladosu Y, Kashiani P. 2017. Genotypic character relationship and phenotypic path coefficient analysis in chili pepper genotypes grown under tropical condition. J Sci Food Agric. 97(4):1164–1171.
- Vidya C, Jagtap VS, Santhosh N. 2018. Correlation and path coefficient analysis for yield and yield attributing characters in chilli (Capsicum annum L.) genotypes. Int J Curr Microbiol Appl Sci. 7(1):3265–3268. doi:10.20546/ijcmas.2018.701.390.